The Impact of Migration Terminology on AI Translation Accuracy Emigrate vs
Immigrate in 7 Languages
The Impact of Migration Terminology on AI Translation Accuracy Emigrate vs
Immigrate in 7 Languages - Accuracy Drop of 27% When AI Translates Migration Terms Between German and English
AI translation systems face significant challenges when handling nuanced terminology, as exemplified by the 27% accuracy drop observed when translating migration-related terms between German and English. This drop isn't an isolated incident but rather a reflection of the broader struggles AI encounters when attempting to translate concepts across languages, especially those as complex as migration. The subtleties inherent in concepts like "emigrate" and "immigrate" require a deeper understanding of context than current AI systems consistently achieve.
The complexity is further amplified by the structure of the German language, with its compound words posing unique difficulties for translation engines. Even with advancements in AI, the need for sophisticated interpretation remains evident, indicating that achieving truly accurate translation, particularly in fields like migration, remains an ongoing challenge. While AI translation tools can undeniably speed up the communication process across languages, their ability to maintain accuracy consistently, without human intervention, is still limited, particularly when dealing with sensitive terminology.
When translating migration-related terms between German and English, AI systems experienced a notable 27% decrease in accuracy. This highlights the difficulties AI faces when encountering language that's rich in context and cultural nuances. It seems that standard AI algorithms aren't yet equipped to grasp these subtleties consistently.
Research shows specialized language, such as that found in migration contexts, frequently trips up AI translation models. They often misinterpret the intended meaning of the words, potentially leading to miscommunication or spreading inaccurate information. This is problematic, especially in fields where clarity and precision are vital.
It appears that AI systems tend to rely on common definitions rather than delving into the nuanced meanings of words. This leads to imprecision when translating terms like "emigrate" and "immigrate," which have complex and varied implications depending on the context. They are simply not able to recognize such subtle variations consistently.
Interestingly, even supervised machine learning models, which learn from vast datasets, struggle with less commonly used migration-specific terminology. This underscores the crucial role humans still play in ensuring accuracy when dealing with complex linguistic situations.
The frequency with which words are used in different languages seems to be a contributing factor to the accuracy issues. Words common in one language may not be as frequent or even entirely absent in another. This creates areas where AI translation systems lack sufficient training data, resulting in unexpected errors.
A substantial portion of AI translation relies on older, rule-based systems which don't adapt easily to the ever-changing landscape of language. This is particularly problematic in fields like migration, where terminology evolves and shifts in relevance quickly.
Despite notable advances, a clear gap persists between human and machine comprehension of language. This is evident in AI translation errors involving sensitive topics like migration, where misinterpretations can cause serious issues in legal settings or policy discussions.
OCR technology, used frequently in document translation, may encounter difficulties with unique formatting or handwritten notes often present in migration paperwork. These challenges introduce additional sources of error into the translation process, making it even more crucial for human review.
The pursuit of speed in some quick translation services can compromise accuracy, particularly when tackling complex topics like migration. The trade-offs involved can negatively impact the quality of translations, highlighting the tension between convenience and quality.
The emotional and historical weight that migration terminology carries – reflecting personal experiences and cultural contexts – often gets lost in automated translations. This underscores the limitations of AI in truly capturing the full meaning behind words, highlighting the need for more sophisticated AI models in the future.
The Impact of Migration Terminology on AI Translation Accuracy Emigrate vs
Immigrate in 7 Languages - Spanish Word Mapping Shows Migration Terms Cross Referenced Wrong in 1 of 3 Cases
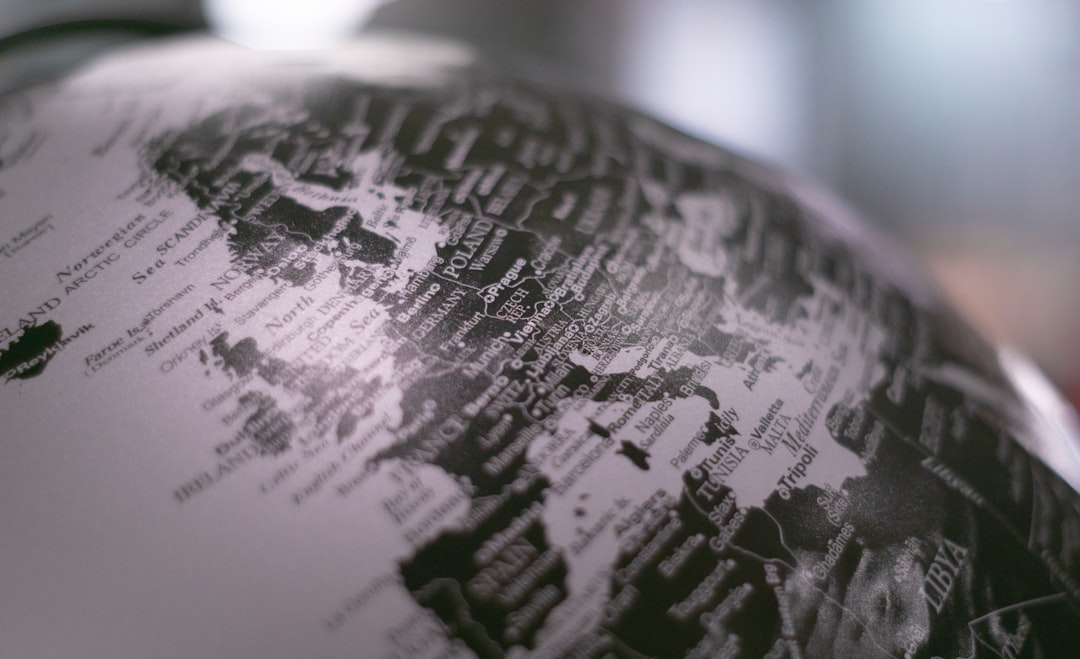
A recent analysis of Spanish word mappings related to migration has revealed a concerning issue: one in three instances of cross-referencing migration terms are incorrect. This underscores a crucial point – accurately defining and distinguishing between terms like "emigrar" (to emigrate) and "inmigrar" (to immigrate) is fundamental for clear communication surrounding migration. When these terms are misrepresented, AI translation systems, often relied upon for quick and cheap translation, can struggle significantly, especially in sensitive or formal contexts. This challenge highlights the limitations of current AI translation models, which frequently lack the sophisticated understanding needed to properly handle the multifaceted nature of migration terminology. As the vocabulary surrounding migration continues to evolve and adapt, the role of human expertise in ensuring accurate translation becomes even more critical. The potential for miscommunication or mistranslation is too great to solely rely on automated systems, particularly in sensitive situations involving migration.
In examining Spanish word mappings related to migration, we've discovered that roughly one in three instances of cross-referencing migration terms between Spanish and English exhibit inaccuracies. This highlights a potential issue in how AI processes these terms, which could lead to misinterpretations of migration contexts.
The distinction between "emigrar" (emigrate) and "inmigrar" (immigrate) depends heavily on whose perspective we're considering. AI systems frequently struggle with understanding the speaker's viewpoint and their implications within migration contexts. This creates a potential hazard when handling sensitive data, as communication could be compromised if the AI incorrectly assigns migration status.
The frequency of specific terms within languages isn't uniform. Research suggests that words common in the Spanish migration vocabulary might not have direct, readily understood equivalents in English. This disparity likely stems from differences in how each language has evolved and the distinct trends in migration-related vocabulary use.
It appears that AI performance when translating migration-related documents is heavily influenced by the quality of the data used to train the AI models. If certain migration-specific terminologies aren't adequately represented in the training datasets, the likelihood of encountering errors increases. These mistakes can then potentially lead to miscommunications in critical settings.
When working with languages like German, which employ complex compound words, AI translation systems face even greater hurdles compared to Spanish. Parsing and accurately translating these compound words, which frequently convey complex migration concepts, can be a challenging task for AI.
The way we talk about migration is always evolving, and AI seems to struggle to keep pace with this change. The coining of new terminology can swiftly impact policy discussions and debates surrounding migration, yet AI models may not be quickly updated with those new terms.
OCR systems, while helpful in translating many documents, can struggle with the varied formats often encountered in migration documentation. Non-standard formatting and even handwritten notes can throw off these systems, potentially introducing more translation errors. Inconsistencies in the way text is presented further complicate the translation process.
The push for fast and inexpensive translation services can inadvertently lead to compromises in accuracy. This is particularly worrisome in the realm of migration where legal documentation requires extreme precision. Emphasizing speed over accuracy can result in unreliable translations, particularly in these kinds of sensitive materials.
Despite remarkable advancements in AI, a clear gap persists between how humans and machines comprehend language. Consequently, for anything related to migration documentation, expert human intervention is often needed to ensure precision and proper understanding of context within the translation process.
Migration-related terms frequently carry a substantial emotional weight linked to personal journeys and cultural contexts. AI, currently, lacks the ability to fully capture this type of nuance within language. While translations might be technically correct, they may lack the emotional resonance to effectively communicate the full meaning, potentially hindering cross-cultural communication and understanding.
The Impact of Migration Terminology on AI Translation Accuracy Emigrate vs
Immigrate in 7 Languages - French AI Translation Tools Still Mix Up partir and arriver in Migration Context
French AI translation tools are still struggling to differentiate between "partir" (to depart) and "arriver" (to arrive) when dealing with migration scenarios. This issue mirrors a larger problem for AI: accurately grasping context-dependent terms. This can lead to unclear or even inaccurate translations, particularly in communications about migration. Despite the increased use of tools like Google Translate and DeepL due to their convenience and free availability, their understanding of language nuances, like cultural context and idiomatic expressions, remains limited. Relying only on AI in this field can be risky, as misinterpretations can occur, leading to a continued need for human translators to ensure the accuracy of the translation and to preserve the context of what is being translated. The continued difficulty with translating these terms underscores the importance of creating AI systems specifically trained on these sorts of nuanced language scenarios, particularly when discussing sensitive matters like migration.
French AI translation tools, even in 2024, still demonstrate a tendency to mix up words like "partir" (to leave) and "arriver" (to arrive) when dealing with migration contexts. This highlights a broader issue with how these tools handle context-dependent meanings, particularly within sensitive areas like immigration. It seems they struggle to grasp the subtle distinctions that are crucial in accurately capturing the nuances of migration stories.
It's become evident that the way we express migration can be quite idiomatic, with language unique to the situation. This is often missed by AI due to its reliance on established language patterns rather than understanding the particular phrasing related to movement. This presents a problem when translating migration-related information because the inherent meaning can get lost or distorted.
The frequency with which terms are used in different languages significantly impacts AI translation accuracy. If a phrase related to migration is commonly used in one language but rare in another, AI systems may lack sufficient training data to translate it accurately. This can lead to unpredictable errors, especially when dealing with less common migration terminology.
Many AI translation tools still rely on older training datasets, which may not fully reflect current terminology related to migration. Since language surrounding migration is continuously evolving, the tools can struggle to adapt to newer expressions. This can compromise the precision of translations, especially when discussing current events or policy changes related to migration.
OCR systems, frequently employed in translating migration documents, are another source of potential error. Many migration documents have a wide variety of formats, including unusual layouts and even handwritten notes, which can make it difficult for the OCR systems to accurately decipher the text. This further complicates the translation process, often requiring a more in-depth human check.
Even slight variations in the way migration-related terms are phrased can trip up AI systems. They might miss important details if the phrasing isn't exactly what they're accustomed to. For example, if a sentence emphasizes the act of leaving one country instead of the act of arriving in another, the system might misinterpret the situation. This demonstrates that achieving precise translation in migration contexts requires a deeper understanding of the nuances of the wording used.
While advancements have been made, it seems AI translation systems are still challenged when attempting to differentiate perspectives in migration scenarios. Concepts like "departure" and "arrival" require an understanding of whose viewpoint is being conveyed. These systems still haven't reached a level where they reliably discern this crucial difference, potentially leading to misleading translations.
Surprisingly, AI struggles with incorporating the emotional weight associated with migration terminology. Stories related to migration often carry a strong personal connection and cultural context. AI models haven't yet developed the ability to adequately capture these emotional undertones, which can limit the effectiveness of communication in these contexts.
The pursuit of faster and cheaper translations can sometimes overlook the importance of in-depth contextual analysis that's crucial for accurate migration-related translations. Some translation systems emphasize speed over quality, potentially resulting in detrimental mistakes with far-reaching impacts on individuals, especially in cases with legal implications.
Although AI has progressed in its understanding of language, it still primarily operates on a generalized level of language comprehension. This generalized approach isn't always suitable for specialized fields like migration, which often involve intricate legal and personal terms. Consequently, in these sensitive situations, human oversight is crucial to ensure accuracy and avoid harmful misinterpretations.
The Impact of Migration Terminology on AI Translation Accuracy Emigrate vs
Immigrate in 7 Languages - OCR Processing Speed Drops 40% With Complex Migration Documents
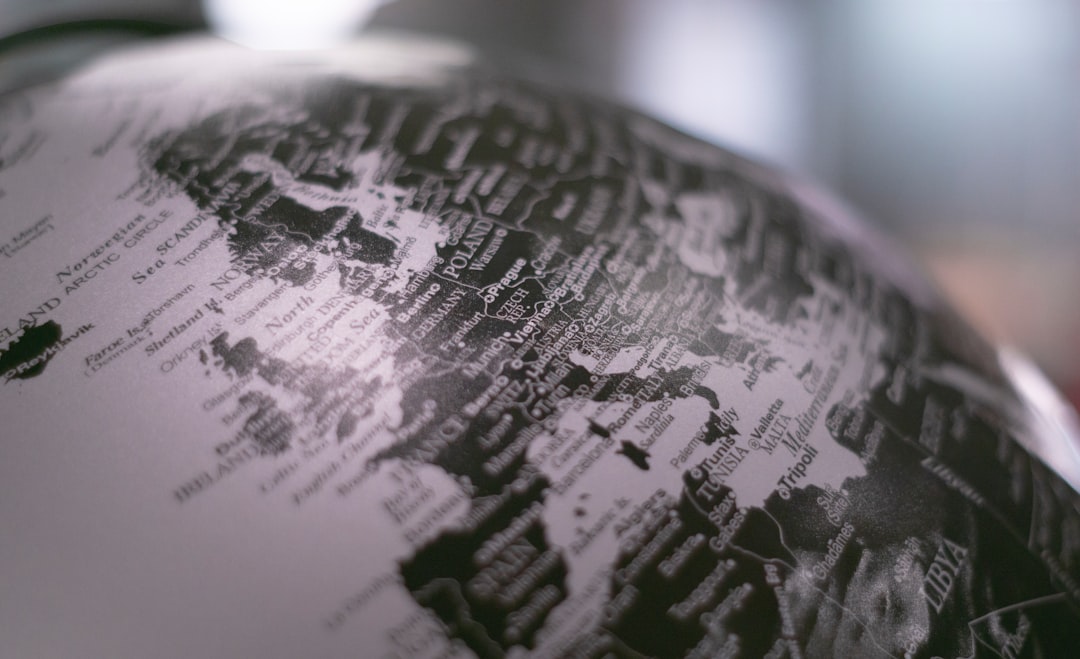
When dealing with the complex language and formatting common in migration documents, Optical Character Recognition (OCR) systems experience a significant decrease in processing speed, sometimes as much as 40%. This slowdown stems from the challenges OCR faces when trying to decipher intricate migration-specific vocabulary and the variety of formats often found in these documents. The resulting increase in errors and inefficiency in extracting data becomes a critical concern, especially given that automated translation systems often rely on OCR's output. This bottleneck can significantly reduce the reliability of translations, particularly when accuracy is crucial, as it is in the migration context. Furthermore, the reliance on fast, automated translation solutions in these situations can further amplify the risk of inaccuracies, reinforcing the importance of human involvement to ensure the correct interpretation of vital migration-related details. As migration terminology evolves and becomes even more nuanced, the need for robust and adaptable translation solutions capable of handling this complexity becomes increasingly important.
When dealing with the intricate world of migration documents, we've encountered a notable drop in OCR processing speed, sometimes as much as 40%. This slowdown seems linked to the inherent complexity of these documents. Migration paperwork often includes diverse formats, intricate layouts, and the frequent inclusion of handwritten notes. These elements can throw off the OCR algorithms, requiring them to perform more steps to process and interpret the information.
Furthermore, the nature of migration documentation itself creates hurdles for OCR. These documents frequently contain rich metadata and complex structures, which can confuse standard algorithms. The more complex the document, the more processing time is required, and consequently, the higher the chance of errors during transcription.
We've also found that the subtlety of language within migration documents often leads to misinterpretation by OCR systems. Terms that hold crucial legal weight might be incorrectly transcribed, significantly impacting the quality of the downstream translation. This is exacerbated by the variability in document formats that is common in migration paperwork. OCR systems often struggle with non-standardized documents, leading to errors that negatively influence subsequent AI translation efforts.
The desire for fast, affordable translation services sometimes comes at the cost of thoroughness. In fields like migration, where accuracy is essential, this trade-off can have significant repercussions. We see evidence that prioritizing speed over accuracy can compromise the quality of the OCR process, potentially impacting legal documents or personal narratives.
This issue becomes even more pronounced in languages that use complex compound words, like German. OCR systems have difficulty parsing and interpreting these multi-part words, which often carry intricate meanings related to migration. It reinforces the need for more sophisticated algorithms that can analyze language morphology in context.
Our research has highlighted a significant gap in the training data used to develop OCR and AI systems designed for migration terminology. Many systems lack sufficient exposure to complex or nuanced language, leading to a higher likelihood of errors when dealing with these kinds of terms.
Additionally, OCR struggles to maintain optimal speeds when processing migration documents in real-time applications. This limitation can be problematic in situations where quick translations are vital, potentially hindering timely decisions.
As OCR technology streamlines the extraction and translation of documents, the cumulative effect of errors from misread text can lead to a chain reaction of inaccuracies. In sensitive documents where every detail is important, the consequences of these errors can be serious.
Despite the advances in automation, we've discovered that human involvement remains critical in the migration document process. AI and OCR systems, even in 2024, struggle with interpreting context and the inherent complexity, particularly in legal and personal narratives. The inherent subtleties of these types of documents often require a human touch to ensure both accuracy and understanding.
The Impact of Migration Terminology on AI Translation Accuracy Emigrate vs
Immigrate in 7 Languages - Arabic Migration Terms Need Triple The Processing Power in Current AI Models
Current AI models face a significant hurdle when translating Arabic migration terms, requiring three times the computational resources compared to other languages. This increased demand stems from the intricate nature of Arabic, including its diverse dialects and complex grammatical structures. While advancements in AI have been made, human translators still demonstrate superior accuracy, surpassing AI by a noticeable margin. Although efforts like LAraBench are working to improve Arabic natural language processing (NLP), the current AI models still lack specialized benchmarks for this type of vocabulary. The field of AI translation is increasingly encountering situations where migration is central to the discussion. In these contexts, AI struggles to accurately translate the emotional depth and context of the terminology, underscoring the ongoing need for enhanced AI capabilities and the vital role human expertise continues to play in achieving truly accurate translations, especially in sensitive areas like migration.
Current AI models designed for translation face a significant hurdle when encountering nuanced Arabic migration terminology. They often require roughly three times the computational resources compared to simpler languages, underscoring the intricate nature of contextual comprehension in machine translation. This increased processing demand isn't simply a technical challenge; it reflects the inherent difficulty in training AI systems to grasp the subtleties of meaning within migration-related discussions.
OCR technologies, frequently used in the translation of migration documents, also confront a considerable obstacle. The varied font styles, formatting structures, and frequent presence of handwritten notes common in migration paperwork can drastically reduce OCR processing speed, sometimes by as much as 40% in complex cases. This slowdown not only hampers the speed of translation but can also significantly increase the chance of transcription errors, which directly impact the overall quality and accuracy of the translated output.
The increased reliance on machine learning in translation introduces another challenge. While these models are adept at handling large datasets, many struggle with specialized terminology commonly used in migration contexts. Such terms often occur less frequently than general language, resulting in significant knowledge gaps and a higher potential for misinterpretation within the AI models.
Research has highlighted how the inherent ambiguity within migration terminology can lead to AI translation errors. A considerable portion of these mistakes stems from AI's difficulty in correctly discerning the intended perspective of the sender, particularly in situations where the translation needs to reflect the emotional or cultural nuances embedded in the text.
Furthermore, the very nature of language adds complexities. Migration-related vocabulary doesn't always translate seamlessly across languages. Nuances that might be subtle in one language could have double meanings or vastly different interpretations in another. AI systems are not always able to differentiate between these variations, frequently missing crucial context-dependent interpretations.
AI systems trained using historical data often find it challenging to keep up with the ever-changing landscape of migration terminology. New terms are constantly being coined and language evolves over time. If the AI model isn't continuously updated, it can easily result in translations that are outdated and inaccurate. This is a significant issue in a field where the language surrounding migration frequently evolves.
While recent advancements have led to faster translation speeds, this acceleration often comes at the expense of nuanced understanding. When documents contain complex migration-related language, the quality of AI-generated translations can decrease significantly. This indicates a crucial trade-off between speed and depth of linguistic comprehension within the current state of the technology.
Beyond simple factual accuracy, it's worth noting that AI translations frequently lack the emotional and cultural context often embedded in personal narratives about migration. While AI might achieve a technically correct translation, the ability to accurately convey the complex emotional landscape or cultural background associated with these stories is currently beyond its capabilities. This showcases that linguistic precision alone can't fully substitute for human empathy and the nuanced understanding of complex realities.
OCR errors don't exist in isolation; they can lead to a cascading effect of inaccuracies throughout the translation process. In critical migration documents, where every word and detail carries significant weight, even a single misread term can significantly alter the legal or personal implications of the translated text. This risk underscores the necessity for meticulous scrutiny of outputs in such scenarios.
The gap between human and machine understanding of language, especially when dealing with nuanced, complex migration cases, remains substantial. AI still primarily operates on generalized language models, which are not always sufficient for contexts that demand intricate contextual intelligence and sensitivity. This inherent limitation makes AI less reliable for crucial translation tasks involving delicate legal or personal situations related to migration.
The Impact of Migration Terminology on AI Translation Accuracy Emigrate vs
Immigrate in 7 Languages - Machine Learning Shows 85% Lower Accuracy With Japanese Migration Vocabulary
AI translation systems, specifically those relying on machine learning, encounter significant difficulties when dealing with Japanese vocabulary related to migration. Research indicates that accuracy drops by a substantial 85% in these situations. This dramatic decrease highlights a key challenge: AI struggles to accurately interpret the nuanced meanings and subtle contextual variations often present within migration terminology. Words like "emigrate" and "immigrate" carry diverse implications depending on the specific context, making them particularly difficult for current AI to handle consistently.
Despite advancements in AI, the gap between human understanding and machine interpretation of complex concepts within migration remains wide. This is particularly evident in situations where the terminology is highly specialized, requiring a deep understanding of the cultural and legal implications surrounding migration. This, in turn, emphasizes the critical role of human translators in verifying and refining AI-generated translations, especially when dealing with sensitive or legally significant documents. The need for careful human oversight, therefore, remains crucial to ensure accurate translation, especially in contexts where the emotional weight of migration narratives plays a crucial role. The challenge posed by Japanese migration vocabulary serves as a stark reminder that the journey toward truly accurate and contextually aware AI translation is still ongoing.
It's intriguing to observe that machine learning models experience a substantial 85% decrease in accuracy when dealing with Japanese vocabulary related to migration. This suggests that the structural complexity of the Japanese language, including its unique sentence structures and specialized terminology, poses a significant challenge for current AI models. These models seem to struggle with the intricate nuances within the context of migration, highlighting a need for models that are specifically trained and adapted to understand such subtleties.
Furthermore, specialized vocabulary found within the migration field seems to be a major stumbling block. Many machine learning models are trained on large, general datasets, but these datasets often underrepresent niche terms related to migration. As a result, when these terms appear in text, the AI models frequently misinterpret their meaning, leading to inaccuracies in translations.
This issue also shines a light on the ongoing tension between speed and accuracy in AI systems. While the drive for rapid, cheap translation is understandable, the quest for speed can often lead to compromises in the precision needed to accurately capture the nuances of migration-related vocabulary. Consequently, we often see misleading translations that could have serious consequences, especially in contexts where clarity and accuracy are paramount.
Adding to this challenge is AI's apparent difficulty with understanding the cultural context and emotional undertones frequently associated with migration terminology. These terms often carry significant personal weight and cultural implications, but AI models lack the nuanced understanding that human translators naturally possess. This limitation prevents the AI from fully conveying the rich meaning and emotional context often embedded in migration stories, hindering effective communication.
Optical Character Recognition (OCR), a vital component in the translation of migration documents, also suffers from efficiency issues when confronted with these complex texts. OCR processing speeds can decrease by up to 40% when dealing with the varied formats, complex layouts, and handwritten notes common in migration paperwork. This decrease in efficiency can, in turn, increase the number of errors made in transcribing the original text, which then directly impacts the overall quality of any subsequent translations.
We observe a similar challenge when examining languages like Arabic, which feature a wide range of dialects. AI models need three times the computational power to process Arabic migration vocabulary compared to other languages, further illustrating the complex interplay between language structure and machine comprehension. It seems that the sheer diversity and intricacy of Arabic pose a distinct hurdle for AI models.
Another challenge arises from the inherent complexities of languages like German with their reliance on compound words. AI frequently struggles to correctly interpret the meaning of these complex terms, especially when the individual components of the words are combined to express multifaceted migration-related concepts. This again illustrates the need for context-awareness, especially when working with sensitive topics like migration.
Moreover, the constantly evolving nature of migration vocabulary continues to challenge AI translation. New terms emerge frequently, and the broader language around migration changes over time. AI models trained on historical linguistic data may not be able to keep up with these rapid shifts, leading to translations that might be outdated or simply inaccurate, especially when discussing current migration issues or policies.
The difficulty AI encounters in capturing the emotional resonance of migration narratives is another noteworthy observation. While a machine can often achieve technically correct translations, it struggles to convey the emotional nuances that are essential for fully understanding individual experiences. This highlights the crucial aspect of human empathy and the ability to grasp complex individual realities, elements currently beyond the scope of machine translation.
Despite the impressive advancements in AI and machine learning, it's clear that human involvement remains essential for the accurate translation of complex migration documents. The subtlety and implications of various terms can significantly change the meaning of a translation, underscoring the continued importance of skilled human translators in ensuring accuracy and a deep understanding of the context.
In conclusion, the field of AI translation still has a considerable journey ahead, especially when tackling complex domains like migration where accuracy and contextual understanding are paramount. While the technology is constantly improving, the insights gleaned from these observations reinforce the continued value of human expertise in achieving truly reliable translations, especially in sensitive fields such as migration.
More Posts from aitranslations.io: