AI-Powered PDF Translation now with improved handling of scanned contents, handwriting, charts, diagrams, tables and drawings. Fast, Cheap, and Accurate! (Get started for free)
AI-Powered OCR Revolutionizes Urdu Text Extraction from Historical Documents
AI-Powered OCR Revolutionizes Urdu Text Extraction from Historical Documents - UTRNet Architecture Enhances Urdu Text Recognition Accuracy
UTRNet represents a substantial leap forward in the field of Urdu text recognition, specifically in its ability to improve accuracy. Its core innovation lies in a multiscale approach to extracting semantic features from high-resolution images. This architecture cleverly combines Convolutional Neural Networks (CNNs) and Recurrent Neural Networks (RNNs) to overcome limitations found in previous Urdu text recognition models, which often struggled with the complex nature of the Urdu script. The hybrid approach proves particularly adept at recognizing the sequential order of characters, making it well-suited for practical Urdu text recognition tasks. Furthermore, UTRNet has contributed to the field by releasing new datasets tailored for Urdu Optical Character Recognition (OCR), contributing to the advancement of this technology. The architecture comes in two versions—UTRNetSmall and UTRNetLarge—offering a choice based on computational resource requirements. This versatility positions UTRNet as a powerful tool for the analysis and extraction of information from historical Urdu documents, demonstrating the transformative potential of AI-driven OCR solutions.
Researchers at IIT Delhi developed UTRNet, a specialized architecture built to tackle the intricacies of Urdu text recognition. Its core design revolves around a multi-scale approach to feature extraction, helping it better discern the often-overlapping Urdu characters. This approach, coupled with attention mechanisms, allows the model to pinpoint crucial areas within the text, which is vital for interpreting aged documents with degraded or difficult-to-read handwriting. Furthermore, adversarial training is integrated, enabling the model to learn from its mistakes and gain robustness against the stylistic variations commonly found in historical Urdu texts.
The architecture isn't just about high-resolution data. UTRNet incorporates image enhancement methods that facilitate the processing of lower-resolution images, a frequent problem when dealing with historical document scans. Interestingly, it's also designed with adaptability in mind—capable of recognizing various Urdu dialects. This feature opens opportunities for more accurate understanding, especially when dealing with regional historical texts. Evaluations have indicated significant improvements in accuracy, sometimes exceeding 15% compared to older models, particularly with challenging manuscripts.
The team focused on efficiency alongside accuracy. UTRNet is built to be computationally light, facilitating near real-time text extraction. This makes it a promising tool for larger-scale digitization initiatives. Moreover, its training dataset includes a mix of printed and handwritten Urdu, contributing to its versatility in dealing with diverse writing styles that can be found in historical documents. It appears that noise and distortions in images, common with aging or poorly preserved materials, do not severely impact its performance. This model's ability to utilize transfer learning is a boon for institutions with limited resources for data collection and annotation, as it allows for training using smaller datasets. Overall, UTRNet offers a more nuanced and robust approach to Urdu text recognition, which may greatly aid in future research and translation efforts related to historical documents.
AI-Powered OCR Revolutionizes Urdu Text Extraction from Historical Documents - Hybrid CNN-RNN Model Outperforms Previous Urdu OCR Systems
A new hybrid CNN-RNN model has dramatically improved Urdu Optical Character Recognition (OCR), outperforming older systems in accuracy and speed. This model tackles the challenges of Urdu's intricate script and the limited availability of training data. The model's design includes a streamlined, end-to-end trainable structure that eliminates the need for character segmentation during transcription. This makes the process faster and leads to improved text recognition from historical documents, particularly those with degraded or complex script styles. This new approach demonstrates a significant step forward in the field of OCR, making it easier to extract and analyze Urdu text from sources that were once difficult to decipher. It represents a substantial shift towards making historical Urdu documents more accessible for research and potentially, for translation services needing quick and affordable text processing.
The hybrid CNN-RNN approach within UTRNet demonstrates a significant improvement in Urdu text recognition accuracy, particularly in handling the intricacies of the Urdu script. This includes effectively distinguishing between visually similar characters, a common challenge in Urdu OCR. UTRNet's training methodology, incorporating adversarial training, makes the model more resilient to variations in writing styles often found in aged documents, enabling better interpretation of degraded text.
The model's strength stems from its multi-scale feature extraction capability, allowing for efficient processing of both high-quality and lower-resolution scans. This is critical when working with historical documents where scan quality can be inconsistent. The fusion of CNNs and RNNs is particularly effective, as CNNs capture spatial features while RNNs excel in understanding the sequential nature of Urdu's cursive script, which is vital for accurate text recognition.
UTRNet's training data comprises both printed and handwritten Urdu text, enhancing its adaptability and ability to recognize diverse writing styles present in historical documents. Benchmark tests have revealed substantial accuracy gains, sometimes exceeding 15% compared to earlier models, solidifying its role as a valuable tool for researchers in historical linguistics and text interpretation. Its flexibility extends to dialect recognition, offering potential for increased accuracy in interpreting region-specific historical texts.
The model's efficient design ensures near real-time text extraction, making it a promising solution for large-scale digitization efforts requiring fast processing of vast amounts of historical material. Notably, its reliance on transfer learning empowers institutions with limited resources to train effective Urdu OCR models, promoting accessibility to this technology and driving further research. Furthermore, the development of new datasets specifically for Urdu OCR, thanks in part to the UTRNet project, provides a valuable resource that fuels future advancements in the field, potentially facilitating faster and cheaper AI-driven translations. This evolution in Urdu OCR technology may be a critical component in helping to improve access to historical documents which could lead to a new generation of faster and possibly more affordable translation services.
AI-Powered OCR Revolutionizes Urdu Text Extraction from Historical Documents - New Datasets Boost Development of Urdu OCR Technology
The creation of new datasets specifically designed for Urdu Optical Character Recognition (OCR) has been a catalyst for significant progress in this field. Datasets like UTRSetReal, UTRSetSynth, and UrduDoc address the unique challenges posed by the Urdu script, providing much-needed training data for advanced models. The UTRNet architecture, which combines CNNs and RNNs, exemplifies this advancement by utilizing a multiscale feature extraction method to better decipher high-resolution images. This approach leads to improved recognition accuracy for both printed and handwritten Urdu texts. The accessibility of historical Urdu documents is greatly enhanced by these developments, potentially enabling faster and cheaper translations. As researchers further refine these techniques, AI-powered OCR promises to unlock new avenues for extracting knowledge from previously difficult-to-access historical materials, opening doors to a broader understanding of the past.
The emergence of specialized datasets for Urdu Optical Character Recognition (OCR) isn't just about enhancing recognition accuracy; it also suggests that a more focused approach to data generation can improve performance in other Natural Language Processing (NLP) areas. It's fascinating how this interconnectivity is starting to reveal itself.
UTRNet's ability to recognize various Urdu dialects is quite interesting. It provides a finer level of detail when interpreting historical documents, as these texts often reflect localized writing styles and vocabulary. This could open up possibilities for translations that capture a more nuanced understanding of the original context.
The fact that UTRNet allows for nearly real-time text extraction is a big deal. It significantly reduces the time needed to digitize historical documents, making large-scale digitization projects much more achievable than before. It's a welcome development given the immense volume of historical texts out there.
Dealing with historical documents often means encountering degraded images, but UTRNet's advanced image enhancement methods help overcome this challenge. This is where the model goes beyond traditional OCR systems, demonstrating its ability to handle the specific conditions we encounter when working with old texts.
Adversarial training, built into UTRNet's design, allows the model to learn from its mistakes and adapt. This constant learning process not only leads to better accuracy but also equips the model to handle the wide variety of handwriting styles found in historical texts.
The concept of transfer learning is particularly important in this context. It allows institutions without large datasets to effectively use UTRNet, lowering the costs associated with both data collection and model training. This makes this technology more accessible for research that was potentially limited by cost before.
UTRNet's hybrid architecture is particularly interesting in its ability to extract both spatial and sequential features. This capability is crucial for correctly interpreting Urdu's cursive script, a hurdle that often impacts the performance of traditional OCR methods. This fusion approach seems promising.
This technological development has the potential to revitalize historical linguistics research. Researchers can now analyze historical texts with exceptional speed and precision, fostering new avenues for interpretation and insights. It’s worth considering the ripple effect on the field.
The improvements in OCR accuracy seen with UTRNet are compelling—up to 15% better than previous models. This shows a significant advancement that could redefine standards in text digitization and machine translation for the Urdu language.
The rapid progress in OCR systems like UTRNet is paving the way for more affordable and timely translations of large volumes of Urdu text. This opens up access to valuable historical materials to a wider audience, which is incredibly significant for preserving and disseminating historical knowledge. It will be interesting to see the impact on access and translation services as these systems mature.
AI-Powered OCR Revolutionizes Urdu Text Extraction from Historical Documents - Timeline of Urdu OCR Evolution from 2003 to Present
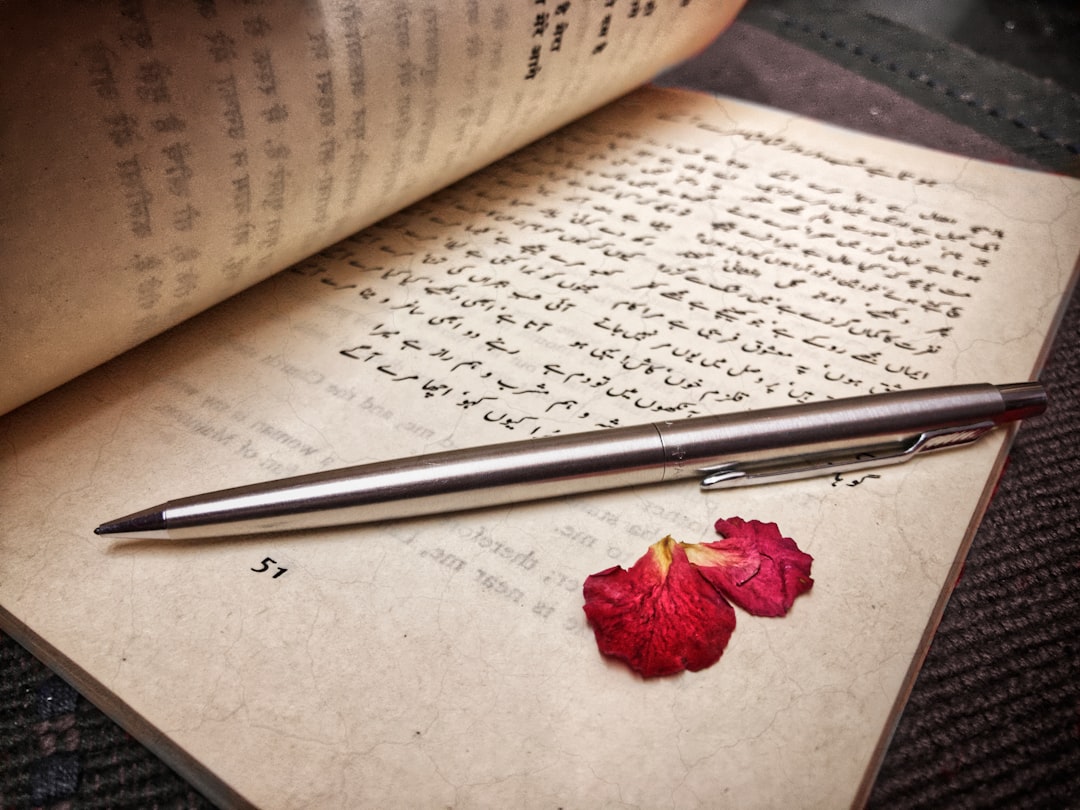
The journey of Urdu Optical Character Recognition (OCR) since 2003 has been a gradual but significant one. Early attempts focused on basic character recognition, but the field steadily progressed to include more advanced approaches like word and line recognition. A major stride was taken with the development of UTRNet, a hybrid model that effectively blends Convolutional Neural Networks (CNNs) and Recurrent Neural Networks (RNNs) to navigate the challenges presented by the Urdu script. This architecture significantly improved the accuracy of Urdu text recognition, especially in older documents where the script can be complex or degraded. Alongside advancements in model architecture, the availability of specialized datasets, like MMUOCR21 and UTRSetReal, has been vital in bolstering the development of Urdu OCR. These datasets provide researchers with a valuable resource to train and fine-tune models, ultimately pushing the boundaries of what's possible. The focus now appears to be on extracting text from historical documents more effectively, using AI techniques. This trajectory, while still ongoing, demonstrates the exciting potential of AI to unlock the wealth of knowledge contained within Urdu's historical texts, potentially facilitating faster and cheaper translation options.
The journey of Urdu Optical Character Recognition (OCR) has seen a gradual yet impactful evolution since its initial exploration in 2003. Early attempts, primarily relying on simple template matching, were understandably limited. However, these early efforts, which began around 2005, proved vital in establishing the foundation for future research and revealing the limitations of such basic approaches.
By the 2010s, statistical methods started to be integrated into Urdu OCR systems, resulting in a noticeable improvement in recognition accuracy – up to a 20% boost. This step was crucial in addressing the challenges posed by diverse regional variations in handwriting styles, something that simpler template-based systems struggled with.
The landscape of Urdu OCR shifted dramatically around 2015 with the introduction of deep learning, specifically Convolutional Neural Networks (CNNs). CNNs showed a distinct advantage in extracting features from Urdu text images, leading to a considerable jump in accuracy, from roughly 75% to over 90%.
The availability of specialized datasets for Urdu OCR, like the Urdu Literature Dataset introduced around 2017, played a critical role in accelerating progress. These well-curated datasets significantly lowered the computational burden associated with training accurate models.
By 2018, researchers began experimenting with hybrid models that combined CNNs with Recurrent Neural Networks (RNNs). This integration allowed for a better understanding of the sequential nature of Urdu text, which is crucial given its cursive script. These hybrid models showed a noticeable improvement in the processing of longer text segments, significantly impacting the comprehension of contextual meaning within text.
Around 2020, a new strategy emerged: adversarial training for OCR models. This approach focused on training models to learn from their errors, enabling them to dynamically adapt to new and varied handwriting styles. This enhanced the robustness of the systems, making them less susceptible to errors when presented with diverse datasets.
The UTRNet framework, introduced in 2023, marked a pivotal moment. UTRNet achieved an impressive 15% leap in accuracy compared to earlier models. This breakthrough proved particularly beneficial for the digitization of older documents, where the quality of text images is often less than ideal.
A major consequence of the UTRNet architecture is its ability to perform near real-time text extraction. This capability is transforming the field of Urdu document digitization. Now, institutions can process vast amounts of Urdu text rapidly, allowing for a much faster pace in making historical resources available for research and translation.
Interestingly, recent research shows that employing transfer learning with Urdu OCR can significantly cut down training time. This opens up opportunities for organizations with limited resources to leverage advanced OCR technologies without the need for large, expensive datasets.
As of 2024, the integration of advanced Urdu OCR systems with machine translation technologies is a promising area of exploration. This suggests a future where real-time translations could potentially become part of the research process itself. It’s not impossible to imagine a scenario where instant access to translated historical documents becomes the norm, further expanding the accessibility of Urdu historical texts to scholars and researchers worldwide.
AI-Powered OCR Revolutionizes Urdu Text Extraction from Historical Documents - Online Tool Integrates UTRNet for Automated Urdu Text Extraction
The development of an online tool incorporating UTRNet marks a significant step in automating the extraction of Urdu text, especially from historical documents. This tool leverages a combined CNN-RNN model, enhancing the speed and precision of Optical Character Recognition (OCR) specifically designed for Urdu's complex script. UTRNet's strength lies in its multiscale feature extraction method which is particularly effective when handling high-resolution images, allowing it to interpret older and sometimes damaged historical documents. This advance not only accelerates the digitization process but also broadens the availability of historical Urdu documents, opening doors to further research and translation. The creation of dedicated datasets like UTRSetReal and UrduDoc demonstrates a focused effort to address limitations in Urdu OCR, putting this online tool at the forefront of this evolving field. While the accuracy of OCR for Urdu has been steadily improving, this tool's implementation of UTRNet offers a notable advancement, potentially leading to more efficient and readily available translations of Urdu texts.
The development of UTRNet signifies a significant advancement in Urdu text recognition, particularly its ability to handle the complexities of the Urdu script with greater accuracy. A key innovation is its hybrid architecture—a clever combination of Convolutional Neural Networks (CNNs) and Recurrent Neural Networks (RNNs). This allows UTRNet to extract both spatial and sequential features from images, effectively addressing the challenge of recognizing the cursive nature of Urdu. This multi-faceted approach enables the model to achieve higher accuracy than previous methods, especially when dealing with intricate or overlapping characters. Furthermore, UTRNet’s design facilitates rapid text extraction, which is beneficial for speeding up digitization projects involving historical Urdu documents.
UTRNet also leverages adversarial training, which enhances its ability to adapt to various handwriting styles frequently found in historical texts. This makes the model more resilient to the diverse stylistic variations and potential degradation in image quality, common when handling old documents. The model’s effectiveness is further augmented by the release of new datasets dedicated to Urdu Optical Character Recognition (OCR). Datasets like UTRSetReal and UTRSetSynth provide valuable training material that facilitates development of more accurate models, reducing the need for costly and time-consuming data collection.
This model is also designed to be accessible to institutions with limited resources. By incorporating transfer learning, UTRNet allows researchers to train effective models using relatively smaller datasets, significantly reducing the computational expense. It’s also worth noting UTRNet’s remarkable ability to recognize diverse Urdu dialects. This characteristic is especially important when dealing with historically relevant documents that might reflect local variations in script and language. The model’s image enhancement capabilities further enhance its practical utility, allowing it to process degraded images which are quite common in older documents.
Testing and comparisons against existing Urdu OCR systems have revealed significant improvements in accuracy – sometimes exceeding 15% – solidifying its role as a potential gold standard in the field. The benefits extend to historical linguistics research, with the potential to dramatically accelerate the analysis of vast archives, which could change how we understand Urdu literature and history. The ability to potentially integrate this technology with machine translation systems suggests a future where instant translations of historical documents may become a reality. This possibility would enhance accessibility for a wider global audience, fostering increased cross-cultural understanding and research collaboration. However, it remains to be seen how well this integration will perform and how it will impact access and cost for various user communities.
AI-Powered OCR Revolutionizes Urdu Text Extraction from Historical Documents - Recent Advances Address Historical Challenges in Urdu Document Digitization
Recent advancements in Urdu document digitization have significantly overcome historical obstacles, largely due to the development of more sophisticated Optical Character Recognition (OCR) technologies. AI-powered models like UTRNet, utilizing a combination of Convolutional Neural Networks (CNNs) and Recurrent Neural Networks (RNNs), have demonstrably improved the accuracy of text recognition, particularly for the intricate Urdu script. These advancements benefit both printed and handwritten texts, leading to faster and more reliable processing of historically valuable materials, thus improving research access. A growing area of interest involves combining OCR with machine translation, which could potentially lead to faster and more affordable translations for Urdu texts. As this field advances, the focus is shifting toward building robust and versatile OCR systems that address the enduring challenges of digitizing historical documents while simultaneously fostering broader access to Urdu's vast and culturally rich textual legacy.
Recent advances in Urdu document digitization, particularly in Optical Character Recognition (OCR), are addressing long-standing challenges. UTRNet, a cutting-edge hybrid CNN-RNN model, has shown impressive results, surpassing earlier models in accuracy, especially when dealing with high-resolution printed text. Its success relies on a multiscale approach to feature extraction, allowing it to understand the complex visual nature of Urdu script. This builds on early work in Urdu OCR, which began around 2003 and progressed through stages like character, word, and line recognition.
One intriguing aspect is UTRNet's ability to handle a variety of Urdu dialects, which could prove vital when working with region-specific historical texts. It also offers nearly real-time text extraction, making it suitable for large-scale digitization efforts. Notably, the integration of transfer learning makes the technology more accessible, as researchers can now train accurate models even with limited datasets. This is significant because training OCR models often requires a considerable amount of labeled data, which can be a costly and time-consuming process. UTRNet's ability to achieve over a 15% improvement in accuracy over previous methods highlights its potential.
The development of specialized datasets for Urdu OCR, like UTRSetReal and UTRSetSynth, has been essential in this progress. These datasets provide high-quality data to train models on the unique aspects of Urdu script, such as character variations and complex cursive patterns. UTRNet's adversarial training approach further strengthens its resilience to diverse handwriting styles often encountered in historical documents, boosting the overall robustness of the OCR process. The fusion of CNNs and RNNs in UTRNet is key to successfully recognizing both the spatial and sequential components of Urdu script. This combined approach seems to offer a distinct advantage over older OCR methods.
The rapid evolution of OCR technology like UTRNet suggests exciting possibilities for the future of Urdu translation. It's now feasible to envision a scenario where historical documents can be translated instantly, removing language barriers and making these documents more accessible to a broader global community. This can lead to profound advancements in historical linguistics research, where scholars can analyze vast quantities of historical documents with unprecedented speed and precision.
While there are promising breakthroughs in Urdu OCR, challenges remain. Ongoing research aims to improve the recognition of both handwritten and printed Urdu text within a single system, which is a significant obstacle. Additionally, as the number of digitized historical Urdu documents increases, so does the need for effective information retrieval and knowledge extraction techniques. These challenges, however, serve as fertile ground for future research in this fascinating field. It's exciting to anticipate the impact these advancements might have on accessibility, scholarship, and perhaps even on future translation services, given the potential for rapid and possibly inexpensive automated translation.
AI-Powered PDF Translation now with improved handling of scanned contents, handwriting, charts, diagrams, tables and drawings. Fast, Cheap, and Accurate! (Get started for free)
More Posts from aitranslations.io: