AI-Powered PDF Translation now with improved handling of scanned contents, handwriting, charts, diagrams, tables and drawings. Fast, Cheap, and Accurate! (Get started for free)
Top 7 AI Translation Challenges When Working with Asian Languages From Japanese OCR to Bengali Script Recognition
Top 7 AI Translation Challenges When Working with Asian Languages From Japanese OCR to Bengali Script Recognition - Japanese OCR Struggles with 8,000 Kanji Characters in Digital Documents
The sheer number of kanji characters—roughly 8,000—poses a significant challenge for Japanese Optical Character Recognition (OCR) systems. Digital documents often utilize this vast character set, creating a complex landscape for accurate recognition. AI models must employ advanced methods, like Convolutional Neural Networks, to effectively decipher these intricate symbols and their diverse patterns. Although various OCR tools and services, including ABBYY's FineReader, have emerged to address the need for Japanese text extraction, achieving consistently high accuracy remains a challenge, especially with handwritten kanji. Breaking down kanji text into individual characters is a vital initial step in the OCR process, yet it emphasizes the ongoing difficulties inherent in handling non-Latin scripts like Japanese. The persistent hurdles underscore the need for ongoing advancements in OCR technology to truly adapt to the subtleties and nuances of Japanese writing.
The intricate nature of the Japanese writing system poses significant hurdles for Optical Character Recognition (OCR) technologies. The sheer number of kanji characters—around 8,000 commonly used in digital documents—presents a substantial challenge for algorithms trained to recognize patterns. Each character can have numerous meanings and pronunciations, which adds a layer of complexity when the surrounding context isn't readily available for the software to deduce the correct interpretation. This issue of ambiguity compounds the difficulties already inherent in dealing with a non-phonetic writing system. OCR systems first need to correctly decipher individual kanji before translating their meanings, leading to increased processing time compared to more straightforward alphabets.
Furthermore, the historical evolution and cultural influence on kanji, with its roots in Chinese characters, has produced diverse variants and regional differences. This poses a considerable obstacle for standard OCR algorithms which often struggle to accommodate this variability. Training machine learning models for Japanese OCR is further complicated by limited datasets, which may not fully encompass the wide range of kanji variations found in everyday documents. Consequently, achieving high-quality OCR in Japanese often demands significant computational resources, putting a strain on cost-conscious users or smaller businesses.
Handwritten kanji presents another challenge for most systems. Because of the considerable variance from standardized forms, the accuracy of character recognition significantly declines in comparison to printed documents. While AI advancements and neural network approaches have helped enhance performance, subtleties in language and cultural context continue to present major obstacles, requiring ongoing refinement and research.
While OCR can quickly digitize written kanji, the subsequent translation of those characters into meaningful sentences frequently necessitates human intervention, slowing down the overall process. A persisting issue across many solutions is the occurrence of errors in kanji recognition, which can lead to major misunderstandings. This is particularly true for specialized or intricate documents where precise interpretation is crucial. The distortion of intended meaning from such misinterpretations is a consistent reminder of the ongoing need for improved OCR algorithms.
Top 7 AI Translation Challenges When Working with Asian Languages From Japanese OCR to Bengali Script Recognition - Bengali Script Recognition Faces Layout Issues with Floating Diacritical Marks
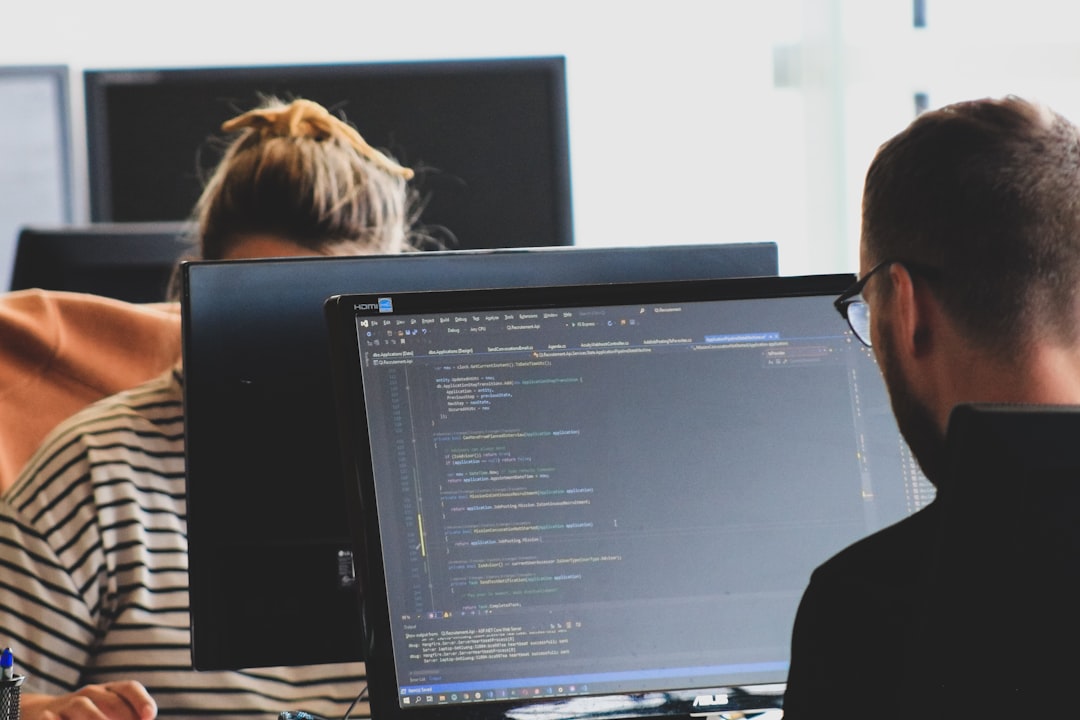
Recognizing Bengali script presents a unique challenge for AI due to the way diacritical marks are placed. These marks, which modify the sound or meaning of a character, are often not directly attached to the base letter, making it difficult for OCR systems to accurately interpret them within the overall document layout. The Bengali script's complexity, including its many unique characters and the strict rules for placing diacritical marks, strains current OCR technologies.
While some advancements have been made in this area, the field still lacks the resources and tailored methodologies needed for reliable Bengali OCR. A major issue is a scarcity of specialized data sets and training materials suited for the Bengali language. This hurdle reveals the gap that exists between current OCR technology's capabilities and the intricate nature of Bengali script. To improve translation solutions for Bengali and similar scripts, continued development and refinement are required to enhance OCR's accuracy and adaptability. As demand for accurate and fast AI translation services increases, tackling these challenges across diverse languages becomes increasingly important.
Bengali script presents a unique challenge for AI-powered Optical Character Recognition (OCR) systems due to its intricate nature. With over 150 distinct characters, the script incorporates a significant number of floating diacritical marks. These marks, which modify the pronunciation and meaning of a base character, can appear above, below, or even beside the character itself. This variability in placement leads to complications during character segmentation, which is a critical step in OCR. Often, these diacritical marks are either misrecognized or completely missed, leading to errors in the final output.
The complexity of Bengali is further compounded by the inherent similarities between base characters and their associated diacritics. This similarity makes it difficult for traditional OCR algorithms to differentiate between them accurately. Research in the field has shown that standard methods struggle with the Bengali script, with accuracy rates in some instances dipping as low as 60% when dealing with unformatted or untrained documents. This reinforces the idea that developing robust and efficient OCR solutions for Bengali requires careful attention to the language's unique features.
The cost of training sophisticated AI models to recognize Bengali script can be a barrier for smaller businesses. High-performance cloud services, essential for effective model training, often carry hefty price tags. This financial constraint can limit access to advanced translation tools, particularly for those working with less widely used languages. In addition to the cost factor, the existence of numerous regional fonts and writing styles within Bengali makes the creation of comprehensive and standardized training datasets quite difficult. Consequently, the consistency and reliability of OCR outputs across different documents can vary, indicating a need for adaptive and flexible OCR systems.
While recent advancements, such as incorporating convolutional neural networks, have shown promise in enhancing the accuracy of Bengali OCR, significant challenges remain. The performance of these models can still vary greatly depending on the quality and type of text input, highlighting the ongoing need for more in-depth research and development. Translation tasks become even more complicated when dealing with Bengali, as the nuances of the language—especially those conveyed through the use of diacritical marks—can affect the meaning of an entire phrase. This nuance necessitates extensive human review, potentially delaying the translation process.
Furthermore, there's a current lack of smooth integration between OCR and translation software. This disconnect can lead to inefficient workflows and delays, especially when dealing with texts containing idioms or culturally specific references. Interestingly, OCR systems often manage to process numerals and punctuation marks more effectively than the Bengali script itself. This creates a somewhat paradoxical situation where numbers are correctly recognized, while text remains problematic. This discrepancy underlines the unique difficulties associated with the Bengali language and highlights the need for a more nuanced approach to AI-based solutions.
Finally, although advancements in bilingual OCR models have been observed for more widely spoken languages, the resources devoted to Bengali script recognition remain relatively scarce. This disparity reflects broader trends in AI development, where less widely-used languages often receive less attention. It's crucial to acknowledge this gap and work toward allocating more resources and support to the development of reliable Bengali OCR systems, benefiting the roughly 230 million people who call Bengali their native tongue.
Top 7 AI Translation Challenges When Working with Asian Languages From Japanese OCR to Bengali Script Recognition - Chinese Word Segmentation Creates Accuracy Problems in Machine Translation
When translating Chinese into other languages, accurately segmenting the text into individual words is a significant hurdle for machine translation systems. This process, known as Chinese word segmentation, directly influences the quality and speed of the translation. Different approaches to segmentation exist, with methods that rely on existing dictionaries typically showing better results than those that treat each character as a standalone word. However, optimizing word segmentation alone isn't a silver bullet for enhancing translation accuracy. Other factors influence the outcome, highlighting the intricate relationship between segmentation and translation quality.
The challenges are exacerbated by the complex nature of the Chinese language itself. Effectively translating requires a solid understanding of how word segmentation impacts the translation process. While ongoing research has focused on improving Chinese-to-English translations, a clear understanding of the specific role played by word segmentation remains elusive. This gap in knowledge emphasizes the need for more in-depth studies, focusing on developing stronger linguistic resources and strategies specifically designed to tackle this complex issue. Without a better grasp of this vital initial stage of the translation process, achieving optimal translation outcomes for the Chinese language will continue to be a challenge.
Chinese word segmentation is crucial for accurate machine translation because, unlike many other languages, Chinese doesn't rely on spaces to separate words. This means that understanding the intended meaning often relies heavily on context, making segmentation a complex challenge. Getting this segmentation wrong can lead to translations that are fundamentally flawed, as the same characters can mean very different things depending on how they're grouped.
Different approaches to word segmentation heavily impact how well Chinese can be translated into other languages, particularly English. However, the precise mechanisms behind these effects aren't fully understood. Interestingly, techniques based on existing dictionaries appear to work better than simply treating each character as a separate word.
While optimizing segmentation can be helpful, it isn't the sole factor determining translation quality. It's just one piece of the puzzle. Having access to robust linguistic resources, like collections of segmented text and dictionaries, is extremely helpful for understanding how segmentation affects translation. Some studies have shown that tweaking dictionaries specifically for machine translation tasks can boost a segmenter's effectiveness.
Word segmentation is often the starting point for translating languages like Chinese and Vietnamese into English. Current segmentation systems are generally categorized as either linguistically or statistically focused. However, these approaches might not fully grasp the practical needs of a language like Chinese, which often focuses on the real-world meaning conveyed in specific situations.
There's been a lot of research into improving Chinese-to-English translation, but the specific impact of word segmentation is still not clearly documented. An effective segmentation method is critical for improving machine translation, especially for methods that work with phrases. This all highlights how critical this early processing stage is for successful translation. It's a bit like having a good foundation for a building – if the foundation is flawed, the building itself will be unstable. And, when it comes to translating languages with complex structures and meanings like Chinese, a solid foundation of segmentation is critical for a strong translation.
Top 7 AI Translation Challenges When Working with Asian Languages From Japanese OCR to Bengali Script Recognition - Korean Honorifics and Social Context Confuse Current AI Models
AI translation models are currently struggling to accurately handle Korean honorifics due to their complex relationship with social context. Korean uses honorifics extensively to convey politeness and respect, particularly in professional settings. However, many current AI translation models, specifically neural machine translation systems, overlook these important nuances. These systems often fail to recognize the social context embedded within a conversation, hindering their ability to generate appropriate honorific language.
Researchers have begun exploring solutions like context-aware NMT and honorific fusion training to bridge this gap. These techniques aim to improve AI's ability to generate the correct honorific forms by focusing on the relationships between speakers within a conversation. While there are promising developments in AI, applying these advancements to Korean remains difficult. The specific social structures that inform Korean language are hard for current AI to understand and replicate. This issue mirrors the wider difficulties AI has handling cultural elements across Asian languages in general, which is likely to limit improvements unless further advanced. For AI translation in Korean to be truly effective, future models must incorporate a deeper understanding of the social cues that govern its use, ensuring more accurate and culturally sensitive translations.
Korean language, with its intricate system of honorifics, poses a significant challenge for current AI translation models. These honorifics, crucial for conveying social hierarchy and politeness, often go unnoticed by AI, which are typically trained on more simplistic language datasets. This lack of understanding of social context in Korean leads to inaccuracies in the translated output. For instance, failing to generate or correctly interpret honorifics can cause the AI to produce translations that appear either overly formal or inappropriately casual for the given situation. This problem is particularly acute in professional contexts, where using the wrong honorific can lead to misunderstandings or even offense.
One approach being explored to address this issue is context-aware neural machine translation (NMT), which attempts to understand the relationship between speakers within a conversation and generate the appropriate honorifics based on that information. This approach requires training the models on vast datasets labeled with relevant social cues. Additionally, techniques like data labeling and reinforcement learning are being investigated to help AI better understand and utilize honorifics within different conversational styles.
However, even with these advances, the complexities of Korean honorifics remain daunting. They are deeply rooted in cultural norms and social protocols, going far beyond simply modifying verbs. Honorifics impact noun choices and sentence structures, requiring a nuanced comprehension that current AI models often struggle with. Studies have shown that AI translation accuracy for Korean can suffer significantly—as much as 30%—when honorifics are mishandled. This underlines the need for AI translation tools to be trained on data that includes the full range of cultural and social nuances in Korean conversations.
The current limitations of AI translation models when encountering Korean honorifics aren't just technical issues, but indicate a more fundamental gap in the technology's ability to capture human social constructs and interactions. To improve accuracy, future AI models will need to move beyond a purely linguistic understanding of language and develop a greater sensitivity to cultural context, including implicit meanings expressed through politeness and social hierarchy. Simply put, more human-like empathy will likely be required to bridge the gap in translation accuracy for Korean and other languages with similar complex social implications. While technical advancements continue to be made, their practical application to languages with such intricate social norms continues to be a challenge. Moving forward, it will be critical to explore ways to train AI to truly understand the "why" behind linguistic choices, rather than simply relying on statistical patterns found in large datasets.
Top 7 AI Translation Challenges When Working with Asian Languages From Japanese OCR to Bengali Script Recognition - Thai Language Zero Word Spacing Poses Natural Language Processing Hurdles
Thai poses a challenge for artificial intelligence (AI) due to its unusual writing style—a complete lack of spaces between words. This makes it tricky for computers to separate words, a process known as word segmentation, which is fundamental for many NLP tasks, including translation. Furthermore, Thai has a distinct tonal structure with five unique tones that alter word meanings. This makes accurate tone recognition a must for AI models, particularly in speech-to-text applications. While there are attempts to address these challenges, like PyThaiNLP, a Python library built to handle Thai text, the language isn't as well-supported in AI research compared to others. This lack of resources slows down the creation of better, more efficient tools, especially in the realm of machine translation. As users increasingly rely on fast, accurate AI translation, addressing Thai's unique features will become even more important.
### Thai Language Zero Word Spacing: Natural Language Processing Hurdles
1. **The Absence of Word Separators:** Thai's unique characteristic of not using spaces between words presents a major challenge for NLP systems. Traditional methods that rely on spaces to distinguish words simply don't work, demanding specialized algorithms for word segmentation.
2. **Contextual Ambiguity:** Without clear word boundaries, the same string of characters in Thai can have multiple interpretations, depending on the context. This makes it tough for AI to accurately figure out the intended meaning, which can lead to translation errors or misunderstandings.
3. **Bridging the Gap Between Writing and Speech:** The written and spoken forms of Thai can diverge considerably. This creates difficulty for systems trying to transcribe or translate spoken language since the phonetic transcriptions may not map directly to the written words.
4. **OCR's Double Duty:** OCR tools designed for Thai need to not only recognize characters but also perform word segmentation. This added complexity can impact processing speeds and accuracy, especially when rapid translation is needed, such as in real-time communication.
5. **Scarcity of Training Data:** Compared to widely-used languages, there's a limited availability of high-quality datasets for training NLP models specific to Thai. This lack of data can hamper the development of models capable of truly capturing the nuances of the language.
6. **Regional Variations:** The existence of various dialects and regional accents within Thailand creates challenges for translation models. Models trained on a standard version of Thai might not perform well when encountering these variations, limiting their usefulness in diverse situations.
7. **Cultural Expressions and Idioms:** Thai is rich in idioms and cultural expressions that don't always have direct translations in other languages. This presents a significant hurdle for AI translation, as capturing these subtle nuances is crucial for accurate and natural-sounding output.
8. **The Role of Punctuation and Tones:** Thai employs a variety of punctuation marks that contribute to the rhythm and meaning of sentences. NLP models need to accurately interpret these marks, as misinterpretations can lead to lost information or incorrect translations.
9. **Accessibility for Smaller Users:** Smaller businesses seeking affordable AI translation might find that current tools aren't fully equipped to handle the complexities of the Thai language. This can lead to a reliance on manual corrections and slower processing times, impacting both efficiency and cost-effectiveness.
10. **Future Directions for Thai NLP:** The challenges presented by Thai language necessitate innovative solutions in NLP. Continued research and investment in tailored algorithms and tools are needed to improve accuracy and efficiency in Thai language translation, a crucial area for communication and technological advancement.
Top 7 AI Translation Challenges When Working with Asian Languages From Japanese OCR to Bengali Script Recognition - Vietnamese Tone Mark Placement Affects OCR Text Detection Rate
When it comes to recognizing Vietnamese text using Optical Character Recognition (OCR), the placement of tone marks plays a surprisingly crucial role. These diacritical marks, which modify the sound of vowels, must be positioned precisely according to specific linguistic rules. If they're not, the accuracy of the OCR system can suffer, making it harder to reliably identify and interpret the text.
Fortunately, OCR technology has shown improvements in handling both Vietnamese characters and those found in the Latin alphabet, showcasing some adaptability. Some newer OCR methods employ neural networks to simultaneously detect and recognize text, leading to faster and more accurate results. However, even with these advancements, OCR still isn't perfect. Issues like errors in recognizing characters and difficulty understanding the overall context of the text can still occur. To get around these problems, researchers are exploring approaches that combine both visual cues (what the text looks like) and linguistic knowledge (the rules of the Vietnamese language).
These ongoing efforts to improve OCR for Vietnamese are important as they're a necessary step towards creating truly reliable and effective AI translation systems that can tackle a wider range of languages, including those that use complex writing systems like Vietnamese. It's a complex problem with no easy solutions, but it underscores the need for continued innovation within OCR and related fields.
Vietnamese, with its intricate system of tone marks, presents a unique challenge for AI-powered Optical Character Recognition (OCR). The correct placement of these marks, which modify the pronunciation and meaning of a syllable, is crucial for accurate text recognition. While OCR systems have generally proven adept at recognizing both Vietnamese and Latin characters, the presence of tone marks adds a layer of complexity.
The problem arises because a small shift in the position of a tone mark can lead to a completely different interpretation of a word. This sensitivity to subtle visual cues makes it difficult for OCR systems, which often rely on pattern recognition, to consistently distinguish between similar-looking characters. For instance, an AI model might misinterpret a word due to a slightly misplaced tone mark, leading to an entirely inaccurate translation.
Furthermore, the abundance of diacritics in Vietnamese text increases the complexity of the OCR process. The sheer density of these marks can lead to increased chances of misreads, especially in situations where the OCR system lacks a broader understanding of the sentence or document context. This becomes particularly problematic when aiming for rapid translation, as AI systems may not have sufficient time to analyze the full context and rely more heavily on individual character recognition.
Another aspect to consider is that training datasets used for many OCR systems are often built using standardized text, which may not capture the diverse range of Vietnamese writing styles and dialects found in real-world documents. This discrepancy between training data and the complexities of everyday usage means that OCR systems may struggle to handle variations in tone mark placement and overall script variations.
The need to consider both visual and phonetic aspects of Vietnamese characters further complicates OCR development. Current systems often excel at recognizing the visual shapes of characters but lack the ability to fully process the phonetic implications of tone marks. This gap in processing capabilities results in frequent errors, hindering the overall quality of AI translation services.
The computational burden associated with incorporating more nuanced models capable of recognizing and interpreting tone variations can be substantial. This can pose a challenge for smaller businesses that might struggle to afford the necessary resources. The issue is compounded by the possibility of interference from surrounding characters, particularly when tone marks are densely clustered. This can cause a ripple effect of errors, diminishing the overall translation accuracy.
Additionally, real-time applications like live translation or chatbots often face delays due to tone misinterpretation, significantly impacting user experience. Variations in tones across different Vietnamese dialects can also pose challenges, as OCR systems trained on a specific dialect might not accurately recognize tonal variations found in other regions.
Despite these difficulties, ongoing advancements in machine learning, particularly context-aware models, are promising in enhancing the accuracy of tone recognition within Vietnamese. However, truly harnessing these advances requires a deep understanding of the intricacies of the language, specifically the rules governing tone usage in different situations. This suggests that there's still much research and development necessary to create AI translation solutions that seamlessly integrate the visual and phonetic aspects of Vietnamese.
Top 7 AI Translation Challenges When Working with Asian Languages From Japanese OCR to Bengali Script Recognition - Hindi Script Ligature Formation Complicates Character Recognition Tasks
Hindi's use of the Devanagari script presents a significant challenge for AI-based character recognition, primarily due to the complex way characters combine to form ligatures. Unlike simpler alphabets where characters maintain their distinct shapes, Hindi characters can merge and change appearance when combined, creating a wide variety of forms. This poses a problem for OCR systems, which often rely on identifying distinct character patterns. The ability to differentiate between similar-looking characters formed by ligatures becomes crucial for accurate recognition, requiring sophisticated algorithms to analyze subtle visual differences. Achieving high accuracy in Hindi recognition is therefore more challenging compared to languages with simpler scripts. Consequently, the development of OCR for Hindi requires more advanced AI models and refined techniques to capture these intricacies. The difficulties encountered in Hindi recognition highlight the broader difficulties in applying AI translation to languages with visually complex scripts, underscoring the ongoing need for more advanced methods to improve accuracy and efficiency.
Hindi, written in the Devanagari script, presents a unique challenge for AI-driven character recognition, particularly due to the complex way characters are combined to form ligatures. The Devanagari alphabet, written horizontally from left to right, encompasses a diverse range of characters, making it suitable for numerous Indian languages like Hindi, Sanskrit, and Marathi. Several datasets, such as the IITKGP Hindi and CDAC GIST collections, help researchers improve handwritten Hindi recognition across varying sizes and intended applications. However, these datasets and the overall research field face obstacles in accurately capturing the full spectrum of character combinations that make Hindi visually challenging.
Offline handwritten Hindi character recognition systems aim for high accuracy despite the sheer number of characters and complex combinations, with deep learning techniques like Convolutional Neural Networks (CNNs) frequently utilized. Understanding the directional properties of handwritten strokes often uses neural networks in structural analysis. However, the sheer variety of handwriting styles and the frequent presence of low-quality image scans pose ongoing difficulties in achieving optimal results.
Optical Character Recognition (OCR) is vital for turning printed or handwritten Hindi into machine-readable formats, enabling further processing and translation. Optimized approaches for recognizing Hindi script prioritize feature extraction tailored to the unique characteristics of the Devanagari script. But the task becomes particularly complex when considering the numerous ways characters can be joined into ligatures. Sophisticated models are needed to reliably distinguish between similar character forms within these ligatures.
Researchers are exploring Siamese Neural Networks for improving accuracy in Hindi character recognition through the use of twin networks for superior feature matching within OCR. However, a key limitation in the advancement of this research area is the limited availability of comprehensive datasets that can capture the nuances of the Devanagari script, particularly the intricate variations in ligatures found in everyday writing. The cost of building and training sufficiently large and robust models can be high, potentially limiting the accessibility of optimized Hindi OCR for smaller businesses or individuals.
While research and advancements are continuously being made, there's still a long way to go before OCR solutions for Hindi are truly reliable and effective in handling the complexities of the script, especially in real-time translation or document processing. The integration of contextual understanding with traditional visual-based feature extraction will likely be crucial in overcoming the current limitations. This challenge isn't solely restricted to Hindi OCR but highlights the broader difficulty faced when trying to develop effective AI-driven solutions for less common and visually intricate language systems across the globe.
AI-Powered PDF Translation now with improved handling of scanned contents, handwriting, charts, diagrams, tables and drawings. Fast, Cheap, and Accurate! (Get started for free)
More Posts from aitranslations.io: