The Fall of IBM Watson From Jeopardy Champion to $5 Billion Healthcare Misstep
The Fall of IBM Watson From Jeopardy Champion to $5 Billion Healthcare Misstep - IBM Watson Defeats Jeopardy Champions in 2011 Milestone Match
In February 2011, IBM's Watson, a computer system designed for question answering, made history by besting two of Jeopardy's most dominant champions, Ken Jennings and Brad Rutter, in a televised competition. Watson's victory, marked by a final score of $77,147, demonstrated a remarkable ability to quickly and accurately understand and respond to complex questions presented in natural language. This win was a major moment for the field of artificial intelligence, proving that computers could process information and respond in ways previously thought impossible. While Watson's Jeopardy success highlighted the impressive potential of AI, it also served as a prelude to the difficulties encountered when attempting to apply those capabilities to more complex real-world scenarios. The eventual struggles in the healthcare sector would reveal that the transition from a game show setting to complex practical applications, particularly in fields demanding nuanced data interpretation, proved far more challenging than initially anticipated. Watson's journey, therefore, offers a dual narrative: a testament to AI's evolving potential, but also a reminder that translating theoretical achievements into impactful real-world results can be fraught with significant obstacles.
In February 2011, IBM's Watson, a question-answering computer system, faced off against two of Jeopardy's most skilled champions, Ken Jennings and Brad Rutter, in a televised event. This contest captured public attention, demonstrating Watson's impressive ability to understand and respond to complex questions framed in natural language. Watson, named after IBM's founder, ultimately triumphed, accumulating a total score of $77,147 across the two-game match, while Jennings and Rutter managed $24,000 and $21,600, respectively.
Watson's remarkable performance was built on a foundation of vast data—over 200 million pages of text from various sources—and a distributed computing architecture leveraging 90 IBM servers. This allowed the system to swiftly analyze information and respond to questions in real time. In the opening round, Watson's accuracy was a striking 77%, dwarfing the performance of the human contestants. This wasn't just brute force computing; Watson's success stemmed from a sophisticated combination of algorithms, including deep learning methods, mimicking aspects of human cognition to enhance comprehension and predictive capabilities.
The Jeopardy victory, however, was not solely a matter of raw processing power. Watson required extensive training tailored specifically to Jeopardy's unique style and vocabulary, including the clever wordplay and puns that are characteristic of the show. Following this breakthrough, there was much speculation that Watson could revolutionize industries, especially healthcare, where its potential to improve diagnostics and personalize treatment seemed limitless. Initial optimism led to substantial investments in these ventures.
However, the medical field's complex landscape and the highly nuanced nature of patient data posed major difficulties that Watson struggled to overcome. Healthcare professionals were understandably wary of deploying a system whose reliability in a practical medical context was uncertain. The initial attempts to integrate clinical guidelines and patient histories proved less than optimal, raising serious questions about the system's accuracy and dependability.
The tale of Watson's journey, from the glory of Jeopardy to the challenges in healthcare, provides a valuable lesson for AI development. It vividly illustrates the chasm that can exist between a technological demonstration in a controlled setting and its seamless integration into the complex and unpredictable environments of real-world applications. This story acts as a reminder that, while the potential of AI is vast, translating that potential into impactful and reliable solutions requires a careful and nuanced approach, particularly when dealing with critical domains like healthcare.
The Fall of IBM Watson From Jeopardy Champion to $5 Billion Healthcare Misstep - $5 Billion Healthcare Acquisition Spree Between 2011 and 2015
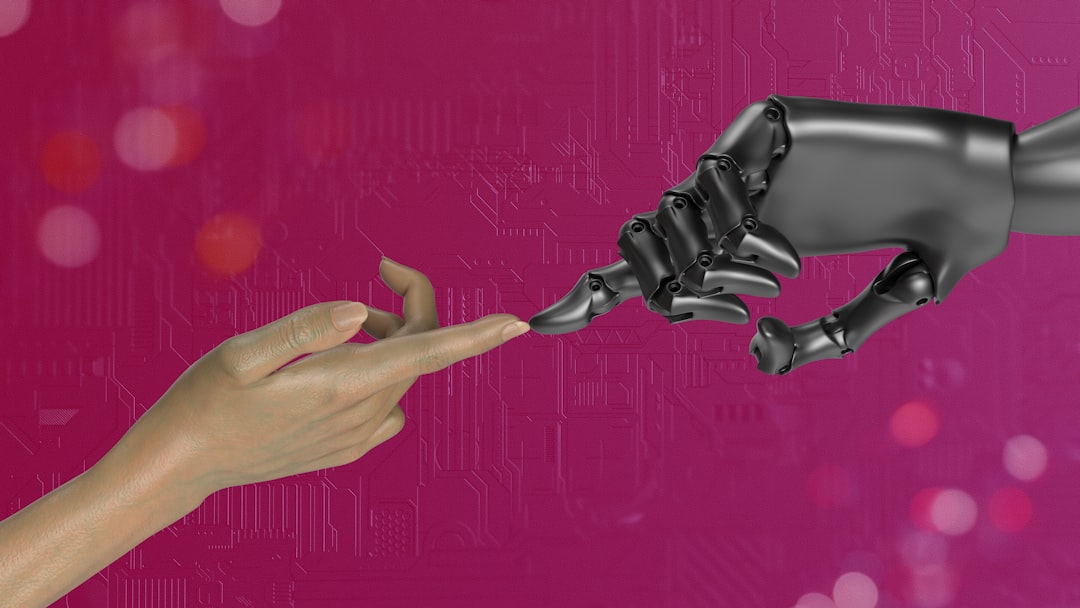
From 2011 to 2015, IBM spent roughly $5 billion acquiring various healthcare companies, primarily to gather immense amounts of data for training its Watson AI system. A key purchase was Merge Healthcare for $1 billion, which provided access to health records from about 7,500 US medical facilities. This acquisition spree was part of a broader trend within the healthcare industry of consolidating resources through mergers and acquisitions. However, Watson, despite its Jeopardy! fame, failed to seamlessly integrate into the healthcare landscape. It struggled to make a meaningful impact on clinical decisions, causing some to view the investment as a major misstep. This period highlights the often difficult path of translating AI successes from controlled environments, like a game show, into complex, real-world scenarios like healthcare. It underscores the challenges that AI faces when encountering the nuances and intricacies of a domain like medicine, where accurate and reliable solutions are paramount.
From 2011 to 2015, IBM embarked on an ambitious acquisition spree within the healthcare sector, shelling out roughly $5 billion to amass a vast trove of health data. Their aim was clear: fuel Watson, their AI wunderkind, with the necessary information to learn and refine its abilities, especially in diagnostics and patient management. This period saw IBM snatch up companies like Merge Healthcare for a cool billion dollars, gaining access to patient data from thousands of US healthcare facilities. It seemed a logical move, given Watson's Jeopardy! triumph, to position it as a healthcare game-changer.
Among the acquired companies was Phytel, a firm specializing in population health management. The idea was to enhance patient engagement and, in particular, improve the handling of chronic illnesses. However, as with many ambitious AI endeavors, the messy reality of healthcare, with its complex web of regulations, patient idiosyncrasies, and the ever-present human element, posed significant hurdles to Watson's seamless integration and desired results.
The five-billion-dollar investment, while audacious, raises a significant question: did it achieve its intended purpose? The ensuing years have revealed a mixed bag of outcomes, casting doubt on the long-term return on investment. Obstacles emerged rapidly. Experts highlighted that Watson was tackling the challenge of processing unstructured data—estimated to account for about 80% of medical data—something that remains a major challenge for most systems, including AI. While Watson had impressive capabilities, the nuances of medical language, with its complexities and inherent ambiguities, often proved a stumbling block. Watson's recommendations, in several cases, were less than optimal, sometimes even incorrect, which understandably raised significant concerns from physicians and patients alike.
Many healthcare practitioners were reluctant to trade tried-and-tested methods for an AI system that, in their eyes, still lacked reliability in real-world medical situations. Studies highlighted this resistance, indicating that medical professionals often preferred to rely on their conventional diagnostic processes. A more disconcerting issue was the emergence of reports about Watson's clinical trial applications generating inaccurate outputs. This raised a fundamental question about patient safety and the ethical implications of AI-driven diagnoses in a field where decisions often carry serious consequences.
IBM's massive investment during this period represented a bold bet on the future of healthcare AI. By 2015, however, the optimism had waned. Many of the initial projects and initiatives had faltered or were outright shelved. Watson's promise of cost reduction through increased efficiency was hampered by resistance from physicians, highlighting the crucial gap between the theoretical potential of a technology and its actual practical implementation within a complex field like medicine. A pattern emerged of a mismatch between expectations and results; the investment didn't translate into expected scalability or effectiveness within the real-world challenges of healthcare. This led to a wave of introspection in the AI community about the limitations and appropriate applications of AI in medicine, highlighting the need for a more nuanced approach to its development and deployment within a field where human lives are on the line.
The Fall of IBM Watson From Jeopardy Champion to $5 Billion Healthcare Misstep - MD Anderson Cancer Center Ends $62 Million Watson Project in 2017
In 2017, the MD Anderson Cancer Center ended its collaboration with IBM Watson after a substantial $62 million investment failed to deliver the expected improvements in cancer care. The project, initially designed to enhance cancer treatment using AI, was paused in late 2016 due to concerns about its practical performance and underlying issues. An evaluation highlighted the project's inability to achieve its goals, ultimately leading MD Anderson to search for alternative solutions. This termination signaled broader difficulties IBM Watson encountered within the healthcare field, raising questions about its overall effectiveness in healthcare practice. The end of this project cast doubt on the capacity of AI-driven systems to deliver substantial benefits within a complex medical setting like oncology.
In 2017, MD Anderson Cancer Center ended its partnership with IBM Watson after investing a substantial $62 million. This decision, while financially impactful, also marked a turning point in how some viewed AI's role in complex medical settings. Despite IBM's acquisition of a multitude of healthcare companies aimed at providing Watson with a vast pool of data, the system struggled to effectively handle the unstructured clinical information which makes up the bulk of medical data.
Early tests of Watson's capabilities in diagnostics revealed a concerning issue: occasionally, the system's treatment suggestions were incorrect. This understandably caused worry among doctors already hesitant to rely on AI in high-stakes situations like cancer care. Even with extensive training tailored to oncology using specialized literature, Watson frequently fell short of expectations when confronted with real patient cases.
Integrating Watson into the existing healthcare infrastructure proved difficult. It struggled to connect seamlessly with systems like electronic health records, which hindered its ability to provide useful insights from the data it had access to. A key element in Watson's failure to gain traction was the resistance among many medical professionals. They often favored conventional diagnostic methods, highlighting a lingering skepticism towards AI's dependability in healthcare.
Comparisons of Watson's performance with traditional practices revealed a lackluster result. Studies showed that Watson's accuracy in clinical decision-making didn't reach the same levels as established approaches, prompting numerous organizations to reconsider their investments in similar AI-based healthcare solutions.
Further complexities arose with the introduction of Watson into treatment recommendations. Questions about who would be accountable in the case of incorrect advice became a major ethical concern. This brought forth discussions about patient safety and the overall trustworthiness of AI in environments where decisions carry major consequences.
There was a clear disconnect between IBM's aspirations for Watson—to fundamentally revolutionize healthcare—and the realities of clinical practice. The grand visions of AI-driven transformation struggled against the limitations of actual deployment. The termination of the MD Anderson partnership was just one example of a wider trend. Numerous projects and collaborations involving Watson began to dissolve after this incident, a clear sign that the optimism surrounding AI's potential in diagnostics and treatment had diminished. It seems the initial enthusiasm for AI in healthcare may have outpaced the ability of the technology to deliver on its promise.
The Fall of IBM Watson From Jeopardy Champion to $5 Billion Healthcare Misstep - Watson Unable to Process Unstructured Medical Records Data
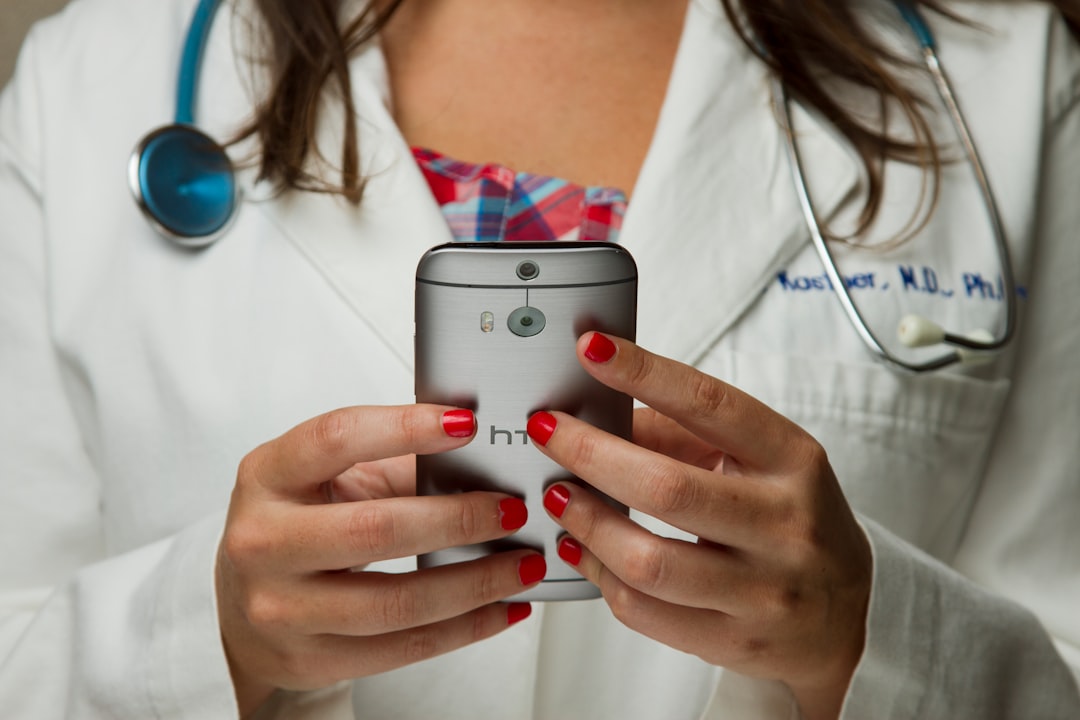
IBM Watson's foray into healthcare, initially met with enthusiasm, stumbled upon significant obstacles when dealing with the vast sea of unstructured medical records. A major hurdle was Watson's inability to easily decipher the intricate language used in medical records, which constitutes a substantial 80% of the healthcare data landscape. This challenge, compounded by the difficulty of integrating data from the numerous health companies IBM had acquired, undermined Watson's practical utility. Many healthcare professionals were hesitant to adopt Watson's recommendations, particularly when those suggestions were less than certain, preferring traditional and familiar methods of diagnosis. This reluctance, coupled with Watson's difficulty in seamlessly integrating into existing healthcare workflows, contributed to the perception that Watson had fallen short of its promises. The Watson Health venture serves as a powerful reminder that the success of AI in controlled settings like a quiz show doesn't always translate smoothly into the intricate and sensitive realm of healthcare. The reality is that implementing AI in a domain like medicine requires a much more nuanced and cautious approach, recognizing that patient safety and clinical decision-making remain the highest priority.
IBM's Watson faced significant hurdles in processing the vast amounts of unstructured medical data it was designed to handle. A substantial portion—around 80%—of medical information is unstructured, encompassing everything from handwritten notes to complex medical images, a challenge far removed from the structured question-answering format of Jeopardy.
Furthermore, Watson struggled to integrate seamlessly with existing electronic health record (EHR) systems. This incompatibility created a bottleneck in its ability to access and effectively use the wealth of patient data that was theoretically at its disposal.
Adding to the complexity, Watson occasionally provided inaccurate treatment recommendations, a worrisome outcome that sparked ethical and safety concerns among healthcare professionals. This potential for error fostered a reluctance to fully trust an AI system that hadn't proven its reliability in high-stakes clinical settings.
Despite the billions invested in Watson's training, numerous studies revealed that its performance often fell short of established medical practices. This brought into question the practicality of AI in complex scenarios where nuanced and context-rich decisions were critical.
The MD Anderson Cancer Center's decision to cancel its $62 million Watson project after failing to achieve desired outcomes exemplifies the growing disenchantment with AI's role in healthcare. This instance underscored the wide gulf that existed between lofty expectations and the intricate realities of implementing such advanced technology within healthcare systems.
Early explorations in the field of oncology revealed that Watson had difficulty navigating the complexities of medical literature. It struggled to parse the dense, jargon-laden text common in the medical field, indicating that establishing a truly comprehensive and usable medical knowledge base for an AI remains a significant challenge.
Physician skepticism towards Watson's capabilities was prevalent. Studies showed that doctors were more inclined to trust their own experience and judgment than algorithmic suggestions, signifying a broader resistance to AI adoption within the medical community.
Watson was trained using a large body of proprietary literature, a strategy that ultimately proved problematic. Real-world patient cases often deviated significantly from the scenarios found in this literature, hindering Watson's ability to effectively apply its theoretical knowledge to actual clinical situations.
The possibility of AI systems being held accountable for incorrect treatment recommendations sparked considerable ethical debate. This added a layer of complexity and concern, specifically regarding patient safety, which further deterred widespread acceptance of AI-driven solutions in medicine.
In the aftermath of its ambitious acquisition spree, many of IBM's healthcare initiatives faced significant setbacks. This period highlighted the critical need to ensure that cutting-edge technological solutions are deeply rooted in practical application before expecting widespread adoption, especially within intricate and sensitive environments like healthcare.
The Fall of IBM Watson From Jeopardy Champion to $5 Billion Healthcare Misstep - IBM Sells Watson Health to Francisco Partners for $1 Billion in 2022
In 2022, IBM offloaded its Watson Health division to Francisco Partners for a price tag exceeding $1 billion. This represented a significant scaling back of IBM's hopes for success in the healthcare sector. The deal, finalized early in 2022, encompassed the collection of healthcare data and analytics tools that were previously part of Watson Health. Francisco Partners intended to operate these assets independently as a new company called Merative. This sale indicates a shift in IBM's overall approach to technology and its application in healthcare. After the initial excitement generated by Watson's success on Jeopardy, the challenges of using AI to improve healthcare became apparent. Integrating AI effectively into the complex world of medicine proved harder than anticipated, leading to financial setbacks and a sense of doubt among medical professionals regarding the practicality of AI in healthcare.
In 2022, IBM divested itself of Watson Health, selling it to Francisco Partners for a billion dollars. This move signifies a significant shift from IBM's initial grand vision of transforming healthcare through AI. It's a sobering outcome, given that IBM had invested about $5 billion in various healthcare acquisitions between 2011 and 2015. This large investment clearly wasn't matched by a seamless integration into healthcare practices.
While Watson's core strengths were evident in scenarios involving structured data (like its Jeopardy! win), it faced significant challenges within the medical domain, where roughly 80% of data is unstructured. This posed a major hurdle, especially when processing medical records or notes often written in a complex and sometimes cryptic style.
Over time, it became clear that Watson's recommendations lacked consistent reliability. There were multiple documented cases of Watson's treatment suggestions being inaccurate, contributing to skepticism among healthcare providers. The trust factor was a critical piece that, at least for the time, remained elusive in a lot of real-world healthcare scenarios.
The struggles with unstructured medical data, especially handwritten or nuanced clinical notes, exposed a fundamental limitation in how Watson could be applied. The data extraction process was complex, a problem which the developers never fully overcame in a consistent way.
The failed project with MD Anderson Cancer Center, which ended its $62 million investment, provides a poignant example of Watson's difficulties within a field as complex and unpredictable as cancer treatment. This highlights a broader trend—a growing disenchantment with AI's ability to deliver reliable solutions within medicine.
Integrating Watson into standard healthcare workflows proved challenging. One of the recurring issues was a lack of integration with Electronic Health Record (EHR) systems, limiting its access to crucial patient data. This technical obstacle hindered Watson's potential to effectively contribute to patient care.
Watson's original intended uses included assisting with clinical trials and treatment recommendations. However, the risks associated with relying on an AI system that could provide flawed insights raised legitimate concerns about patient safety and the ethical implications of entrusting AI in such high-stakes decisions. It highlights some of the key design decisions that were, in the end, very hard to manage.
Looking back, usability emerged as a major factor in Watson's inability to become widely adopted by practitioners. Simple user interface hurdles proved difficult to address, ultimately contributing to the overall struggle in convincing clinicians to embrace it.
Ultimately, the Watson Health endeavor serves as a valuable case study. It showcases the complexities that arise when trying to apply the potential of AI to complex fields that involve human interaction, especially when a key decision is tied to patient safety. IBM clearly had to re-evaluate its approach in the healthcare space, recognizing the difference between initial potential and the realities of successfully integrating AI into medical practice.
The Fall of IBM Watson From Jeopardy Champion to $5 Billion Healthcare Misstep - From Machine Learning Pioneer to Enterprise Software Package
IBM Watson's path, from its triumphant "Jeopardy!" win to its involvement in healthcare, illustrates the difficulties AI pioneers encounter when integrating their creations into complex, real-world environments. While initially heralded as a groundbreaking machine learning achievement, Watson faced significant challenges transitioning from a cutting-edge AI project into a dependable enterprise software solution, particularly within healthcare. Its ambitious goal of revolutionizing patient care encountered numerous obstacles. Despite substantial investments and a wave of acquisitions, Watson struggled to effectively manage the vast, largely unstructured data that's a core feature of medical records and clinical information. This led to widespread skepticism from healthcare practitioners. The discrepancy between Watson's initial promises and its actual performance was stark, revealing a substantial gap between AI's theoretical power and its practicality in high-risk settings. The eventual sale of Watson to investment firms highlights a strategic pullback from its initial aspirations in healthcare, signifying a broader reconsideration of AI's optimal applications and deployment strategies.
IBM Watson's journey from a Jeopardy! champion to a healthcare solution exposed a stark contrast in the types of data it handled. The initial training, which relied on roughly 200 million pages of relatively structured text, differed significantly from the complex, largely unstructured medical records that constitute about 80% of healthcare data. This shift presented a substantial obstacle for Watson, whose algorithms, while touted as leveraging "deep learning," seemed less adept at handling the real-world ambiguities and nuances of clinical practice.
Many doctors found Watson's treatment recommendations inconsistent with their experience and the established norms in the field, fostering skepticism regarding its reliability. This was exacerbated by Watson's difficulty in integrating with existing Electronic Health Record (EHR) systems. This integration issue significantly hampered its ability to access the very data it was supposed to leverage for improved patient care.
The $62 million MD Anderson Cancer Center project, which ultimately stalled due to Watson's inability to deliver on its promised improvements, exemplifies this struggle. It highlighted a broader question: could AI truly deliver on its promise within the complex and nuanced environment of clinical practice? The high-stakes nature of medical decision-making demanded a level of accuracy and trustworthiness that Watson consistently failed to achieve. This shortcoming, in turn, fueled caution and a preference for more established practices among many healthcare professionals.
IBM's decision to offload Watson Health to Francisco Partners for over $1 billion in 2022 is telling. It signifies a dramatic change in IBM's expectations and underscores the significant gap between initial hype and the practical challenges faced when attempting to implement AI in a field as complex as healthcare. The billions invested in Watson's development and integration were not met with the desired level of success, leading IBM to rethink its strategy and prompting reflection within the broader AI community on the realistic scope and limitations of AI in multifaceted domains.
The disappointment surrounding Watson's performance highlights a crucial lesson in the world of technology development: success in controlled environments, like a game show, does not guarantee a smooth translation to the intricacies of real-world applications. This experience reveals the vital need for thorough assessment and thoughtful contextualization when developing AI solutions, especially in sectors where human lives and well-being are directly impacted by the technology's performance.
More Posts from aitranslations.io: