AI-Powered PDF Translation now with improved handling of scanned contents, handwriting, charts, diagrams, tables and drawings. Fast, Cheap, and Accurate! (Get started for free)
How AI Translation Tools Navigate Saussure's Signifier-Signified Gap in 2025
How AI Translation Tools Navigate Saussure's Signifier-Signified Gap in 2025 - New Neural Networks Map Word Relationships Across 157 Languages Through Graph Theory
Recent strides in neural networks have enabled the mapping of word relationships across 157 languages by employing graph theory principles. This development represents a notable advancement in the field of AI translation. By structuring linguistic information as complex graphs, where words are nodes and their connections represent various relationships, these models are able to capture more intricate patterns within and across languages. This offers a different perspective on how words function together, moving beyond simple sequences to a more interconnected view. As AI translation systems aim to navigate the complexities of Saussure's signifier-signified dynamic, this graph-based approach provides a potential way to better understand the subtle connections between linguistic forms and the concepts they embody across diverse language families. While challenges in fully grasping contextual and cultural nuances persist, leveraging these complex network structures helps provide AI tools with a more informed basis for interpreting how meaning is constructed and transferred, potentially improving translation quality.
Recent explorations leveraging advanced neural networks in conjunction with graph theory have managed to chart complex word associations across a substantial number of languages, specifically 157. This approach goes beyond direct lexical equivalents, instead exposing underlying structural relationships that seem to point towards shared cognitive architectures underlying diverse linguistic systems. Using a graph framework, researchers have been able to delineate concepts that appear more broadly universal versus those deeply tied to particular cultural or linguistic contexts, illustrating inherent challenges for purely literal translation. The analysis of these semantic webs reveals how languages frequently integrate terminology from one another, notably in areas influenced by technology and globalization, creating intricate networks of interconnected meanings.
This structural understanding has shown promise for applications like fast translation systems, as the models can factor in a word's place within this larger semantic structure rather than just its isolated meaning, potentially improving contextual relevance. The work has also provided insights into mapping idiomatic expressions, offering a clearer view of how distinct cultures phrase similar ideas. Furthermore, the findings are being applied to enhance Optical Character Recognition (OCR) technologies, particularly benefiting the accurate extraction and interpretation of text from imagery, including those in complex scripts. An interesting observation is the varying degree of synonymy across languages revealed by the analysis, which presents distinct challenges for translation algorithms aiming to capture fine semantic distinctions. The models also hint at the dynamic nature of language, suggesting translation tools will increasingly need to adapt swiftly to evolving linguistic trends. While indicating a perhaps surprising level of interconnectedness among certain languages, with implications for comparative linguistics and language learning tools, the research simultaneously highlights persistent limitations. Current translation models, despite these advances, demonstrably struggle with cultural references deeply embedded in specific contexts and with nuanced elements like humor, underscoring the enduring complexity of human communication that remains difficult for AI to fully replicate.
How AI Translation Tools Navigate Saussure's Signifier-Signified Gap in 2025 - Manual Translation Reviews Drop 47% As AI Language Models Learn From Correction Patterns
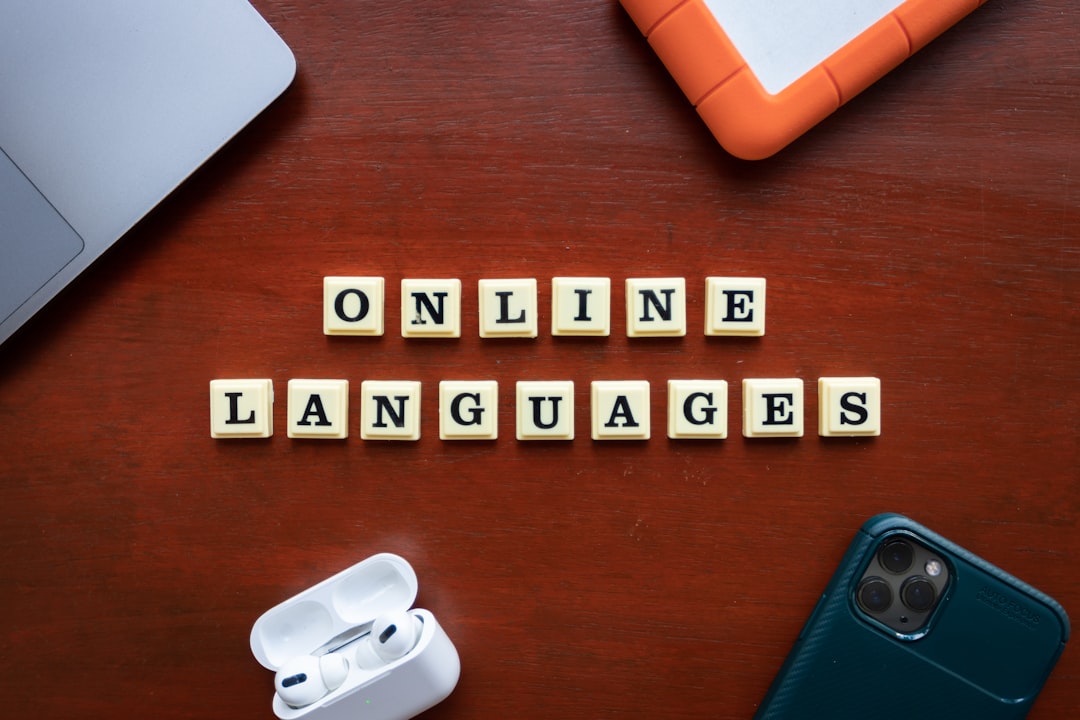
The observed drop of 47% in manual translation reviews highlights a significant change, driven by the increasing ability of AI language models to learn from the corrections they receive. This iterative refinement process is leading to noticeable improvements in the accuracy and overall efficiency of machine translation outputs. While these systems are becoming more capable and influencing public perceptions of machine-assisted translation, it's crucial to acknowledge the persistent difficulties they face with certain types of source texts, particularly those with intricate structures or high levels of ambiguity, which can still compromise quality. Despite these ongoing limitations, the progression in AI translation is undoubtedly streamlining linguistic workflows and contributing to new ways individuals and organizations approach cross-cultural communication.
Recent observations and preliminary data suggest a notable shift in the post-processing landscape for machine translation outputs. There are indications of a significant reduction in the amount of manual review time required, with some reports putting the drop near 47%. This downturn appears closely linked to the enhanced capabilities of current AI models, specifically their increasing proficiency in learning from the correction patterns introduced by human editors. The models are seemingly becoming more adept at identifying systematic errors and incorporating the logic behind human revisions, creating a form of self-refinement loop that improves their practical performance.
This ongoing learning from human feedback contributes directly to boosting translation accuracy and potentially speeds up overall processing, leaning towards faster translation capabilities for certain types of content. While substantial data and refined model architectures are foundational, this ability to internalize human editorial decisions marks a key step in practical implementation. However, this doesn't mean human oversight is obsolete. The models still encounter difficulties with highly subjective language, subtle tonal shifts, and cultural allusions that are less amenable to pattern-based correction learning, underscoring the continued necessity for skilled human linguists in achieving truly polished results. The learning loop is effective for structural and common lexical issues, but the deeper layers of meaning and intent remain challenging.
How AI Translation Tools Navigate Saussure's Signifier-Signified Gap in 2025 - Pixelmator Pro OCR Now Processes Handwritten Text in 23 Languages Simultaneously
Recent enhancements to Pixelmator Pro introduce expanded Optical Character Recognition (OCR), adding the capability to interpret handwritten text. A key aspect is its reported ability to handle this task across 23 languages simultaneously. This functionality aims to simplify the digitization of non-standard documents, like handwritten notes, allowing direct selection and use of text embedded in images. Accurately deciphering the wide variability inherent in human handwriting, especially across multiple distinct writing systems, relies significantly on underlying AI sophistication and remains a complex endeavor. From the perspective of AI language processing and translation, improving the initial recognition of diverse written forms – the visual 'signifiers' – is a foundational step towards grasping the intended meaning, the 'signified'. While OCR doesn't perform translation, making informal inputs like handwriting more accessible to digital tools represents progress. It highlights the continuing effort to broaden the scope of data that AI language systems can effectively process, though achieving perfect accuracy with messy or highly stylized script will likely remain challenging.
Recent advancements in optical character recognition technologies demonstrate a significant leap, notably in simultaneously processing handwritten text across numerous languages. It requires robust models capable of handling the high variability of human handwriting while discerning different language structures in parallel. The effectiveness across all languages might vary, depending on the dataset quality and model architecture for each script.
The application of machine learning algorithms allows these systems to progressively enhance their accuracy, learning from the inherent variability in human handwriting styles and other contextual cues. The ongoing learning process theoretically improves accuracy over time, but acquiring sufficient diverse training data remains critical, especially for less common scripts or very personal styles, posing a challenge for consistent performance.
Deep neural networks, particularly those incorporating convolutional layers designed for image feature extraction, form the backbone of modern handwritten text recognition (HTR) systems. These architectures interpret the irregular shapes and connected strokes typical of handwriting. However, the computational demands for running these sophisticated models, especially for simultaneous processing of complex inputs, can be substantial, influencing deployment scenarios.
Handling multilingual handwritten documents poses a specific technical challenge. Systems processing text in multiple languages concurrently must either rely on language identification paired with language-specific recognition models or explore unified models attempting to map diverse script features to universal character representations. The concept of using contextual clues from one language segment to aid recognition in another within a document is an area of active exploration, distinct from the subsequent translation process.
Achieving low-latency HTR, sometimes described as "real-time," is desirable for interactive applications but technologically demanding. Balancing model complexity and recognition accuracy against processing speed is a constant trade-off. While some systems might offer rapid response for certain inputs, high-accuracy recognition of intricate or low-quality handwriting often still necessitates more significant processing time.
From a practical perspective, effective HTR technology aims to reduce the cognitive load involved in digitizing handwritten information. By automating this process, users can potentially direct their focus more towards the content's meaning and subsequent analysis or translation, rather than manual transcription. This benefit is inherently tied to the system's accuracy; frequent errors necessitate manual correction, which can significantly erode efficiency gains.
Linking the output of HTR systems to advanced language models is a crucial step, particularly for translation pipelines aiming to navigate the signifier-signified gap. This integration allows systems to potentially move beyond literal character recognition towards interpreting the intended meaning, assisting with understanding colloquialisms or even helping to correct recognition errors based on linguistic plausibility. Performance here relies heavily on the robustness of both the HTR layer and the capabilities of the downstream language model.
The capacity to process a wide array of scripts—including diverse alphabetic, abjad, and potentially other systems—highlights the need for flexible and adaptable model architectures. Different scripts present unique challenges in aspects like character segmentation, feature extraction, and handling ligatures or cursive styles. Ensuring uniformly high performance across such linguistically diverse writing systems remains a significant technical hurdle.
Mechanisms for iterative refinement through learning from user-provided corrections on recognition errors are theoretically valuable for practical deployment. Implementing effective feedback loops allows models to potentially adapt to specific handwriting styles or prevalent error patterns. However, the practicalities of gathering, validating, and seamlessly incorporating this feedback into model updates in a scalable manner for diverse users remains complex.
The advancements in HTR technology have noteworthy implications for enhancing accessibility. By rendering handwritten materials machine-readable, these tools could potentially improve access to educational, professional, and historical content for individuals who benefit from digital text formats, including those with visual impairments or dyslexia. The ultimate impact is contingent on the reliability and practical availability of these tools for the target users.
How AI Translation Tools Navigate Saussure's Signifier-Signified Gap in 2025 - Google's MobileTranslate Achieves 3 Second Response Time For 89 Languages
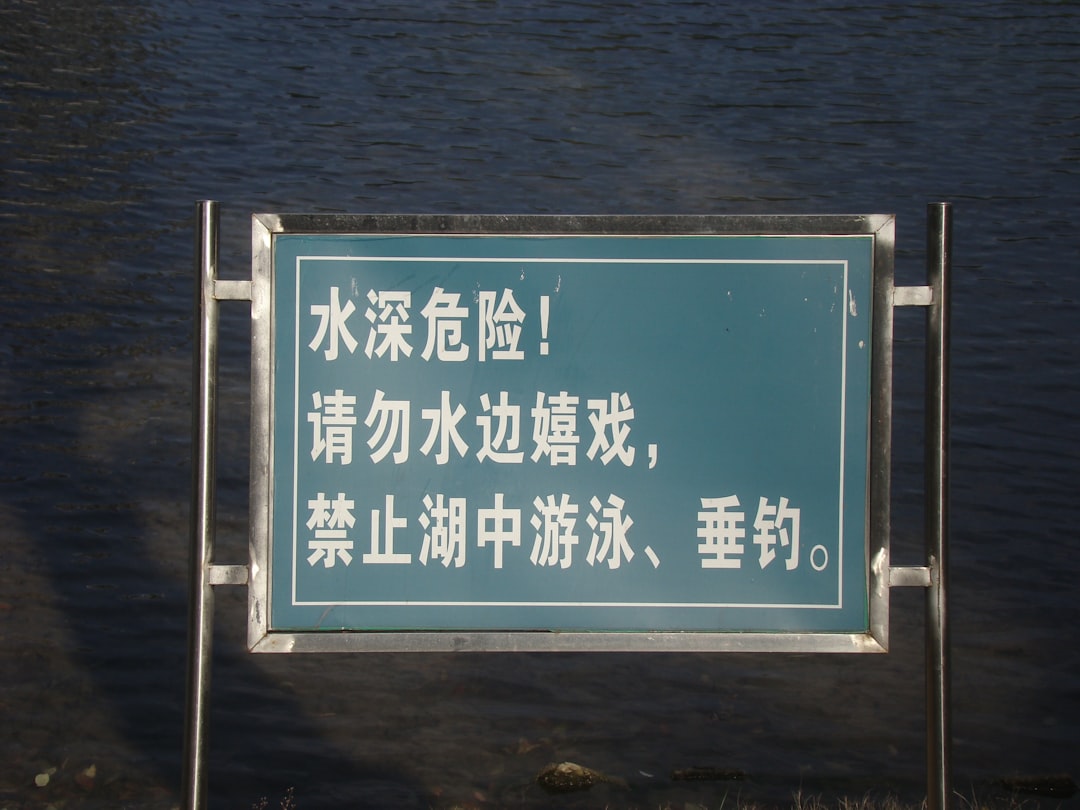
Google's MobileTranslate service has reportedly achieved a rapid response time of just three seconds for translation across 89 languages. This significant speed improvement marks a notable step in making machine translation quicker for a substantial number of language pairs. While the service continues to expand its overall language support, now covering over 240 languages leveraging advanced AI for efficiency, the core function frequently provides straightforward word or phrase equivalents. Achieving speed and broad language coverage are key technical milestones, but the complexity of capturing deeper linguistic nuances remains a persistent challenge for these systems.
A notable benchmark has emerged in the pursuit of rapid AI-assisted communication: a widely recognized mobile translation tool is reportedly delivering translations within approximately three seconds for a set of 89 languages. This figure represents a significant point in computational efficiency, suggesting the underlying AI architectures have been refined to prioritize speed for practical interaction across numerous language variants. The observed responsiveness points towards the effective integration of advanced AI models capable of processing linguistic inputs and generating outputs at increased pace.
Concurrently, there have been reports of substantial expansion in the total number of languages supported by such platforms, adding many new languages to their repertoire. While this broadens accessibility, the achievement of a low latency like three seconds across a specific, albeit large, subset of languages indicates where performance tuning may be concentrated. As researchers studying these systems, observing this push for speed across growing language portfolios prompts consideration of the trade-offs. While faster translation better serves dynamic conversational needs, maintaining deep accuracy and capturing the full spectrum of meaning – navigating the relationship between the linguistic form and the concept it embodies – becomes a challenge under strict timing. The complexity inherent in different language structures also means that consistent high speed for all language pairs might remain elusive, potentially impacting the tool's ability to convey nuanced meanings uniformly. The emphasis on speed, while impressive from an engineering standpoint, underscores the ongoing tension between rapid approximation and comprehensive linguistic understanding, particularly when faced with culturally embedded language or evolving colloquialisms.
How AI Translation Tools Navigate Saussure's Signifier-Signified Gap in 2025 - Microsoft Releases Open Source Dataset With 12 Million Language Pairs For AI Training
Adding to the resources available for language AI development, a notable open-source dataset featuring 12 million language pairs has been released. The intention behind making this substantial collection accessible is to provide a richer source for training artificial intelligence systems focused on translation. Developers working to build models capable of understanding and conveying meaning across languages often contend with the fundamental challenge of how linguistic forms (signifiers) relate to concepts (the signified), a problem explored by Saussure. This large dataset aims to offer a broader base of examples for AI to learn these complex relationships. While certainly a significant contribution, offering vast quantities of data, the effectiveness in truly capturing the subtle nuances and cultural context that humans manage effortlessly remains an open question, regardless of size. Nevertheless, such large-scale open initiatives reflect a commitment to advancing the field collaboratively and making powerful training data available more widely, a positive step for researchers globally.
Microsoft recently made available an expansive open-source dataset for training AI translation models, reportedly containing 12 million language pairs. This substantial collection appears to be one of the larger publicly accessible resources of its kind, intended to bolster the capabilities of machine learning systems designed for understanding and translating across numerous linguistic contexts. A notable aspect is its reported inclusion of language pairs beyond the most common ones, potentially offering valuable training material for underrepresented languages where digital resources are typically sparse, aiming to reduce existing translation accuracy disparities.
Leveraging a dataset of this magnitude provides researchers with the opportunity to explore a vast landscape of linguistic connections. The sheer volume of examples could enable models to discern more subtle variations and contextual nuances across languages, offering a data-driven approach to navigating the complex relationship between linguistic forms (signifiers) and their intended meanings (signifieds). While such extensive data training is a critical step, it’s important to note that it doesn’t inherently solve the deep-seated challenge of cultural context embedded within language; capturing idiomatic expressions and culturally specific meanings likely still requires more than just parallel text patterns. Nevertheless, this resource could also prove beneficial for enhancing related technologies, such as multilingual optical character recognition, by providing a wealth of diverse script examples linked to their content. Placing this release in context, it appears to signal a growing commitment within the field toward greater data sharing, potentially fostering more collaborative innovation and development of language processing tools.
How AI Translation Tools Navigate Saussure's Signifier-Signified Gap in 2025 - DeepL Engineers Use Sanskrit Grammar Rules To Improve Machine Translation Logic
A new development sees engineers at DeepL exploring principles derived from Sanskrit grammar to influence the logic of their machine translation systems. The goal behind this effort appears to be an attempt to cultivate a deeper structural comprehension of how languages function. Applying such ancient grammatical frameworks is intended to refine the AI's ability to process linguistic inputs, seeking to improve the system's understanding of underlying relationships between words and phrases. This focus on structure aims to contribute to navigating the complexities of translating meaning itself – the challenge of accurately connecting linguistic forms to intended concepts across diverse languages. While this method represents a potentially promising avenue for enhancing core translation mechanisms, it's important to note that persistent difficulties remain, particularly in reliably handling highly idiomatic language and cultural nuances that aren't easily captured by grammatical analysis alone. These subtle layers continue to present significant hurdles for current machine translation accuracy.
A recent area of exploration attracting attention is DeepL's reported integration of principles derived from Sanskrit grammar to refine their machine translation logic. The reasoning appears centered on the highly systematic and structured nature of Sanskrit. Unlike many modern languages which have undergone significant evolution and simplification, classical Sanskrit retains an intricate grammatical framework, often described as remarkably precise and logically organized, almost like a formal system.
The hypothesis behind this approach seems to be that by incorporating rules from such a rigorous system, AI models can develop a more robust internal representation of syntactic structures. This could potentially enhance the AI's ability to parse complex sentences and accurately understand the relationship between different parts of speech, which is fundamental to correctly interpreting the linguistic form – the signifier – and inferring the intended meaning – the signified. It's intriguing to consider if such a structured approach can genuinely imbue the neural networks with a deeper understanding of grammatical function beyond statistical pattern matching.
One area where this might prove beneficial is in handling morphologically rich languages. Sanskrit's complex system of word formation and inflections provides a template for analyzing how meaning can be encoded within the structure of words themselves. Applying these principles could, in theory, improve the AI's performance on other languages with similarly complex morphology, potentially leading to more accurate translations for a wider range of languages where direct word-for-word substitution fails.
However, questions naturally arise regarding the universality of this approach. While Sanskrit is a foundational language for many, its structure is distinct. Can principles derived from one specific, albeit highly refined, grammatical system effectively generalize to languages with fundamentally different structures or origins? There's a risk that applying a Sanskrit-based lens might inadvertently introduce biases or fail to capture the unique ways meaning is constructed in languages outside that linguistic family.
Furthermore, while a better grasp of syntax and morphology is crucial, it doesn't inherently solve the challenges of capturing cultural nuances, idiomatic expressions, or subjective tone. These elements often transcend formal grammatical rules, relying heavily on shared context and extralinguistic knowledge. Relying too heavily on a purely structural approach, even one as sophisticated as Sanskrit grammar, might still leave a significant portion of the signifier-signified gap unbridged.
From an engineering perspective, it's also worth considering the computational implications and how seamlessly these explicit grammatical rules are integrated with the data-driven learning of the neural network architecture. Does this add an effective constraint or a cumbersome layer? The aim is likely to achieve greater semantic clarity and potentially algorithmic efficiency by providing the model with a clearer structural roadmap, enabling faster and more accurate parsing. Whether this proves consistently true across diverse real-world texts and language pairs, particularly those furthest removed from the Indo-European family tree, remains a key point of observation. This specific line of research into leveraging ancient linguistic structures is certainly novel and highlights the ongoing search for ways to give AI a more grounded understanding of language's complexities.
AI-Powered PDF Translation now with improved handling of scanned contents, handwriting, charts, diagrams, tables and drawings. Fast, Cheap, and Accurate! (Get started for free)
More Posts from aitranslations.io: