The Evolution of AI Translation From Chatbots to Task-Completing Agents
The Evolution of AI Translation From Chatbots to Task-Completing Agents - ELIZA's 1966 Debut Paves Way for AI Translation
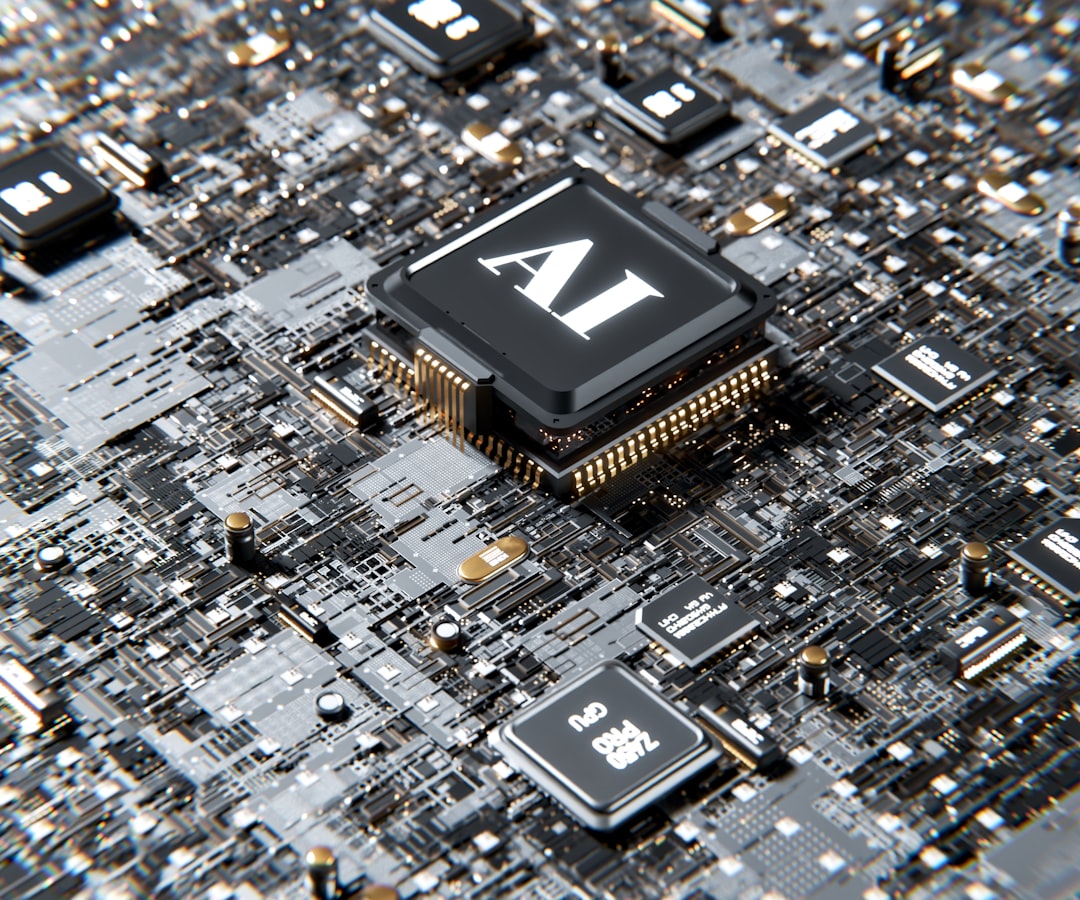
In 1966, ELIZA's introduction as the first chatbot marked a turning point in the field of artificial intelligence, laying the foundation for future progress in AI translation. Developed using a rudimentary pattern-matching approach, ELIZA simulated conversations, demonstrating the possibility of machines engaging in human-like interactions. This early attempt at conversational AI, though simplistic, paved the path for future translation tools that prioritize context and subtle meanings. The progression from ELIZA to modern AI models is a testament to the substantial leaps in natural language processing, leading to quicker and more precise translations. The evolution from basic chatbots to the sophisticated task-oriented AI agents we see today highlights the growing complexity and value of AI in overcoming language barriers.
ELIZA, a program crafted by MIT's Joseph Weizenbaum in 1966, stands out as one of the initial attempts at simulating human conversation through a computer. Its approach relied on basic pattern recognition to generate responses that, surprisingly, made users feel they were interacting with something intelligent. Despite its limited understanding, ELIZA managed to create the illusion of a conversation, primarily designed to mimic the role of a Rogerian psychotherapist. This meant its core function was to reflect user inputs back, creating a façade of empathy without any true comprehension.
Remarkably, the original code for ELIZA was surprisingly compact, fewer than 200 lines. This simplicity underscored that even rudimentary algorithms could craft the appearance of conversational intelligence, challenging the prevailing belief that complex systems were needed for human-computer interaction to be effective. While ELIZA's "therapy" capabilities were restricted, it opened doors for the burgeoning field of language processing. The program showcased that relatively simple linguistic techniques could captivate users, setting the stage for exploring the intersections of AI and translation.
Weizenbaum's creation also sparked philosophical debates about the implications of machines mirroring human interactions. Many users misinterpreted ELIZA's programmed responses as genuine understanding, prompting concerns about the potential for misinterpretation. This situation highlighted the psychological complexities inherent in human-machine interactions, including user expectations and the inherent difficulty in defining what AI should strive to emulate.
Intriguingly, the fundamental ideas behind ELIZA remain relevant. Today's sophisticated chatbots and voice assistants still rely on pre-defined patterns and contextual cues to create the illusion of a meaningful conversation, although often without genuine comprehension. While ELIZA's ability to perform complex tasks was limited, it provided a glimpse into the potential for machines to assist in specific contexts. This early concept has since evolved significantly with advancements in neural networks and machine learning, expanding into areas such as customer service and rudimentary translation.
Essentially, ELIZA's capacity to produce responses based on user input highlights a fundamental aspect of human communication: our inherent desire for validation and reflection. This core idea remains central in the development of more sophisticated AI translation and interaction techniques. The legacy of ELIZA serves as a potent reminder of how user perception can often obscure the limitations of a system—a concept still relevant in ongoing discussions about AI effectiveness in domains like translation and customer service interactions. It continues to challenge us to critically evaluate what constitutes genuine interaction in the evolving landscape of AI and its potential to aid us in the real world.
The Evolution of AI Translation From Chatbots to Task-Completing Agents - 1990s Chatbots Enhance Interaction Capabilities
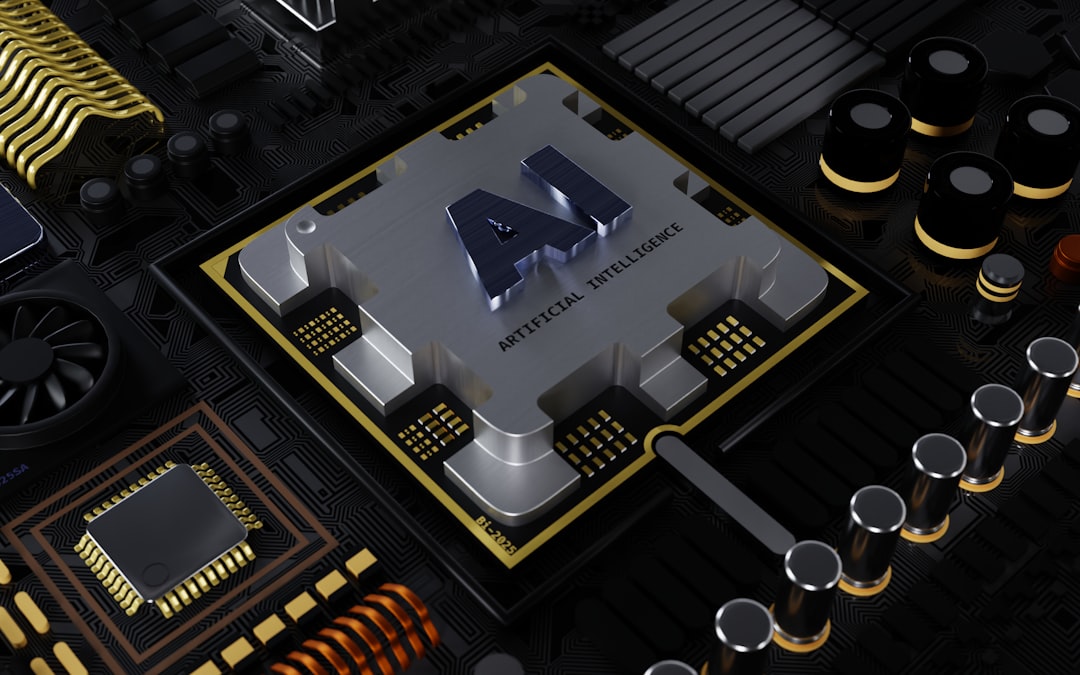
The 1990s marked a turning point for chatbots, with notable improvements in their ability to interact with users. This era brought about more sophisticated algorithms and the nascent stages of natural language processing, allowing chatbots to engage in more nuanced and relevant conversations than their earlier counterparts. Early chatbots relied heavily on pre-programmed, rule-based responses, but the 1990s saw a shift towards systems that could learn from interactions and adapt their behavior. This was a crucial step in the development of AI translation, as the demand for effective communication across languages grew. Not only did these enhanced chatbots offer more appropriate responses, but they also began to demonstrate a rudimentary understanding of context, a capability that would profoundly influence the design of AI tools for language translation. This period of advancement laid the foundation for future breakthroughs in AI translation and highlighted the potential of chatbots to bridge communication barriers.
The 1990s witnessed the emergence of chatbots like ALICE and A.L.I.C.E., which, while representing a leap forward from their predecessors, still faced limitations. These chatbots utilized extensive pattern matching to manage interactions but often struggled with context and complex inquiries, revealing the need for better natural language understanding. The expanding internet landscape during this time spurred the adoption of chatbots in customer service roles, offering businesses a cost-effective means to address high volumes of inquiries.
Researchers were simultaneously exploring rule-based systems for machine translation, mirroring the developments in chatbots. This pursuit aimed to refine how users interacted with machines to translate language without needing significant human intervention—a critical step towards automation. However, early chatbots struggled with slang, idioms, and cultural nuances, resulting in inaccurate translations and frustrating user experiences. A deeper understanding of linguistic intricacies became apparent for improving user interactions.
The 1997 launch of "Julie", the first commercial chatbot, showcased the potential of integrated translation within a conversational interface. This provided users with rudimentary support across languages, hinting at the advanced multilingual tools we have today. Optical Character Recognition (OCR) advancements during this era offered the prospect of chatbots deciphering text from scanned documents, hinting at a future where visual and textual data are seamlessly integrated within translation services, enhancing user experience.
The late 1990s also marked the beginning of web-based translation tools relying on rudimentary chatbot frameworks. This emphasized the importance of real-time interaction and the need for speed and precision, especially in dynamic online environments. Despite advancements, chatbots of this era were heavily reliant on predetermined conversations, hindering their ability to learn from user feedback. This constraint prevented them from achieving a smooth, adaptive communication experience, which was crucial for more sophisticated translation services.
Voice recognition technology, though nascent, was starting to be incorporated into chatbots. This foreshadowed the integration of spoken language translation, a technology that has since been significantly advanced by modern AI. By the end of the 1990s, the inadequacies of these early chatbots became apparent, necessitating a shift towards more dynamic, adaptive systems. This evolution was critical for navigating the complexities of translation in a world increasingly reliant on global connections.
The Evolution of AI Translation From Chatbots to Task-Completing Agents - Neural Networks Boost Language Processing Accuracy
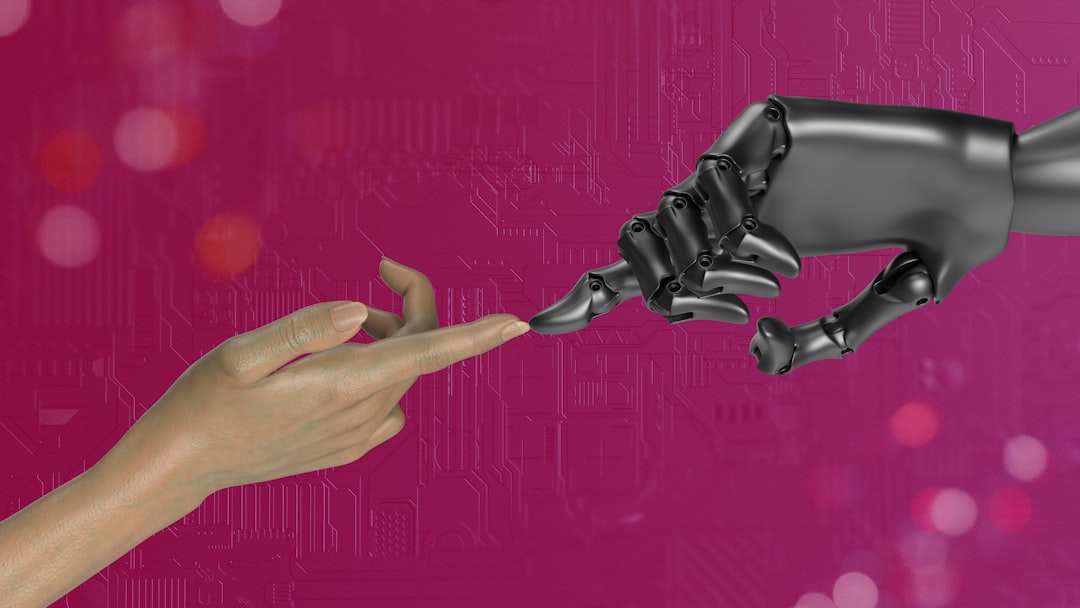
The rise of neural networks has revolutionized how computers handle language, particularly in the field of machine translation. Neural machine translation (NMT), powered by deep learning, has proven far more accurate than older methods like rule-based systems. These new systems can process massive amounts of language data, leading to translations that are more nuanced and contextually aware. This approach, known as end-to-end learning, tackles a key limitation of previous systems—their struggles with the complexities of language. By handling the entire translation process within a single neural network, NMT promises to bridge the gap between human and machine understanding of language. While ongoing research explores the full potential and challenges of NMT, it has shown remarkable promise in making language barriers less significant. This exciting development continues to drive innovation in AI translation, shaping a future where communicating across languages becomes significantly easier and more accessible for everyone. This evolving landscape underscores the crucial role of continual progress in shaping how we approach translation tasks and the potential for a future where languages are less of a barrier to communication.
Neural networks have fundamentally altered how computers process languages, particularly in translation. They leverage deep learning, allowing systems to grasp context and nuances that were previously challenging for older, rule-based methods. This approach results in translations that often feel more natural, reflecting subtle meanings that were previously lost.
One major leap forward was the emergence of the Transformer architecture in 2017. This novel neural network design notably improved machine translation by handling the intricate relationships between words across longer stretches of text.
Intriguingly, certain neural machine translation (NMT) systems are now reported to surpass human translators in specific language pairs and fields. They excel in consistently producing translations quickly, especially beneficial for high-volume tasks.
The use of neural networks has also propelled the progress of Optical Character Recognition (OCR). By employing convolutional neural networks, OCR systems can now interpret diverse fonts and even handwritten text with remarkable accuracy—often exceeding 90%.
However, not all languages experience the same benefits from these advanced translation techniques. Languages with limited data, also known as low-resource languages, struggle with accurate translation. It underscores the need for ongoing research and development to ensure more equitable access to AI-powered translation across all languages.
Surprisingly, cultural factors are starting to be integrated into these language models. By considering cultural context, translations can resonate better with their target audiences, going beyond simple word-for-word replacements.
Google's incorporation of neural networks in their translation system is a telling example. It's estimated that translation speeds have increased by roughly 20% compared to earlier methods. This reveals the potential for AI to significantly improve efficiency in translation tasks.
Despite their advancements, these systems are not without limitations. A common challenge is that they can produce sentences that seem grammatically correct but lack factual accuracy. This demonstrates that even complex AI models struggle to perfectly grasp context in difficult scenarios where facts and logic are crucial.
The design of these neural network architectures has resulted in task-specific AI models. For instance, a model trained exclusively on legal texts excels at translating legal documents compared to more general AI translation tools. This hints at the promise of developing tailored AI tools for specialized translation needs.
It’s also encouraging that user feedback is now being used to improve AI translation systems. These neural networks can learn from users' corrections and preferences, establishing a feedback loop to continuously enhance translation quality. This ongoing refinement is a testament to the potential of AI to improve over time, becoming more attuned to the intricacies of human language.
The Evolution of AI Translation From Chatbots to Task-Completing Agents - AI Agents Expand Beyond Conversation to Task Completion
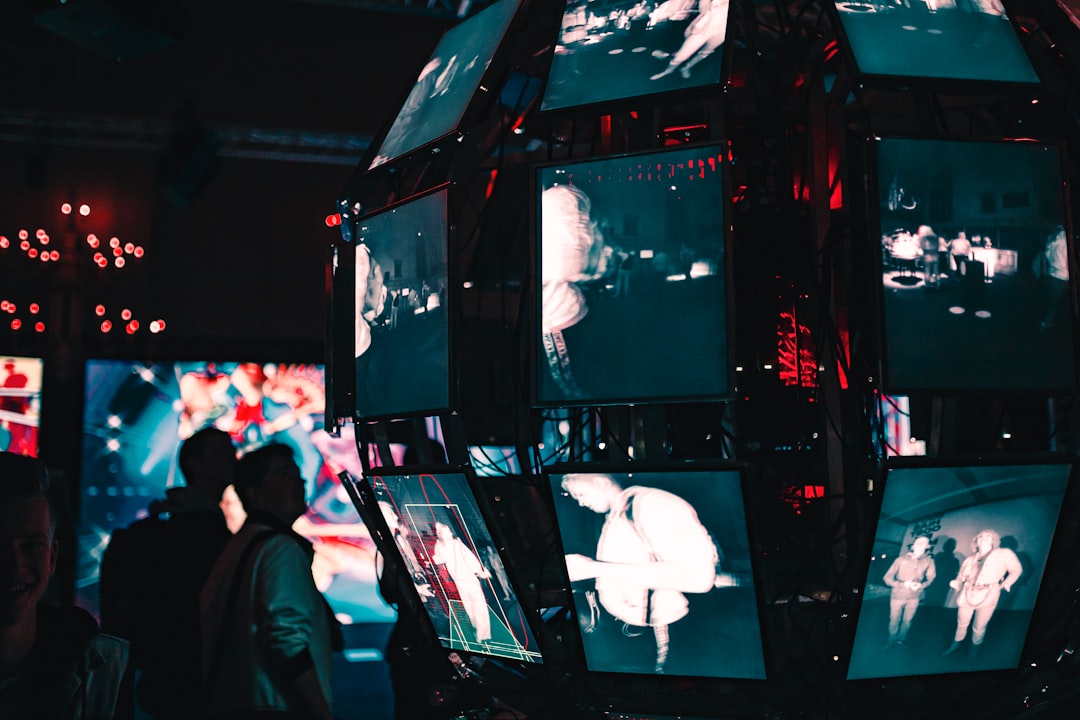
AI agents are no longer confined to simple conversations; they're evolving into powerful tools capable of independently completing complex tasks. This shift underscores their potential to optimize workflows and boost individual productivity, making them increasingly valuable across various domains. These agents rely on the advanced language processing capabilities of large language models to tackle intricate tasks, breaking them down into smaller, manageable steps and seamlessly interacting with digital environments. Unlike their conversational chatbot predecessors, which focus mainly on dialogue, these agents are specifically designed to achieve defined goals. This includes automating procedures and, in the context of translation, optimizing tasks for speed and quality. The growing complexity of AI agents offers exciting possibilities for improving user experience and efficiency, particularly in translation where rapid and accurate results are essential. This advancement could revolutionize the way we interact with translation tools, potentially making them faster and more accurate than before.
AI agents have progressed remarkably beyond their initial conversational role, now capable of independently completing multifaceted tasks. This shift from mere chatbots to task-oriented agents highlights a new frontier in AI, with the potential to streamline various processes, including translation. Take, for instance, the advancements in OCR. AI-powered OCR tools now leverage deep learning, achieving impressive accuracy rates – sometimes surpassing 90% – when processing diverse text formats, including handwritten inputs. This increased accuracy and speed offers a significant advantage over manual data entry for translation purposes.
Another interesting development is the growing ability of AI translation systems to incorporate user feedback. This iterative process allows the agents to refine their translations over time, adapting to individual preferences and needs. The result is a more nuanced and tailored translation experience. However, these systems still face challenges when dealing with languages with limited digital resources. The scarcity of training data for what we call "low-resource languages" presents a major hurdle in achieving equitable access to AI-powered translation across all language groups.
Furthermore, AI translation has evolved to encompass a more holistic understanding of language. These advanced systems now strive to grasp the broader context of a phrase or passage, producing more natural-sounding translations that maintain the original intent and tone. Building upon this, some systems are even incorporating cultural context into their models. This development allows for translations that better resonate with the target audience, going beyond a simple word-for-word replacement.
Researchers are also experimenting with developing task-specific AI agents. These models, trained on specific industry datasets (such as legal or medical texts), can deliver more precise and specialized translations compared to general-purpose AI translation tools. In certain situations, some AI translation systems have even outperformed human translators in speed, which is highly advantageous for high-volume tasks or real-time applications.
Despite these successes, limitations remain. While neural networks have enhanced semantic understanding, they can sometimes generate grammatically sound sentences that are factually incorrect. This issue underscores the importance of human oversight, particularly in situations where factual accuracy is critical. Moreover, the introduction of the Transformer architecture has been a game-changer in the field. This innovative approach, initially conceived for translation tasks, has since impacted various domains within AI, signifying a crossover of techniques.
The continuous evolution of AI agents signals a profound shift in human-machine interaction. As they become more adept at handling complex tasks, we can anticipate a future where language barriers are significantly diminished. However, it's crucial to remain mindful of the potential drawbacks and biases that may exist within these sophisticated systems. Maintaining a critical perspective and focusing on ethical considerations will be vital as AI continues its rapid advancement in areas like translation.
The Evolution of AI Translation From Chatbots to Task-Completing Agents - Web-Integrated Chatbots Offer Real-Time Information Access
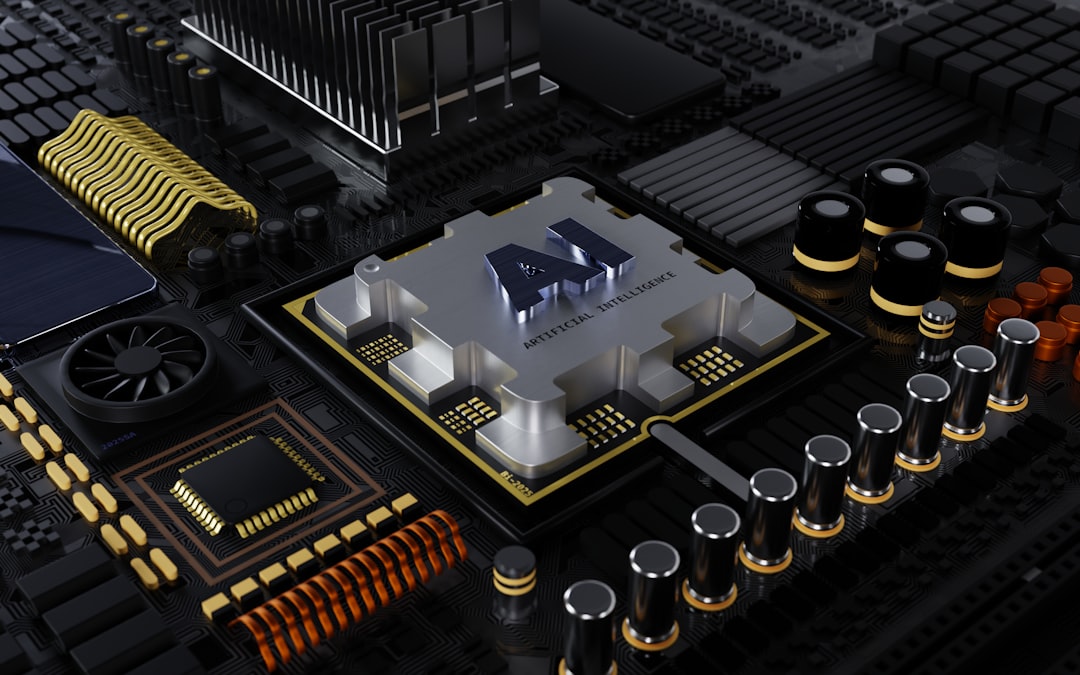
Web-integrated chatbots have become vital for accessing information in real time. They are now embedded within websites and apps, enabling users to quickly obtain help and data. These chatbots utilize AI capabilities to understand and respond to user requests in a natural way, fostering smoother interactions. This feature makes them valuable across various applications like translation or customer service where quick, interactive solutions are important. The use of natural language processing allows chatbots to not only provide information but also to interpret complex questions and offer tailored responses. This marks a significant evolution towards efficient and readily available solutions in our digitally connected world. Yet, it also highlights the importance of recognizing and addressing limitations. Ongoing evaluation and refinement of these chatbots is needed to maintain the quality and dependability of the information and assistance they offer, as the field is rapidly evolving.
Web-integrated chatbots are becoming increasingly valuable for accessing information in real-time, particularly when dealing with language barriers. These systems can provide immediate translation during online interactions, which is beneficial for businesses operating across various language groups and individuals navigating global communications. For instance, a traveler could utilize a web-integrated chatbot to get quick translations during a trip, facilitating smooth interactions with local vendors or service providers.
While the implementation cost for such chatbots can be relatively low, often starting in the hundreds of dollars, the actual capability can vary greatly depending on the design and desired functionality. This makes such technologies potentially accessible to smaller businesses, but they still require careful consideration of the intended applications.
The improvements in Optical Character Recognition (OCR) are particularly interesting. Modern chatbots can now effectively decode text from images or documents, including handwritten materials in many languages. In numerous instances, OCR accuracy rates exceed 90%, significantly streamlining processes where scanned or image-based information needs translation.
Another intriguing development is the capacity of some chatbots to manage multilingual conversations. Imagine a scenario where the chatbot seamlessly transitions between several languages within a single interaction – it highlights a real-time translation aspect. However, the actual range of languages each chatbot can handle varies. While large language models can process hundreds of languages, their accuracy can differ greatly. Popular languages with ample training data tend to fare better than those with fewer resources, showing a bias in the current technological development.
Neural network-based systems have demonstrably decreased translation latency in certain chatbots. This reduction, often around 20%, is particularly notable in applications that demand fast information retrieval. However, research suggests that the performance of chatbots can match or surpass humans in specialized translation fields, like medicine or engineering, where precise terminology is paramount. This shows that AI's application in specific niches can produce superior results.
The ability to capture sentiment and context within the original message is another plus for these tools. By doing so, chatbots can produce translations that effectively convey not only the words but also the underlying emotional or contextual tone.
Furthermore, users interacting with web-integrated chatbots contribute to a constant feedback loop, which can refine the translation quality over time. The chatbot learns from user corrections and preferences, leading to a more tailored and personalized translation experience.
Despite these advancements, it's crucial to acknowledge that chatbots still generate translations that may be grammatically sound but contextually flawed at times. This emphasizes the importance of human review, especially when accuracy is critical. For example, sensitive topics, legal documents, or medical instructions should still be vetted by human experts due to the current limitations of these AI tools.
The integration of chatbots and AI into our online experience continues to evolve rapidly. While they present great opportunities to break down language barriers, these advancements should also be critically evaluated. Continued research and development in this field remain crucial to ensure that the future of translation technologies serves the widest range of languages and users while being consistently mindful of potential biases or errors.
More Posts from aitranslations.io: