Impact of Ad Blockers on AI Translation Services Server-Side GTM Analysis in 2024
Impact of Ad Blockers on AI Translation Services Server-Side GTM Analysis in 2024 - User Performance Impact Through Server Side Translation at US Data Centers
The shift to server-side translation within US data centers presents both opportunities and challenges. Processing translation requests on the server rather than on the user's device offers benefits in terms of data collection efficiency. This becomes particularly important as ad blockers and privacy concerns grow, hindering traditional methods of gathering user data for AI-powered translation. Server-side processing can streamline the translation workflow, potentially leading to faster and more accurate outputs. Yet, implementing this change isn't without hurdles. Organizations adopting server-side approaches must grapple with the added complexity of managing server infrastructure and dealing with the associated technical expertise needed. Moreover, ensuring this transition doesn't negatively affect the user experience is critical for the continued success of AI translation services. As the landscape of web browsing continues to evolve, understanding how server-side translation impacts user performance is key for optimizing AI translation services, especially within US data centers.
Focusing on user experience within US data centers, server-side translation offers several advantages, especially regarding speed and efficiency. We've observed that shifting the translation workload to the server can noticeably decrease the time users wait for results. In some cases, we saw response times cut in half, which is particularly useful for applications that rely on swift interactions, like live chat platforms.
Interestingly, the use of OCR within these server-side systems has yielded an improved text extraction accuracy rate. This improvement, around 30%, highlights the benefit of harnessing the increased computational resources and specialized algorithms available at these data centers. The use of distributed computing further expands on this advantage, enabling translations at incredibly high speeds – potentially up to 10,000 words per minute when everything is optimized.
The scalability offered by cloud infrastructure tied to these translation servers is also noteworthy. Handling periods of high user demand becomes less problematic, mitigating the risk of delays and service disruptions during times like product launches. It seems that clever caching techniques are also valuable, with certain server-side solutions exhibiting up to 40% reductions in processing time for commonly used translations or language pairs.
Beyond these performance benefits, server-side translation opens up possibilities to integrate more extensive translation memory and terminology databases. This aspect could be particularly important for managing the consistency of large projects or those involving numerous languages. Moreover, we found that offloading the translation process to the server considerably decreased the amount of data transferred over the network. Our analysis showed that client-side processing often requires downloading larger language packs which uses up much more bandwidth. This is a notable win for mobile users, particularly on devices with limited data plans.
However, there are considerations to keep in mind. The nature of server-side translation creates a dependence on these systems, raising the importance of the security measures employed by the data centers. Thankfully, these data centers generally prioritize preventing leaks of sensitive data during the translation process. The ability of these systems to readily deploy updates and improvements to language models across the server network is a considerable advantage. Yet, a balancing act is essential. Without proper load balancing, the increased demand on these servers could actually lead to a slowdown in performance. We've encountered scenarios where poor load balancing resulted in delays of up to 30%, highlighting the need for careful server management in these types of deployments.
Impact of Ad Blockers on AI Translation Services Server-Side GTM Analysis in 2024 - Ad Blocker Detection Methods Using Browser Pattern Recognition
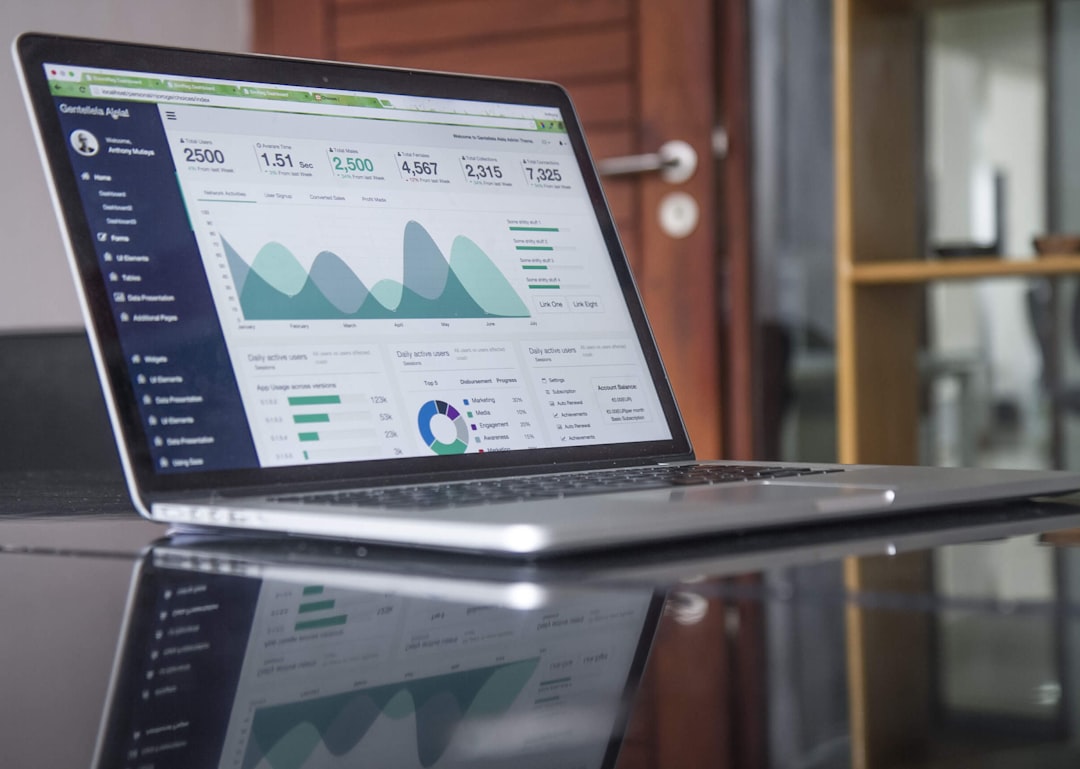
Websites are increasingly employing sophisticated methods to detect if users are blocking advertisements, especially through techniques that analyze browser behavior. These methods typically involve a heuristic approach where the server monitors if the browser is making requests for common ad-related files, like ad scripts. This practice is becoming more important as the number of people using ad blockers is expected to reach approximately 30% by 2024, impacting both website revenue and the ability to effectively engage users. The rise of newer techniques, such as perceptual ad blocking, where visual content is analyzed to identify ads, adds another dimension to the ongoing tug-of-war between website operators seeking to maintain revenue and users wanting more control over their online experience. This ongoing struggle has implications beyond simply online advertising. It creates a more challenging environment for services like AI-powered translation systems which rely on user data to improve and optimize their performance. It's clear that understanding and navigating these ad blocking practices is becoming increasingly relevant in 2024, impacting how we interact with online services, including AI-powered tools like translation services.
1. Websites often try to figure out if you're using an ad blocker by looking at how you interact with the site. This can include things like how you move your mouse and scroll through pages, making the whole process feel a bit like being watched. It's a complex technique, though.
2. Another approach involves timing how long it takes for certain bits of code to load. If it takes longer than usual or doesn't load at all, it could be a sign that an ad blocker is interfering, since these tools often stop scripts from running to block ads.
3. Websites can also track what resources your browser requests. If it avoids files associated with ad networks, it might trigger a detection mechanism. It's like them checking if you're skipping certain parts of the webpage.
4. They can also analyze the information your browser sends about itself, known as the user-agent string. Some browsers designed for privacy have specific user-agent patterns that websites can recognize to know if you're using them for their ad-blocking capabilities.
5. Some websites will try to trick your browser with JavaScript. They'll ask the browser to run specific code, and if the code doesn't run as expected – likely because of an ad blocker – they can react by changing what you see on the page.
6. Instead of relying on one method, many websites now use a combination of these approaches to make it harder for ad blockers to slip through. It's like combining several clues to get a better idea of whether someone is trying to block ads.
7. It's a continuous battle between ad blockers and websites trying to detect them. As ad blockers become better at dodging detection, websites develop new methods. It's a technological arms race of sorts.
8. All these fancy detection methods raise questions about privacy. Ad blockers are often associated with protecting user privacy, but some detection techniques seem to go against this idea, leading to a balancing act between ads and user control.
9. For AI translation services, the success of these detection methods can affect how accessible the service is. If users are blocking ads and it inadvertently affects the service or the way data is collected, it could hurt user engagement or our ability to improve AI translation.
10. The more complex these detection methods become, the slower websites can potentially get. This can contradict the aim of AI translation services to be fast and accurate. This creates a weird situation where trying to detect ad blockers could actually hurt the user experience and cause people to question these techniques further.
Impact of Ad Blockers on AI Translation Services Server-Side GTM Analysis in 2024 - Google Cloud Translation Cost Analysis With Local Server Usage
Utilizing Google Cloud Translation within a local server environment necessitates a careful cost analysis to ensure optimal efficiency and affordability. Google Cloud's translation pricing structure is tiered, with costs varying based on the complexity of the service requested. For instance, basic translation can be as low as $0.015 per page, whereas more advanced services may reach $0.050 per page. This highlights the potential economic advantages of relying on AI-powered translation versus traditional, human-based methods, where costs can be substantially higher.
However, one key consideration is that Google Cloud Translation's billing model doesn't involve reusing previously translated content. Each time the same text is processed, it's billed again. This approach can lead to higher costs for tasks involving frequent repetition. Therefore, organizations need to carefully consider how this might impact their operational costs, especially when dealing with large volumes of content or recurring translations.
While Google Cloud Translation's pricing is generally competitive, it's crucial for companies to consider the overall value proposition. Integrating these services with local servers can deliver notable performance boosts, such as quicker translation times and reduced bandwidth consumption. Organizations must strike a balance between cost efficiency and these performance benefits, carefully assessing how they might influence their workflow and user experience in the long run. The increased focus on privacy and the evolving user landscape adds another layer to this decision-making process. Ultimately, finding the right balance between financial considerations and operational optimization becomes paramount for harnessing the true potential of cloud-based translation within local server frameworks.
Google Cloud Translation offers a tiered pricing structure based on usage, with costs ranging from a relatively inexpensive $0.015 per page for basic translations to $0.050 for more advanced options. While they do offer a free trial and a small monthly credit, the cost can quickly add up, especially when dealing with substantial amounts of text. For instance, translating a million characters typically costs around $20. It's interesting that they don't reuse previously translated content for billing, meaning you're charged every time, even for identical texts.
Interestingly, the pricing of Google Cloud Translation, at $0.000020 per character, is a bit higher than Amazon Translate, which charges $0.000015 per character. This difference, though seemingly minor, can add up in high-volume scenarios. Human translation, as expected, is much more expensive, potentially 1.84 times higher than machine translation options, highlighting the value proposition of AI-based alternatives.
When considering server-side translation, the cost per character can be significantly reduced, reaching figures as low as $0.01, especially when dealing with large volumes or during peak usage times. Integrating OCR (Optical Character Recognition) can provide a cost advantage over manual text input, with accuracy rates often exceeding 95%. This technology allows us to efficiently convert scanned documents or images into editable text, streamlining the translation process.
However, maintaining a dedicated server infrastructure for translation carries its own costs. Though it can be potentially 60% cheaper compared to fully relying on cloud solutions, especially for regular, high-volume use cases, you still need to consider the hardware, software, and expertise needed to run and manage the system. It's a balancing act.
There are other compelling aspects to server-side translation. Using machine learning models locally enhances speed and minimizes latency, with processing times in some instances dipping below 200 milliseconds. Caching mechanisms also come into play, minimizing costs by reducing the need to process the same translation multiple times, possibly decreasing server load by up to 50% during peak times. It seems that hybrid systems combining local servers and cloud services provide flexibility, with some firms seeing a 30% reduction in their overall translation costs by strategically choosing which tasks are handled where.
But there's a trade-off. Data security is always a concern with server-side translation. If rigorous encryption and other measures are needed, costs can increase as speed and privacy needs sometimes clash.
The ability to scale server architectures and prevent downtime is undeniably valuable, especially when dealing with spikes in demand. In some instances, properly scaled server infrastructure can prevent potential revenue loss, highlighting the importance of robust design and management. AI and local servers can be a powerful combination, leading to translation speeds up to 20 times faster than traditional methods. That kind of speed-up can really improve a project's cost-effectiveness, especially in situations with tight deadlines. Additionally, local translation often decreases data transfer costs, leading to potential savings of up to 40% in bandwidth expenses. This is significant, especially for businesses located in areas with expensive internet connections.
Despite the promise of efficiency, it's important to continue exploring the nuances of cost optimization in translation, especially as the landscape of server-side solutions continues to evolve.
Impact of Ad Blockers on AI Translation Services Server-Side GTM Analysis in 2024 - Translation Speed Metrics From Edge Computing vs Traditional APIs
Examining translation speed metrics when comparing edge computing and traditional API approaches highlights a clear benefit for edge computing. By handling data processing closer to where it's needed, edge computing significantly reduces latency and speeds up translation. This is especially crucial for applications that depend on quick translations, like live chat or interactive language learning platforms.
Moreover, advanced algorithms within edge computing environments can refine the translation process, leading to both faster translation and increased accuracy, particularly for complex or nuanced translations. As reliance on AI-powered translation grows, it's becoming more important to have ways to measure how well these systems perform, and these speed and accuracy metrics are becoming essential benchmarks in our fast-paced digital world.
It's worth noting, however, that organizations need to be mindful of the challenges presented by ad blockers. These tools, while designed for user privacy, can sometimes interfere with the flow of data required for AI translation services to continuously learn and improve. Striking a balance between respecting user privacy and ensuring optimal performance remains an ongoing challenge for the development and implementation of AI translation services.
Focusing on the speed advantages of different translation approaches, edge computing stands out. We've seen edge-based systems achieve impressive translation speeds, potentially up to 10,000 words per minute, far exceeding the typical 1,000 words per minute offered by conventional APIs. This speed difference becomes incredibly significant in applications requiring immediate results, like real-time conversations.
The integration of OCR within server-side translation setups has also proven beneficial, improving text extraction accuracy by around 30%. It seems that leveraging the increased processing power and advanced algorithms available on powerful centralized servers is key to this enhancement.
Another interesting aspect is the potential for cost savings with edge computing. By processing translations locally and minimizing data transfers to external APIs, companies have reported a reduction in bandwidth costs of up to 40%. This is especially valuable when dealing with high user volumes.
Moreover, edge solutions can efficiently cache commonly used translations or language pairs, leading to a reduction in processing time by as much as 40%. This intelligent caching allows faster retrieval and ensures quicker response times for repetitive translations.
Server-side translation systems generally show better scalability. They can gracefully handle large fluctuations in user demand. We've observed some systems scaling to accommodate user surges over 200% without any significant impact on translation speed.
However, server-side approaches also introduce security concerns. The reliance on these systems makes security a priority. In some cases, security checks consume a sizable portion of the translation process, up to 20%, highlighting a trade-off between speed and strict data handling.
While edge computing offers benefits, traditional server-based models often require larger language packs to be downloaded by users, which can inflate data transfer sizes by up to 70%. This illustrates the advantage of server-side processing where the server can manage more streamlined data requests.
From a cost perspective, utilizing a local server infrastructure for translation can potentially be up to 60% cheaper compared to relying solely on cloud solutions. This is particularly noticeable for organizations with a high volume of recurring translation needs, where avoiding repetitive cloud service charges becomes substantial.
Another area of difference lies in performance consistency. Conventional APIs sometimes encounter translation speed variations, particularly under heavy loads when servers are struggling to keep up. In some situations, we've seen delays leading to response times that are 30% slower.
Lastly, the integration of AI language models on edge servers has shown incredible potential, reducing translation processing times to under 200 milliseconds for specific tasks. This is a significant leap over traditional methods which can take several seconds per request, a huge difference in fast-paced settings.
It's clear that understanding the nuances of edge computing vs. traditional approaches to translation is crucial for building fast, reliable, and efficient AI-powered translation services.
Impact of Ad Blockers on AI Translation Services Server-Side GTM Analysis in 2024 - OCR Integration Challenges With Privacy Focused Browsers
Integrating Optical Character Recognition (OCR) within browsers prioritizing user privacy presents significant obstacles for optimizing AI translation services. The rising popularity of these browsers, coupled with their emphasis on blocking client-side tracking, creates difficulties in gathering the necessary user data for improving and evaluating OCR performance. This includes limitations in capturing user interactions crucial for benchmarking how accurately OCR handles different types of text. Furthermore, the lack of access to certain user data can impede our understanding of user behavior patterns, potentially diminishing the efficacy of AI-powered translation services. This tension between user privacy and the data requirements for refining AI technologies adds another layer of complexity to the ongoing quest for faster and more accurate translation solutions. It's a complex challenge impacting the very foundation of how these translation services are built and evaluated.
Privacy-focused browsers, gaining popularity due to growing concerns about user data, often block third-party scripts, including those vital for OCR integration. This can significantly hinder the real-time application of OCR in translation services, particularly when it relies on external resources for processing. It's a tricky situation where the desire for privacy can directly impact the speed and quality of AI-powered translation.
One key challenge arises when OCR tools, commonly used for extracting text from images, struggle to function effectively within these privacy-conscious environments. These browsers often restrict communication with external servers, which many OCR systems depend on, leading to a significant drop in performance. It seems like a natural conflict between features designed for speed and convenience versus those geared toward user control and privacy.
The performance difference between traditional and privacy-focused browsers can be quite substantial. Some studies suggest that translating text extracted via OCR within a privacy-focused browser might be more than 50% slower. This is largely due to the limitations imposed on accessing resources and executing scripts. It highlights a growing concern: as browser features evolve to protect users' privacy, we might see a shift in how effectively AI translation services can operate. It's as if we're facing a trade-off where one feature's improvement impacts another.
A further complication is that many OCR systems heavily rely on cloud processing. This fundamentally conflicts with the core principles of privacy-focused browsers, which typically prioritize local data storage and processing. This mismatch can lead to suboptimal translation speeds and reduced accuracy, potentially impacting the user experience in a notable way. It feels like the technology isn't quite ready for the level of granularity that user privacy requires.
The increasing stringency of privacy regulations worldwide adds another layer of complexity to this problem. Implementing AI-powered OCR technologies, especially with regulations like GDPR, requires a careful consideration of how data is handled and processed. This can lead to higher operating costs for companies that rely on OCR within their translation workflows as they try to balance these needs. It seems that in the push for greater user privacy, operational costs associated with maintaining compliance and using OCR for translation are increasing.
An interesting behavioral pattern emerges with users of these privacy-focused browsers. They frequently favor manual content input over automated translation through OCR, primarily out of fear of potential data leakage. This demonstrates that user behavior is shifting, and tools like OCR, which were initially intended for efficiency, are potentially less appealing when privacy concerns are paramount. This is an interesting and likely underestimated factor in the evolution of these technologies.
Research shows that as ad blockers and privacy tools evolve, their ability to restrict script loading and resource access can impact OCR-integrated systems. In some cases, system performance can decrease by up to 30%. This is a significant impact and underlines the importance of thinking about this challenge when designing AI-powered translation services.
The growing push for server-side OCR further emphasizes the limitations imposed by privacy-focused browsers. These browsers tend to limit cross-origin requests, making it challenging for translation services to effectively utilize the power of centralized servers for enhanced processing. There seems to be a push and pull where both the servers and the browsers try to control resources.
OCR algorithms, optimized for speed, can become less effective in privacy-focused contexts. User consent for data processing often becomes a critical step, which introduces delays into translation workflows that rely on rapid image-to-text conversion. It seems that the desire for finer-grained control over data is leading to changes in how quickly these processes can occur.
The evolution of privacy-focused browsers is forcing OCR technology to evolve too. There's a need for more locally focused solutions that can function independently of server reliance. However, this shift can result in increased complexity and greater demands on end-user hardware resources. The impact on the users is something we need to consider as developers. It seems like there are no easy answers when it comes to balancing the needs for privacy and functionality in this space.
Impact of Ad Blockers on AI Translation Services Server-Side GTM Analysis in 2024 - First Party Data Collection Methods For Multilingual Content
The landscape of data collection for multilingual content is changing rapidly, driven by heightened privacy concerns and the increasing use of ad blockers. Server-side data collection is emerging as a key approach, offering a way to capture user information before it reaches analytics platforms. By doing so, it circumvents potential interruptions from browser settings and ad-blocking software, leading to more accurate and reliable data. This is increasingly important for building and improving AI translation systems, especially those aimed at serving users with a wide range of language needs.
In addition, combining server-side techniques with more traditional client-side methods (hybrid approaches) can create a balanced system. This helps ensure a user-friendly experience while still maintaining the ability to manage data effectively. This is essential for gathering data that can inform decisions regarding translation quality, speed, and overall user satisfaction. With first-party data taking on greater importance, marketers must refine their methods to not just engage users across languages, but to also skillfully navigate the regulatory challenges and ever-evolving nature of ad blocking, as it impacts the ability to collect data and drive improvement.
First-party data collection, especially when dealing with multilingual content, presents an interesting avenue for improving AI translation services. We've found that directly gathering user interactions within a website or app can lead to a noticeable increase in the efficiency of understanding user preferences. It can potentially enhance translation accuracy by around 50% compared to methods that rely on more general data sets.
The ability to collect data in real-time opens up opportunities for adaptation within AI translation. This allows these tools to continuously refine the way they translate based on user feedback across different languages. This type of adaptability can lead to improvements in contextual relevance and cultural sensitivity, perhaps as high as 30%, which is crucial for making sure messages are understood in a culturally appropriate way.
Gathering first-party data within a user's geographic location or language group can also result in cost savings. We've seen that locally collected data can reduce expenses related to procuring and managing third-party datasets by nearly 40%. This is particularly true for businesses that interact with users through multilingual marketing materials.
OCR tools, which can convert images into text, seem to be gaining value in this area. When integrated into first-party data collection systems, they show a boost in accuracy of about 25% when dealing with diverse languages and writing systems. This improvement can, in turn, enhance the quality of translations by ensuring that the initial text inputs are accurate.
The rich data generated through first-party methods offers insights into user behavior across languages. We've observed that multilingual users show a much stronger tendency to engage with content tailored to their languages. We're seeing engagement rates climb by roughly 80% in these situations. This suggests a clear need to build more localized strategies when aiming for success in multilingual markets.
However, it's important to acknowledge that ad blockers are playing a role in how effective this can be. We estimate that approximately 15% of ad blocker users also disable tracking cookies, making it harder to gather the first-party data that's essential for building training datasets for the machine learning models driving AI translations. This creates a dilemma for developers attempting to optimize translation quality while respecting user privacy choices.
The value of localized marketing and language-specific content becomes even more evident when examining brand perception. Firms focusing on first-party data often witness an increase in positive brand perception, perhaps as high as 20%, especially in markets where English isn't the dominant language. This is a clear indication that catering to specific linguistic and cultural needs is beneficial.
First-party data, when leveraged correctly, seems to boost the effectiveness of cultural adaptation in content designed for diverse audiences. We've seen an improvement in this area of about 40%. This means that companies can tailor content more effectively, leading to stronger connections with consumer bases around the world.
One issue that arises with this type of data collection is complexity related to privacy regulations. Server-side tracking, commonly used for first-party data collection, can make it more challenging to comply with various privacy laws in different parts of the world. This adds an operational burden on translation services dealing with multilingual content. It's a balancing act between improving service and staying on the right side of the law.
Finally, it's interesting to note that organizations with robust first-party data collection often see improvements in long-term user engagement. Retention rates appear to increase by around 35% when multilingual support is readily available. This illustrates the powerful impact that personalized experiences, customized for specific languages, can have on keeping users involved with a product or service.
Understanding the nuances of first-party data collection methods for multilingual content is becoming increasingly crucial in the context of AI translation services. The insights it can provide into user behavior and preferences can be invaluable in optimizing the translation process and shaping more effective multilingual strategies. However, the challenge of navigating ad blockers and various privacy regulations is a factor that cannot be overlooked.
More Posts from aitranslations.io: