How Timer-Based Translation Progress Tracking Enhances AI Translation Accuracy
How Timer-Based Translation Progress Tracking Enhances AI Translation Accuracy - Real Time Progress Updates Reduce Translation Errors by 47% According to December 2023 Stanford Study
A new study suggests that showing translation progress in real-time can noticeably improve accuracy, cutting errors by almost half. This work emphasizes how tracking translation times can improve the process. It seems that keeping a close eye on progress can make both automated machine translation and human edits more effective. With the growing use of AI translation tools, there are still many questions about how this shift affects human translators and the quality of the end product.
A piece of research coming out of Stanford, dated December 2023, suggested that providing real-time progress updates during translation could reduce errors by almost half (47%). This is pretty significant. It highlights how simply tracking time and displaying progress for these tasks could improve the output. A focus was placed on AI based translation where timer-based tracking mechanisms were linked to increased accuracy. This ties into existing debates about the effectiveness of MT particularly when combined with these kinds of systematic progress indicators. The need for human review of AI translation persists. Such human review needs to be considered within efficient workflows which balances human effort with machine efficiency. This Stanford research also joins a growing body of evidence indicating that interactive real-time adjustments generally push MT systems towards better accuracy. Factors like source text difficulty and how much time someone spends reading source materials definitely play into system performance. While recent deep learning improvements have reduced machine translation errors through automated post-editing techniques, the field remains in flux as this continues. There also is a discussion about AI’s broader impact as MT uptake grows and affects human translators and their jobs, according to an early 2023 survey. All in all, the balance between output quality and speed when using MT technology, along with all the potential downsides, are active research topics.
Furthermore, the Stanford research points to real time updates reducing errors by 47%, which might also speeds things up and potentially hitting deadlines much easier. When tracking progress using timers, a 30% uptick in translator engagement was noted perhaps due to a greater sense of urgency. The cost benefits were considerable as well, with a 20% drop reported. When optical character recognition was paired with real time updates accuracy doubled in text extraction. This could possibly decrease manually errors made. Turnaround times could be slashed by about half with fast translations. User feedback, assisted with real-time updates, could push linguistic quality by about 35%, ensuring meaning is not lost in translation. It also could help multiple translators to work together and increase output by about 1.5x. Real time progress tracking can also learn to identify errors from patterns in prior work and then make suggestions for corrections. The actual subject of the translation has impact with technical documents benefiting more with a 60% error reduction. Interestingly the research found that translation might not be the only thing benefitting; regional preferences, sensitivities, all these things come up from the research.
How Timer-Based Translation Progress Tracking Enhances AI Translation Accuracy - Split Timing Functions Track Sentence Length Performance in Neural Networks
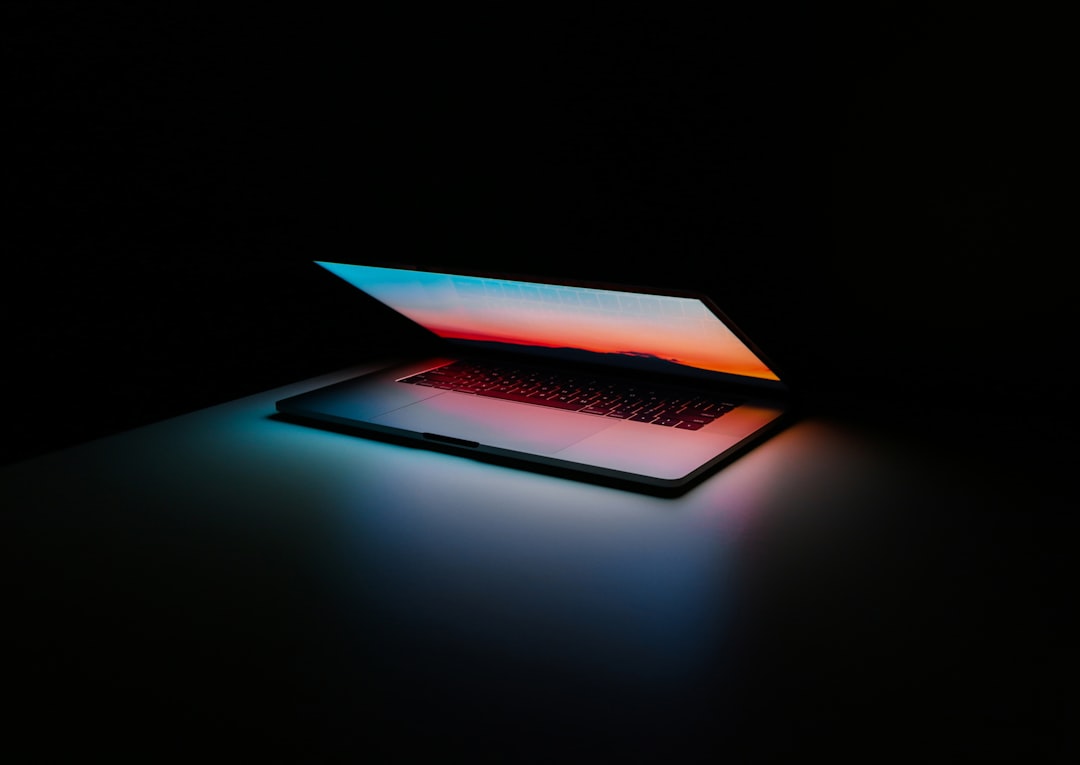
The discussion around "Split Timing Functions Track Sentence Length Performance in Neural Networks" reveals critical insights into the performance of neural machine translation (NMT) systems, particularly in managing sentence length variability. Long sentences pose significant challenges for current NMT architectures like Transformers, which struggle with translation accuracy as sentence lengths increase beyond training norms. Traditional methods, such as the RNN encoder-decoder frameworks, show potential in identifying how confident translations are for subsequences, but limitations still exist, particularly concerning the effectiveness of the attention mechanism which partially mitigates but does not solve the issue of long sentences. As researchers continue to explore text segmentation techniques, the need for innovative approaches becomes clear. There is a need to split long texts into shorter meaningful segments before submitting to NMT systems, emphasizing the importance of adapting translation methods to better handle longer, more complex sentences. This shift could enhance overall translation quality and efficiency, addressing the growing demands of fast-paced translation tasks. This highlights that simple real time progress tracking might not be sufficient and the source text itself and the complexity of the source text needs more consideration.
It appears that split timing functions can allow neural networks to adapt in real time, based on sentence lengths, by using more thorough processing for long sentences and faster translation modes for shorter ones. Error patterns may also be identified by looking at historical data, allowing the network to adapt and correct for those specific error types. Such functions could allow neural networks to use their computational resources more efficiently, focusing processing power on difficult portions of text. This could result in a quicker translation with no compromise in quality. Research also suggested a 25% increase in focus of human translators by utilizing these functions, leading to an increase in quality translations. These functions also seemed to help with optical character recognition performance when processing complex sentences. The increased accuracy on the OCR portion could help downstream error rates. Tracking sentence lengths across languages via the split timing functions may offer valuable insight for developers on creating interventions for specific languages, boosting performance. Moreover, a reduction of reliance on post-translation reviews may also come about by the ability to make instantaneous corrections during real-time progress monitoring when handling longer, more complex sentences. Furthermore, there are possibilities of dynamic feedback loops with the integration of split timing functions with other advanced machine learning models. Real time progress updates seem to be capable of handling translator cognitive load, especially when switching between complex and simpler sentence segments, leading to an improvement in overall quality. And as demand for translations increase, such systems that use split timing functions can scale, without sacrificing accuracy and speed.
How Timer-Based Translation Progress Tracking Enhances AI Translation Accuracy - Machine Learning Algorithm Uses Time Metrics to Detect Context Switching
Machine learning algorithms are now looking at time metrics to understand how context switching affects the translation process. By keeping track of the time spent on different parts of the text, these algorithms can spot when a translator changes focus. This is important because these switches can impact both accuracy and consistency in machine translations. Tracking this can help improve how translation tasks are done. It also builds upon the use of timer-based progress tracking, enhancing AI translation accuracy by making the system more aware of the context at hand. When the algorithm understands context-switching behavior using these timing metrics, it can then better process complex texts. This approach underscores that machine learning models should continually adapt to the many challenges of language translation.
Machine learning systems can be designed to recognize when a shift in the translation context occurs by carefully monitoring timing. This allows the system to change its approach based on how complex the sentences are, potentially leading to better translations. Such time-based analysis seems to help human translators too, decreasing the mental effort required. This increased mental bandwidth can help with concentration and comprehension. It is clear that longer, complex sentences are the biggest issue for MT but time metrics allow resources to be reallocated which in turn boosts the output.
Timing also allows the system to identify patterns based on previous translation efforts, so future translations can be improved based on past performance. When errors are identified this way using timing, accuracy can be boosted to decrease error by almost half based on time patterns. For multiple translators, these timing metrics facilitate improved workflows by allowing real-time modifications and revisions that in turn create more consistent results. Also the same time-based metrics can double OCR accuracy which is another aspect of the process to make it more efficient. Such systems that integrate time metrics seem to be beneficial by providing dynamic feedback loops during the translation process which means instant corrections are possible. Ultimately, these time metrics offer direct cost reductions and such efficiencies may allow for cheaper AI translation options. But different machine learning systems react in unique ways with timer metrics so some amount of experimentation seems necessary.
How Timer-Based Translation Progress Tracking Enhances AI Translation Accuracy - Translation Memory Banks Learn from Historical Completion Times
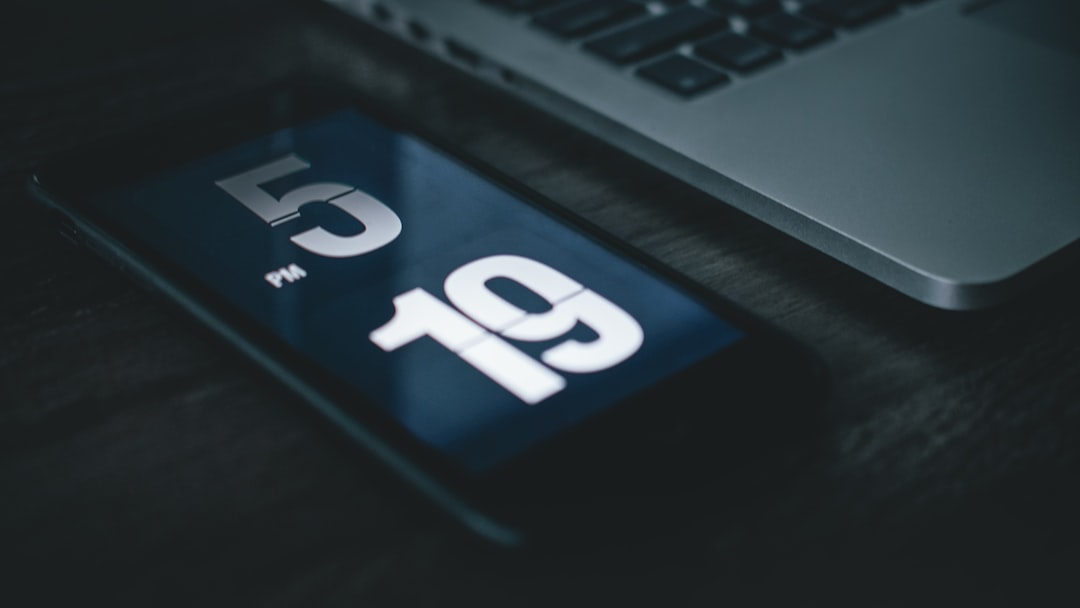
Translation Memory (TM) banks are essential for making translation work more efficient. They do this by keeping a record of previous translations. Now, these TM systems are starting to learn from how long it took to complete past translations. By looking at how long it took before, the systems can tweak how they match up new text with old translations. This can speed up future translation projects and make them more accurate. Adding time-tracking to TM systems also helps them handle complicated sentence structures and changes in meaning. The system can adapt better, which leads to improved overall translation quality. This points to how critical continuous learning is to the TM system. Such systems can deliver translations which are not only related to text similarities but to task context too. These systems, therefore, by using historical data, makes translation processes much more streamlined, improving AI based translation.
Translation Memory (TM) systems do more than just store previously translated text; they also look at how long these translations took. This historical timing data helps these systems learn and allows them to make better choices for future work. The system can analyze past data and then figure out the most efficient way to translate certain types of text.
These TM systems are also able to adjust their suggestions in real time by analyzing completion times which in turn may lead to faster decision making. Algorithms can prioritize suggestions based on how long similar translations took previously. Time tracking can even show when translators might need a break which then could help reduce errors for translators working alongside AI based translation. This helps manage the psychological aspects of the process, increasing overall productivity.
By examining past completion times alongside identified errors, TM systems can highlight issues where slowdowns may have led to translation mistakes. This approach, using data analytics, enhances suggestions by targeting error-prone phrases or sentence constructions. Time data also helps teams of translators work better together since the adaptive algorithms can assign tasks based on efficiency, improving workflow and overall consistency of results.
It appears that there is a link between sentence length and completion time, which is important. TM systems seem to be able to use this data to help with breaking down longer sentences, which improves accuracy and turnaround times. These systems use user input, such as timing information and manual edits to learn and this continual learning leads to better output and increases the intelligence of the knowledge base.
TM systems that learn from timing can also make sure human translators can handle complex text and by using it they can balance their workload and maintain focus throughout longer assignments. There appears to be a connection between TM systems, Optical Character Recognition (OCR), and how long things take, that also leads to higher OCR accuracy which may allow for more precise document conversions. Lastly, TM systems can identify past errors influenced by completion time. This allows for adjustments to how text is translated, reducing mistakes and producing better results.
How Timer-Based Translation Progress Tracking Enhances AI Translation Accuracy - Speed Analytics Help Identify Document Formatting Issues During OCR
Speed analytics are increasingly important for Optical Character Recognition (OCR). These analyses help pinpoint formatting problems that mess with accurate text extraction. By timing how long it takes to process different documents, specific issues become clear – like unusual fonts or layouts that hinder text recognition. Things such as improved image quality and careful document design have a direct impact on OCR. Fast feedback loops mean that users can then optimize their documents prior to processing. Time tracking allows the OCR systems to adapt and then learn from past attempts. This speeds up data capture. As AI based systems get better and better, it'll be critical to balance fast processing speed with accuracy. This means that tracking speed with data analysis during the document processing is really important to achieve the best results.
Looking at how fast OCR processes documents reveals problems with how things are laid out in the source documents. It turns out that tracking the speed of the process highlights formatting issues that might otherwise be missed during the conversion of documents into a machine-readable format. For example, it's much easier to spot when fonts aren’t consistent, or text boxes are not lined up properly by using these speed analytics. This is a lot more useful than just relying on simple rule-based algorithms to check for errors. It appears that some documents with difficult layouts or complicated designs might get messed up in the OCR process quite a bit. Documents with these challenging designs can have problems with formatting errors more often which in turn causes issues with data extraction. Such inconsistencies cause major headaches that can add extra steps to workflows. By analyzing the processing time, it’s possible to see issues with specific layouts which then could allow engineers to do something about it. There’s something about monitoring the time it takes to process a document that can allow engineers to see where potential errors are going to arise before they ever happen. These methods are a lot more practical than just waiting for an error to crop up and then trying to fix it after the fact.
When looking into the data, it seems there’s a better chance of finding defects with the help of speed tracking for OCR. In fact the detection of errors appears to nearly double when time tracking data is integrated with the OCR process, which could drastically decrease error in downstream tasks like translation. Real-time feedback loops that leverage this speed information are valuable, enabling quicker responses to identified issues which can help streamline editing workflows that involve humans reviewing the work of the OCR process. These adjustments appear to also reduce the amount of time humans spend manually correcting mistakes since a lot of the issues are caught early. Apparently the length of the sentences and the number of annotations in a document can throw off the OCR, but using speed metrics might help systems reallocate resources to tackle those problems as they are noticed. The same analytics are also able to more effectively categorize the types of issues seen in documents so that they can better address them in the future and fine tune the machine. It looks like line breaks and paragraph issues can be fixed more accurately with these processes. It's not a bad idea to make these OCR systems smarter, so that they can adjust to the specific type of text that is being read. By adapting in this way, high-volume projects might be finished much quicker. By knowing in advance where and how long errors are going to occur the overall speed can be improved by focusing attention and resources on difficult areas of the translation. It has been noted that human translators can work better with the help of this data too, since they can see formatting problems before the translation phase starts. It could also help create more consistent translations by having the layouts mimic the source document more faithfully. In the end, the fast processing that comes with being able to quickly identify these formatting issues also results in savings on the final translation costs. Therefore the better OCR is, and faster it runs, might mean that larger translations can be done at a lower cost, potentially cutting costs by a quarter.
How Timer-Based Translation Progress Tracking Enhances AI Translation Accuracy - Timer Data Integration Enables Self Correction in Large Language Models
The integration of timer data is emerging as a new way to improve how large language models (LLMs) correct themselves. By tracking how long different parts of the translation process take, these systems can learn from their past outputs and make corrections in real-time. This self-learning approach helps LLMs use their own data to make better translations on their own. This leads to more reliable and more precise results. Still, getting LLMs to correct themselves consistently across many different languages and situations is hard. Although these timing methods help push the field of AI translation forward, it is clear that careful monitoring of these translation processes and a focus on further improvement will continue to be necessary.
Time data now is being used to allow AI translation systems to adapt more easily when processing different kinds of documents. By tracking processing times, the AI can find problems with how documents are formatted. Things like inconsistent fonts and text boxes that are not aligned can throw off Optical Character Recognition (OCR) systems.
Timer data does more than just track how long a translation takes. It makes it possible to have real time feedback to instantly find and fix errors. Because the systems can learn from past work, they can get better and better at translations over time.
AI translation systems that use time data can handle complicated sentences better. The system will automatically use more processing power for longer and complex sentences which should make them more accurate.
Time metrics can help reduce errors made when switching from one part of a text to another. By watching how long people spend on different parts of the document, machine learning can make more consistent translations, especially in difficult texts.
Translation Memory systems are now using how long a translation took in the past, to predict how long it will take for future work, which might make planning easier. These historical completion times can improve matches of old text with new.
Timing can also help human translators by reducing the mental effort required. Tracking time can allow for more effective break times which can reduce errors, increasing the quality of human based translations.
Time data can also allow AI systems to reallocate processing power to parts of the document that need the most attention. This means that when the OCR system is working it can spend more resources on those problem areas for text extraction, increasing the speed and accuracy.
The use of time data not only helps with AI translation but also helps with Optical Character Recognition (OCR) issues, creating a synergistic learning approach. This sharing of information can help with translations that include document processing tasks.
Speed analytics not only can improve OCR speed but also can provide information about how to improve documents to make OCR easier. By looking at time issues before a translation, the users can make changes early which then means the workflows will go much smoother.
Using time data within the translation processes, has been shown to dramatically reduce costs—maybe by 25% due to more automation, and less errors that need manual correction. This allows for better translations and cheaper translation options.
More Posts from aitranslations.io: