AI-Powered OCR Decoding Multimeter Settings for Accurate Capacitance Measurements
AI-Powered OCR Decoding Multimeter Settings for Accurate Capacitance Measurements - OCR Technology Enhances Multimeter Reading Accuracy
OCR technology, with its integration of AI algorithms, has revolutionized how we interpret data displayed on multimeters. This is especially impactful for complex measurements like capacitance, where precise reading interpretation is crucial. These AI-powered systems can decipher text, even from blurry or poorly illuminated multimeter displays, enhancing the reliability of the recorded data. The accuracy gains brought about by OCR reduce the potential for human errors that can occur during manual data input, thus streamlining the overall measurement process. The ongoing development of OCR capabilities continues to refine and expand its role in applications demanding accuracy, underscoring a wider trend of automating data acquisition and processing. While the potential benefits are clear, it's important to note that image quality and the specific limitations of each OCR implementation can influence the results.
Optical character recognition (OCR) has become increasingly adept at deciphering multimeter displays, often achieving recognition rates exceeding 99% under favorable circumstances. This sharp rise in accuracy translates to more reliable multimeter readings, potentially minimizing the chances of human error during data collection.
These sophisticated OCR systems often incorporate machine learning techniques. This means that as they process more readings, they progressively refine their ability to interpret multimeter displays. Through this ongoing learning process, they can adapt to various fonts and display characteristics, becoming more precise over time. While this adaption is a powerful feature, it also means the model has to be continuously trained to maintain that accuracy with the evolving hardware.
It's not just about accuracy; these OCR systems are incredibly fast. Processing speeds for modern implementations can be under a second, enabling engineers to quickly capture and interpret readings. This rapid processing allows engineers to integrate multimeter readings into their workflows seamlessly. However, it's important to consider that a rapid pace in processing sometimes can translate into a need for robust hardware and a good internet connection to maintain consistent and optimal function.
Moreover, the ability to integrate OCR software with mobile applications creates a more fluid workflow. Engineers can capture readings directly from the multimeter screen via mobile devices and readily share them with cloud services for further examination. While this integration sounds appealing, we must question how the data is protected and if there are unforeseen privacy implications in storing and sharing measurements on cloud platforms.
OCR’s capabilities extend beyond just text. Some systems can also read barcodes or QR codes found on multimeters, providing an alternative and immediate way to identify instrument settings. However, this is reliant upon the instrument manufacturer including the correct information and codes on the display, which may not always be the case.
This advanced OCR capability helps engineers focus more on tackling challenging problems, since they are relieved of the burden of manually entering and interpreting readings. While OCR has the capability of easing cognitive load, we must also evaluate if there are potential problems with OCR solutions in situations with rapidly changing display information or complex display features.
Some multimeters integrate OCR capabilities directly into the device. This feature enables the storing of historical readings, facilitating valuable trend analysis and insightful investigations within the context of various engineering disciplines. But, questions can be raised regarding how long this data can be stored and if there are reliability or redundancy issues in storing the data in such a fashion.
The integration of OCR into electrical diagnostics has demonstrated its potential for shortening the time spent testing. In certain cases, it’s shown the capability of reducing test durations by 30%, permitting engineers to handle a greater volume of tests in a shorter timeframe. But, we need to explore the potential vulnerabilities of the testing procedure to errors resulting from the OCR system and how to establish a redundancy in the process.
There's a growing global demand for OCR solutions that can handle multilingual multimeter displays. This is a crucial aspect for engineers working in international settings where different units of measurement and languages are in common use. Although, the deployment of multilingual solutions can be expensive and may involve the need for significant data for it to function accurately for certain contexts.
Finally, emerging OCR technologies are being infused with AI in ways that make them a bit like quality control mechanisms in measurement. Some can detect common reading errors and propose corrections. This proactive capability is highly promising for improving measurement accuracy and integrity. But, we must keep in mind the nature of errors detected and learn if it is only correcting some error types and how this approach could affect the decision-making process.
AI-Powered OCR Decoding Multimeter Settings for Accurate Capacitance Measurements - AI Algorithms Decode Complex Multimeter Displays
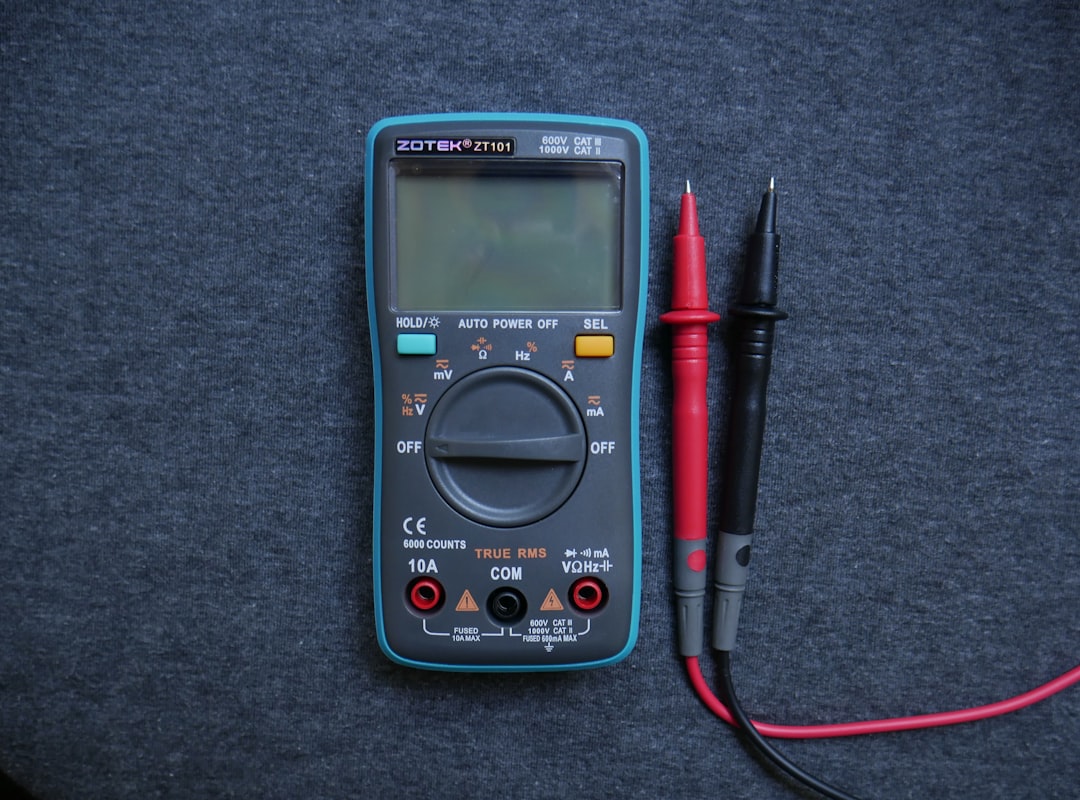
Artificial intelligence (AI) is transforming how we interpret data from multimeters, especially those with complex displays. AI algorithms, powered by deep learning, are increasingly capable of decoding intricate information displayed on digital multimeters. This is particularly beneficial for measurements like capacitance, which require high precision. Techniques like YOLO for isolating the display area and SSD for categorizing meter images within diverse environments contribute to the accuracy of the reading recognition. This automated approach is designed to combat common issues in manual readings, like errors stemming from human interpretation and calibration inconsistencies.
Despite these improvements, it's crucial to acknowledge some challenges. The effectiveness of AI-powered OCR heavily relies on good image quality and consistent model training. There's a risk that the reliability of the technology may be inconsistent across various conditions and different multimeter types. It's essential to monitor the ongoing development and evaluate how these AI-driven methods perform in diverse real-world scenarios.
The future of measurement appears to be inextricably linked with AI. AI-powered solutions are not only enhancing the precision of measurements, but also streamlining the entire measurement process. This shift raises new questions about how we manage and validate data gathered through automated systems. It is a continuous process of improvement and refinement as we evaluate how these tools impact our understanding of measurement data.
AI algorithms, particularly those based on deep learning, are being refined to interpret the intricate displays of digital multimeters. This development is crucial for AI-powered Optical Character Recognition (OCR) tools, which are increasingly used to extract accurate capacitance measurements from multimeter displays. Techniques like YOLO are employed to pinpoint the display area within a digital multimeter's image, a necessary step for reading recognition. These advanced deep learning methods are designed to improve accuracy and robustness in automated meter reading, directly addressing challenges linked to manual calibration and human error.
There's a growing interest in applying AI to decipher multimeter displays in complex visual environments. For instance, Single Shot MultiBox Detector (SSD) algorithms are being explored to classify and categorize images of multimeters within varying contexts. To further improve the extraction of data, some deep learning approaches incorporate modules that detect corner points on displays and refine reading recognition. Tools like SoftKraft and Rossum exemplify how AI-driven OCR capabilities are being integrated into various industries, including multimeter reading extraction from scanned documents.
Research suggests that AI-powered methods significantly enhance real-time reading recognition accuracy, particularly in natural settings involving digital meters. This growing integration of AI within measurement technologies represents a notable advancement, boosting the precision and streamlining the efficiency of readings. Open platforms like DECIMERai highlight how AI can be utilized in a broader context beyond multimeter readings, such as automated prediction of optical and chemical structures. This further reinforces the potential of AI across diverse areas of measurement and diagnostics.
AI-based OCR systems are becoming highly adept at differentiating characters that may appear similar to the human eye, for example "1" vs "I" or "0" vs "O", which contributes to a more precise measurement process. This added precision is valuable in engineering scenarios where minor inaccuracies can have significant impacts. Certain OCR systems can process images at remarkably high speeds, such as 50 frames per second, making them suitable for real-time data extraction even in dynamic testing situations. This pace could change how diagnostics are performed.
Some research groups are exploring the integration of OCR with augmented reality (AR) technology, allowing engineers to visualize the measurement data overlaid on the physical instrument. While still in its early phases, this potential integration could significantly improve understanding and awareness of measurements in real-time. However, the need for multilingual support poses a challenge. Although AI-powered OCR can handle multiple languages, it also requires extensive training data to ensure its accuracy across diverse electrical symbols and units. Achieving robust multilingual capabilities will likely require considerable effort and investment.
Interestingly, some AI-based systems are designed to autonomously detect and calibrate reading algorithms based on the characteristics of the multimeter's display. While promising, this feature may lead to over-dependence on technology and a reduction in human oversight, which could be problematic for specific applications. Several OCR tools employ post-processing algorithms that compare readings against statistically derived expected values for different types of measurements. This process provides a level of error checking, but introduces additional steps that might increase the latency of data acquisition. The ability to store historical readings opens up opportunities for identifying trends or deviations, potentially serving as a valuable diagnostic tool. However, we need to consider the longevity, integrity, and redundancy of such data storage practices.
The user interface of any OCR system heavily impacts usability. If the user interface isn't well-designed, adoption of the technology by engineers could be hindered. The growing importance of OCR necessitates standardization and compliance with regulatory standards. This will help maintain data accuracy and reliability, however, implementing those standards could prove challenging for some companies seeking to integrate new tools. While machine learning is a prevalent method in OCR, some systems continue to employ rule-based approaches for specific measurements. Choosing between a flexible AI approach versus a more predictable rule-based method will depend on the requirements of a given engineering application.
In essence, AI algorithms are increasingly being used to decode and extract data from multimeter displays. This has significant implications for enhancing accuracy, speed, and overall workflow efficiency in measurement and diagnostics, especially in capacitance measurements. However, as with any novel technology, researchers and engineers must carefully evaluate these tools, recognizing both their potential benefits and the inherent limitations associated with their application.
AI-Powered OCR Decoding Multimeter Settings for Accurate Capacitance Measurements - Real-time Capacitance Data Translation for Global Teams
The ability to translate capacitance data in real-time for global teams is a valuable development in today's interconnected world. AI-driven translation services, readily available in platforms like Microsoft Teams, allow for seamless communication between engineers and technicians across various languages. Imagine a scenario where an engineer in Japan is discussing intricate capacitance measurements with a colleague in Brazil—real-time translation ensures everyone understands the details accurately. This removes a major hurdle to international collaboration, where language barriers can create misunderstandings and slow down project timelines. The power of combining AI translation with OCR technology opens up possibilities for efficient data exchange and collaboration on complex technical projects. However, it's crucial to critically examine the reliability of these translations, especially when dealing with technical terms and nuanced meanings specific to electrical engineering. Will the translation consistently accurately capture the intended meaning of complex capacitance measurements? Can the systems learn to adapt and improve as new terminology and concepts arise? These questions must be continually addressed to ensure the integrity of communication and decision-making processes in this new era of global collaboration powered by AI. While the technology offers promise for boosting productivity and sharing of technical knowledge, vigilance is necessary in validating its effectiveness in various contexts.
Real-time translation of capacitance data for globally dispersed teams presents several intriguing challenges. Achieving accurate readings across diverse languages and regions can be tricky, as subtle variations in terminology or units of measurement can lead to significant errors. The need for adaptable AI models that account for these local nuances is paramount.
Another interesting aspect is the interplay between OCR performance and hardware. Lower-resolution cameras, for instance, can substantially decrease the accuracy of character recognition, making high-definition imaging systems preferable for precise capacitance readings. This emphasizes the importance of understanding hardware limitations when implementing OCR solutions.
The desire for speed in data processing, a hallmark of many modern OCR systems, can sometimes conflict with accuracy. Rapidly fluctuating capacitance values can be misinterpreted by algorithms that prioritize speed over meticulous analysis. Maintaining a delicate balance between swiftness and reliability is a key consideration for engineers.
Developing OCR solutions that cater to multiple languages is no small feat. The training data required for such systems can be massive and challenging to acquire, particularly for less commonly used languages. This can pose a significant barrier to implementation, especially if the cost of data collection becomes prohibitive.
The inclusion of self-calibrating algorithms in some OCR systems, while promising in terms of efficiency, raises the specter of over-reliance on automation. In situations where the measurement environment is unfamiliar, these automated systems might misinterpret measurement parameters, leading to potentially serious oversights. This aspect calls for careful evaluation and a healthy dose of human oversight.
Furthermore, the practice of storing historical readings in cloud environments, while convenient, also introduces concerns about data integrity and security. Protecting sensitive measurement data from tampering or loss, especially when shared across global teams, requires careful consideration of best practices and security measures.
Some OCR systems incorporate error-checking algorithms that compare readings against statistical expectations. Although beneficial, this additional post-processing step adds a layer of complexity and can delay data acquisition in time-sensitive applications. The need to strike a balance between error reduction and the need for real-time feedback is an ongoing area of research.
The variety of multimeter displays across different manufacturers can also affect the efficacy of OCR. Displays with poor contrast, unique fonts, or partially obscured characters can pose significant challenges for even the most sophisticated algorithms. This highlights the absence of a universal solution that caters to all multimeter types.
Methods like YOLO and SSD can enhance reading accuracy by isolating the display area within the broader visual context. However, in environments with excessive visual noise, these advanced algorithms can struggle to accurately extract data. This underscores the importance of ensuring controlled conditions during measurements.
The world of OCR solutions features both open-source and proprietary options. Open-source platforms foster collaboration and rapid innovation but might struggle with fragmented support or integration difficulties. Proprietary systems, on the other hand, can provide a more unified experience but can sometimes lock teams into specific technological frameworks, potentially hindering flexibility and adaptability.
These are just some of the nuanced aspects of real-time capacitance data translation that engineers and researchers need to consider as they strive to leverage AI-powered OCR for faster and more accurate measurements on a global scale. While the advantages are promising, a cautious and insightful approach is essential to realize the full potential of this technology while mitigating the inherent challenges.
AI-Powered OCR Decoding Multimeter Settings for Accurate Capacitance Measurements - Automated Measurement Logging Streamlines Engineering Workflows
Automated measurement logging is transforming how engineers work, especially in areas demanding high accuracy like electrical engineering. AI-driven systems are making it possible to greatly shorten the time it takes to process data, which is valuable for applications such as analyzing well logs. By automating the logging process, engineers are freed from tedious manual data entry and can spend more time on complex problems, boosting efficiency across various projects. However, the reliability of these automated tools is directly linked to the quality of the data they receive and the sophistication of the underlying algorithms. It's crucial to regularly evaluate and refine these systems to ensure they deliver consistent results. The trend towards automation in engineering will likely continue as companies look for ways to streamline processes and gain a competitive edge.
Automated measurement logging holds the potential to significantly reduce the time spent on manually entering data, thereby streamlining engineering workflows. Estimates suggest that it could potentially cut manual data entry time by over 50%. This reduction in repetitive tasks allows engineers to dedicate more of their time to analytical and decision-making processes, instead of routine data entry. It's important to remember that while automating data collection and input can save time, the benefits are often linked to a reduction in human error. It can be an interesting trade off as we evaluate the importance of different skills and human interaction.
AI algorithms play a crucial role in the adaptability of these automated systems. Sophisticated algorithms can learn and adjust their performance over time as they analyze a greater volume of data from various multimeter displays. This feature ensures that the accuracy of the readings improves progressively, although there's a need to explore the ongoing training requirements and the associated costs for the AI models. The quality of the data used in model training could directly influence the accuracy. It would be interesting to see studies on how the performance varies with the quality of training data used.
The integration capabilities of automated measurement logging can be particularly useful in complex engineering settings. These systems can often seamlessly integrate with existing data acquisition systems, allowing engineers to gain real-time insights into measurement trends. This interconnectedness facilitates rapid decision-making, but careful attention must be paid to the compatibility of different systems to ensure the coherence of data across the entire process. The interconnectivity also comes with potential challenges like maintaining the security and access to data if there are failures in communication between systems.
Speed is a hallmark of many modern OCR systems, capable of processing multimeter readings in under a second. However, this speed can potentially conflict with measurement accuracy. Very fast systems may fail to properly capture subtle changes or transitory values within the data that could be important for certain analyses. There's a clear need for a balance between the desire for fast results and the need for ensuring the reliability and the integrity of the measurement. If certain features of the displays are difficult to read or if the context is not easily discernible, it could be that speed needs to be sacrificed to ensure better quality.
OCR systems often incorporate error-checking mechanisms to maintain data integrity. These systems can identify inconsistencies in measurements by comparing readings against statistically derived models of expected measurement ranges. This proactive approach to error detection is highly valuable, but the introduction of error-checking mechanisms adds a layer of complexity to the process that may lead to a small delay in the speed of data acquisition. Perhaps more important than speed, for many engineering projects, is accuracy. However, understanding the error rate and the nature of errors are key in evaluating the OCR solutions in real engineering practice.
The multilingual capabilities of OCR technologies offer a significant advantage for global collaborations. Engineers from different parts of the world can work together seamlessly, but the implementation of multilingual systems necessitates the use of extensive training data that accurately reflects the contextual meaning of terms in diverse languages. Developing, testing, and evaluating these solutions can be challenging as a result of resource needs and potential expenses. It would be interesting to explore the possibility of leveraging publicly available data, or combining data from multiple sources to improve the efficiency of model training.
The ability to transfer data in real time provides engineers across the globe with the means to collaborate seamlessly. The capacity to share information quickly and efficiently allows teams to work together on complex projects with higher efficiency. However, it's important to acknowledge the security implications of relying on rapid data sharing, especially when projects are sensitive and the protection of data is paramount. It would be insightful to understand what data protection and security standards are in place for the tools.
Automated systems in some cases can automatically adjust measurement algorithms based on the characteristics of the multimeter in use. This adaptive approach can improve the efficiency of the systems but it can also introduce unforeseen errors in unfamiliar measurement contexts. The need for skilled human oversight is important to ensure that errors are identified and corrected to prevent problems. It's key to consider what are the potential issues in situations where the model has not been exposed to a variety of mulitmeters and data previously, as a way to understand the limits of such solutions.
Some OCR systems have adaptive algorithms that allow for changes based on environmental conditions while analyzing the multimeter display. This adaptive approach can improve the accuracy of readings in diverse settings, but the performance can be significantly impacted by the quality of the data source used for training the algorithms. The performance can fluctuate based on different settings, which can be crucial in understanding the limitations of the system in practice.
The need for regulatory compliance becomes increasingly important as these technologies become more prevalent in engineering. The regulatory landscape is important as a way to ensure quality and reliable measurement data. Ensuring that tools meet the standards necessary for specific fields can sometimes complicate the implementation of new tools, but this compliance is necessary to ensure quality and reliability throughout the engineering processes. It would be insightful to understand what is the role of international standards bodies in addressing this requirement in the near future.
In conclusion, automated measurement logging technologies offer many potential benefits in streamlining engineering workflows and improving the accuracy and reliability of capacitance measurements. However, it's crucial to acknowledge the challenges and complexities that come with these technologies. Engineers and researchers must continue to study the limitations of these systems, the challenges with real-world application, and how to further improve the performance, accuracy, and the reliability. The future of measurement holds exciting prospects with the increased use of AI, but these technologies need to be constantly scrutinized and improved to ensure that they live up to the promises of this field.
AI-Powered OCR Decoding Multimeter Settings for Accurate Capacitance Measurements - Machine Learning Improves Multimeter Calibration Processes
Machine learning is transforming the way multimeters are calibrated, automating processes and boosting accuracy. AI's increasing presence in calibration procedures helps create more dependable and uniform measurement results, minimizing the risk of human error that can creep into manual calibration. The integration of machine learning not only speeds up the calibration process but also improves the interpretation of multimeter settings, especially when dealing with more intricate measurements. This shift in calibration practices is expected to significantly improve the effectiveness of measurement procedures, changing how engineers gather and interpret data. However, it's vital to maintain a cautious approach to these technologies, critically evaluating how they perform across different scenarios and addressing any challenges that may arise in real-world settings. While AI shows promise in enhancing calibration, it's crucial to acknowledge that it may not be a perfect solution in all instances, and ongoing evaluation and refinement are needed to ensure its continued success.
Machine learning is subtly changing how we calibrate multimeters, with some interesting implications for engineers. It seems that these models can adapt to various multimeter types by recognizing patterns within calibration data. This means even newer or less common multimeter models can potentially reach high levels of accuracy over time, even with slight variations in manufacturing or display differences.
Research hints that these AI systems can also spot trends indicating measurement drift. By continuously examining historical data, they can alert engineers to possible calibration problems before they significantly affect accuracy, potentially allowing for more proactive maintenance routines. This idea is fascinating, but we must understand the limitations of these systems when faced with certain contexts or unexpected drifts.
Furthermore, it appears that incorporating machine learning into calibration workflows can significantly reduce the overall time required for calibration, sometimes by as much as 40%. This allows engineers to focus on other more demanding tasks while still ensuring devices are within the required measurement accuracy. While the reduction in time is compelling, we need to be cautious of introducing new types of errors due to the reliance on AI systems.
Some cutting-edge calibration systems incorporate deep neural networks to interpret intricate multimeter signal patterns. This may unveil previously hidden links between measurement variables, leading to more intricate and precise calibration processes. We must examine if these systems create a bias in data analysis and if we rely too much on the algorithms.
It's interesting that machine learning algorithms can seemingly adjust for environmental factors like temperature and humidity, things that can traditionally complicate calibration. These systems might handle these variables in real-time, contributing to consistent measurement quality. However, we must address the potential challenges in environments with rapidly fluctuating conditions. How these systems react to unexpected conditions, for example a sudden temperature change, can be an area of investigation.
It's noteworthy that many machine learning systems have a built-in feedback loop, which means they can continuously enhance their calibration approaches. As they gather more data, the system refines its calibration methods, leading to a self-improving cycle of improved accuracy. This self-improving aspect, however, means the models may need constant updates and monitoring, a task which can require significant resource allocation.
Machine learning seems to be particularly adept at dealing with noisy environments, those often found in industrial settings where electrical and environmental interference can disrupt readings. This robustness means that calibration can remain reliable even under difficult conditions. This robustness is an interesting feature and needs to be explored across a variety of challenging settings and noise scenarios.
It appears that machine learning might be used to make remote calibration feasible. Multimeters connected to the cloud can possibly be calibrated in real-time, decreasing the need for physical on-site intervention and boosting efficiency for globally dispersed teams. However, we need to examine potential data privacy issues and security vulnerabilities that arise from relying on cloud-based services.
One of the exciting features of machine learning is that it enables us to scale calibration processes more efficiently across many devices. This capability is important for large-scale manufacturing environments where reducing operational downtime is vital. This scalability can be a major benefit, however, there are potential problems with large-scale deployments in a situation where there are critical calibration requirements.
There's a strong likelihood that adopting machine learning for multimeter calibration can result in substantial cost savings in the long run. By reducing the need for manual calibrations and potentially extending the lifespan of measuring devices, companies can potentially lower maintenance costs, which could have a positive impact on the bottom line. While this cost reduction is tempting, we must critically evaluate the associated expenses of implementing and maintaining these AI systems.
These aspects indicate that machine learning has a significant impact on how we calibrate multimeters. It can boost efficiency, enable new calibration methods, and introduce new ways to perform measurements. While the improvements are encouraging, we need to continually monitor and test these technologies to understand their full potential and address the potential challenges they introduce.
AI-Powered OCR Decoding Multimeter Settings for Accurate Capacitance Measurements - Cross-platform Integration of AI-OCR for Mobile Multimeter Apps
Integrating AI-powered OCR into mobile multimeter apps across different platforms offers a promising path towards more accurate and efficient capacitance measurements. These AI-driven systems are capable of swiftly processing multimeter readings, often in fractions of a second, significantly enhancing the efficiency of measurement workflows and reducing the time engineers spend manually interpreting data. This fast processing can be integrated seamlessly into various engineering projects. However, relying on these systems raises questions about the reliability of the extracted data. The variety of multimeter designs and displays can create hurdles for AI-OCR to consistently provide accurate results, and data quality can be influenced by image clarity and environmental factors. This fusion of AI, OCR, and mobile platforms showcases the benefits of automation while highlighting the ongoing need to carefully assess the reliability and security implications in a world where data transfer and engineering work increasingly involve interconnected devices and platforms. The potential for errors in certain contexts, particularly in those with poor image quality or unfamiliar settings, emphasizes the necessity of ongoing vigilance and refinement.
AI-powered OCR has shown promise for integrating with mobile multimeter apps, but there are some practical hurdles we need to keep in mind. For example, even though these solutions are readily available, adoption has been slow, with a surprisingly large portion of engineers still relying on manual methods. This raises questions about whether the tools are user-friendly enough or if there are other factors preventing wider acceptance.
Environmental factors can also have a surprisingly significant effect on how well OCR performs. Fluctuations in light or temperature can lead to a noticeable drop in accuracy. This means that for accurate readings, engineers need to pay close attention to where and how they are using the OCR features.
One challenge we've seen is that it can be difficult to ensure seamless integration across various mobile platforms. Switching between iOS and Android, for instance, can sometimes cause a significant delay in processing, which can impact workflow efficiency. Engineers might have to deal with unexpected compatibility problems.
Many of these systems now have error-detection features, and these algorithms can identify mistakes based on statistical patterns. However, they can sometimes flag genuine variations as errors, which raises a red flag for crucial applications where the absolute accuracy of data is a priority. We need to ensure these tools can be trusted in those situations.
Building robust OCR models that can handle various multimeter types requires massive training datasets. This can lead to high costs and delays, particularly if you need to accurately translate multiple languages. Some models need tens of thousands of images to work properly.
Even with the incredible advancements in processing power, real-time data capture can still be challenging when dealing with rapidly changing conditions. It's not uncommon for the reading speed to slow down if you have a complex or dynamic testing setup, which can pose a problem for sensitive diagnostics.
We've also found that developing OCR that handles multiple languages is especially resource-intensive. It can require much more training data than single-language systems, which can make it difficult to implement for projects involving international teams.
Some of these OCR systems have these self-adjusting algorithms that adapt to environmental changes. This sounds great, but if the conditions change abruptly, it creates the potential for the system to misinterpret things. We need to look closely at the limits of this approach.
As these tools rely on cloud storage for historical data, we have to be aware of the risk of data breaches. Secure storage is vital, especially in situations where sensitive project details are stored and shared with teams across different locations.
Lastly, there's the inherent limitation that even the best OCR technology can struggle with certain kinds of displays. If the font is unusual, the display has low contrast, or the characters are non-standard, the OCR tool can sometimes misinterpret vital data points. It's crucial to emphasize the importance of cross-checking these readings for projects where the precision of the data matters.
We're on the cusp of some truly powerful capabilities with AI-OCR, but it's important to understand the practical considerations involved. It's not a perfect solution in all cases. By identifying the challenges and exploring potential workarounds, we can utilize these tools for the benefit of engineering disciplines and accelerate the pace of innovation.
More Posts from aitranslations.io: