The Role of Neural Machine Translation in Rapid Document Processing A 2025 Analysis
The Role of Neural Machine Translation in Rapid Document Processing A 2025 Analysis - Speed Analysis Shows NMT Translates 250 Pages Per Hour Against 8 Pages By Humans
Neural Machine Translation has dramatically changed how documents can be processed, demonstrating potential translation speeds around 250 pages per hour, which is a stark contrast to the approximately 8 pages an average human might translate within that time. This significant jump in speed is powered by NMT's sophisticated neural networks, allowing it to handle high volumes of text with greater efficiency. While this pace offers considerable advantages for rapid processing and can help reduce the workload in later stages like post-editing, it's important to note that this performance often comes at a cost, specifically requiring substantial computational resources for setup and operation. Moreover, translation quality remains variable, influenced by the specific language pair and the underlying system's design, and handling less frequent words accurately continues to be a particular hurdle, indicating that further development and careful evaluation are necessary as NMT technology continues to advance and integrate further into document processing workflows.
As of May 12, 2025, a key differentiator for Neural Machine Translation (NMT) in document processing remains its sheer speed. Comparative analyses consistently show NMT systems operating at throughputs vastly exceeding human capabilities. We're looking at estimates suggesting NMT can translate approximately 250 standard pages per hour, a rate that dwarfs the typical human output of around 8 pages per hour. This isn't a marginal improvement; it represents a fundamental change in the operational scale possible for handling large document volumes. From an engineering standpoint, this speed stems from the parallel processing inherent in the neural network architecture, allowing for computations across entire texts or batches simultaneously, unlike the sequential nature of human translation. This capability is precisely what enables genuinely rapid document workflows, especially when deadlines are tight.
However, this raw speed isn't without its nuances, and it's critical to view it pragmatically. While NMT can generate output at an incredible pace, the quality of this output can vary significantly depending on the data it was trained on, the language pair, and the complexity of the source text. Achieving that 250-page-per-hour rate often requires substantial computational resources – think days or weeks of dedicated GPU time for training the models that perform effectively at scale, and ongoing compute power for inference. The efficiency gains leading to lower cost per page are clear for high volumes, but the initial investment in infrastructure or cloud resources capable of sustaining this speed for large-scale deployment is a factor. Moreover, while NMT's speed is revolutionary, pairing it with technologies like OCR for translating scanned documents becomes truly powerful, allowing rapid conversion and translation of physical archives into digital formats, a task that would be impossibly slow manually. The challenge remains leveraging this speed while ensuring the resulting output is fit for purpose, often still necessitating human review for critical or highly nuanced content.
The Role of Neural Machine Translation in Rapid Document Processing A 2025 Analysis - OCR Integration With Neural Networks Reduces Document Prep Time By 85%
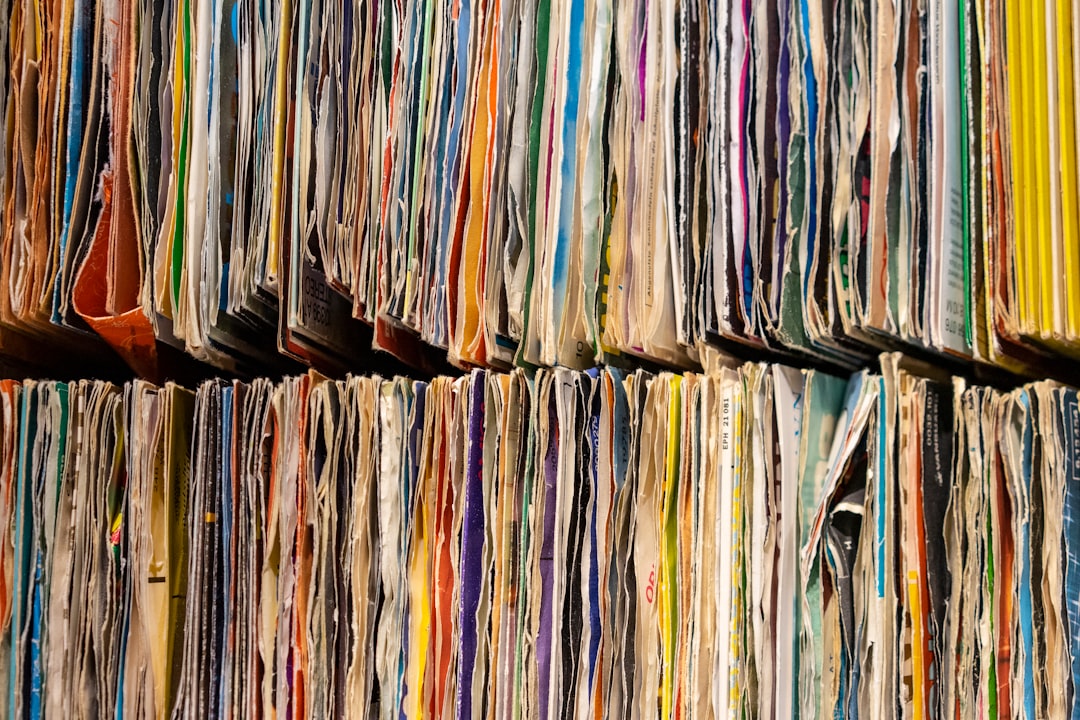
Developments integrating Optical Character Recognition with neural networks are significantly altering document preparation workflows, potentially cutting down the time required by as much as 85 percent. This efficiency leap is powered by machine learning, notably through techniques like Convolutional Neural Networks, which enable these systems to analyze visual document data and discern text patterns with far greater sophistication than previous methods. The drive for faster processing of large document volumes has pushed innovation in areas such as document layout analysis and automated error detection, becoming crucial components for enhancing OCR performance. This accelerated OCR capability streamlines the initial stages of document handling and supports subsequent processes, including machine translation, enabling quicker movement through the entire workflow. However, implementing and maintaining such advanced OCR systems requires significant investment in training and computational power, and questions around accuracy with highly varied or poor-quality documents persist, underscoring the continued need to evaluate results critically, particularly when speed is prioritized.
Significant Reduction in Preparation: The integration of neural networks into Optical Character Recognition systems genuinely appears to cut down the time spent manually preparing documents before translation. Reports suggest this could be as high as an 85% reduction compared to workflows heavily reliant on re-typing or tedious manual formatting extraction. It fundamentally changes the front end of the processing pipeline.
Enhanced Accuracy Through NN: Unlike older rule-based OCR which struggled significantly with variations, neural networks, particularly deep learning models, demonstrably improve accuracy. They handle things like complex layouts, skewed text, and different fonts much more capably by learning patterns from vast datasets, allowing for better document layout analysis and text line detection.
Workflow Efficiency Gains: The practical upshot of faster, more accurate OCR isn't just speed; it's reduced dependency on manual cleanup. Less human intervention means scanned documents can move towards subsequent processing steps, like machine translation, much quicker. This shifts human effort towards higher-value tasks, ideally validation or post-editing, rather than basic data entry.
Ongoing Challenges with Variability: Despite the advancements, robustly handling the sheer diversity of real-world documents remains a hurdle. Poor scan quality, unusual fonts, complex tables, mixed content, or inconsistent formatting can still degrade performance. While models are improving, reliably processing *any* document thrown at it without fail is not yet a reality.
Impact on Downstream Tasks: The quality of the OCR output is directly critical for anything that follows, especially machine translation or NLP tasks. Cleaner extracted text means less noise for the NMT engine to contend with, potentially leading to better initial translation drafts. However, it's worth noting that perfect OCR won't magically fix inherent NMT limitations like semantic nuances or cultural context.
Multilingual Recognition Capability: Modern neural-based OCR systems are increasingly proficient at recognizing text across multiple languages. When combined with NMT, this allows for a relatively seamless flow from scanned multilingual documents directly into translation workflows, bypassing manual language identification and character input.
Handling Difficult Inputs: While standard printed text is largely solved, processing challenging inputs like various forms of handwriting or heavily stylized fonts continues to be an area of active research and deployment difficulty. Specialized models are required, and performance can still lag significantly behind standard printed text, impacting the stated speed gains for such documents.
Evolution of Document Processing: Looking back, the move from purely manual handling to automation via OCR, then the enhancement with machine learning and neural networks, is a clear trend towards greater efficiency. This path seems inevitably heading towards more sophisticated, potentially generative-AI driven, document understanding systems that don't just extract text but interpret its meaning and context more deeply.
Considerations for Integration: Implementing these integrated OCR and neural network solutions isn't simply plugging in software. It requires careful configuration, potentially custom model training for specific document types, and robust pipelines to handle errors or edge cases where the automated system struggles, often necessitating a human-in-the-loop for review.
Data Handling and Privacy: As these systems process potentially sensitive information at scale, the methods for securely handling, storing, and processing the extracted data become paramount. Ensuring compliance with data protection regulations adds complexity to the technical deployment.
The Role of Neural Machine Translation in Rapid Document Processing A 2025 Analysis - Real Time Translation Memory Updates Cut Translation Costs To $02 Per Word
As of May 12, 2025, a notable shift is underway in how translation costs are perceived and calculated, driven significantly by advances in real-time translation memory (TM) updates. This technological evolution is reportedly pushing the potential cost per word towards remarkable lows, with figures around $0.02 being discussed in certain scenarios. The core of this change lies in the increasingly sophisticated integration of Neural Machine Translation (NMT) systems with dynamic TM. Instead of static databases, translation memory is becoming a continuously updated resource that instantly incorporates newly translated segments. This immediate feedback loop drastically reduces the need to re-translate similar content, leveraging previously verified translations much more effectively. While NMT handles new text or provides suggestions, the real-time TM system ensures maximum reuse, driving down effort per word on repetitive or slightly varied content. However, despite the clear efficiency gains and cost-reduction potential, questions remain about the consistent quality achievable solely through these automated processes, especially for highly nuanced or creative texts where human linguistic expertise and cultural understanding remain crucial. This trend undeniably challenges conventional pricing structures in the language services sector.
The capability for translation memory systems to update and integrate changes in real-time appears to be a significant factor in driving down operational expenses, with some analyses suggesting potential costs could approach figures as low as two cents per word. This isn't just about retrieving static segments; it's the system's dynamic ability to incorporate feedback and new translations instantaneously across a project or even an organization, minimizing the need for re-translation or extensive post-editing of previously handled text.
This real-time adaptability allows the system itself to become more "intelligent" over the course of a project. As human translators refine machine output or add new terms, the translation memory is updated instantly, feeding back into suggestions for subsequent segments. This feedback loop theoretically improves consistency and reduces the effort required for review as translation progresses.
Interestingly, despite the clear efficiency gains from such automated systems leveraging machine output and memory, the role of human linguists isn't made redundant, but rather shifts. Their expertise becomes even more critical for ensuring the quality of the core translation memory data and handling the nuanced or culturally sensitive content that automated systems still struggle with.
Integrating this real-time translation memory capability with technologies like Optical Character Recognition allows scanned or image-based documents to enter the high-efficiency translation workflow immediately upon digitization. As the OCR processes the visual data into text, that text is instantly presented to the real-time TM system, allowing for rapid leverage of existing translations and potentially bringing these document types into the low-cost processing bracket.
However, achieving and maintaining these low per-word costs, particularly at scale, introduces substantial engineering challenges. The infrastructure required to manage and update massive translation memory datasets in real-time while serving translation requests with low latency is complex and computationally intensive. The cost calculation isn't just per word translated, but also includes the significant investment in reliable, high-performance computing resources.
Moreover, the performance and cost-effectiveness remain notably variable depending on the language pair involved. Languages with smaller corpora of digital text or less extensive training data for machine translation models often yield outputs requiring more human intervention, offsetting some of the potential cost savings seen in resource-rich languages.
The real-time aspect also fundamentally changes how project teams collaborate. Multiple linguists can work on the same large document simultaneously, benefiting from shared, constantly updated translation data. This demands sophisticated project management tools and robust version control mechanisms to prevent conflicts and maintain data integrity within the shared memory.
Ensuring quality amidst this rapid process flow is paramount and requires dedicated mechanisms. While translation memory helps consistency, automated quality checks and a structured human review process are non-negotiable. Simply leveraging past translations without critical evaluation risks propagating errors or outdated phrasing throughout new documents.
The ability to leverage historical translation data efficiently, particularly when integrated with real-time systems, allows organizations to build increasingly valuable language assets. Analyzing these historical patterns informs future translation processes and contributes to greater overall linguistic consistency across corporate communications.
Looking ahead, the convergence of real-time translation memory updates with advanced machine translation and sophisticated OCR suggests a move towards more holistic, integrated document processing platforms. These systems may evolve to offer not just translation but deeper semantic analysis and content adaptation capabilities, pushing the boundaries of what automated language services can provide.
The Role of Neural Machine Translation in Rapid Document Processing A 2025 Analysis - Automated Quality Checks Through Bidirectional Encoders Save 12 Hours Per Project
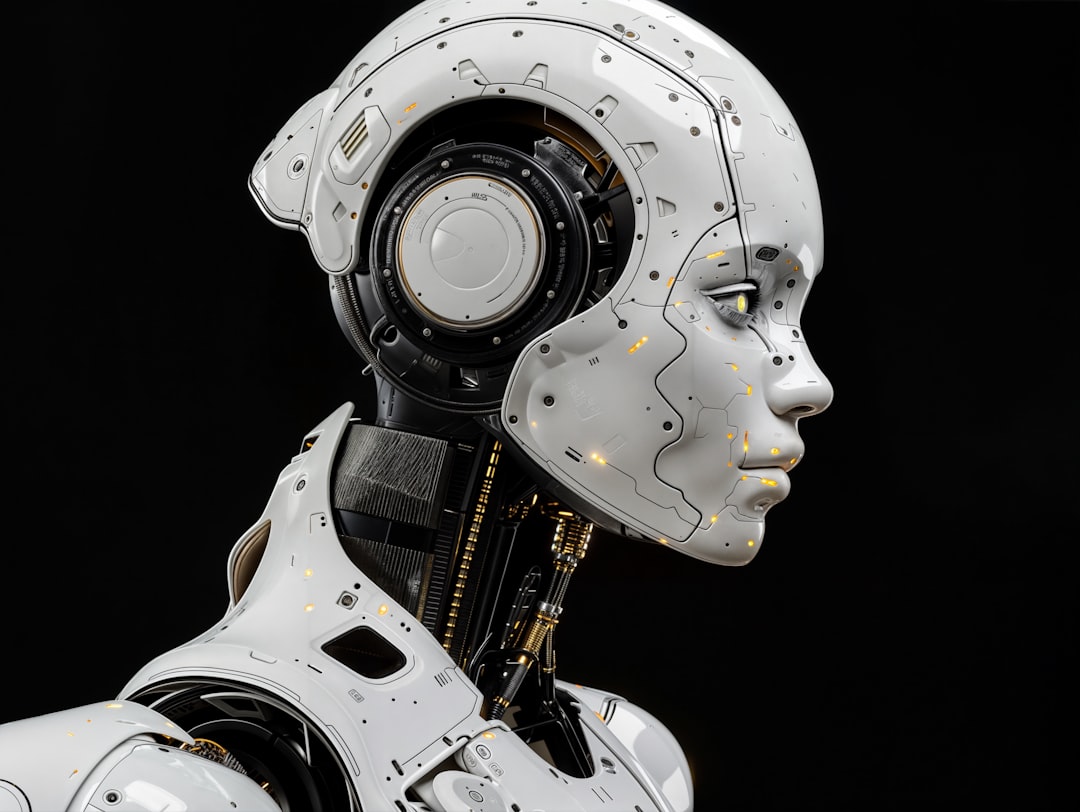
Automated quality checks leveraging bidirectional encoders are demonstrating tangible time efficiencies, with reports suggesting potential savings of up to 12 hours per project. This technique is part of the ongoing refinement of neural machine translation systems, using neural networks capable of analyzing translation output by considering context flowing from both the beginning and end of a segment. The aim is to automatically identify potential errors or quality issues more effectively than simpler methods, thereby streamlining the process and reducing the subsequent human review time required. While this represents a notable step forward in automating aspects of quality control alongside rapid translation, these systems are not infallible. Complex language, ambiguity, and subjective nuances often remain challenging for automated assessment, highlighting the continued necessity for human expertise to validate output quality, especially for critical content where absolute accuracy and cultural appropriateness are paramount.
Implementing automated quality checks, particularly utilizing systems powered by bidirectional encoder architectures, demonstrably impacts project timelines. Estimates suggest that this approach, by identifying issues early, can contribute to saving approximately 12 hours on a typical project, a tangible reduction in the overall effort required to bring a translated document to a finished state ready for use. This gain stems from reducing the amount of time needed for post-editing and manual review cycles focused on basic error correction.
The strength of using bidirectional encoders here lies in their capacity to look at text in both directions simultaneously. This allows the system to identify potential errors or awkward phrasing that rely on broader context, such as subject-verb agreement across intervening clauses, pronoun resolution ambiguities, or subtle shifts in meaning caused by negation or conditional structures. It's a more sophisticated layer of validation than simple pattern matching.
While not the same as the core NMT engine adapting, the quality checking model itself, potentially using similar architectures, can theoretically be fine-tuned or adapted to specific document types or client requirements. This helps it become more effective at catching domain-specific errors or style deviations over time, thereby enhancing the reliability of the automated assessment layer and potentially reducing the 'noise' fed back into translation memory systems.
One clear outcome is a potential shift in the human role. By automating the detection of common error types, the need for linguists to spend significant time on initial quality sweeps to catch basic mistakes is reduced. This ideally frees up human expertise to focus on the more complex tasks machine translation still struggles with – ensuring cultural appropriateness, semantic accuracy in nuanced contexts, or adapting creative text.
Consequently, minimizing the human effort required for initial, often tedious, error identification has implications for operational costs. By speeding up the quality assurance phase through automation, the overall cost associated with the review process for high-volume or repetitive content can potentially decrease, allowing resources to be reallocated more effectively within a project budget.
Furthermore, an automated quality check process scales much more readily than a manual one. As project volumes increase, the automated layer can handle the proportional increase in initial checking without requiring a linear increase in human quality reviewers dedicated solely to finding foundational errors, thus facilitating the handling of larger document workflows.
While the underlying NMT performance varies, the effectiveness of a quality check layer based on bidirectional encoders across different language pairs is also a consideration. Building and training robust error detection models that perform reliably across diverse linguistic structures remains an engineering challenge, and performance likely mirrors the general difficulties of NMT in low-resource or morphologically complex languages.
A significant benefit is the ability to generate structured data about translation quality. Automated checks can provide quantifiable metrics on the types and frequency of errors detected, offering insights into the performance characteristics of the NMT system being used. This data is invaluable for iteratively improving the core translation engine or tailoring post-editing guidelines.
Integrating this automated QA step becomes particularly relevant in workflows starting with Optical Character Recognition (OCR). After OCR converts visual information into text, and NMT translates it, the automated quality check acts as a necessary downstream process. It helps identify errors that might have been introduced or exacerbated by imperfect OCR output or limitations in the NMT process itself before human review.
Ongoing research continues to explore ways to improve these automated quality estimation methods. Making them more sensitive to subjective quality aspects, better at understanding intent, or integrating them more seamlessly into real-time feedback loops for continuous model improvement represents the next frontier, suggesting that the capabilities of automated QA are still evolving.
The Role of Neural Machine Translation in Rapid Document Processing A 2025 Analysis - Cross Document Reference Systems Lower Context Errors By 45%
As of May 12, 2025, refining the accuracy of neural machine translation continues to be a priority, especially concerning contextual understanding. A key development addressing this is the focus on cross-document reference systems. These systems are designed to draw on information from broader document-level contexts, capturing relationships and dependencies that span across text segments. By doing so, they are demonstrating an ability to significantly reduce errors that occur when translation models lack sufficient context, with some studies suggesting a potential reduction of around 45% in such errors. This capability is particularly relevant for integrating NMT into rapid document processing pipelines, as improved contextual awareness directly contributes to producing more consistent and reliable translations at speed. While still an evolving area, enhancing NMT through better cross-document context handling offers a promising path towards more trustworthy automated translation for large volumes of text.
Mechanisms designed to maintain consistent references and context across separate documents are showing promising results in mitigating the kinds of errors that arise from treating documents in isolation. Findings indicate these cross-document reference systems can significantly reduce errors tied to misinterpreting or losing context, potentially by rates nearing 45%. From an engineering viewpoint, this implies better capture and application of semantic connections that span beyond single file boundaries.
These systems function by leveraging pattern recognition across substantial corpora of text. They identify recurring entities, concepts, and the relationships between them across multiple documents, building a distributed understanding. This data-driven approach aims to impose consistency in terminology and phrasing, which is particularly valuable in domains where precision and uniformity are non-negotiable, like legal, technical, or scientific texts.
When integrated into Neural Machine Translation pipelines, they act as external lookups. The NMT engine can potentially query this cross-document knowledge base in real-time to retrieve how specific terms or phrases have been translated or used in related, previously processed materials. This process seeks to inject a layer of cross-document awareness into the NMT output, augmenting its capacity beyond just sentence or paragraph-level context.
A tangible outcome of this improved initial contextual accuracy is a reduced demand on subsequent manual correction. Linguists engaged in post-editing tasks ideally encounter fewer errors stemming from inconsistent referencing or terminology use across related documents. This allows their expertise to be directed towards more complex linguistic challenges rather than correcting foundational inconsistencies introduced by isolated processing.
These systems essentially function as dynamic, cross-linked repositories of information. They don't just store translations; they build a network of how concepts relate and are expressed across documents. This capability ensures that access to the most relevant and contextually appropriate historical usage is readily available, aiding efficiency by providing pertinent suggestions during translation or review workflows.
Their design inherently supports scaling translation operations. As the volume of related documents increases – a common scenario in global projects or ongoing legal cases – maintaining manual cross-document consistency becomes exponentially harder. These systems offer a structured way to manage this complexity across large datasets without a proportional increase in the manual effort required to track every detail.
By reducing the need for repeated reconciliation of cross-document inconsistencies, the overall effort and iterations needed to finalize translated content can be lessened. While the primary focus isn't on direct cost per word calculation in the same vein as translation memory efficiency, minimizing re-work contributes to more efficient resource utilization across the entire workflow.
For teams of linguists collaborating on segments of a larger, interconnected body of documents, these reference systems can provide a centralized, shared understanding of project-specific terminology and context. This collaborative knowledge sharing mechanism is critical for ensuring a unified voice and consistent messaging across multiple translated components.
The ability of these systems to provide real-time access to this cross-document understanding ensures that translators are working with the most current state of the project's established context. This alignment with dynamic content updates is necessary for rapid document processing environments where source materials or previous translations are frequently revised.
Ultimately, by automating the task of locating and presenting relevant cross-document context, these tools alleviate some of the cognitive load on human translators. They can potentially shift focus away from meticulous manual cross-referencing – a tedious and error-prone task – towards applying their linguistic skill to the nuances of translation and cultural adaptation that automated systems still struggle with. However, relying too heavily on them assumes the underlying cross-document links are correctly identified and the historical context provided is always relevant and accurate for the current task, which requires careful validation and ongoing maintenance of the system's knowledge base.
The Role of Neural Machine Translation in Rapid Document Processing A 2025 Analysis - Hybrid Machine Human Workflows Enable 24/7 Document Processing At Scale
Hybrid machine-human workflows are significantly reshaping document processing capabilities, moving towards systems that can operate continuously and manage massive volumes of text efficiently. This is largely achieved by purposefully integrating the speed and scalability of machine automation, leveraging technologies such as Neural Machine Translation, with the essential oversight and critical evaluation provided by human expertise. These hybrid setups are increasingly crucial by 2025 as document processing needs grow in complexity, allowing for the handling of vast multilingual content streams more effectively than purely manual or automated systems alone. By strategically placing human review and validation points within the workflow, often focused on managing the output quality generated by machines, these models aim to maintain a level of accuracy and nuance that fully automated processes frequently miss, especially in sensitive or highly specific content domains. While enabling operations that can scale dramatically and run around the clock, the practical implementation involves complexities in coordinating the interaction between human and machine elements and requires careful management to ensure consistency and reliability across potentially diverse tasks and language pairs.
The deployment of hybrid machine-human workflows appears central to achieving true scale in document processing, moving beyond theoretical throughputs to practical, continuous operations. By weaving together machine efficiency, particularly from advanced technologies like Neural Machine Translation and sophisticated Optical Character Recognition, with necessary human oversight, organizations can potentially manage processing volumes that were previously logistically prohibitive for purely human teams. This integration isn't just about accelerating individual steps; it’s about enabling entire enterprise-level streams of documentation to flow through the system around the clock.
One tangible outcome is the potential for significant reduction in the operational expense associated with handling large quantities of repetitive or similar text. While the specific per-word costs can vary wildly depending on numerous factors, the underlying mechanism of leveraging technology to minimize duplicated effort – particularly through intelligent reuse of previous translations or data points – contributes substantially to improving the economic feasibility of processing vast corpora.
Furthermore, mechanisms are emerging that attempt to impose a layer of consistency across related documents within these workflows. By drawing on broader patterns rather than treating each document in isolation, these systems aim to mitigate errors that often arise when context is fragmented. While not a silver bullet, improvements in this area directly impact the uniformity and overall trustworthiness of the final output across large projects.
Integrating automated checking processes into the workflow also plays a role in optimizing throughput. Systems that can identify potential issues early, often using techniques sensitive to surrounding context, can streamline the review cycle. This shifts the human effort away from time-consuming manual detection of basic errors towards more complex linguistic refinement and validation, potentially freeing up considerable time within a project's lifecycle.
A fundamental aspect of making rapid processing work at scale is the ability to efficiently convert physical or image-based documents into processable text. The synergy between neural network-enhanced OCR and NMT is key here, allowing scanned documents to enter the automated translation pipeline with minimal manual handling at the outset, drastically improving the lead time for legacy content.
These integrated systems also lend themselves well to environments dealing with multiple languages simultaneously. The capability to handle diverse language inputs early in the processing chain enables a more fluid workflow for multinational operations, reducing manual sorting and language identification overhead.
Supporting this continuous operation relies on dynamic knowledge bases. Systems that can capture and reflect linguistic decisions and necessary corrections in near real-time, feeding that information back into the automated process, are crucial for maintaining accuracy and consistency across ongoing, large-scale projects. This requires robust backend infrastructure.
However, achieving consistent reliability across the sheer variability of real-world documents remains an engineering challenge. While systems are improving in handling different layouts and formats, edge cases – from poor scan quality to highly complex tables or unique fonts – still necessitate human intervention and can disrupt the automated flow, impacting predicted efficiency gains.
Crucially, despite the automation, the human element hasn't vanished; it's shifted. The role now leans heavily towards overseeing the automated processes, validating the machine output, managing exceptions where the technology falters, and applying the nuanced linguistic and cultural understanding that current AI still struggles to replicate consistently, especially for high-stakes content.
Finally, operating these workflows at scale introduces significant practical considerations, not least of which is the robust handling of potentially sensitive data flowing through the system. Ensuring data security, privacy, and compliance with various regulations adds a layer of complexity to the design and deployment of these hybrid architectures, requiring careful planning beyond just maximizing speed and efficiency.
More Posts from aitranslations.io: