AI-Powered PDF Translation now with improved handling of scanned contents, handwriting, charts, diagrams, tables and drawings. Fast, Cheap, and Accurate! (Get started for free)
Global Translation Volumes Hit 3333M Words Daily How AI Makes It Possible
Global Translation Volumes Hit 3333M Words Daily How AI Makes It Possible - OCR Technology Processes 433M Scanned Documents Per Day For Translation
Optical Character Recognition (OCR) technology is rapidly processing a massive 433 million scanned documents every day, a vital component of the translation ecosystem. This high-throughput capability is essential for handling the sheer volume of global translation work, which now reaches 3.333 billion words daily. The integration of AI is steadily improving OCR's ability to convert printed material into editable digital formats. This makes translations faster and more efficient for businesses. Notably, cloud platforms such as Microsoft Azure and Google Cloud are simplifying document translations, allowing direct translations without the need for a separate OCR step. This trend highlights the growing interconnectedness between AI and translation solutions. The evolution of this technology is fundamentally changing how companies manage multilingual content and communication in an increasingly global marketplace, paving the way for potentially more efficient but also potentially less accurate translation processes.
It's fascinating how OCR has become a critical part of the translation landscape. We're talking about 433 million scanned documents processed daily for translation—a huge number that showcases its growing importance. While OCR has come a long way from its early days, its accuracy is still a consideration. Achieving 1-2% error rates on pristine documents is impressive, but dealing with complex or poorly scanned documents remains a challenge. This highlights a potential area of research – improving OCR performance on less-than-ideal inputs.
The integration of AI and machine learning in OCR has been a major step forward. Training models on vast datasets helps them learn diverse fonts, languages, and even handwriting styles. Yet, there's always room for improvement—handling variations in handwriting across individuals or with aging documents continues to be a hurdle. It's curious to observe how AI can improve here.
One area where OCR shines is its ability to process documents in multiple languages. This opens doors for businesses to efficiently and consistently translate materials for diverse audiences, ensuring consistent messaging globally. The integration with AI translation engines further accelerates the process, which is especially beneficial for organizations needing fast turnaround times.
Of course, the sheer volume of data stored in image format – a staggering 95%— underscores the importance of OCR. Without it, we'd struggle to access and utilize a vast pool of knowledge. It's remarkable how OCR doesn't just handle text anymore. With progress in image analysis, it can interpret charts, tables, and graphs, significantly expanding its scope within the technical documentation realm. It's interesting to think about where these capabilities might lead us.
Finally, the cost-benefit aspect of OCR for translation is compelling. It automates tasks, reduces manual efforts, lowers costs, and minimizes errors associated with manual handling. This is quite attractive, particularly with organizations managing large-scale translation projects. The ability of these solutions to scale to accommodate high volumes—millions of documents daily—emphasizes their practicality and operational benefits. It is exciting to see the potential of scaling further in the future.
Global Translation Volumes Hit 3333M Words Daily How AI Makes It Possible - Machine Translation Handles 2B Words in Asian Languages Every 24 Hours
Machine translation, powered by AI, is now handling a massive 2 billion words daily across Asian languages. This substantial contribution is part of the larger global translation landscape, which sees an astounding 3.333 billion words translated each day. While this growth is remarkable, it also highlights challenges, specifically within the realm of Asian languages. Many of these languages are considered "low-resource," meaning there's a limited amount of digital data available to train AI models effectively. This scarcity of training data makes it difficult for AI to achieve the same level of accuracy and fluency as it has with more widely-spoken languages.
Researchers and developers are actively tackling this challenge, experimenting with new AI techniques and approaches to improve the quality of machine translation for languages that have fewer resources. Their goal is to develop more inclusive and capable AI translation systems. The future of multilingual communication depends heavily on this ongoing progress, with the potential to make translation faster, cheaper, and more accessible for a wider range of languages and cultures. Ultimately, the development of AI in this area could profoundly change the way people communicate across language barriers.
It's remarkable that machine translation systems are now handling 2 billion words daily across Asian languages. This volume speaks to the expanding role of these languages in global communication and highlights the ever-increasing demand for efficient linguistic tools. While exciting, this growth also presents challenges. For example, many Asian languages have unique writing systems, like Hanzi or Hangul, demanding specialized model development and adjustments for character recognition and processing.
Accuracy in Asian language machine translation has certainly improved. Some systems boast accuracy rates exceeding 80% on average, which is very encouraging. Yet, aspects like idioms, contextual nuances, and even tonal elements continue to pose challenges. These nuances are particularly important in languages like Mandarin or Cantonese, where subtle tone changes can alter a word's meaning completely. Navigating these areas effectively remains a key research direction.
The need for speedy and economical translation solutions has spurred a significant shift toward machine translation. Estimates suggest that it can reduce translation time and cost by as much as 80%. This efficiency is clearly appealing for companies seeking rapid content localization, especially in today's fast-paced business environment. However, this reliance on automation introduces another concern - the potential for increased translation errors due to a decreased human-in-the-loop.
The advent of neural machine translation (NMT) has undeniably revolutionized the field. By leveraging neural networks, these systems can capture the context of sentences and language more accurately, resulting in more natural and fluent translations. However, training these advanced models requires massive amounts of parallel text data in multiple languages. For languages with smaller datasets, especially those spoken by smaller communities, it becomes much harder to train highly performant translation engines.
Machine translation has progressed to the point where real-time translation is now feasible. This enables applications like facilitating international business meetings or offering instant translations on online platforms. But, realizing true multilingual communication that preserves nuances, context, and cultural subtleties in these dynamic settings remains a considerable challenge. The need for accurate translations and culturally aware outputs will become even more important as we continue to become more connected globally.
The integration of machine translation with OCR technologies is becoming indispensable. This partnership is crucial because a massive portion of global data – around 95%— exists in image format. Without effective OCR, we'd be severely limited in our ability to utilize this wealth of information. Furthermore, machine translation, paired with OCR, provides an ideal approach for extracting information from image-based documents that may require quick translations in a variety of applications, including healthcare and legal proceedings.
A really interesting area of study is how machine translation systems can learn from user interaction. Many systems are incorporating user feedback loops to improve future translations. Users can flag incorrect translations, and the system adjusts its algorithms accordingly. While this learning capability is incredibly valuable, it also raises concerns about data privacy and the potential for the reinforcement of biases present within the user feedback.
The massive volume of translations processed through these systems naturally creates a risk of perpetuating biases. These biases can be inherent in the training data or introduced through user feedback. It's vital that developers are conscious of this issue and implement safeguards to ensure fairness and accuracy across different languages. This issue deserves significant attention as it can inadvertently amplify existing inequalities present in our society.
Finally, it's worth noting that the performance of machine translation systems varies depending on the specific language pair. Some language pairs show better results than others, which is often linked to the availability of high-quality training data or the degree of linguistic similarity between the two languages. This disparity highlights the ongoing need for research and development to address the imbalance in machine translation performance between languages.
Global Translation Volumes Hit 3333M Words Daily How AI Makes It Possible - Neural Networks Cut Translation Costs From 20 Cents to 2 Cents Per Word
Artificial intelligence, specifically neural networks, has revolutionized the cost of translation, dramatically reducing it from a hefty 20 cents per word to a mere 2 cents. This remarkable decrease is a testament to the evolving capabilities of machine translation (MT) systems, particularly neural machine translation (NMT). The global translation landscape is booming, with a daily volume of 3.333 billion words, further emphasizing the need for these cost-effective solutions. While NMT has demonstrably improved translation quality, surpassing previous methods by nearly half, challenges remain. Developing accurate translation systems for languages with limited digital resources is a persistent hurdle, as the scarcity of training data impacts the models' proficiency. The shift towards AI-driven translation introduces a trade-off: while incredibly fast and cheap, it also presents the risk of increased errors and a potential loss of contextual nuances. This highlights a critical question for the future of translation: how can we balance the efficiency of automation with the need for accurate and nuanced translations in a world increasingly reliant on global communication?
Neural networks have dramatically lowered translation costs, bringing the price per word down from around 20 cents to just 2 cents. This significant reduction, a 90% decrease, has made multilingual communication considerably more accessible for various businesses and individuals. This trend indicates that the barriers to translation are steadily diminishing, potentially enabling greater global understanding.
Neural Machine Translation (NMT) has been crucial in tackling the complexities of language. It's improved translation quality by employing techniques that account for context within sentences. This leads to more natural-sounding translations compared to older statistical methods, which frequently resulted in awkward or unnatural output. It's fascinating how NMT can grasp the meaning behind the words, moving beyond simply replacing words in a source language with equivalent words in the target language.
While accuracy has increased in NMT, with some systems reporting over 80% accuracy for specific language pairs, challenges remain. Many less-common languages still lack the large datasets required to train these sophisticated models. This data scarcity restricts the capacity of AI to achieve comparable accuracy and fluency for all languages. One wonders how to best address this issue and develop AI systems that are truly inclusive for all languages.
One of the exciting aspects of NMT is that these systems can evolve and improve through user interaction. They're equipped with feedback loops that allow users to flag errors or suggest better translations. This capability is valuable because it allows the system to constantly learn and adapt, becoming progressively more accurate over time. However, it also raises important questions about the potential for bias within the feedback data. Is it possible for bias to be inadvertently reinforced within these systems? Further research is needed to ensure these learning processes remain fair and unbiased.
AI-powered translation isn't just about cost savings and efficiency; it's also facilitating real-time applications like video conferencing and live chat. While this is a major advancement in breaking down language barriers, the challenge of ensuring accurate and culturally nuanced translation in these dynamic settings persists. The nuances of language can be complex, and while AI can handle a great deal of the translation burden, there are situations where a deep understanding of the context and culture is essential.
The growth of global translation volumes is staggering—we're talking about 3.333 billion words translated daily. This surge in demand highlights the rising need for multilingual services. Yet, relying solely on automated systems carries a risk. Errors may slip through the cracks without immediate detection, so balancing automation with human oversight is critical to ensure high-quality translations.
The future of machine translation seems bright, with the goal of achieving high-quality translation for practically every major language in the world. However, language structures are diverse, leading to differences in performance. Some languages prove to be more challenging than others, leading to inconsistencies in accuracy and fluency. This highlights the continuous need for research and refinement to close the performance gap between languages.
The combination of OCR and machine translation creates a seamless workflow. It's particularly relevant for industries like healthcare and legal sectors where information often resides in image formats, like scanned documents. This integration efficiently bridges the gap between image and text, making information accessible for translation. It's interesting to see how this synergy accelerates workflows, enabling more timely access to crucial information across languages.
Machine learning is advancing to the point where translation systems are beginning to recognize not just individual words, but also phrases, idioms, and even some cultural references. Despite these advancements, capturing the implicit meanings, which are essential for professional settings, can still be difficult. There is a clear need to explore how to enhance the semantic understanding of AI systems to grasp the full complexity of human communication.
Considering that 95% of global data is in image format, the confluence of OCR and neural networks is paramount. Without this critical partnership, access to and translation of a vast reservoir of knowledge would be significantly hindered, affecting industries that rely on translating information captured in images. It's fascinating to imagine the vast potential that lies in unlocking this wealth of information.
Global Translation Volumes Hit 3333M Words Daily How AI Makes It Possible - AI Translation Creates 89% Time Savings Over Human Translation Methods
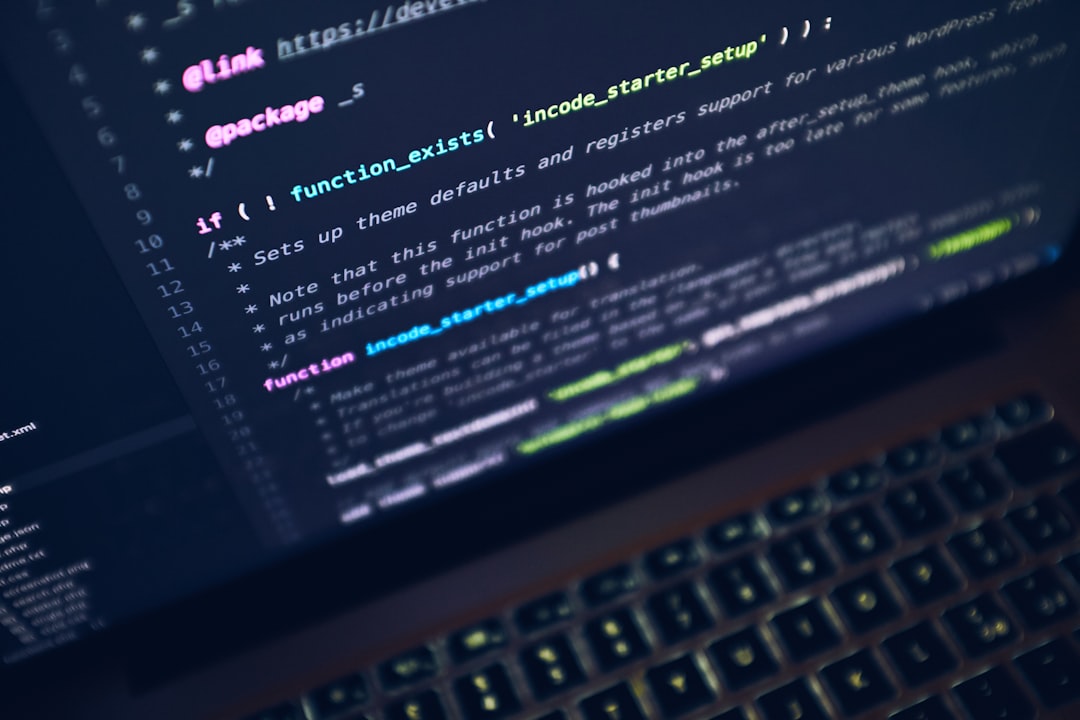
AI-powered translation is dramatically changing the landscape of translation by significantly reducing the time it takes to complete projects. It's estimated that AI can deliver results up to 89% faster than traditional methods that rely solely on human translators. With a global daily translation volume reaching a staggering 3.333 billion words, the need for faster, more efficient translation solutions has never been greater. Businesses are increasingly incorporating these AI tools into their operations, motivated by the potential to streamline processes and reduce translation costs. While AI translation tools are improving in terms of accuracy and speed, there's a growing concern that crucial nuances and cultural context might be lost in the translation process. This makes it important to think carefully about how AI can best be integrated in a way that balances speed and accuracy with cultural sensitivity. It's becoming increasingly apparent that the future of translation will likely be a collaborative effort between AI and human translators— leveraging the speed and efficiency of AI alongside the nuanced understanding and expertise of humans to provide high-quality translations. This blended approach can help ensure that translations are both accurate and culturally appropriate in an increasingly interconnected world.
AI translation systems have become remarkably efficient, boasting an impressive 89% time reduction compared to traditional human translation methods. This speed boost can be a game-changer for businesses seeking faster go-to-market strategies, especially in industries where rapid adaptation to global markets is critical. The efficiency gains are particularly relevant considering the sheer volume of translation work we see today – a staggering 3.333 billion words daily. While this rapid pace of translation is exciting, it's important to remember that this speed often comes at the cost of human oversight.
The cost implications of AI translation are just as profound. The ability to achieve translation at a mere 2 cents per word compared to the previous 20 cents is a significant shift that democratizes access to translation services. This affordability potentially opens doors for smaller businesses to engage with global markets, previously an arena often dominated by larger entities with larger translation budgets. However, with the decreased cost, there is a valid concern that accuracy may also suffer, especially in situations where subtle cultural or linguistic nuances are crucial.
Neural networks underpin much of this cost reduction and increased efficiency. These systems have the ability to learn from vast datasets, which allows them to move beyond simply substituting words. They start to understand the contextual meaning of phrases and idiomatic expressions, which has historically been a weak point for simpler statistical translation approaches. However, it's vital to note that neural networks require massive amounts of data to be effective. This requirement creates an inherent bias toward commonly spoken languages with vast amounts of digital text, which raises issues around translation quality for less-common languages.
Another fascinating development is the integration of OCR with AI translation. This combination addresses the core issue that a large majority of the world's information – roughly 95% – is currently stored in image formats. By integrating these technologies, we can unlock this vast information reservoir for translation, significantly broadening the scope of translation capabilities. This is particularly useful in areas like healthcare or legal proceedings where documents are often stored as images or scanned copies. It's quite impressive how AI is bridging the gap between image and text in these crucial applications.
The ability of AI to translate in real-time is also gaining momentum. This breakthrough can revolutionize communication in areas like international business meetings or global conferences. While the concept of real-time translation is compelling, there is a significant challenge in ensuring the translations are accurate and convey the nuances of the language, especially those linked to cultural contexts. It's a bit like a language translator having to improvise on the spot. AI's capacity to truly grasp the cultural and contextual meaning of language remains a research challenge.
As AI translation models learn from user interactions, there's also a growing concern about bias. If users consistently provide feedback that reinforces inaccurate or culturally insensitive translations, the system may inadvertently perpetuate these biases. Developers must take steps to mitigate these risks and implement checks and balances to ensure that their systems remain fair and equitable in their translation approach. The societal impact of these translations can be substantial and needs careful consideration.
The issue of data scarcity for certain languages remains a hurdle. The quality of AI translations hinges on the quantity and quality of training data. Less common languages often have insufficient data to develop robust AI translation engines. This inequality underscores a crucial challenge in AI development – ensuring that these powerful tools are inclusive and benefit all languages and communities, not just the more widely spoken ones. There's an inherent responsibility to address this imbalance and create a future where all languages have equal access to the benefits of AI translation.
The ability of AI translation to scale is another critical advantage. It can seamlessly handle the processing of millions of documents per day without needing to increase human labor significantly. This scaling capability is especially important in high-volume translation environments like legal or healthcare fields, where the speed and accuracy of translation can significantly affect outcomes. However, this scalability also highlights a key consideration: as the volume of work increases, ensuring that the quality of translations does not suffer is imperative.
Furthermore, the performance of AI translation systems can be uneven across different language pairs. Some languages demonstrate high accuracy, while others lag behind, often linked to the amount and quality of the available training data. Addressing these performance disparities requires focused research and development efforts to create systems that deliver a uniformly high standard of translation regardless of the language pair.
Finally, while AI has made significant strides in understanding the explicit meaning of words and phrases, it's important to recognize that it's still developing its understanding of the more subtle aspects of human communication. Elements like sarcasm, humor, and cultural references can be quite challenging for current AI systems to translate accurately. Continued research on semantic understanding is needed to create systems that can truly mirror the complexities of human language and communication.
In conclusion, AI translation has dramatically altered the landscape of language processing. It's delivered unparalleled speed, cost reduction, and scalability. While it holds immense potential, we must address concerns about bias, the limitations of data availability, and the complexities of human communication. These considerations are essential as we move forward in harnessing the power of AI to bridge the gap between languages and foster a more interconnected and globally understanding world.
Global Translation Volumes Hit 3333M Words Daily How AI Makes It Possible - Cloud Processing Enables Simultaneous Translation of 144 Languages
Cloud computing has enabled a significant advancement in translation, now allowing simultaneous translation across a remarkable 144 languages. This development directly addresses the substantial global need for translation, which has reached an astounding 3.333 billion words daily. AI is at the forefront of these improvements, making translation faster and more accessible. However, while the capacity to translate across so many languages is impressive, concerns remain regarding the accuracy of translations and the preservation of critical cultural and contextual nuances. The combination of cloud processing and AI in translation is fundamentally changing how we connect across languages, but its potential to introduce errors or misinterpretations requires continued vigilance to ensure the integrity of the translated message.
Cloud computing has enabled a significant leap in simultaneous translation, with systems now capable of handling 144 languages in parallel. This feat is made possible by the ability of cloud platforms to distribute processing tasks across a vast network of computers. It's impressive how this distributed approach allows translation systems to handle a massive number of requests at once, potentially exceeding the throughput of traditional systems that relied on human translators. However, one can't help but wonder if this sheer speed comes at a cost in terms of accuracy.
It's interesting to note that the majority of translation requests within these cloud-based systems are related to business communication, highlighting the critical role of translation in a globalized economy. This sheer volume of translation demands—likely surpassing billions of words daily—also underscores the need for rapid and cost-effective translation solutions that can adapt quickly to ever-changing demands. This highlights the practical need for cloud solutions capable of scaling to these requirements.
Furthermore, these AI-driven translation systems are constantly evolving. They can learn from user feedback, allowing them to adapt and refine their translations over time. This continuous learning ability is valuable for refining the accuracy of translations, particularly for industry-specific terminology and contexts. However, there's also a question of how effective this learning process can be if bias is present in user feedback data, which could potentially lead to perpetuating inaccurate or skewed results.
However, not all language pairs are treated equally. The accuracy of machine translation can be quite uneven, varying drastically depending on the specific languages involved. Some pairings, perhaps with more abundant digital resources or linguistic similarities, are able to achieve translation accuracy exceeding 90%, which is remarkably good. Others, however, struggle to reach the same levels, suggesting a disparity in translation quality across different languages. It’s quite interesting to consider the factors that lead to such differences in performance.
The development of AI translation also allows for spoken language processing, offering exciting possibilities for real-time interpretation in various scenarios, including international conferences. While this ability to break down language barriers is valuable, there's a notable challenge in capturing the nuances of language, particularly those relating to cultural context. This highlights the complexities inherent in human language and the need to consider these nuances in the design and development of these AI systems.
The integration of OCR technology into cloud-based translation systems further enhances capabilities. This allows for the translation of both digital and image-based text, including printed documents or handwritten notes. This integration is particularly important because the vast majority of the world's information is stored in image format. It's amazing to consider the amount of data that becomes accessible through OCR and how this capability fundamentally changes how information can be processed and translated.
The shift toward AI-powered translation has significantly reduced costs, driving down the price per word to a mere 2 cents. While this development makes translation more accessible, it raises concerns about potential trade-offs in the accuracy and quality of the translation. This issue deserves careful consideration.
However, there's a crucial area where current AI translation systems often fall short: cultural context. Human language is filled with subtleties like idioms, slang, and culturally specific references. AI struggles to accurately interpret and translate these aspects, which can lead to misinterpretations or even offensive translations. Overcoming this limitation is essential for the continued advancement of AI translation, as increasingly global communication necessitates culturally sensitive translations.
It's important to remember that AI translation models, particularly those built using machine learning, can inadvertently pick up on biases present in the data they were trained on. This can lead to biases in the translations themselves, resulting in inaccurate or potentially harmful interpretations. It’s a topic that demands serious attention from researchers to understand and mitigate potential harm.
The cloud-based nature of these translation systems allows businesses to easily scale their translation efforts. This ability is a crucial factor for organizations in industries like marketing and legal services where the efficiency and speed of translation can significantly impact operations. This scalability highlights the potential of AI-powered translation for handling large-scale translation tasks that were once unimaginable.
The ability of AI to make translation more accessible and affordable is undoubtedly a major advancement. However, it's crucial to address the challenges and limitations that still exist to ensure that AI translation tools continue to develop responsibly and ethically. It’s clear that this field remains a dynamic area of research, requiring a constant and critical eye toward technological advancements and their potential implications.
Global Translation Volumes Hit 3333M Words Daily How AI Makes It Possible - Translation Memory Banks Store 890M Previously Translated Segments
Translation Memory (TM) systems now store a vast repository of 890 million previously translated segments, which is transforming how translation projects are managed. These banks of translated phrases and sentences offer a powerful tool for ensuring consistency across translations and promoting smoother collaboration among translators. By reusing existing translations, TM systems help eliminate redundant work and significantly reduce costs, a critical factor in an era where daily global translation volume reaches a staggering 3.333 billion words. The synergy between TM and AI-powered translation solutions is particularly notable, further enhancing efficiency and accelerating the translation process. Nevertheless, the increasing reliance on automation introduces challenges. It's crucial to ensure the ongoing quality of translation, especially in capturing the subtle nuances of various languages and considering their cultural contexts. The ongoing development of TM technologies, alongside AI and machine translation, presents a fascinating blend of opportunity and concern, underscoring the continued importance of human expertise and oversight in maintaining accurate and meaningful communication across languages and cultures.
Translation memory banks, holding a massive 890 million previously translated segments, are essentially a vast library of linguistic knowledge. This extensive repository plays a key role in speeding up the translation process. By recalling past translations, these systems ensure consistency and efficiency across projects. It's fascinating to think of how this 'memory' function can significantly impact translation workflows.
The ability to reuse previously translated pieces, or segments, is where these banks shine. This reuse not only cuts down on time— potentially halving project durations, which is quite advantageous in today's fast-paced global economy— but also reduces costs. It's curious to see how this seemingly simple concept can deliver such tangible benefits, especially in industries with stringent deadlines.
Moreover, translation memory helps to improve the quality of translations. When a bank contains validated translations, it minimizes the potential for human errors that can creep in during manual processes. It's like having a constant pool of reliable references, making it less likely to introduce inconsistencies or inaccurate translations. The size and scope of these banks become increasingly important over time, strengthening the accuracy and reliability of future translations.
Modern AI-powered systems are even taking on the task of populating these memory banks. By learning from ongoing translation projects, these systems can automatically add new entries. It's an example of a self-learning system that constantly evolves and expands the knowledge base, improving quality and consistency in a continuously feedback loop.
Perhaps most strikingly, translation memory is particularly useful in multilingual projects. Maintaining consistent terminology across different languages is crucial in many fields, particularly in highly regulated areas like law or medicine. It's intriguing to consider how these repositories enable a smoother and more accurate translation experience for specialized fields.
Yet, the ever-increasing reliance on translation memory does raise concerns. As AI and these memory banks improve, one has to wonder about the future role of human translators. While AI can be highly efficient, it also lacks the nuanced understanding that humans bring to interpretation. It's a critical area where further investigation is necessary to ensure the human element remains an integral part of the process, especially when cultural or contextual understanding is crucial.
Integrating translation memory with other emerging technologies like real-time AI systems offers tremendous opportunities to make translations even more efficient. Organizations can manage massive daily word counts at a fraction of the previous cost. This aspect is particularly attractive for smaller businesses venturing into the global marketplace, where cost-effectiveness can be a determining factor.
However, it's important to acknowledge that translation memory can be a breeding ground for biases. If the original translations stored within the banks are inaccurate or contain specific cultural biases, those errors will likely be reproduced in subsequent translations. This issue underscores the critical need to carefully manage the data entering these repositories.
The quality of these memory banks is also dependent on user interaction. Translators often provide feedback that feeds back into the system, making it 'smarter' over time. While this dynamic feedback loop can enhance accuracy, it can also introduce biases if the feedback itself is inaccurate or prejudiced. It's fascinating how the constant interaction with human translators shapes the nature and quality of these memory banks.
Finally, there are also practical challenges in seamlessly integrating translation memory with other translation technologies like OCR or machine translation. For these systems to work harmoniously, they must share a common data format and be compatible. It's a complex engineering problem that requires creative solutions to ensure smooth interoperability. This underlines how important proper system design and integration become as the landscape of translation continues to evolve.
AI-Powered PDF Translation now with improved handling of scanned contents, handwriting, charts, diagrams, tables and drawings. Fast, Cheap, and Accurate! (Get started for free)
More Posts from aitranslations.io: