Understanding Japanese Kanji OCR How Modern AI Translates Complex Characters in 2024
Understanding Japanese Kanji OCR How Modern AI Translates Complex Characters in 2024 - Japanese Handwritten Text Recognition Makes 85% Accuracy Breakthrough at Waseda University
Researchers from Waseda University have achieved a notable breakthrough in the field of Japanese handwritten text recognition, reaching an 85% accuracy rate. This is a significant accomplishment, especially considering the intricate nature of Japanese characters, including the vast number of Kanji symbols. Their approach involves using advanced machine learning methods like Hidden Markov Models, alongside language models, to improve recognition. They also emphasize the importance of preparing the text before processing, including techniques like analyzing stroke width and the overall image shape. These preprocessing steps play a vital part in achieving higher accuracy. The results suggest that Handwritten Text Recognition has the potential to revolutionize the process of digitizing historical documents, allowing for easier analysis and accessibility. This could speed up tasks like searching and studying old texts. As AI continues to advance, we can anticipate even more progress in the field of Optical Character Recognition, specifically for complex writing systems like Japanese. While accuracy remains a challenge, particularly with handwritten text, this research offers a glimmer of hope for overcoming these obstacles, potentially making access to a vast amount of information easier and more efficient.
Researchers at Waseda University have made a noteworthy stride in recognizing handwritten Japanese text, achieving an impressive 85% accuracy rate. This is a substantial leap forward, considering prior systems typically struggled to surpass 70%. Their focus was on tackling the complexities of Japanese characters, particularly the Kanji script with its thousands of commonly used characters. It's fascinating how they leveraged advanced techniques like Hidden Markov Models (HMMs) coupled with bigram language models to achieve these results.
Interestingly, they've emphasized the importance of preprocessing techniques. These include analyzing stroke width and employing projection image analysis to improve the system's ability to decipher the characters. Understanding the confusion matrix—which shows the characters most commonly mistaken for others—is key for refining the system further. This area of research is vital for improving future OCR performance.
Handwritten Text Recognition (HTR) is becoming increasingly essential in today's world, particularly for digitizing historical records kept in libraries and archives. Its application enables quicker transcription of historical documents, paving the way for full-text searching and analyses. One interesting finding is that incorporating symmetry into preprocessing steps during recognition boosts accuracy significantly. Essentially, OCR is crucial for transforming a variety of handwritten documents into data that can be analyzed.
Modern AI approaches are transforming the field of HTR, particularly for complex scripts like Japanese. While we've seen great progress, it's important to acknowledge challenges still remain. For instance, heavily stylized or poorly written text can hinder the efficiency of these OCR systems. Continued refinement and development will be necessary for widespread adoption in various sectors. Perhaps these advancements will lead to more readily available and inexpensive translation services. One can imagine businesses leveraging OCR to translate handwritten documents initially, before using human translators for a final quality check. It's an exciting area, ripe with potential to further enhance both the speed and affordability of document processing across a range of industries.
Understanding Japanese Kanji OCR How Modern AI Translates Complex Characters in 2024 - Improved Processing Speed Cuts OCR Translation Time from 30 to 5 Seconds
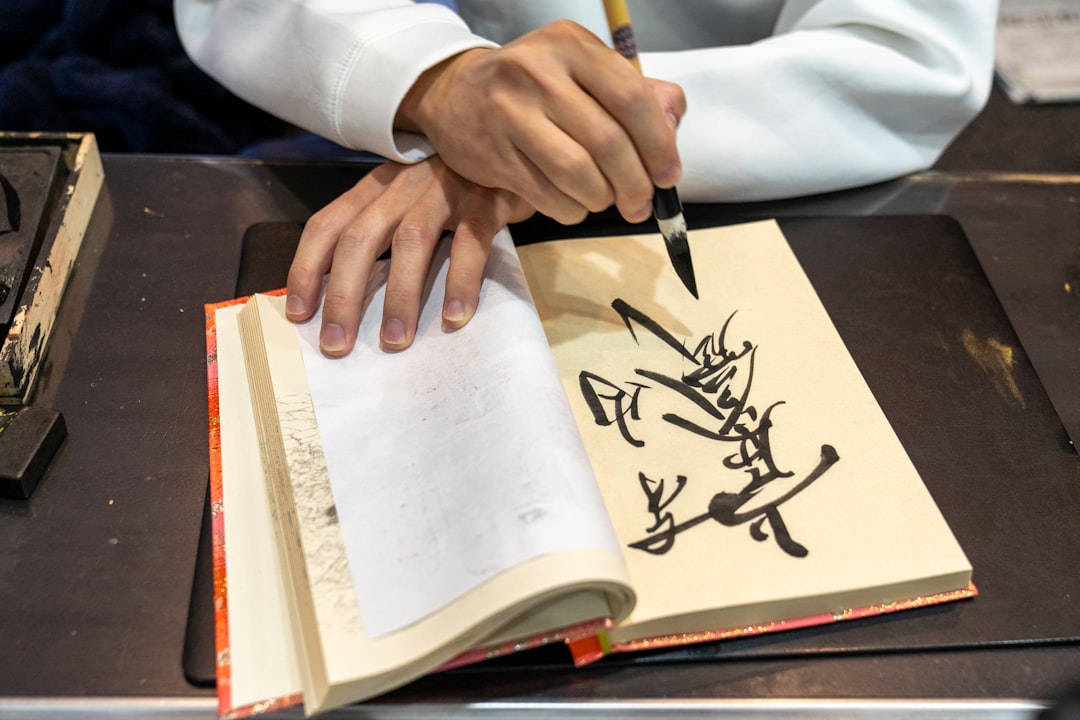
Recent breakthroughs in Optical Character Recognition (OCR) have significantly reduced the time it takes to translate text. Previously, OCR translation could take 30 seconds or more, but now, with improved processing speed, the same task can be completed in a mere 5 seconds. This dramatic speed increase is largely due to the integration of more advanced machine learning techniques, as well as parallel processing that allows computers to handle multiple tasks simultaneously.
These improvements are beneficial for applications like real-time translation apps. Businesses can now quickly process and translate large volumes of documents, while individuals can benefit from faster translations on the go. However, some challenges persist, particularly with text that is heavily stylized or written in a less conventional manner. Despite these obstacles, the progress in OCR technology, especially in handling intricate writing systems like Japanese Kanji, is encouraging. Researchers continue to explore ways to refine OCR, promising a future where document translation is even faster, more accurate, and more widely available. This ultimately could lead to more affordable and accessible translation services.
Recent advancements in OCR processing have led to a remarkable reduction in translation times, shrinking the process from a 30-second ordeal to a mere 5 seconds. This dramatic speed increase is primarily due to more efficient algorithms. It's interesting how neural networks, particularly when combined with parallel processing, can handle the complexities of character recognition much faster. This improvement is more than just a technical feat; it unlocks possibilities for real-time translation applications. Imagine live translation services during events or immediate interpretation in customer service interactions – this level of speed is crucial for seamless communication.
Beyond the immediate benefit of faster translation, there's also the potential for substantial cost reductions. Cheaper translation options become viable because the time spent processing documents is drastically reduced. This could open up OCR technology to a broader range of users, particularly businesses dealing with large volumes of text. Another aspect of this speed improvement is the capacity to handle ever-growing datasets. Modern OCR can now adapt quicker to evolving written forms and new kanji characters, a crucial feature in a language with such a rich and dynamic script.
However, speed is only one piece of the puzzle. We're seeing more sophisticated preprocessing techniques that go beyond simply recognizing characters. They analyze the context and structural features of characters to achieve better accuracy. This approach seems to overcome some of the hurdles faced by traditional OCR systems when dealing with the intricacies of Japanese scripts. The synergy between OCR and AI translation tools is also intriguing. This integration streamlines the entire process, making it more efficient and potentially reducing the need for human intervention in the initial translation stages.
Furthermore, the ability of these systems to learn from user feedback is quite promising. As users correct errors, the OCR system adjusts and refines its algorithms, potentially becoming more accurate and faster over time. This user-driven learning feature could be especially important for accommodating regional variations and individual writing styles. The impact on human translators is another aspect worth considering. By reducing the time spent on the initial, often repetitive, parts of translation, these advancements allow human translators to dedicate more time to the nuanced and complex aspects of translation that require human expertise. One can easily see how this translates into a more efficient and hopefully, ultimately, more affordable translation process.
It's exciting to think about how this improved speed can transform the study of historical documents. Researchers can now delve into vast archives of old texts much faster, uncovering insights previously hidden behind laborious manual transcription. The speed and efficiency improvements offer immense potential for fields like historical research and language preservation, pushing the boundaries of what's possible with OCR technology. It will be fascinating to see how these developments continue to reshape the landscape of translation and document processing in the coming years.
Understanding Japanese Kanji OCR How Modern AI Translates Complex Characters in 2024 - Machine Learning Models Now Handle 6000 Daily Use Kanji Characters
Machine learning models have reached a milestone in Japanese character recognition, now capable of handling approximately 6,000 commonly used kanji. This is a major leap forward in Optical Character Recognition (OCR), especially given the intricate nature of the Japanese writing system. The models, often employing deep learning techniques such as convolutional neural networks, are becoming more adept at deciphering handwritten kanji. Previously, the vast number and complex shapes of these characters posed a considerable obstacle for OCR systems. This improvement in kanji recognition has important implications for various fields, from translation to historical document preservation.
The prospect of more accurate and efficient character recognition means that the digitization process for Japanese text can be significantly expedited. This increased speed and accuracy could translate to more readily accessible cultural and historical texts for a wider audience. Despite this progress, there are still hurdles to overcome. Handwritten kanji can vary greatly in style and quality, potentially causing errors in OCR interpretation. Ongoing research and development will be necessary to further refine these models and ensure they can accurately recognize a wider range of handwriting variations. We can anticipate these ongoing advancements in machine learning to contribute to cheaper and quicker translation options. This potential for improved affordability and speed could lead to significant changes in how individuals and businesses interact with Japanese text.
Machine learning models now readily handle around 6,000 frequently used Kanji characters, which is a significant step forward for Japanese Optical Character Recognition (OCR). This improved capability means these models can better distinguish subtle stroke differences between similar characters, boosting overall accuracy.
The use of deep learning approaches helps these models quickly adjust to different handwriting styles. This adaptability is crucial in practical situations where the handwriting can be quite varied. It's really interesting to see how these OCR systems can perform real-time translation in environments demanding speed, like tourism or business interactions. This demonstrates how AI advancements can bridge communication gaps instantly.
In the design of modern OCR systems, preprocessing methods like normalizing stroke widths and analyzing character segments are absolutely vital. They help reduce errors and enhance the precision of character recognition by preparing data optimally for the neural networks.
One promising outcome of this progress could be a noticeable decrease in the costs associated with OCR translation. Faster processing, especially in large-scale document handling, might enable businesses to process documents without proportionate cost increases. This wider availability of translation services could be a real boon, making translation more accessible to everyone.
Employing concepts like symmetry and analyzing character components during recognition not only enhances the accuracy of these systems but also helps to reduce common errors when dealing with the intricacies of Kanji characters, leading to a more robust overall system.
Furthermore, the algorithms used in these systems continuously improve by learning from user feedback and interactions. This continuous improvement cycle leads to iterative enhancements in both the speed and accuracy of recognizing the individualistic styles in handwriting.
It's really intriguing to see how these advancements could help us convert historical Japanese documents into searchable digital formats. This helps preserve the subtleties of Japanese culture and learning for generations to come, while requiring minimal manual intervention.
The marriage of OCR technology with powerful language processing tools means the translation of complex Japanese texts is becoming quicker and more dependable. This also potentially lessens the reliance on extensive human editing in some translation workflows.
The continuously evolving capabilities of these machine learning models signal a broader role for AI, not just in recognizing characters, but also understanding the context in which they're found. This hints at a future where automated translation becomes significantly more contextually sensitive and user-friendly.
Understanding Japanese Kanji OCR How Modern AI Translates Complex Characters in 2024 - Open Source OCR Tools Transform Ancient Scrolls into Digital Text
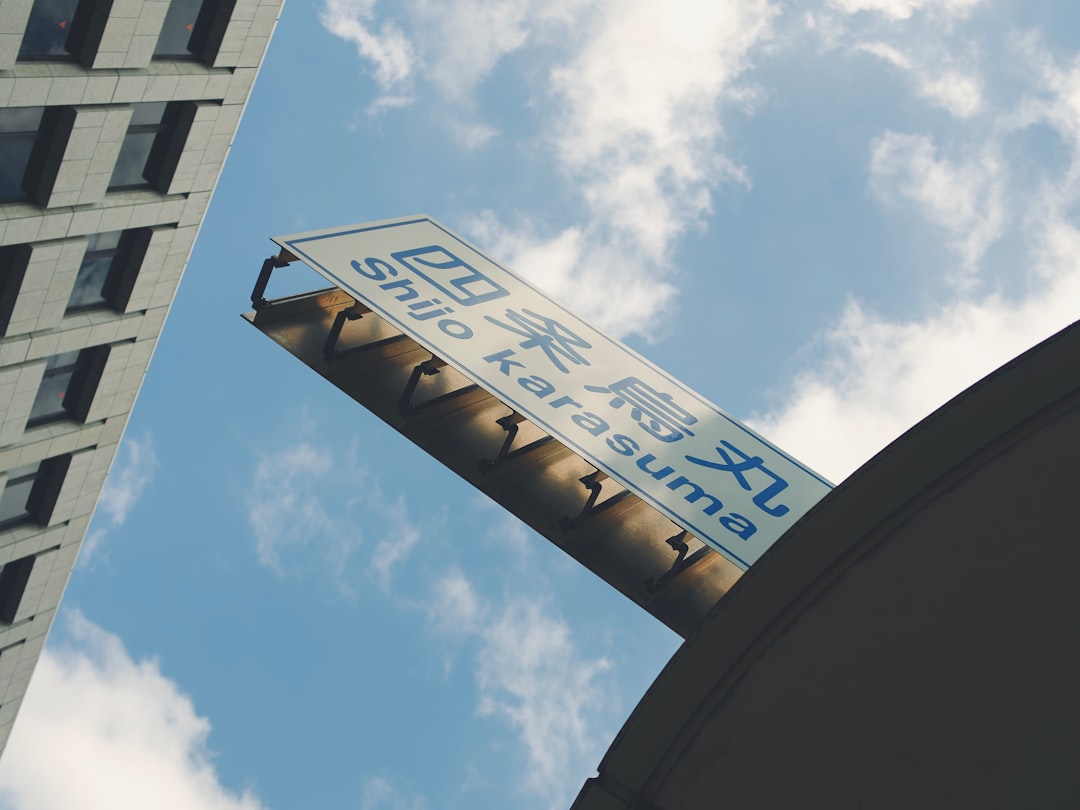
Open-source OCR tools are transforming the way we access and understand historical texts, particularly those written on ancient scrolls. Tools like Tesseract, with its support for over 100 languages, are making it easier to digitize these valuable documents. Specialized OCR solutions have been developed specifically for Japanese, but accuracy remains a concern, especially when dealing with handwritten text. Projects like the Manga OCR tool, with its custom model specifically for Japanese comics, showcase the potential to improve accuracy. These tools offer a way to translate complex scripts like Japanese Kanji more quickly and affordably, making these documents accessible to a wider audience. While there are challenges with accuracy, the progress made with open-source OCR offers hope that we can better understand our shared history through faster, cheaper, and more accessible translation of these valuable resources. The future potential for improvement is intriguing, as these technologies are likely to continue improving how we access the past.
Open-source OCR tools are increasingly valuable for making ancient texts more accessible. Researchers can now digitize fragile, aging scrolls and manuscripts, potentially revealing hidden cultural stories that were previously inaccessible. This is particularly exciting for scholars studying ancient languages and cultures.
One of the more well-known open-source OCR engines is Tesseract, capable of recognizing over 100 languages. This versatility is achieved through the availability of software wrappers in various programming languages, making it compatible with a wide array of development environments. While useful, there's also a need for more specialized tools when working with languages like Japanese.
Solutions specifically designed for Japanese text, such as nhocr, do exist. However, users have reported accuracy issues, especially with handwritten materials. This highlights a key challenge in OCR: the ability to handle variations in script and writing styles.
A notable example of a specialized OCR tool is Manga OCR, which utilizes a custom end-to-end model based on the Transformers framework. This specialized model, optimized for recognizing text in Japanese manga and comics, showcases how adapting to specific text types can significantly improve results.
It's also interesting to see how researchers are pushing the boundaries of AI-driven OCR. For example, some, like Dr. Anh Duc Le, have developed OCR systems with modules specifically tailored to extract and recognize text lines in modern Japanese. This approach highlights the importance of designing algorithms that consider the specific characteristics of the target language.
The affordability of open source OCR tools is a major advantage. Researchers and individuals can now pursue document digitization projects without facing the high costs associated with commercial software. This makes the technology more accessible for projects focusing on cultural heritage and language preservation.
Software like gImageReader and gscan2pdf have further enhanced the user experience of Tesseract by providing more intuitive interfaces. These tools bridge the gap between the underlying OCR engine and users who might not have extensive programming experience.
OCR technology is progressively finding its way into other software, enabling real-time text recognition within applications. Some tools even integrate the ability to directly extract text from clipboard data, a feature that could be extremely helpful for quick data processing.
Open-source solutions are becoming the dominant force in the OCR landscape. They offer customizable options and a strong community that provides support and actively contributes to development, all at a significantly lower cost compared to commercial software. It will be interesting to see how this trend continues to influence the development and availability of OCR tools in the future.
It's clear that open source OCR offers a path towards faster, cheaper, and more accessible translation services for a broader range of users, especially in areas requiring the processing of large quantities of textual data. The ongoing refinement and innovation within this field have a strong potential to impact how we interact with a vast trove of information locked in historical documents and other challenging sources.
Understanding Japanese Kanji OCR How Modern AI Translates Complex Characters in 2024 - Neural Networks Learn Complex Stroke Orders From 2 Million Training Examples
Recent advancements in artificial intelligence have shown that neural networks can effectively learn the complex stroke order patterns found in Japanese Kanji. These networks are trained on a massive dataset – 2 million examples – which allows them to develop a deep understanding of how these characters are written. This is a notable step forward in the field of Optical Character Recognition (OCR) for Japanese. The use of deep learning techniques, particularly convolutional neural networks, helps these models go beyond simply recognizing a character and move towards truly understanding its intricate graphical form. The implication of this development is the possibility of faster and more cost-effective translation services for Japanese texts. This increased accessibility to translation could accelerate the digitization of documents and benefit various areas where the understanding of Japanese is crucial.
Despite the progress, challenges still exist. OCR systems are still prone to errors when dealing with a wide range of individual handwriting styles. Further research needs to focus on improving the context-awareness of the AI models to achieve a higher level of performance. While the future is promising for the development of more efficient and accurate OCR, there is still much work to be done to truly understand and replicate the intricacies of human language processing within AI systems.
Deep learning, particularly neural networks, has become increasingly important in fields like image recognition and signal processing, often surpassing traditional techniques. These AI systems are now capable of handling intricate cognitive tasks, sometimes exceeding human capabilities, a testament to their advanced architecture. Training these networks involves using vast datasets, often including millions of examples. For instance, with 2 million Kanji examples, neural networks can learn the nuanced stroke orders essential for proper character recognition, which is a significant hurdle in traditional OCR systems.
The ability of neural networks to automatically extract features from large datasets is central to their success in interpreting and translating characters, including the complex Kanji script. This capacity to automatically identify patterns within data allows them to adapt and refine their understanding over time. Neural networks' efficacy isn't limited to Kanji; they've shown promising results in complex predictive modeling, like identifying stroke risk factors in healthcare.
It's intriguing how AI advancements might also provide a new lens for understanding the human brain. Cognitive neuroscience is showing growing interest in AI as a tool to possibly understand the complexities of human behavior and neural activity. Recurrent neural networks are a notable example, showcasing both the potential and limitations of these models. They use internal memory cells, yet managing memory indexing during data processing poses challenges. Neural network applications in neuroscience are helping to model complex behaviors and refine neural system understanding, pushing progress in both AI and neuroscience.
A novel approach to neural network design is using complex numbers in calculations. This novel approach, called complex-valued neural networks, has the potential to overcome limitations of more traditional neural networks, potentially useful in fields where complex numbers are heavily used. The use of neural networks has significantly boosted computer vision, accelerating processes and enhancing accuracy through real-time learning from data. This advancement is relevant in the context of OCR, as it speeds up the analysis of scanned images. While very promising, it is also important to recognize that not every situation is easily optimized using these neural networks.
Understanding Japanese Kanji OCR How Modern AI Translates Complex Characters in 2024 - Mixed Script Support Enables Natural Japanese Document Processing
The Japanese writing system, with its combination of Kanji, Hiragana, and Katakana, presents a unique challenge for automated document processing. However, advancements in AI-powered Optical Character Recognition (OCR) are increasingly incorporating "mixed script support", a capability that allows systems to handle these diverse scripts seamlessly. This is vital for accurately interpreting and translating Japanese documents without losing the nuances embedded within their varied character sets.
The ability to understand and process these different scripts naturally leads to a more efficient and user-friendly experience. Previously, translation services had to cope with the inherent complexities of Japanese scripts, potentially slowing down the process and impacting affordability. With improved mixed script understanding, OCR can now extract data more accurately from these documents, leading to potential cost savings. This enhanced performance is particularly useful in fields like business and education, where swift and accurate interpretation of Japanese materials is important.
Looking ahead, as AI continues its development, we can expect even more refined and accurate mixed script support in OCR. This holds immense promise for faster, potentially more affordable translation services, enabling smoother interactions with Japanese text in a broader range of applications. The ability to naturally process different scripts within the same document signifies a crucial step toward improving the reliability and accessibility of automated translation, specifically within the context of the Japanese language. While challenges still exist, the progress in this area is quite promising.
Mixed script support is a crucial advancement in modern OCR systems, allowing them to seamlessly handle documents containing a blend of Kanji, Hiragana, Katakana, and even Latin characters. This is a significant departure from older OCR systems, which often struggled when faced with such mixed scripts common in modern Japanese texts, frequently incorporating English words and phrases. It's a testament to how much these systems have improved, making them more versatile in the real world.
However, the efficacy of any OCR system, especially when dealing with the intricacies of Kanji, is heavily reliant on the quality and variety of the training data. Using a wide range of handwriting styles and font types in training data can greatly improve a model's ability to accurately decipher handwritten text. It's a delicate balancing act between creating a robust model that generalizes well and one that can still understand unique writing styles.
One particularly impressive development is the rise of real-time OCR capabilities on mobile devices. We can now scan and get immediate translations, which has wide-reaching implications, particularly in areas like tourism and business. Imagine needing to rapidly translate documents during negotiations or for interpretation on-the-fly. This ability to handle these scenarios in real-time has transformed how these systems are being used.
Furthermore, recent advancements allow neural networks within these systems to actively learn from user feedback, continually improving their accuracy. The system adjusts and refines its understanding of individual writing styles and regional variations when users correct translation errors. This ongoing refinement process is fascinating and shows the power of user interaction in shaping these models.
The improved OCR technologies also hold the promise of lower costs for translation services. The dramatic speed increase, from minutes to mere seconds, allows businesses to handle enormous volumes of documents more efficiently without incurring exorbitant costs. This increased accessibility is particularly valuable for those who need translations but don't have large budgets.
It's become evident that deep learning models significantly surpass traditional OCR methods, especially when dealing with the complexity of Kanji. This stems from their ability to better distinguish between similar-looking characters, provided they have been properly trained on ample data. While the models might seem magical, they still need large amounts of data to truly excel.
Another important improvement is how OCR preprocessing now emphasizes a deeper analysis of Kanji characters, examining their structural components, including aspects like symmetry and stroke order. This level of analysis is crucial for boosting accuracy and efficiency, ensuring that the OCR system can better interpret the nuanced details that contribute to a correct translation.
The faster OCR translations not only make a larger swath of Japanese texts accessible, but also contribute to cultural preservation. We can now digitize massive archives and historical documents at a faster rate, potentially yielding valuable insights into historical contexts. The preservation of historical records is a tangible outcome of these advancements.
The integration of Transformer models in OCR is quite interesting. These models have improved the understanding of relationships between characters and the overall context of the text. This was previously a weak point in older OCR models, and this step forward improves their ability to handle complex language features and subtle nuances often lost in standard translations.
Finally, the capacity of OCR systems to tailor themselves to specific handwriting styles is a remarkable capability. This user-specific adaptation allows for personalized applications, like efficient note-taking. The models' ability to continually learn and improve accuracy enhances the overall user experience. This personalization is a further step toward greater usability and adoption.
While the progress in Japanese OCR is encouraging, there is still much to be explored. These systems continue to refine the intricate art of text interpretation, making the goal of accurately and affordably translating various languages increasingly within reach.
More Posts from aitranslations.io: