AI-Powered PDF Translation now with improved handling of scanned contents, handwriting, charts, diagrams, tables and drawings. Fast, Cheap, and Accurate! (Get started for free)
How AI Translation Pattern Recognition Mirrors SETI's Search for Extraterrestrial Signals A Technical Analysis
How AI Translation Pattern Recognition Mirrors SETI's Search for Extraterrestrial Signals A Technical Analysis - Pattern Recognition in SETI Radio Signals Mirrors AI Translation of Ancient Scripts
The quest to understand both ancient languages and potential extraterrestrial communication hinges on the ability to identify intricate patterns within vast datasets. The application of AI in SETI, much like its use in deciphering ancient scripts, showcases this principle. AI's capacity to analyze radio signals in SETI parallels its role in interpreting historical texts, where recognizing subtle recurring structures unlocks hidden meaning. Similar to how AI can accelerate and improve the translation of ancient languages, it has drastically increased the speed and precision of identifying faint radio signals in space. These signals might be the subtle cues of advanced civilizations beyond Earth. This parallel highlights the potential of AI to revolutionize our understanding of both the human past and the broader universe. However, the complexities and ambiguities inherent in both fields present challenges. Advanced machine learning models are essential to effectively analyze massive amounts of data and identify relevant patterns amidst the noise.
The methods employed in SETI to identify patterns within radio signals share striking similarities with the techniques used in Optical Character Recognition (OCR) for translating scanned documents into digital text. Both domains heavily rely on sophisticated statistical models and machine learning algorithms to discern patterns, be it in the form of radio wave fluctuations or written characters.
Just as AI breakthroughs enabled the decryption of long-undeciphered ancient scripts like Linear B and Mayan glyphs by recognizing subtle variations in symbol patterns, SETI researchers leverage similar approaches in their search for extraterrestrial signals amidst a sea of cosmic noise. This ability to extract meaning from complex patterns, whether from ancient glyphs or radio wave frequencies, is a testament to the power of AI in uncovering hidden structure.
The algorithms developed for SETI data analysis can sift through massive quantities of data, often processing terabytes in mere hours. This remarkable capability significantly extends the boundaries of what was previously possible using conventional computational methods, mirroring the rapid advancements in AI translation, which can handle immense volumes of text.
Moreover, the process of filtering out naturally occurring astrophysical phenomena to isolate potential extraterrestrial signals in SETI bears resemblance to how AI translation tools differentiate between closely related languages and dialects. Both tasks demand precision in pattern recognition and the ability to recognize nuanced linguistic features essential for accurate interpretations.
Interestingly, the core machine learning techniques underpinning both AI translation and SETI often leverage neural networks. These networks are designed to emulate the human brain's pattern recognition capabilities, continually improving their accuracy over time through iterative training cycles. This shared reliance on neural networks highlights a fascinating overlap in the fundamental logic used across seemingly disparate fields.
This connection between SETI and AI translation isn't unidirectional. The signal detection algorithms developed for the search for extraterrestrial intelligence have been adapted and repurposed within language processing systems, resulting in more efficient and accurate translation across diverse languages. It's a fascinating example of cross-fertilization between distinct areas of research.
Further, the development of AI translation systems is increasingly integrating techniques traditionally used in SETI's anomaly detection. Through unsupervised learning, these systems attempt to reconstruct the meanings of phrases from entirely new languages, often working with remarkably limited data. It's a bold endeavor that aims to unlock the potential for understanding entirely novel communication systems.
Both SETI and AI translation encounter challenges associated with noise. For SETI, this means the pervasive cosmic background radiation. In AI translation, it manifests as colloquialisms, context-specific meanings, and idiomatic expressions that can obscure the true message within a text. These "noise" elements introduce obstacles that require sophisticated algorithms to overcome in order to extract meaningful information.
The search for intelligible patterns in seemingly random radio signals shares an intriguing connection with the linguistic concept of phonetic patterns. Just as human language relies on specific sound frequencies and rhythmic structures, it's conceivable that extraterrestrial communication might also utilize analogous principles. This shared dependence on a structured framework suggests the existence of a potential universal architecture for communication across different species.
Finally, similar to how SETI uses predictive models to infer the potential structure of extraterrestrial signals, AI-driven translation systems are adopting predictive approaches to hypothesize the structure of previously unknown languages. This suggests that we might be at a point where we can begin unlocking not only the mysteries of languages on Earth, but potentially even the secrets of communication from beyond. While speculative, it certainly hints at the possibilities.
How AI Translation Pattern Recognition Mirrors SETI's Search for Extraterrestrial Signals A Technical Analysis - Machine Learning Algorithms Bridge Gap Between Cosmic Noise and Language Detection
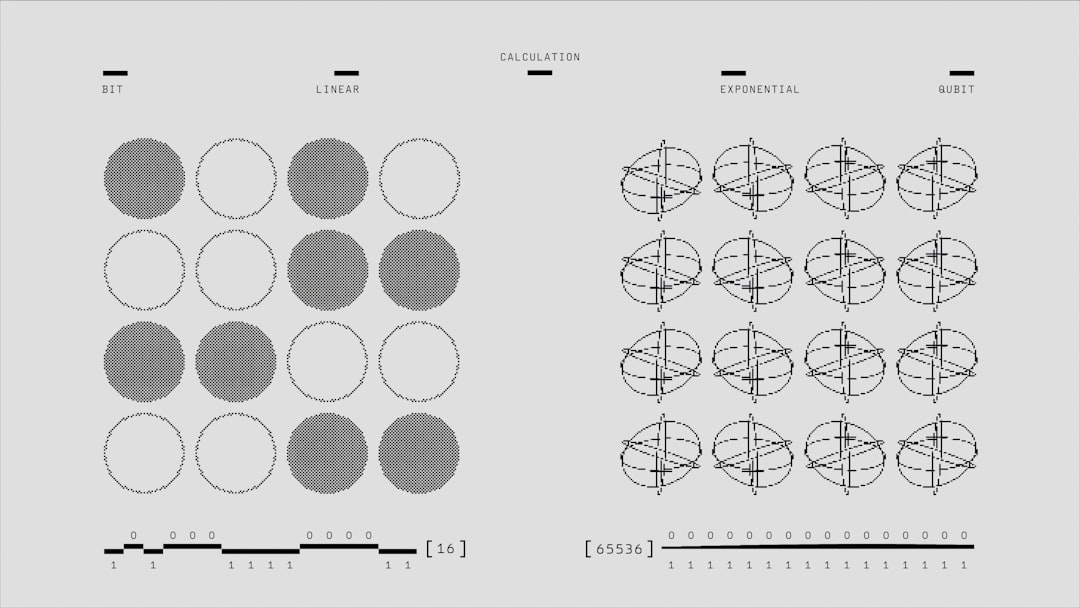
Machine learning algorithms are proving crucial in deciphering meaningful patterns from a sea of noise, whether it's the cosmic background radiation in SETI or the ambiguity inherent in natural language processing for AI translation. These algorithms, leveraging sophisticated pattern recognition, can sift through massive datasets to isolate meaningful signals from background noise. This capability is especially relevant in fields like AI translation, where diverse linguistic features, idiomatic expressions, and colloquialisms can cloud the intended meaning of a text.
Furthermore, the incorporation of noise embeddings from audio data into language model training highlights the challenges and opportunities associated with cross-modal learning. This integration aims to bridge the gap between different forms of data, such as sound and text, but also presents hurdles in ensuring optimal model performance. The successful application of machine learning in both SETI and AI translation necessitates handling these complexities to achieve a desired level of accuracy and clarity in extracting information. The continued advancement of these AI technologies will depend heavily on the development of innovative approaches for navigating the noise and complexities inherent in both fields.
Machine learning algorithms, particularly those leveraging neural networks, are proving instrumental in bridging the gap between cosmic noise and the potential detection of extraterrestrial signals. This parallels how these same algorithms are being used to improve AI translation, which is experiencing rapid advancements in speed and accuracy.
The sheer volume of data analyzed in both fields is staggering. SETI researchers process petabytes of data from radio telescopes daily, a scale comparable to handling enormous video archives. Similarly, AI translation systems are trained on vast corpora of bilingual text, highlighting the need for computationally efficient solutions. This shared challenge has led to the adaptation of algorithms initially developed for SETI signal detection, improving natural language processing capabilities. The ability to effectively filter out noise, be it cosmic radiation or colloquialisms in language, is essential for extracting meaningful information. Just as SETI models must learn to identify error patterns in radio signals, AI translation systems face the challenge of understanding idiomatic expressions that may not translate directly.
Interestingly, the training methods used in both fields share similarities. Neural networks used in SETI are trained on simulated datasets, much like translation systems learn from massive bilingual texts. This reliance on similar training techniques underscores the underlying relationship between the fields. Furthermore, the decoding process in both SETI and language translation relies on probabilistic frameworks to interpret signals or language structure. It’s intriguing to consider the possibility that a shared universal structure might underpin both extraterrestrial communication and human language. Research suggests a cognitive overlap in how humans process information for both understanding spoken/written language and potentially interpreting signals from outer space.
The flow of innovation isn't just one way; AI translation models are increasingly benefiting from SETI's signal noise reduction techniques, allowing for better differentiation between languages and subtle dialectal nuances. Moreover, the capabilities of real-time processing have revolutionized both fields. Near-instantaneous translations and signal detection are now possible, drastically changing the speed and accuracy of both communication and research. This rapid pace of advancements and cross-fertilization underscores the value of interdisciplinary approaches in tackling complex scientific and engineering challenges, particularly in pushing the boundaries of human communication, both on Earth and potentially beyond.
How AI Translation Pattern Recognition Mirrors SETI's Search for Extraterrestrial Signals A Technical Analysis - Neural Networks Process Radio Telescope Data Similar to OCR Translation Methods
Neural networks are increasingly vital for processing the massive amounts of data collected by radio telescopes, employing methods remarkably similar to how Optical Character Recognition (OCR) translates images into text. Both areas rely on deep learning to identify and categorize patterns embedded within noisy data. SETI's SonATA software, for example, processes radio signal data in real-time much like an OCR system translates images into digital text. Both approaches rely on sophisticated machine learning algorithms to make sense of complex information. As these deep learning models excel at separating relevant signals from noisy backgrounds, they highlight a powerful link between disciplines, aiding in our understanding of both space and language. This shared approach suggests that the principles of pattern recognition could have wider applications, perhaps leading to innovations through collaboration between seemingly unrelated fields like astronomy and linguistics.
The way neural networks handle radio telescope data bears a striking resemblance to how they process information in Optical Character Recognition (OCR) systems. Both rely on convolutional layers to pick out intricate patterns, whether it's the unique shapes of radio wave signals or the forms of handwritten characters. This ability to recognize hierarchical structures is key to both applications.
Interestingly, both fields often begin by preparing the data – normalizing it to make sure variations due to noise or distortion don't muddle the analysis. This standardization is vital for accurate pattern recognition.
Furthermore, processing vast quantities of data is a challenge shared by both AI translation and the search for extraterrestrial intelligence (SETI). The sheer volume of data, requiring similar levels of parallel processing, highlights a crucial element in both domains.
A common characteristic of training these systems is a feedback loop to improve their predictive ability. In SETI, algorithms learn from mistakes by adjusting parameters to filter out false positive signals, much like OCR systems learn from misread characters, refining their ability to decipher written text.
The efficiency of modern neural networks allows both SETI and AI translation to perform real-time analysis, whether that means monitoring radio signals from space or rapidly translating languages. This speed is essential to both fields for prompt responses and immediate insights.
One of the key aspects shared by these systems is feature extraction. This involves identifying crucial information within the raw data, like frequency components in radio signals or distinctive traits in letter forms. It's the key to boosting the recognition abilities of the systems in each domain.
Perhaps the most fascinating link between these areas is the potential to improve low-resource language translation using algorithms initially developed to find unique signals from space. These algorithms, geared towards isolating important patterns from noise, can be incredibly useful in translation as well.
The development of unsupervised learning techniques has been a significant advancement in both fields. In SETI, these methods can help identify novel signals, while in AI translation, they can reveal hidden relationships between seemingly unrelated languages, even with limited data.
Neural networks have a distinct advantage when it comes to handling the complex, non-linear patterns inherent in both radio waves and human languages. This flexibility makes them adaptable tools across both areas.
Lastly, the possible evolution of AI in SETI and AI translation runs in parallel. As both fields advance, we gain valuable insights into the way complex systems of communication – whether natural human languages or extraterrestrial signals – might emerge and be interpreted. These advancements highlight a shared goal in the study of communication systems, whether they exist on Earth or beyond.
How AI Translation Pattern Recognition Mirrors SETI's Search for Extraterrestrial Signals A Technical Analysis - Fast Batch Processing Techniques Link SETI Analysis with Modern Translation Systems
"Fast Batch Processing Techniques Link SETI Analysis with Modern Translation Systems" showcases how advanced computing techniques are revolutionizing both the search for extraterrestrial intelligence and the field of AI translation. Just as SETI researchers rely on rapid data processing to sift through massive astronomical datasets, AI translation systems now utilize batch processing to quickly and accurately translate large volumes of text. This common thread emphasizes the growing need for efficient, real-time analytics across diverse fields.
The ability to rapidly extract meaningful patterns from massive amounts of data – whether it's radio signals from space or the complexities of human language – is crucial for both disciplines. However, significant challenges remain. The "noise" in these systems, whether it's cosmic radiation or the subtleties and ambiguities of human languages, necessitates the development of increasingly sophisticated algorithms.
The interesting connection between these seemingly disparate fields highlights the potential benefits of cross-disciplinary collaboration. Understanding the parallels between how SETI and AI translation systems process data might offer valuable insights for further development in both areas. This includes improving how we search for potential alien signals and pushing the boundaries of efficient, accurate language translation.
The sheer volume of data handled by both SETI and AI translation systems presents a compelling parallel. SETI researchers process vast amounts of radio telescope data—on the order of tens of terabytes daily—while AI translation systems are trained on massive corpora of bilingual text. This shared challenge of data scale highlights the need for computationally efficient methods in both fields.
Furthermore, the ability to perform real-time analysis is a critical advancement in both SETI and AI translation. Whether monitoring radio signals or instantly translating languages, near-instantaneous processing drastically enhances the speed and quality of research and communication. This focus on speed underlines a crucial element in pushing the boundaries of both fields.
Interestingly, techniques like convolutional neural networks (CNNs) are leveraged across SETI and the realm of OCR for text translation. This shows how deep learning methodologies can be adapted and used in remarkably different domains. Similarly, methods developed to filter out cosmic noise in SETI analysis find counterparts in AI translation, where algorithms address colloquialisms and contextual ambiguities. Essentially, both domains benefit from refined noise reduction to improve the accuracy and trustworthiness of the information being analyzed.
Both fields employ feedback learning approaches to improve their performance. In SETI, this involves refining algorithms to eliminate false positives, while in AI translation, feedback mechanisms adjust the system to correct errors in translation, demonstrating a common approach to iterative learning and improvement.
Extracting crucial features from raw data is a critical step in both domains. Whether it's discerning specific frequencies within radio waves or recognizing the distinctive shapes of written characters, feature extraction is a key ingredient for enhancing pattern recognition.
It’s fascinating to note that some algorithms initially created for SETI have been repurposed in language processing, leading to enhancements in translation speed and precision. This interdisciplinary transfer highlights how the basic principles of signal analysis can be utilized in diverse ways.
One of the areas where this shared methodology shows potential is low-resource language translation. The algorithms used in SETI to find unusual signals are now being considered in efforts to enhance the translation of less-commonly studied languages. This cross-fertilization illustrates the power of looking at seemingly disparate areas for innovative solutions.
Additionally, unsupervised learning, which plays a role in SETI's search for novel signals, also offers benefits for AI translation systems. It helps reveal unexpected linguistic connections, even in cases where data is limited. These overlaps continue to strengthen the link between the two areas.
Research suggests that the underlying cognitive processes behind both language comprehension and signal interpretation might share intriguing similarities. This discovery raises questions about the nature of communication across different forms of intelligence, human or otherwise. The parallels between the two disciplines continue to create fertile ground for exploration.
These connections illustrate that cross-disciplinary work can help advance both fields in unexpected and beneficial ways. This is especially true as we tackle complex challenges in communication, both on Earth and potentially beyond.
How AI Translation Pattern Recognition Mirrors SETI's Search for Extraterrestrial Signals A Technical Analysis - Breakthrough Listen Project Shows Parallel Development with Machine Translation
The Breakthrough Listen project exemplifies a major leap forward in the search for extraterrestrial intelligence, primarily through its reliance on machine learning for analyzing enormous datasets. This project, dedicated to exploring almost a million stars for signs of technology (technosignatures), leverages advanced algorithms to minimize noise interference and improve signal detection. This approach closely mirrors how AI tackles translation – using sophisticated techniques to identify patterns within language. Breakthrough Listen's use of deep learning showcases how these computational methods are not just useful for cosmic data interpretation but also have a shared foundation with the pattern recognition essential for translation and understanding languages. The ongoing progress in both fields, coupled with the convergence of machine learning applications, creates promising opportunities for discoveries that might fundamentally reshape our grasp of communication – both within our world and potentially from beyond.
The Breakthrough Listen project, a leading effort in the search for extraterrestrial intelligence (SETI), exhibits intriguing parallels with the development of machine translation systems. This isn't just a coincidence; rather, it hints at shared underlying principles in how we approach the challenges of deciphering complex patterns from noise-filled data.
Breakthrough Listen leverages massive radio telescopes like the Green Bank Telescope and Parkes Telescope to observe millions of stars, seeking technosignatures—signs of extraterrestrial technology. The sheer scale of data processed daily—petabytes of information—mirrors the massive datasets used in modern AI translation systems. Both projects rely on incredibly fast processing speeds to keep up with the influx of data. They need to analyze this information in real-time, whether it's identifying potential alien signals or delivering instant translations of text.
Interestingly, noise reduction techniques are essential to both. Just as SETI researchers filter out noise from cosmic sources like pulsars and quasars, AI translation tools need to decipher the complexities of natural languages, including colloquialisms and idioms, to accurately convey meaning. Both use similar algorithms to identify and isolate the important patterns amidst noise.
Training methods also show surprising similarities. Neural networks utilized in SETI often employ feedback loops to refine their ability to identify potential alien signals. These methods are similar to how machine translation systems are trained, refining their accuracy over time through iterative learning. In fact, some algorithms originally developed for SETI have found new life within AI translation systems, especially in the area of low-resource language translation. This shows the potential for cross-disciplinary knowledge transfer.
Furthermore, the emphasis on feature extraction is a core concept in both fields. Whether it's identifying distinct radio frequencies in SETI or recognizing the shapes of letters in OCR, feature extraction helps to highlight the most critical pieces of information in the raw data. This makes pattern recognition more robust and accurate.
There are hints that the cognitive processes involved in understanding language and interpreting signals from the cosmos might share some underlying structure. This is a fascinating area of speculation, with potential implications for understanding communication beyond Earth. The complexities faced in deciphering the nuances of idiomatic language parallel those involved in interpreting subtle variations in radio frequencies.
Batch processing techniques, crucial for both high-speed signal analysis in SETI and rapid translation in AI, emphasize the importance of efficient data handling. These methods help optimize the processing of massive datasets.
The ultimate goal of both SETI and AI translation systems – understanding communication—leads us to ponder the possibility of universal structures for communication. Perhaps there are fundamental principles that govern how intelligent beings, whether from Earth or beyond, communicate. By continuing to explore these connections, we can unlock new insights into the nature of intelligence itself.
The surprising similarities between the Breakthrough Listen project and machine translation highlight the potential for cross-fertilization between these areas. The innovations in one area could potentially spark progress in the other, allowing us to refine our ability to both understand human language and potentially detect signs of extraterrestrial life. While still in its early stages, this exploration of the connections between SETI and AI translation offers exciting prospects for both future scientific discovery and a deeper understanding of communication itself.
How AI Translation Pattern Recognition Mirrors SETI's Search for Extraterrestrial Signals A Technical Analysis - Signal Analysis Methods from 2024 SETI Projects Apply to Language Processing Tasks
The recent advancements in SETI projects, particularly those leveraging signal analysis methods in 2024, reveal fascinating parallels with language processing tasks. For instance, the SETI Ellipsoid Technique, which strategically leverages spatial data to pinpoint promising targets for alien signal detection, has interesting implications for how we might approach pattern recognition in languages. The way AI is being used to filter out vast amounts of cosmic noise to find potential extraterrestrial signals echoes how AI is now able to refine its analysis of complex linguistic patterns, especially in AI-driven translation. Machine learning and deep learning approaches, crucial in handling the enormous datasets associated with SETI and AI translation, point to shared obstacles and innovative solutions in both fields. The ability to decipher complex patterns in radio signals is now mirrored in the ability of AI to decipher the complex nuances of human languages, demonstrating a potential connection between interpreting cosmic signals and interpreting the subtleties of language. These developments show the growing relevance of signal analysis, not just for the search for extraterrestrial intelligence, but also for enhancing our understanding of human communication and its diverse forms. While the fields of SETI and AI translation might seem disparate, these new approaches show a potential for cross-pollination that may lead to insights and innovations in both. It remains to be seen if these similarities represent a fundamental relationship between how intelligence, across different species and domains, interprets and processes information, or if it is merely a coincidental convergence of methods. Nonetheless, it's a promising avenue for exploration.
1. The Breakthrough Listen project, a major effort in the search for extraterrestrial intelligence, has shown how machine learning can analyze radio signals at incredible speeds, potentially uncovering signs of technology from millions of stars in real-time. This is similar to how AI quickly processes huge amounts of text for translation purposes.
2. The way SETI projects filter out the constant noise of space to find important signals highlights a challenge that's also crucial in AI translation. In both areas, being able to identify meaningful patterns amidst a ton of data is key—whether it's understanding the context of words or distinguishing real signals from space noise.
3. Both SETI and AI translation are built on algorithms that improve over time. In SETI, the algorithms learn from incorrect interpretations of space signals, while in AI translation, they refine their translations based on feedback from users and corrections. It's a fascinating example of how both domains leverage adaptive systems.
4. Multi-layered neural networks have become a crucial part of both SETI and AI translation. In SETI, they're used to understand the complex patterns found in radio signals, while in AI translation, they help discern sentence and meaning structures within text. It's intriguing how these same methods are found in such different areas.
5. It's interesting how the techniques for reducing noise in SETI are now being used to improve how AI handles language. For example, they're helping AI systems tell the difference between closely related languages and dialects, which makes translations much more effective. This transfer of knowledge is a positive sign for innovation.
6. One of the common challenges in both SETI and AI translation is dealing with noise. In SETI, it's the background noise of the cosmos. In AI translation, it's the complex and sometimes ambiguous nature of human language – slang, idioms, and the like can make interpreting text difficult. That's why both fields have needed to develop sophisticated techniques to remove noise or reduce its impact.
7. AI translation has taken advantage of some of the algorithms originally developed for SETI, particularly when it comes to dealing with lesser-known languages. This shows how ideas developed in one area can help others, even if they seem quite different at first.
8. The computational power needed for SETI and AI translation are comparable. Both deal with massive amounts of data—SETI from radio telescopes, AI translation from huge sets of bilingual text. This need for parallel processing reflects how both are pushing the limits of computing.
9. The similarities in the cognitive processes used to understand language and process signals are intriguing. It leads to questions about how communication might work across different types of intelligence—whether it's human or extraterrestrial.
10. The constant drive to improve batch processing techniques in both SETI and AI translation shows a shared desire for immediate insights. Both fields want to get information as quickly as possible. That need for speed is a testament to how these areas are constantly seeking improvements to better understand both language and the universe around us.
AI-Powered PDF Translation now with improved handling of scanned contents, handwriting, charts, diagrams, tables and drawings. Fast, Cheap, and Accurate! (Get started for free)
More Posts from aitranslations.io: