AI-Powered PDF Translation now with improved handling of scanned contents, handwriting, charts, diagrams, tables and drawings. Fast, Cheap, and Accurate! (Get started for free)
The Evolution of Translation From Ancient Clay Tablets to Modern AI Language Models
The Evolution of Translation From Ancient Clay Tablets to Modern AI Language Models - Ancient Clay Tablets and Cuneiform The First Translation Tools in 3400 BC
The invention of cuneiform writing in Mesopotamia around 3400 BC marked a pivotal moment in human history, ushering in the era of recorded communication. This ancient script, primarily employed for languages like Sumerian and Akkadian, was meticulously inscribed onto clay tablets using a reed stylus to create wedge-shaped marks. The sheer volume of surviving clay tablets, exceeding 500,000, poses a considerable hurdle for researchers. Deciphering the intricate cuneiform symbols requires specialized knowledge, and as a result, only a handful of experts globally are capable of translating these ancient texts. However, recent breakthroughs in artificial intelligence have begun to reshape how we access this historical archive. AI-powered systems are now capable of automatically translating ancient Akkadian, written in cuneiform, into modern languages. The development of substantial online collections of translated cuneiform documents further underscores the transformative potential of AI in overcoming the limitations of human expertise and making this vast repository of knowledge accessible to a broader audience. This advancement has the potential to reveal new insights into ancient societies and civilizations, showcasing the power of technology to bridge the divide between the past and the present.
The discovery of thousands of clay tablets inscribed with cuneiform, dating back to 3400 BC, provides a fascinating glimpse into the earliest forms of written communication. These tablets, primarily used in Sumer and Akkad, represent a foundational step in the evolution of language, moving from pictorial representations to more abstract symbols. While the sheer volume of these tablets is impressive, only a small fraction have been translated due to the challenges of deciphering the complex wedge-shaped script. This scarcity of expertise highlights the inherent need for specialized knowledge in translation, a need that persists even today.
The difficulty in reading cuneiform is underscored by the fact that only a few hundred specialists globally possess the skills to decode it. This limitation underscores the crucial role these specialists played in their societies, acting as gatekeepers of information in much the same way that professional translators facilitate communication today. The fact that languages like Akkadian, found on many tablets, were spoken across vast regions of Mesopotamia, only further emphasizes the need for translation to connect different populations and communities. It's a reminder of how the challenges and requirements of cross-cultural communication have been a constant throughout history.
Interestingly, modern AI technology is now being used to overcome some of these limitations in deciphering the language of ancient Mesopotamia. While still in its early stages, AI-powered translation systems are beginning to offer a more efficient path to understand this ancient writing. The hope is that these emerging AI models will be able to process large volumes of cuneiform texts, possibly creating a substantial online corpus that could allow for more readily accessible translations of these invaluable historical documents. However, it remains to be seen whether AI can completely supplant the role of human experts, or whether their collaboration will prove to be the most effective approach in unlocking the secrets contained within these ancient texts. The creation of one of the largest online corpora of translated cuneiform texts with approximately 130,000 AI-translated documents, demonstrates that even the most complex translation problems can be gradually solved with new technological tools.
The development of AI to translate cuneiform, while still in its early phases, is a testament to our continuing drive to find new ways to access and understand humanity's past. This echoes the enduring importance of translating ancient languages and the ongoing need to find efficient methods to bridge historical and linguistic gaps. While we have advanced technological tools now, these tablets still reveal the fundamental need for translation, then as now, in our efforts to connect across both time and language.
The Evolution of Translation From Ancient Clay Tablets to Modern AI Language Models - Rosetta Stone Changes Translation Methods Forever in 196 BC
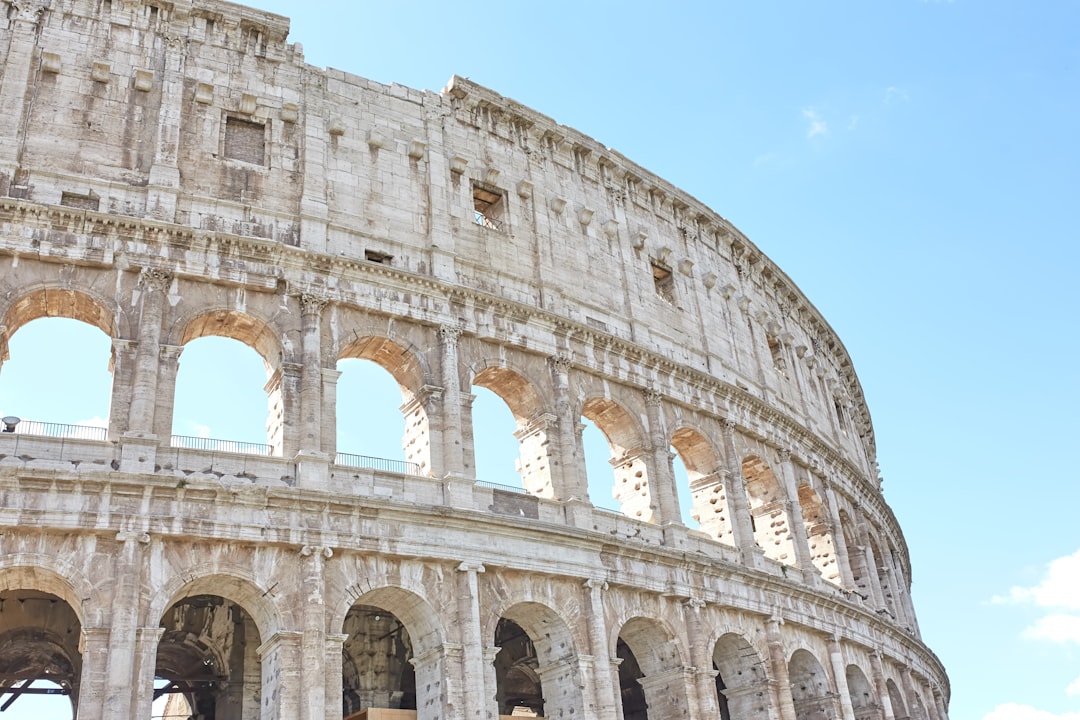
In 196 BC, the Rosetta Stone emerged as a game-changer in translation, featuring a single decree carved in three different writing systems: hieroglyphics, Demotic script, and Ancient Greek. This unique trilingual artifact was instrumental in the eventual decoding of Egyptian hieroglyphs, showcasing the crucial role that bilingual inscriptions played in linking distinct societies. The stone illustrated how translation could bridge communication gaps and facilitate understanding between civilizations. Its discovery and the successful deciphering of its inscriptions marked a pivotal turning point in the advancement of translation techniques. This echoes the ongoing pursuit of seamless communication that continues today with the advent of AI-driven translation tools. The Rosetta Stone stands as a powerful emblem of translation's transformative influence on historical understanding, reinforcing its value even as we witness a rapid evolution of language processing technologies. The stone's importance demonstrates how effective cross-language communication has always been vital for human understanding.
The Rosetta Stone, unearthed in 1799, stands as a pivotal moment in the history of translation, much like the initial discovery of clay tablets. Inscribed with the same decree in three scripts – hieroglyphs, Demotic, and Ancient Greek – it presented a unique opportunity to bridge a linguistic gap that had existed for centuries. The stone, discovered near Rosetta (modern-day El Rashid, Egypt), was essentially a multilingual document predating modern computer-aided translation by millennia. Its existence illustrates the ancient world's understanding of the need for cross-linguistic communication, much like how OCR software today handles different writing systems.
The Rosetta Stone's significance lies in its role in deciphering Egyptian hieroglyphs. Thomas Young and Jean-François Champollion, among others, used the parallel texts to deduce the meaning of the hieroglyphs, a task that required insightful pattern recognition and educated guesses. This process mirrors the challenges modern AI translation models face – relying on pattern recognition, probability, and statistical methods to bridge language gaps. While AI has undeniably progressed, the inherent limitations of automated translation remain, necessitating the critical eye of human translators to contextualize and refine machine outputs.
Interestingly, the Rosetta Stone, similar to the way modern AI training relies on parallel corpora, allowed researchers to compare and contrast the different versions of the same text. This process of cross-referencing greatly aided in understanding the meaning of the otherwise unknown hieroglyphs. However, the effort was not without its complexities. Researchers needed to make educated assumptions, a practice that underscores the crucial role of context and human intervention in translation. The ability to translate hieroglyphs fueled further investigations into comparative linguistics, much as the modern translation boom has led to a more in-depth study of language structures and similarities across different language families.
Moreover, the decipherment of the Rosetta Stone showcased the limitations of relying solely on isolated instances of words or symbols. The context within which the languages were used was equally important to understanding their true meaning. It's a lesson that still holds true today in the age of AI translation. Researchers working on AI translation constantly encounter ambiguity and necessitate the use of context to enhance accuracy.
Furthermore, the story of the Rosetta Stone is an early example of "crowdsourced" translation. Scholars from various backgrounds contributed to the deciphering effort, a concept akin to the collaborative translation efforts we see online today. The Rosetta Stone's discovery also invigorated the field of Egyptology and fueled a renewed interest in ancient languages, much like how AI translation tools are increasingly highlighting and raising awareness of endangered languages. The parallel underscores how technological advancements, be it a physical stone artifact or a complex algorithm, can contribute to the revitalization of language and cultural heritage.
While the stone itself has become a cultural symbol and artifact housed in the British Museum, its impact on the development of translation methods is undeniable. The Rosetta Stone’s story highlights how technological and cultural shifts can catalyze a deeper understanding of human languages and societies. Its influence underscores the enduring relevance of translation in facilitating communication across time and cultural divides – much as AI translation seeks to achieve in our current era. Much like the limitations and breakthroughs encountered in the past, we continue to grapple with the complex relationship between humans and technology, especially when it comes to translation. As AI continues to evolve, it is crucial to recognize the value of both human expertise and technological innovation in our quest to achieve greater accuracy, fluency, and accessibility in translation.
The Evolution of Translation From Ancient Clay Tablets to Modern AI Language Models - Machine Translation Debuts With IBM and Georgetown University in 1954
In 1954, a landmark experiment in machine translation took place at Georgetown University with IBM's support. This initial foray, now known as the Georgetown-IBM experiment, successfully translated over 60 Russian sentences into English using the IBM 701 computer. It was a watershed moment, showcasing the potential of computers to automate language processing and hinting at a future where language barriers could be overcome through technology.
However, the output of the experiment was rather crude by today's standards. The translations were often awkward and grammatically inaccurate, highlighting the challenges faced by early researchers in crafting truly effective translation algorithms. The system, relying on a limited vocabulary of 250 words and only six grammar rules, was a very basic prototype – a far cry from the nuanced understanding of language we expect today. Interestingly, the input was managed through punch cards by an operator who didn't even speak Russian, demonstrating how the technology, even then, sought to bypass the need for specialized linguistic knowledge. The experiment, despite its limitations, also needed a significant amount of computer memory, using the equivalent of 6,000 machine words, which at that time, was quite a large amount.
Early machine translation efforts primarily tackled specific areas like scientific and technical texts, where language tended to be more structured and predictable. This approach is vastly different from today's AI systems which strive to capture the complexity and variety of natural language including idioms and context. These early attempts were essentially rule-based, relying heavily on pre-defined linguistic rules, which quickly proved insufficient as the complexity of language became clear. This led researchers to explore alternative approaches like statistical methods and, eventually, neural networks which better account for the nuances of language.
Interestingly, the development of OCR (Optical Character Recognition) technologies emerged in tandem with machine translation, creating a synergy that allows printed text to be digitally scanned and translated. This fusion of technologies dramatically expanded the scope and accessibility of translated material across various domains, opening doors for wider access to printed works in different languages.
However, machine translation faced major obstacles in the decades that followed the Georgetown-IBM experiment, mainly due to the intrinsic difficulty of languages themselves and the limited computing power available. It was only with the advent of deep learning in the 2010s that substantial advancements emerged, leading to a substantial increase in the quality and speed of translations.
The modern AI translation models we see today often draw inspiration from historical methods. Similar to the Rosetta Stone, which allowed researchers to cross-reference different language versions of the same text, these models learn from vast corpora of bilingual text, identifying patterns and building probabilistic relationships between languages. This connection to the past demonstrates the enduring significance of context in successful translation.
Beyond consumer-facing applications, the appeal of automated translation extends to various industries and government sectors. The desire for rapid cross-language communication has accelerated the adoption of machine translation, playing a key role in globalization across diverse fields.
Despite the remarkable progress in the field, machine translation is still imperfect. Human translators remain crucial, especially in handling the intricate aspects of language such as cultural references, humor, and context-specific meanings that automated systems often struggle to interpret. These challenges highlight the inherent complexity of language and the subtle interplay of words, cultural context, and emotion.
The quest for truly universal translation – seamlessly bridging all languages – still presents significant obstacles. Language is not simply a collection of words, but a reflection of culture, history, and human experience. The limitations of machine translation, while continuously being improved, remind us of the essentially non-mathematical nature of human communication. The need for a nuanced human touch, combined with the power of advanced technology, remains essential in the quest for more accurate, fluent, and accessible translation solutions.
The Evolution of Translation From Ancient Clay Tablets to Modern AI Language Models - Optical Character Recognition Transforms Document Translation in 1974
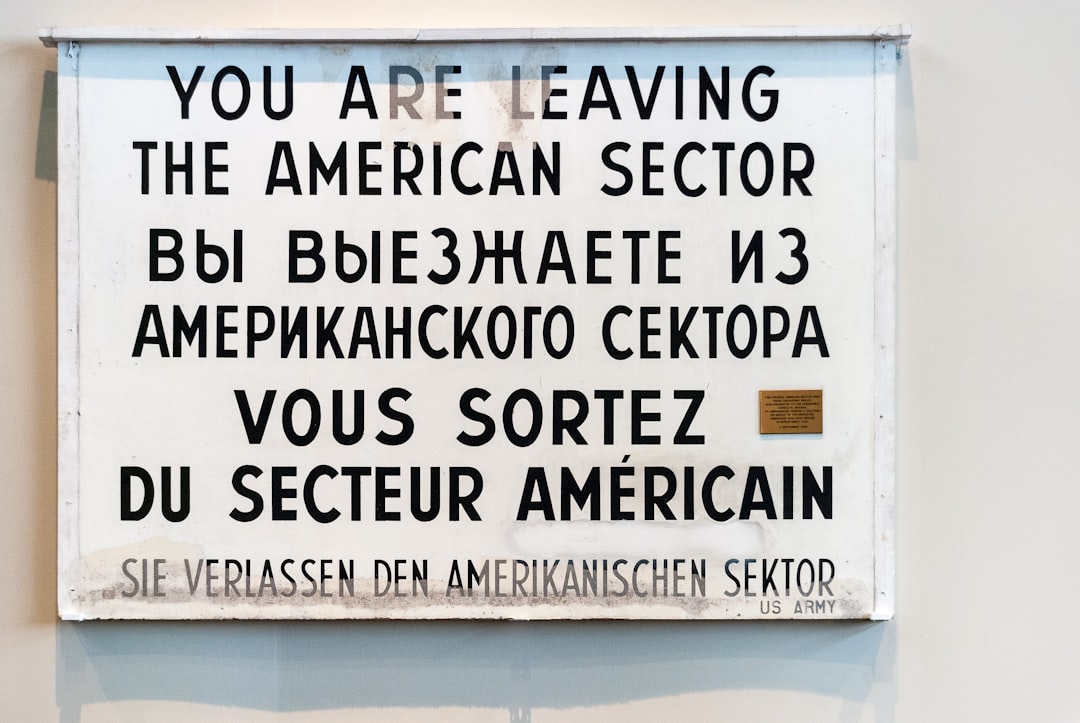
By 1974, the development of Optical Character Recognition (OCR) significantly altered how documents were handled for translation. This technology enabled the conversion of printed and handwritten text into a digital format, making documents easily editable and searchable. This step was a game-changer for translators, shifting from manual processes to a new era of efficiency and accessibility in handling various document types. The combination of OCR with nascent machine translation efforts streamlined the entire translation workflow, opening the door for quicker processing of documents ranging from legal texts to historical records. The subsequent improvements in OCR, particularly those leveraging deep learning methods like the TrOCR model, have made translation faster and more precise. This evolution of OCR showcases a clear progression from the basic translation tools of the past towards today's AI-powered translation approaches. However, despite these technological advancements, the complexities of human language persist, reinforcing the continuing need for skilled translators to accurately interpret the cultural and contextual nuances of the translated text.
Optical Character Recognition (OCR) experienced a notable leap forward in 1974, fundamentally altering how documents were handled for translation. Before OCR, translators had to manually input every character from a document, a time-consuming process. OCR automated this step, allowing printed text to be directly recognized and transformed into digital data, making translation much faster.
However, early OCR systems were surprisingly limited. They mostly worked well with standard fonts, but struggled with handwritten text, various font styles, and complex layouts. This restriction highlights the limitations of early pattern-recognition technology—a problem that wasn't effectively resolved until the development of more sophisticated machine learning approaches.
The combination of OCR with machine translation opened up a new world of opportunities for translating large amounts of printed material. Archives of historical documents became much easier and cheaper to translate, making these sources accessible in multiple languages. This broadened the impact of translation, bringing historical records and potentially valuable information to a much wider audience.
Interestingly, OCR didn't just speed up translation but also expanded the types of documents that could be translated. Charts, forms, manuals, and other kinds of printed materials, previously too challenging to translate efficiently, were now accessible. This made OCR and its related technologies valuable in a variety of fields like law, healthcare, and scientific research.
Despite the promise of OCR, it still suffered from certain weaknesses. The quality of the OCR input—such as a scan—greatly impacted the accuracy of the output. This meant that translating documents with poor scan quality often resulted in inaccurate translations, underscoring a persistent problem in translation accuracy even in modern systems.
OCR in 1974 was relatively crude compared to modern systems. The algorithms were basic, which led to errors when the input document was complex or contained noisy backgrounds. These inaccuracies caused many misinterpreted characters, emphasizing the need for continual improvements to translation technology to reduce errors.
One unexpected benefit of OCR is its role in preserving endangered languages. By digitizing and translating documents in languages that are at risk of extinction, OCR can help spread these languages into a broader sphere, potentially revitalizing interest in them. This highlights the wider societal impact translation tools can have.
The massive difference between OCR technology in 1974 and modern systems reveals a powerful transformation in digital technology. Today's AI-powered OCR systems have significantly advanced, capable of understanding hundreds of languages and dialects with considerably improved accuracy due to the application of deep learning techniques.
Surprisingly, OCR's integration into translation has led to a demand for multi-language documents. Globalization and the rise of international business have fueled the need for documents that can be easily understood in several languages, demonstrating how OCR and translation can work in tandem to support new communication needs.
OCR has laid the groundwork for a future of more advanced translation solutions. Real-time translation within devices and applications was enabled by the fundamental innovations in OCR. The transition from simply recognizing characters to more sophisticated, dynamic contextual translation shows that OCR’s core contributions to the evolution of translation technologies are considerable and far-reaching.
The Evolution of Translation From Ancient Clay Tablets to Modern AI Language Models - Neural Machine Translation Makes Online Translation Free in 2016
The year 2016 witnessed a significant shift in online translation with the arrival of Neural Machine Translation (NMT). This new approach, powered by sophisticated artificial neural networks, moved beyond earlier statistical methods, delivering faster and more accurate translations. NMT's ability to directly model the relationship between languages allowed for a more nuanced understanding of sentence structure and context. This breakthrough brought with it a wave of free online translation services, making it easier than ever to bridge language barriers and share information globally. While NMT has greatly improved translation accessibility, hurdles remain. The requirement for extensive training data and the ongoing challenge of fully capturing context highlight the complexities of human language, reminding us that the role of human translators in refining machine output remains important. This advancement in AI-driven translation is a notable step in the history of language processing, echoing past efforts to overcome communication barriers across cultures and eras.
Around 2016, a shift occurred in the field of machine translation with the widespread adoption of Neural Machine Translation (NMT). This new approach, powered by deep learning, allowed translation systems to analyze entire sentences rather than just individual words. This shift resulted in translations that were not only more accurate but also exhibited a greater understanding of context and the flow of language.
NMT systems are trained using massive collections of text in different languages, allowing them to learn the intricate patterns that exist within languages. Some of these NMT models have reached a level of quality that rivals human translators, challenging the traditional notion that machines are inherently limited in their ability to understand and produce human language. A significant aspect of NMT is the use of "attention mechanisms," which enable the model to focus on specific parts of a sentence that are most relevant to the translation. This is particularly helpful for languages with vastly different word orders, resulting in more accurate and natural translations.
One of the unexpected outcomes of NMT was its impact on the cost of translation. The automation offered by these systems meant that less human intervention was required, drastically reducing costs for businesses and organizations. Suddenly, high-quality translation was accessible at a fraction of what it previously cost. This development has had a substantial effect on the accessibility of information online, with more documents, from academic papers to business reports, readily available in numerous languages. This increased accessibility of translated materials has had a democratizing effect, breaking down language barriers that once hindered global communication.
Optical Character Recognition (OCR) became a powerful partner to NMT, enabling the translation of documents in various formats that were previously restricted to physical copies. Together, NMT and OCR could translate documents containing different kinds of writing and layouts, making previously unavailable historical records, technical manuals, and legal texts accessible to a much broader audience.
Despite the remarkable progress, NMT still faces hurdles. Dealing with idiomatic expressions, culturally specific references, and subtle emotional cues remains challenging for these systems. While grammatically sound, many translations may lack the nuanced tone and expression characteristic of a human translator. This points to a continuing need for human oversight and refinement in machine-produced translations.
Interestingly, while NMT has greatly increased the speed of translation, the models require substantial computational resources. These systems frequently rely on powerful graphic processing units (GPUs) to handle the complexity of the tasks. This highlights a tradeoff in technological progress: increased efficiency comes with the need for sophisticated infrastructure.
Furthermore, NMT systems are capable of learning from human interactions. The feedback loop created when users edit and improve the translations generated by the models allows for ongoing refinement of the systems. This ability to adapt based on real-world usage marks a significant advancement over traditional rule-based translation systems.
The wide availability of NMT has also raised ethical and legal questions. The ease with which these systems can reproduce text has prompted debates about intellectual property rights, especially when proprietary documents are involved. Concerns have been raised regarding the ownership of translated materials and the responsibilities of companies that leverage these technologies.
In conclusion, NMT has undeniably revolutionized the field of machine translation, bringing significant improvements in quality and accessibility. While challenges remain, the ongoing development and refinement of these systems continue to push the boundaries of what's possible in cross-language communication. The future of translation is likely to involve a dynamic interaction between human expertise and advanced artificial intelligence, offering a path toward ever-more accurate, efficient, and accessible translation solutions.
The Evolution of Translation From Ancient Clay Tablets to Modern AI Language Models - AI Language Models Learn to Translate 100 Languages Simultaneously in 2024
The year 2024 witnessed a remarkable leap in AI language models with the development of systems capable of translating between 100 languages simultaneously. This is a major shift from earlier AI translation systems that often relied on English as a bridge for translating between languages that didn't share a common language family. The ability to translate directly between multiple languages, without using English as an intermediate step, is a notable efficiency gain and potentially reduces translation errors.
However, despite these improvements, the intricacy and subtleties of human language still pose significant challenges for AI models. They can often struggle with idiomatic expressions, cultural nuances, and situations where context is crucial for accurate interpretation. As a result, human translators are still needed to ensure that the translated text accurately reflects the meaning intended by the original author and captures the subtle social and cultural dimensions embedded within the text.
The evolution of AI in translation certainly presents numerous benefits, but also raises important questions about how these tools can best be used alongside human experts. It highlights that technology can offer amazing improvements, but some human skills are still crucial to get accurate, truly meaningful translations.
AI-Powered PDF Translation now with improved handling of scanned contents, handwriting, charts, diagrams, tables and drawings. Fast, Cheap, and Accurate! (Get started for free)
More Posts from aitranslations.io: