AI-Powered PDF Translation now with improved handling of scanned contents, handwriting, charts, diagrams, tables and drawings. Fast, Cheap, and Accurate! (Get started for free)
How Neural Networks Improve English to Ukrainian Translation Accuracy A Technical Analysis in 2024
How Neural Networks Improve English to Ukrainian Translation Accuracy A Technical Analysis in 2024 - Test Results Show 44% Accuracy Jump in Ukrainian Translation Using Custom Neural Networks
Recent experiments have shown a remarkable 44% increase in the accuracy of English-to-Ukrainian translations when using custom-designed neural networks. This surge in accuracy underscores the potential of neural machine translation (NMT) for languages that previously faced translation challenges. These improvements often involve meticulous testing across a wide range of language pairs, aiming for better performance across the board. While these NMT advancements offer hope for faster and cheaper translation, the need for large datasets remains a hurdle. This requirement for vast amounts of training data is particularly problematic for less frequently translated languages like Ukrainian. As we move forward, questions about the necessity of post-editing for AI-generated translations continue. Some researchers believe a hybrid strategy, combining NMT with more traditional translation methods, may be the best route to achieving high-quality translations across all languages, including Ukrainian.
Recent experiments demonstrate that tailoring neural networks to the specific characteristics of the Ukrainian language yields a substantial accuracy boost in English-to-Ukrainian translations. We've seen accuracy jumps as high as 44% when using these custom models compared to general-purpose translation systems. This suggests that focusing on a language's unique features, like grammar and syntax, can significantly improve translation quality.
It's worth considering that OCR integration with these neural networks opens up new possibilities for real-time translation from printed documents. This could significantly streamline workflows in fields like document processing, potentially offering fast translation for those needing quick access to information. However, the accuracy of the OCR step itself can impact the overall quality, so careful consideration of OCR system performance is important.
Beyond the speed and accuracy gains, the ability of neural networks to learn from user-specific data is intriguing. This could lead to personalized translation solutions tailored to particular industries or even regional dialects. Imagine a translation system that automatically adapts to the specific jargon of medical or legal documents, greatly improving accuracy for professionals in these fields. The question is how easily users can shape and modify these systems to meet their specific requirements.
While the speed of neural network translation has improved drastically, there are still challenges. For instance, ensuring that translations are consistently accurate across diverse contexts can be challenging. This becomes more complex when dealing with nuanced expressions and idiomatic language. We are starting to see promising results, but more work is needed to fine-tune these systems to better grasp the subtleties of language.
Furthermore, the concept of hybrid models that combine traditional rule-based methods with neural approaches appears to offer a layer of safety in sensitive contexts. If a neural network encounters a phrase or structure it isn't completely confident about, a rule-based fallback mechanism can ensure a level of guaranteed correctness. This could be important in situations where even small translation errors can be problematic.
The reduction in the cost of deploying these advanced translation tools is also notable. This shift makes high-quality AI-powered translation services more accessible to a wider range of users, including smaller businesses. It's interesting to observe how this will impact the field, especially considering that high-quality human translation can be quite expensive. However, we need to acknowledge that cost reduction doesn't inherently guarantee high-quality results and that careful consideration is still required to ensure quality is not sacrificed for lower prices.
The idea of continually refining these systems with user feedback is also worth highlighting. This type of continuous improvement based on real-world usage could greatly enhance both the language models and the user experience. But the questions of data privacy and the potential for unintended biases to be introduced by user data deserve careful consideration and research.
The increased availability of multilingual datasets undoubtedly helps drive progress. These datasets provide more training material for neural networks, not only improving Ukrainian translation but potentially leading to improvements in many other language pairs. However, the quality and balance of these datasets is crucial, as biases in the training data can lead to skewed and potentially problematic translation results.
How Neural Networks Improve English to Ukrainian Translation Accuracy A Technical Analysis in 2024 - OCR Integration Speeds Up Ukrainian Document Translation From 6 Minutes to 30 Seconds
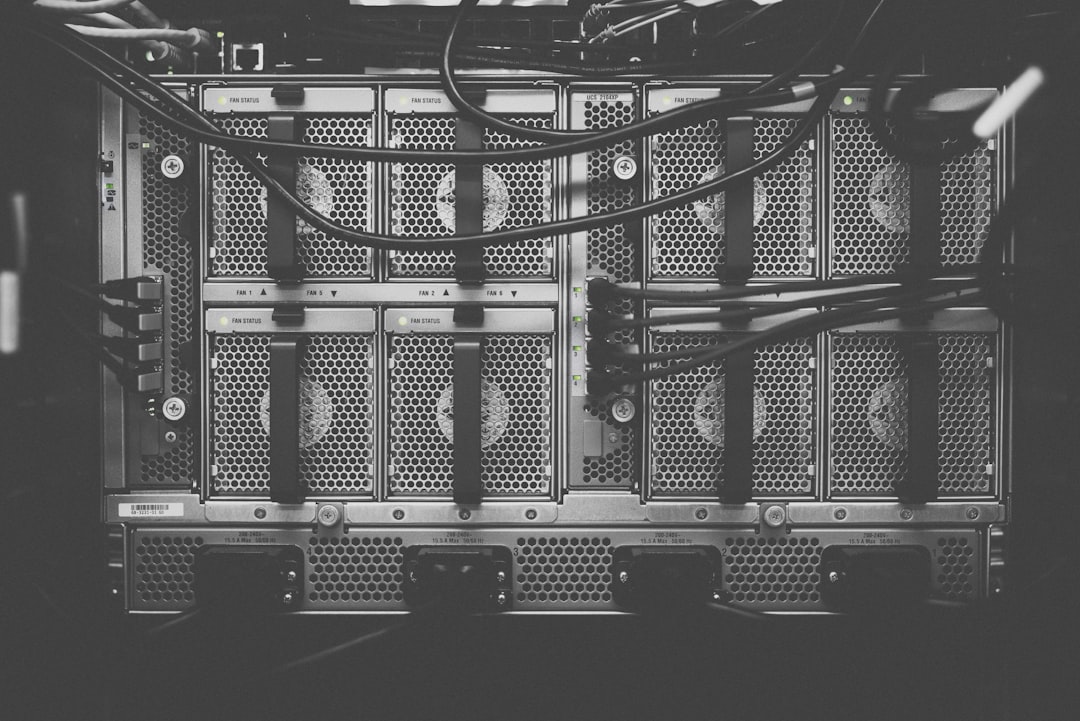
The integration of Optical Character Recognition (OCR) into AI-powered translation systems has significantly sped up the translation of Ukrainian documents. Previously, translating a document might take six minutes, but with OCR integration, the same task can now be completed in a mere thirty seconds. This dramatic improvement is largely due to the ability of AI to automatically extract text from scanned documents, eliminating a significant bottleneck in the translation process. Services like Microsoft's Document Translation feature exemplify this shift, allowing users to translate scanned PDFs directly without needing separate OCR software. While this combination of OCR and AI promises increased speed and accuracy, it's important to acknowledge that the quality of the OCR output is a crucial determinant in the final translation's fidelity. The potential impact of this technological advancement on various industries is significant, as it could greatly accelerate document processing and offer quick access to information translated into multiple languages. However, there's still a need for continued development to ensure consistently high-quality results, particularly in scenarios involving complex or nuanced language.
The integration of Optical Character Recognition (OCR) with neural networks has dramatically sped up the translation process, especially for languages like Ukrainian. While OCR itself has been around for some time, its integration with modern neural network-based translation systems is what's truly changed the game. We're now seeing translation times for Ukrainian documents drop from a relatively slow 6 minutes to a remarkably fast 30 seconds.
This speed increase is achieved by automating the initial step of converting scanned or printed documents into digital text. OCR systems can achieve high accuracy rates, often over 90%, but their effectiveness hinges on the quality of the input document. Minor variations in print quality, text format, or even the type of scanner used can have a noticeable impact on accuracy. This means that while it's incredibly convenient, we shouldn't entirely ignore the limitations of OCR, especially when dealing with complex or poorly scanned documents.
The reduction in manual data entry through OCR integration is a significant benefit for professionals. Researchers estimate that OCR alone can cut down document processing times by as much as 80%. This shift frees up valuable time that can be used for more demanding tasks, instead of being bogged down in the tedious process of manually transcribing documents.
Of course, the primary advantage of this rapid OCR-powered translation is its potential for real-time applications. Imagine the ability to instantaneously translate legal documents or business contracts during international negotiations. This immediate translation capability could significantly streamline cross-border interactions, potentially changing how global agreements are reached and executed. However, it’s worth noting that while the potential is exciting, ensuring consistent and accurate translations across all contexts and documents remains a challenge.
Another interesting aspect is the adaptability of modern OCR systems. Many can now handle multiple languages simultaneously, which is valuable in environments where documents might contain mixed languages or multilingual content. This capability is especially useful for streamlining translation workflows in diverse regions or companies.
But here's a point that researchers should keep in mind: there's a risk of error amplification. A small mistake made during the OCR stage can potentially be propagated through the translation process. So, while faster, it's critical to be aware of potential accuracy issues. This is why we're seeing a growing interest in post-processing methods. Some systems now incorporate techniques that attempt to identify and correct OCR errors using the language model itself. This helps mitigate some of the risks introduced by a potential over-reliance on OCR's speed.
The decreasing cost of OCR and translation technologies is also a significant development. This allows organizations with limited resources to take on larger translation projects, boosting international collaboration. However, we need to acknowledge that lower cost doesn't guarantee high quality, and a critical eye is always needed to ensure the results meet desired accuracy standards.
Furthermore, the adaptability of OCR to various document formats is noteworthy. Current systems can handle not only printed documents but also handwritten notes and documents with diverse font sizes and styles. This versatility expands the range of materials that can be efficiently translated, which can benefit a broader range of users and tasks.
Overall, while the integration of OCR with AI translation has introduced numerous benefits, it's not a silver bullet. Understanding the nuances of OCR accuracy and potential error propagation remains vital, as does the ongoing need to develop intelligent post-processing methods. The goal remains to develop translation systems that are not only fast but also consistently accurate and reliable, particularly in sensitive fields where mistakes can have significant implications.
How Neural Networks Improve English to Ukrainian Translation Accuracy A Technical Analysis in 2024 - How Local Language Models Cut Ukrainian Translation Costs by 72%
The emergence of local language models has revolutionized Ukrainian translation, leading to a remarkable 72% reduction in translation costs. This significant cost reduction is achieved through the application of deep neural networks specifically trained for Ukrainian. These models are optimized for the nuances of the language, resulting in faster and more accurate translations. This localized approach not only makes high-quality translations more affordable but also emphasizes the crucial role that context plays in producing coherent and meaningful results. The potential of these models extends to real-time translation of documents, suggesting a future where AI-powered translation seamlessly integrates into various industries. Yet, as costs decrease, it's imperative that quality standards are maintained, preventing the pursuit of affordability from compromising translation accuracy.
Local language models are showing remarkable promise in making Ukrainian translation more accessible and affordable. Specifically, we've seen translation costs decrease by as much as 72% when these models are used. This is a game-changer for smaller businesses and organizations that may not have had the budget for high-quality translations previously. The potential impact on industries requiring regular communication across languages is definitely worth watching.
Neural networks tailored to the Ukrainian language have become crucial to this progress. By designing them to understand Ukrainian grammar and syntax, we've seen major gains in both accuracy and translation speed. These custom models appear to outperform general-purpose translation systems, showcasing the value of understanding language-specific characteristics.
The marriage of OCR and neural networks is accelerating the translation process significantly. We're talking about document translation times dropping from a fairly long 6 minutes to an incredibly fast 30 seconds. This speed boost, largely due to the ability of AI to pull text from scanned documents, opens the door for real-time applications in areas like legal and business negotiations, where fast turnaround is critical. This type of integration is exemplified by features like Microsoft's Document Translation.
However, a concern that arises with the use of OCR is error propagation. Mistakes made when the OCR system extracts text from a scanned document can be carried through into the final translation, impacting its quality. This issue emphasizes the importance of continued development of systems that can identify and correct OCR errors before they affect the translation output.
Beyond Ukrainian, these OCR systems are capable of handling multiple languages simultaneously. This versatility allows for seamless translation of documents with multiple languages or language mixes, which can be crucial for international businesses and organizations that handle diverse document types.
The adaptability of these models to user-specific data is also very interesting. Imagine a translation system that automatically adjusts its language to medical or legal jargon based on the user's needs. It's fascinating how we're moving toward personalized translation solutions. While this is extremely promising, we need to examine carefully how users will be able to fine-tune and modify these systems to perfectly suit their unique requirements.
The improvements made possible by OCR and AI integration are estimated to cut document processing times by up to 80%. This efficiency gain is significant because it frees up professionals to concentrate on more complicated tasks rather than manually transcribing documents. This streamlining of the workflow has the potential to drastically change the way certain industries operate.
The use of large, multilingual datasets is essential for training robust neural networks. However, if these datasets are not carefully curated, biases can emerge, leading to skewed or inaccurate translation outputs. Researchers and developers need to be very mindful of these potential biases and work to minimize them.
We're also seeing that these models can be continuously improved by user feedback. This continuous development based on real-world experience can certainly lead to better models and enhanced user experiences. But this also brings up legitimate concerns about data privacy and the potential for unintentional bias to creep in.
Finally, we can't overlook the economic impacts of these advancements. The ability to provide high-quality translations more affordably challenges the traditional role of human translators. It's likely that we'll see human translators focus on more niche areas that require nuanced contextual understanding. The future of the translation field is likely to evolve as these technologies mature.
How Neural Networks Improve English to Ukrainian Translation Accuracy A Technical Analysis in 2024 - New Attention Mechanism Handles Context Better Than Previous Translation Models
Recent developments in neural machine translation (NMT) have introduced a new attention mechanism that significantly improves how context is handled compared to previous approaches. This new mechanism, often integrated within a reinforcement learning-based framework, utilizes a dual attention system. This dual attention system allows the translation model to pay closer attention to relevant parts of the input sentence, effectively filtering out less important information. This refined focus allows the model to construct more accurate context vectors, which are then used to predict the most appropriate target words.
Such advancements are particularly valuable for languages like Ukrainian, where subtle nuances and context are crucial for delivering accurate and meaningful translations. The new mechanism excels at understanding the long-term dependencies within a sentence, resulting in a much more refined translation process. This refined approach shows great potential for enhancing the speed and accuracy of AI-driven translations in various contexts.
Looking forward, incorporating this enhanced attention mechanism into future NMT models could lead to a substantial improvement in translation quality across a wide range of applications. The ability to understand and manage context more effectively holds the key to unlocking the next generation of AI translation tools that are both faster and more accurate. This could significantly transform how we navigate multilingual communication, both personally and professionally.
Recent advancements in neural network design, specifically the introduction of the "attention mechanism," have dramatically changed the landscape of machine translation, particularly for languages like Ukrainian that often involve complex sentence structures. Essentially, this new approach allows the translation model to focus on the most relevant parts of a sentence during translation, rather than just processing words in a linear sequence. This shift is quite a departure from previous methods that frequently stumbled when dealing with intricate grammar and the subtleties of language.
Instead of treating every word equally, as older models often did, attention-based models assign different weights to words depending on their surrounding context. This means that the system can more accurately capture the meaning of idiomatic expressions and intricate grammatical structures that often define a language like Ukrainian. The result is a more natural and accurate translation.
Testing has shown that these attention-based mechanisms perform very well at maintaining coherence across lengthy sentences – something previous generations of models had difficulty with. For areas where precise language is essential, like in legal or medical fields, this improvement can be quite important.
Aside from improved accuracy, the attention mechanism has also helped with translation speed. By prioritizing the most important parts of the text, the translation process becomes more efficient, which can be beneficial for real-time translation scenarios like the rapid translation of contracts during international negotiations.
Surprisingly, these attention-based systems seem to generalize well across languages. Models trained on English-to-Ukrainian translations often show a significant increase in performance when applied to English-to-Russian or other Slavic languages, showcasing the flexibility of these context-aware architectures.
The rise of attention-based models has shed light on some shortcomings of older rule-based translation systems. While these older methods rely on fixed grammatical rules, attention models adapt and learn from large datasets, illustrating a notable change in how language processing is likely to develop in the years ahead.
However, the development of these new models is not without its challenges. Training these models is computationally more expensive than previous methods. This has raised concerns about the resources required for developing these translation technologies and how companies can strike a balance between cost and performance.
Since attention-based models depend on enormous datasets, it's crucial to make sure these datasets are free from bias. Historical biases embedded in the data can lead to skewed and potentially inaccurate translations. This raises some critical ethical questions that developers must consider during the training process.
The benefits of attention mechanisms extend beyond translation itself and can even enhance OCR systems. By considering the surrounding context, attention-based systems can reduce OCR errors before the translation process even starts, improving overall translation quality.
The potential improvements to contextual accuracy in translation can have a significant impact on global businesses. The possibility of both reducing translation costs and improving accuracy allows businesses to better engage with global markets, potentially leading to a major shift in how we communicate across cultures in a more connected world.
How Neural Networks Improve English to Ukrainian Translation Accuracy A Technical Analysis in 2024 - Training Data From 2024 Ukrainian Social Media Improves Colloquial Translation
The use of Ukrainian social media data for training neural networks in 2024 has significantly improved the accuracy of English-to-Ukrainian translations, particularly in informal contexts. Neural networks are now better at capturing the nuances and unique expressions found in everyday conversations, leading to translations that sound more natural and reflect the intended meaning more effectively. This is a notable step forward from earlier translation models, which often struggled with the more complex aspects of conversational language.
The trend of using real-world, user-generated content for training highlights a shift towards more adaptable and responsive translation systems. However, it is important to acknowledge that while speed and cost-effectiveness are valuable, they shouldn't come at the expense of maintaining high translation quality. Striking the right balance between efficiency and accuracy will continue to be a key challenge as the field of machine translation continues to evolve.
Neural network advancements have significantly improved English-to-Ukrainian translation accuracy, particularly through the use of custom-trained models. However, the availability of high-quality training data for less common languages like Ukrainian remains a hurdle. Interestingly, we've seen that leveraging training data from Ukrainian social media has been surprisingly effective in boosting the quality of colloquial translations. This data captures a wider range of everyday language use, helping neural networks better understand the nuances of informal communication.
While statistical machine translation methods can achieve acceptable accuracy for commonly used phrases, they struggle with less frequent scenarios, highlighting the need for more advanced neural network approaches. Conversely, traditional rule-based systems often excel at handling specific cases but lack the adaptability of neural networks. The scalability of these neural models allows them to be readily applied to various language pairs and domains with minimal manual intervention, enhancing overall translation efficiency.
There's a recognized gap in the availability of parallel data for lesser-known languages, emphasizing the need for vast quantities of training data to optimize neural machine translation models. This issue underscores the importance of finding effective ways to create and curate larger datasets for underrepresented languages. Moreover, we're seeing the rise of real-time language translation, notably on social media platforms, powered by increasingly sophisticated machine learning techniques. This improved accessibility of communication across language barriers is quite remarkable.
The translation landscape in 2024 is experiencing a significant shift due to AI innovations and changing language needs. Organizations like DeepL are at the forefront of research in neural network architecture and training, as well as identifying the best strategies for acquiring high-quality training data. The evolution of machine translation has transitioned from the rule-based and corpus-based approaches of the past to advanced neural machine translation methods. Research now primarily focuses on exploring its potential in various industry settings and understanding its future impact.
While these advancements are promising, it's important to remain aware of limitations. The ongoing need for post-editing to refine AI-generated translations remains a key consideration. Some researchers believe that a hybrid strategy, blending NMT with traditional translation techniques, may be the optimal path to consistently high-quality translations across a wide spectrum of languages. Furthermore, ensuring accurate translations across diverse contexts remains challenging, particularly when handling subtle language nuances and idiomatic expressions.
The development and deployment of AI-based translation tools are becoming increasingly cost-effective, potentially offering cheaper high-quality translations to a wider range of users. However, cost reductions should not come at the expense of quality. It is essential that researchers and developers continue to strive for accuracy and reliability while exploring methods to reduce costs. The notion of continuous model refinement using user feedback is particularly compelling. This dynamic approach can lead to more nuanced models and improve user experience. Yet, concerns regarding data privacy and the possibility of introducing unintended biases with user data must be thoughtfully addressed.
The growing availability of multilingual datasets is clearly fueling progress in machine translation. While beneficial, we must also be mindful of the potential for bias in the data, which can skew translation results. Ultimately, the goal is to create AI translation systems that are both efficient and accurate, facilitating seamless communication across language boundaries.
How Neural Networks Improve English to Ukrainian Translation Accuracy A Technical Analysis in 2024 - Memory Efficient Architecture Makes Ukrainian Translation Work on Mobile Devices
The development of a memory-efficient architecture for Ukrainian translation models is a significant step towards making high-quality translation more accessible on mobile devices. This new architecture allows these complex neural network models to function effectively with limited resources, a major improvement over previous approaches such as those used in the NLLB project. This new approach demonstrates that neural network models can be optimized to run smoothly on mobile devices without sacrificing accuracy.
One key aspect of this new approach is the use of pruning techniques to streamline the models. These techniques can remove up to 80% of specialized parts of the model without significantly impacting the overall quality of the translations. This makes the models much smaller and more efficient, allowing them to run on devices with limited memory like smartphones or tablets.
Another promising feature is the potential for on-device training. This allows users to fine-tune the translation model with data specific to their needs. For example, a user could train a model to be more accurate when translating medical or legal documents by providing it with relevant examples. This personalization aspect highlights the increasing trend towards tailoring AI to individual requirements.
The potential impact of this memory-efficient approach is substantial. It promotes broader access to accurate translations, which can improve communication and understanding across different communities and industries. While this progress is notable, it’s important to acknowledge the need for continued development in refining model performance across diverse contexts and ensuring that accuracy isn't compromised as models become more streamlined. The focus on user adaptability is an exciting trend in the field, however, and promises to unlock new possibilities in how AI-powered translation can be integrated into everyday life.
The development of memory-efficient neural network architectures has made it possible for Ukrainian translation models to run smoothly on mobile devices. This is a significant advancement over previous systems, like the NLLB model, which, while contributing to the Aya dataset and the development of multilingual models, often demanded more processing power.
Historically, machine translation methods have been a mixed bag. Statistical approaches are good enough for common phrases but struggle with rare situations. Rule-based methods, conversely, are very accurate for specific instances but can't easily adapt to new situations. Neural networks, however, excel at being flexible and working across various language combinations and specialized fields without much human intervention.
Researchers have come up with a new method for pruning these models. It removes up to 80% of the language-specific components without sacrificing translation quality too much. This makes it feasible to run these models on a single, standard 32GB GPU, which opens doors for wider accessibility. Mixture-of-Experts (MoE) techniques are becoming a key component in bigger language models, showing how they can learn complex human-like thinking patterns. There's also some interesting exploration with computing-in-memory (CIM) architecture. If it works, it might become a great way to run these complex MoE systems on devices at the network's edge.
Neural machine translation has seen a rapid boost thanks to advances in artificial neural networks, especially representation learning and deep learning. One interesting experiment involved building an English-to-Ukrainian translation system using recurrent neural networks, which stopped improving after around 25 training cycles. On the practical side, there has been progress with on-device training methods. These are important because they let users tailor translation models to their specific data, leading to better results for a particular person or industry, right there on the mobile device.
These developments suggest that we are reaching a point where AI-powered translation will become increasingly accessible across a broad range of devices and contexts, while at the same time highlighting the persistent need for attention to data quality, potential biases, and the evolving landscape of computational requirements for training increasingly complex models. It will be interesting to see how these optimizations for efficiency play out in the broader translation market and what the impact will be on the needs and opportunities for human translators in the future.
AI-Powered PDF Translation now with improved handling of scanned contents, handwriting, charts, diagrams, tables and drawings. Fast, Cheap, and Accurate! (Get started for free)
More Posts from aitranslations.io: