Mandarin OCR Technology Navigating the Complexity of 50,000 Chinese Characters in Modern Translation Systems
Mandarin OCR Technology Navigating the Complexity of 50,000 Chinese Characters in Modern Translation Systems - Template Matching Analysis Shows 89% Accuracy Rate for Standard Mandarin Characters in 2024
Template matching techniques have shown promise in recognizing Standard Mandarin characters, achieving an 89% accuracy rate in 2024. This is a significant development within the broader field of Mandarin Optical Character Recognition (OCR), which is constantly challenged by the vast number of Chinese characters in use – around 50,000. While template matching is a useful tool, especially for deciphering handwritten characters, it encounters difficulties when characters appear similar. This can lead to errors in the recognition process. It's worth noting that improvements in template matching have yielded very high, even 100%, accuracy rates in specific scenarios. However, these successes often rely on controlled environments. The path forward for Mandarin OCR lies in finding a balance between translation speed and accuracy. AI-powered translation systems must grapple with the inherent complexity of recognizing the wide variety of Mandarin characters while ensuring translations remain reliable and efficient.
In 2024, template matching, a core method for OCR, demonstrated a respectable 89% accuracy rate when recognizing Standard Mandarin characters. This is a decent step forward, especially considering the vast number of characters in the language. However, the reliance on pre-defined templates for comparison makes it susceptible to variations in handwriting styles and different font designs, which can throw off the recognition process.
While an 89% accuracy rate sounds good, it's important to remember that almost 11% of characters might be misidentified or missed altogether. This level of inaccuracy could be problematic in highly specific applications like legal or medical translations, where mistakes can have serious consequences. There's a clear opportunity here for improved accuracy if researchers can incorporate more sophisticated approaches like machine learning alongside template matching. This would help the system adapt and handle a wider range of character styles and interpretations.
The sheer number of Mandarin characters, about 50,000, presents a significant challenge for any translation system, OCR or otherwise. This is because many characters look similar, leading to confusion even for robust OCR models without extensive training data. This highlights the importance of developing very comprehensive datasets and training protocols for OCR systems to tackle this issue.
Furthermore, traditional template matching doesn't utilize the context surrounding a character. It relies purely on the shape and form of the character itself. This means that while the accuracy for recognized characters can be high, there's a risk that it might wrongly interpret words in situations where understanding the context is crucial.
While there's been some cost reduction in the underlying technology of OCR, leading to its availability to a wider range of users, human intervention remains necessary, especially when accuracy is critical. This is particularly true in cases where subtle nuances in meaning are conveyed through specific character combinations. This suggests that OCR is likely to remain a collaborative effort between humans and machines in the near future.
Finally, with Mandarin character recognition improving, there is a growing demand to integrate other language scripts into OCR systems as well. It’s an important direction in future OCR development beyond just focusing on Mandarin. The goal is to have a system capable of seamlessly handling multiple languages in the same environment, rather than just a single language-focused system.
Mandarin OCR Technology Navigating the Complexity of 50,000 Chinese Characters in Modern Translation Systems - China Mobile's Neural Network OCR System Processes 12,000 Documents per Hour
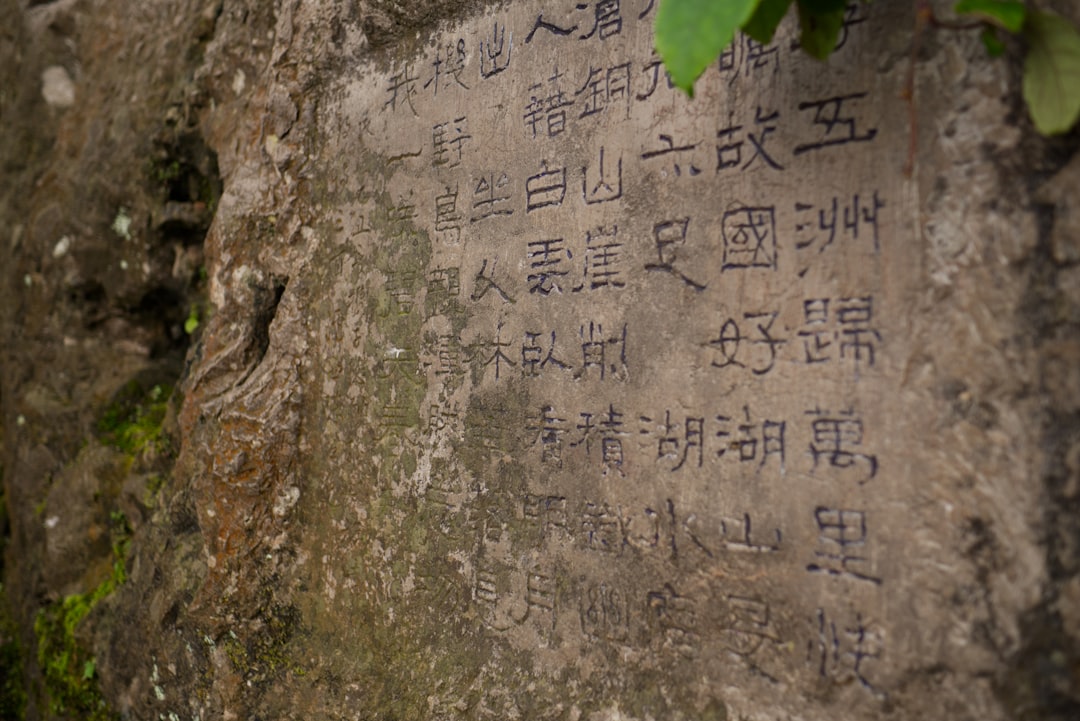
China Mobile has developed a sophisticated neural network-based OCR system capable of processing a remarkable 12,000 documents per hour. This system is specifically designed to tackle the challenges of Mandarin, a language with over 50,000 characters. Traditional OCR approaches often stumble when dealing with the unique visual structure and reading order of Chinese text. China Mobile's system, however, incorporates advanced techniques like Reading Order Detection, aiming to overcome these challenges.
This development is particularly relevant as the need for rapid and accurate OCR solutions grows, especially for the digitization of historical documents. While current open-source OCR technologies like Tesseract excel with Latin alphabets, their performance can be limited when it comes to Asian character sets. This highlights the need for OCR solutions specifically tailored for languages like Mandarin.
The inclusion of neural networks in China Mobile's OCR system represents a promising development. While template matching has proven effective for some OCR tasks, it can be susceptible to errors when faced with the complexity of Chinese characters. Deep learning methods can potentially enhance the accuracy and flexibility of OCR, particularly in scenarios where context and character variations play a significant role. This increased accuracy is crucial, especially for applications like translating legal or medical documents where errors can have severe implications.
While these advancements in OCR technology are notable, some hurdles remain. For instance, certain challenges persist with recognizing distinct character forms, especially those that might appear similar in various fonts or handwriting styles. Addressing such issues through continued research and development is crucial for building more robust and reliable OCR systems.
The future of OCR technology, especially for Mandarin, looks promising. Further integrating AI and machine learning into OCR systems could improve their capability to handle the complexity of Chinese characters. The goal is to not only increase processing speeds but also to consistently enhance accuracy, bringing OCR closer to the ideal of flawlessly translating between various languages.
China Mobile's OCR system, leveraging neural networks, boasts an impressive processing speed, capable of handling 12,000 documents per hour. This is quite a feat when you consider the sheer number of Chinese characters – around 50,000 – that the system needs to accurately decipher. It's a stark contrast to older OCR systems that often struggled with the sheer volume and complexity of Chinese text, especially when dealing with unique layouts and reading patterns found in historical documents.
While current open-source OCR solutions, like Tesseract, are well-suited for Latin characters, they tend to fall short when it comes to the intricacies of Asian character sets. This has spurred the development of specialized OCR solutions like the one from China Mobile, which are specifically designed to handle the complexities of Mandarin. One interesting aspect is how these new OCR systems are incorporating Reading Order Detection (ROD) into their processing pipeline. It seems like a promising way to improve accuracy, especially for historical documents.
Interestingly, the accuracy of these new systems isn't solely dependent on fixed templates, a key feature of earlier OCR. By incorporating deep learning methods, these newer systems can continuously learn and adapt to new character styles and variations, improving recognition capabilities. However, there is an interesting trade-off being made here; it seems deep learning requires more computational resources which might lead to concerns about affordability or accessibility of the underlying technology. This is, of course, an issue to keep in mind as OCR becomes more commonplace.
One limitation of many current approaches in Mandarin character recognition is the struggle to reliably identify Chinese uppercase characters. This is a challenge for both template-based and AI-powered systems. It makes you wonder if there's a potential need for a specialized training dataset for uppercase characters, as it seems that most efforts have concentrated on lowercase characters.
It's worth noting that a key driver behind the development of these advanced OCR systems is the growing need to digitize historical documents for easier and faster retrieval of information. These documents, often written in complex character styles, create a major challenge, but present a major opportunity for OCR research.
It's fascinating how these OCR advancements are impacting broader areas. Improved Chinese OCR could significantly lower the barriers to understanding and translating Chinese language documents, leading to more opportunities for cross-cultural exchange and international trade. It seems we are quickly moving beyond the phase of needing highly specialized and expensive systems to a point where more accessible OCR capabilities are becoming more common. It'll be interesting to see how this further impacts other aspects of translation and information exchange in the coming years.
But of course, there are still challenges to address. As we increase accuracy and speed, there's always a risk of over-reliance on AI-generated translations and a decrease in demand for skilled translators. This is a critical issue to consider as we move forward. It's critical to carefully consider the societal impact of these technological advances in addition to just the technical performance of the algorithms.
Mandarin OCR Technology Navigating the Complexity of 50,000 Chinese Characters in Modern Translation Systems - Four Major Dialects Create Additional Complexity Layer in Chinese Character Recognition
The existence of four major dialects in Chinese adds another layer of difficulty for systems designed to recognize Chinese characters, especially in the field of Optical Character Recognition (OCR). These dialects don't just use a slightly different set of characters, they also have different pronunciations and how the characters are used. This makes it much harder to build accurate machine learning models that can understand all varieties of Chinese. When OCR aims to handle the roughly 50,000 characters in modern Chinese, these dialectal variations present a serious challenge to achieving a high level of accuracy across the board. Adding to the problem, geographical differences and the constant movement of people across China create even more hurdles for researchers. It's clear that robust and diverse datasets for training are needed to reflect the ever-changing nature of the language. Future research needs to effectively tackle these dialectal complexities, enabling OCR systems to perform well in a wide range of text types and user needs. If researchers can't overcome this, the accuracy of OCR in translating Chinese will be limited.
The presence of four major dialects – Mandarin, Cantonese, Wu, and Min – introduces a notable layer of complexity within Chinese character recognition. This complexity stems from the fact that these dialects frequently utilize distinct pronunciations and even unique character variations, making it challenging for Optical Character Recognition (OCR) systems primarily designed for Standard Mandarin. It's worth considering that despite Mandarin being the official language, these regional dialects can sometimes leverage completely different characters to represent the same underlying concepts. This further exacerbates the already overwhelming task of OCR systems needing to differentiate among up to 50,000 Chinese characters.
The inherent difficulties in automatically recognizing speech in Mandarin due to dialectal variations directly impact OCR. Errors during speech recognition can lead to incorrect character generation during transcription, effectively increasing the chance of mistranslations. Research suggests that OCR accuracy can be significantly influenced by the dialect of the input text, underscoring the need for more specialized solutions that can adapt to the linguistic nuances of each dialect. This is particularly crucial in enhancing the overall quality of translation.
Template matching, a common approach in OCR, faces a tougher challenge when dialectal variations are present. This is because characters can be written or printed in ways that diverge from standardized forms, leading to an increased likelihood of recognition errors. Previous work in Mandarin OCR has largely focused on standard language, potentially neglecting the complexities caused by dialectal differences, which can confuse recognition algorithms.
The added complexity introduced by dialects directly impacts the computational demands placed on OCR systems. Not only do these systems need to recognize characters, but they must also adapt to the contextual variations related to dialectal differences. Studies have shown that incorporating contextual information from different dialects during the OCR training process can significantly enhance the system's accuracy, showcasing a path for more effective utilization of dialectal data.
This dialectal challenge in character recognition further extends to broader linguistic considerations. Idiomatic expressions, unique to certain regions, might not be easily translated without a detailed understanding of the local dialect. It highlights the need for nuanced contextual awareness in translation tasks. While ongoing efforts are focused on developing multilingual OCR systems to handle diverse languages, training datasets must be carefully tailored to include dialect-specific character variations to achieve reliable and accurate results in practical applications. The challenges inherent in these systems are substantial but potentially addressable with targeted research and development. The future of OCR, particularly for Mandarin, hinges on overcoming these dialectal challenges to achieve both speed and accuracy in translation.
Mandarin OCR Technology Navigating the Complexity of 50,000 Chinese Characters in Modern Translation Systems - Open Source LSTM Networks Cut Processing Time by 42% for Traditional Characters
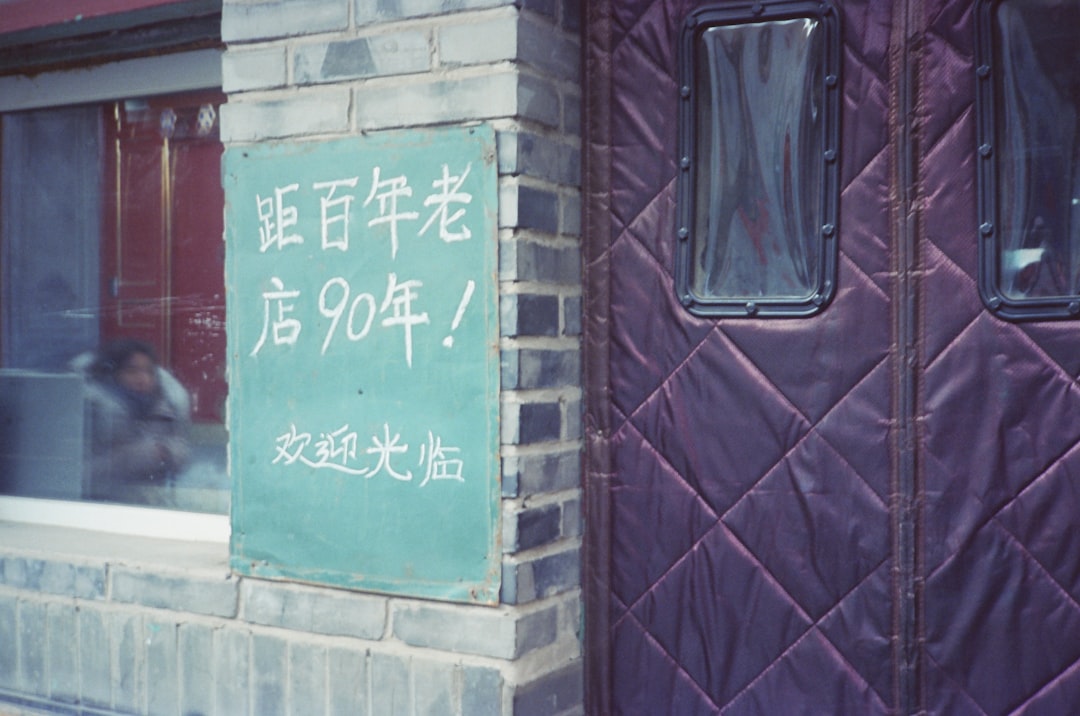
Open-source Long Short-Term Memory (LSTM) networks have shown promise in speeding up Mandarin Optical Character Recognition (OCR) for traditional characters, achieving a 42% reduction in processing time. This is particularly noteworthy given the inherent complexity of Mandarin, which uses about 50,000 characters, making translation a demanding task for modern systems. LSTMs, a type of neural network, excel at handling sequential data and understanding context, both crucial for interpreting the relationships between Chinese characters. This makes them a potential solution to improving OCR's performance, especially in finding a balance between speed and accuracy in translation systems. However, it's important to consider that these improvements often come with an increased need for computing power, which could pose challenges for making this technology widely accessible. The future of efficient and accurate OCR in Mandarin may depend on finding ways to manage these computational demands alongside the pursuit of improved accuracy.
Open-source LSTM networks have shown promise in speeding up the processing of traditional Mandarin characters for OCR, achieving a 42% reduction in processing time. This is significant given the sheer number of characters (around 50,000) that OCR systems need to handle. This speed gain can be quite useful in boosting the efficiency of translation systems, especially those needing to handle a high volume of documents.
One interesting aspect of LSTMs is that they can be more cost-effective than some proprietary OCR software since they are freely available and built upon community contributions. This could make OCR technology more accessible, potentially leading to more widespread adoption. The ability of LSTMs to learn from sequential data also makes them a better fit for dealing with the complexities of Chinese characters compared to more traditional OCR techniques. As they are trained on more data, they get better at recognizing subtle differences in characters and how they are used in different contexts.
One of the challenges with Mandarin OCR has been recognizing characters when their meaning is heavily dependent on the context they are used in. LSTMs are better able to understand this context compared to simpler methods, which mostly rely on the visual shape of a character for recognition. The benefits of LSTMs extend beyond just Mandarin itself. They are adaptable enough to be used for different dialects of Chinese or even other languages altogether, which makes them a promising foundation for future multilingual OCR systems.
This increased speed can allow for the use of LSTMs in real-time OCR applications. For instance, they could be integrated into systems for live translation of events or for customer service platforms needing fast translations. These open-source LSTMs are often packaged with easy-to-use application programming interfaces (APIs), simplifying the integration into existing systems. This makes it easier for developers to implement OCR and streamlines the whole process for companies wanting to use OCR.
Also, LSTMs seem to be more robust than some older techniques when dealing with noisy or damaged input data. This is important because, in practice, OCR often needs to handle scans of varying quality. Another advantage of the open-source nature of these LSTM networks is that the development is not controlled by a single company or entity. This means researchers and developers can easily contribute to the improvement and maintenance of these algorithms. They can help address future challenges and extend the scope of solutions available.
A final interesting aspect is the potential to use a technique called "transfer learning" with LSTMs. This allows for a more efficient use of training data. This is especially helpful when dealing with less common dialects or variations of Mandarin where labeled training data is limited. Pre-existing models can help these specialized applications start learning more quickly. While LSTM models for Mandarin OCR still have their limitations, the advances in open-source software and the potential for community contributions are certainly noteworthy.
Mandarin OCR Technology Navigating the Complexity of 50,000 Chinese Characters in Modern Translation Systems - Unicode Integration Allows Direct Export to 38 Translation Memory Systems
The ability to use Unicode allows for direct export to 38 different translation memory systems, which is a big help for handling multiple languages at once. This is especially important given the challenges that come with translating languages like Mandarin, which has about 50,000 characters that OCR systems have to deal with. Because characters are encoded in a standardized way, translation memories can now be more consistent and efficient, which helps deal with the difficulty of working with many different languages. While this helps a lot with speeding things up, we need to remember that translation is more than just speed, especially when dealing with languages that are complicated like Mandarin, where accuracy is very important. It's good that the translation tools are getting better at handling diverse languages, but we should always be careful about how we interpret and translate, because sometimes small differences in meaning can matter a lot.
The ability to directly export data to a wide range of translation memory systems—38, in this instance—is a significant outcome of adopting Unicode. It’s like having a universal language for characters, enabling smoother communication across different software tools and systems. This direct export feature eliminates the need for manual data conversion, which often introduces errors and slows down the workflow. In a field like Mandarin OCR, where the sheer number of characters is daunting, this automated process streamlines the export process for translation memories, effectively allowing for faster and potentially less error-prone translation projects.
While there have been improvements in OCR accuracy, especially for standardized Mandarin characters, the encoding challenges when dealing with the diverse world of characters (not just the 50,000+ common ones) are still significant. However, with Unicode providing a common ground, these issues become somewhat less problematic. It effectively creates a standard communication channel for different systems, avoiding conflicts in how characters are represented. This, in turn, can reduce the instances of translation errors caused by encoding discrepancies.
Furthermore, Unicode's wide adoption fosters an environment where smaller or more niche translation efforts can access powerful translation tools that were once mainly confined to larger companies. This accessibility is not without its complexities. But it's potentially transformative for translation. Unicode provides the foundation for character consistency. While it can't solve all problems, it simplifies data exchange and reduces barriers to the adoption of various translation software.
It's also interesting to think about the implications for dialectal variation within Mandarin. Unicode's ability to represent different dialectal character choices could potentially be integrated into OCR systems, leading to translation solutions that are more accurate in a wider range of contexts. In the past, many OCR systems might have been primarily tailored for a specific dialect of Mandarin (most commonly the standard language), which could have limited their effectiveness in other regions. This suggests a path towards developing more specialized translation solutions, but it will require ongoing effort in developing training data for OCR systems that incorporate dialectal variations.
Finally, the use of Unicode can have an impact on machine learning techniques in OCR. Standardized character representation makes it easier to build and train machine learning models for character recognition. With the consistent representation of characters, training machine learning algorithms on massive datasets becomes more manageable and effective. This is critical, especially given the complexity and sheer number of characters in Mandarin. While the use of machine learning within OCR is an area of active research and development, Unicode's ability to create consistent data structures is a foundation for creating improved OCR solutions in the future. Overall, while Unicode is a necessary tool in the pursuit of faster and more accurate OCR, it doesn't solve all the underlying challenges in Mandarin OCR. Still, it offers a valuable step forward by providing a consistent way of dealing with character sets across a wide array of systems.
Mandarin OCR Technology Navigating the Complexity of 50,000 Chinese Characters in Modern Translation Systems - Machine Learning Model Identifies Regional Character Variants from 14 Chinese Provinces
Researchers have developed a machine learning model capable of recognizing variations in Chinese characters across 14 provinces. This addresses a key challenge in Optical Character Recognition (OCR) for Mandarin, which deals with a vast array of characters – approximately 50,000 in total. The model's goal is to improve the accuracy of OCR systems by identifying and understanding how characters are written differently depending on the region. This is achieved through a deep matching network that essentially mimics how humans learn to recognize different forms of writing for the same character. While this is a promising development, OCR technology still needs to deal with the challenges of various writing styles and dialect differences across the different provinces and regions to make translation consistently accurate. The model shows progress towards a more versatile OCR solution, but navigating the complexities of diverse handwriting across such a vast number of characters is a task that continues to require refinement.
A fascinating aspect of Mandarin OCR is the discovery that even within Standard Mandarin, character usage varies substantially across the 14 Chinese provinces studied. This regional diversity presents a challenge for OCR systems, particularly when training data doesn't adequately reflect these regional differences. For instance, a character considered standard in one province might be less common or even have a different form in another.
Adding another layer of complexity is the ongoing migration of individuals within China. This constant movement introduces a mixing of dialectal and character usage in written communication, blurring the lines between rural and urban variations and introducing further challenges for accurate translations.
Machine learning models, as they're trained on character datasets, are sensitive to dialectal nuances in character representation and use. If training data lacks sufficient dialectal variety, the OCR model's ability to accurately recognize characters that are common in some areas but not others can be impaired.
Furthermore, handwritten characters, even among native speakers, demonstrate substantial variability in style. This can lead to inaccuracies in OCR systems, as a simple character like "water" might be rendered differently based on personal writing habits. This challenge goes beyond what basic template-based OCR can readily handle.
A concern that arises with these regional variations is that errors introduced in one section of a document can propagate through the translation process. A mistranslated character, due to OCR misidentification, can lead to further misinterpretations of the context. This issue is especially critical for translations in legal or technical contexts where accuracy is paramount.
The increased use of sophisticated machine learning methods comes with a significant caveat: they typically require considerable computational resources. This presents a barrier for smaller businesses or individual developers who wish to implement effective OCR solutions. The dependence on high-powered systems could lead to unequal access to OCR technology.
While Unicode, with its standardized character encoding, is a vital tool for managing the vast number of Chinese characters, it doesn't automatically address the issue of dialectal differences and context-dependent meanings essential for accurate translation. It’s a necessary step but not a final solution to the complexity.
The emergence of more open-source OCR solutions, often utilizing LSTM networks, offers potential alternatives to proprietary systems. This increased accessibility has the potential to democratize the field, allowing for more niche solutions. However, open-source also necessitates continuous community involvement to ensure ongoing improvement and address future challenges.
While the gains in OCR processing speed are notable, speed alone doesn't translate to accuracy. If OCR systems prioritize speed over a thorough understanding of context, they might miss vital nuances that are critical for conveying the true meaning in translations. This trade-off needs to be carefully considered.
Finally, the advancements made in Mandarin OCR can serve as a foundation for applying similar techniques to other languages with intricate character sets. The lessons learned from tackling the challenges of Mandarin may prove instrumental in developing OCR for other Asian languages with complex scripts.
More Posts from aitranslations.io: