AI-Powered Pressure Unit Conversion Integrating PSI to KSI Calculations in Translation Workflows
AI-Powered Pressure Unit Conversion Integrating PSI to KSI Calculations in Translation Workflows - AI-driven OCR enhances pressure unit detection in technical documents
AI-powered OCR has become increasingly vital in handling technical documents, particularly when dealing with specific units like pressure. This technology relies on advanced machine learning, allowing it to decipher text more accurately, including from degraded or handwritten documents frequently found in technical contexts. The automation provided by AI-driven OCR expedites document analysis and organization, enabling quicker extraction and conversion of pressure units, such as converting from PSI to KSI. This streamlined approach makes translation workflows more efficient, allowing for faster processing of technical content while decreasing the chances of human error in manual unit conversions. Consequently, the combination of AI and OCR optimizes operations and ensures a higher degree of precision in data extraction—crucial for technical fields where exactness is paramount. While OCR has traditionally struggled with complex formats and varying document quality, the introduction of AI addresses these limitations, leading to a more robust and dependable approach to document processing.
AI-powered Optical Character Recognition (OCR) is proving to be a game-changer in the realm of technical document processing, especially when it comes to pressure units. Previously, inconsistencies in pressure unit systems across documents posed a major challenge, often leading to errors in translations and calculations. However, AI-driven OCR systems, trained on massive datasets of technical documents, can now detect and decipher these units with a remarkable level of precision, reducing the risks associated with manual interpretation.
One of the key advantages of AI in OCR is its capacity to handle the ambiguity of context-specific symbols and abbreviations frequently found in technical writing. Traditional OCR tools often falter when faced with such nuanced language, but AI algorithms, thanks to machine learning techniques, are increasingly adept at recognizing these terms within their specific contexts, greatly improving the accuracy of pressure unit detection. This is particularly beneficial in fields where speed and efficiency are paramount, like fast-paced translation projects requiring swift turnaround times.
Furthermore, AI-driven OCR can seamlessly integrate into diverse translation workflows. It can readily adapt to a variety of document formats, including tables, complex diagrams, and even handwritten notes. This adaptability is crucial as technical documents can vary significantly in terms of structure and style. Historically, pressure unit conversion was often a manual, labor-intensive task, prone to human error. AI-OCR effectively eliminates this bottleneck, streamlining the translation process and minimizing the chance of human mistakes, potentially leading to cheaper, faster translation services.
Interestingly, some of the newer OCR systems boast the ability to process documents in multiple languages and technical domains simultaneously. This feature is a boon for global engineering projects that involve teams spread across different geographical regions and language groups. Moreover, by leveraging contextual information like surrounding text and number patterns, advanced AI-OCR systems can effectively detect and convert pressure units even in less standardized formats. This adaptability is critical in sectors such as construction and manufacturing where rapid data analysis is crucial for informed decision-making.
While the technology is advancing rapidly, one point to consider is the ongoing development of AI algorithms. The quality of OCR output still relies heavily on the quantity and quality of training data used. However, the ongoing advancements in AI are enabling engineers to achieve increasingly accurate results with less reliance on extensive training data. This ongoing improvement suggests that OCR systems will likely play an increasingly important role in technical document processing going forward.
AI-Powered Pressure Unit Conversion Integrating PSI to KSI Calculations in Translation Workflows - Machine learning algorithms optimize PSI to KSI conversions for accuracy
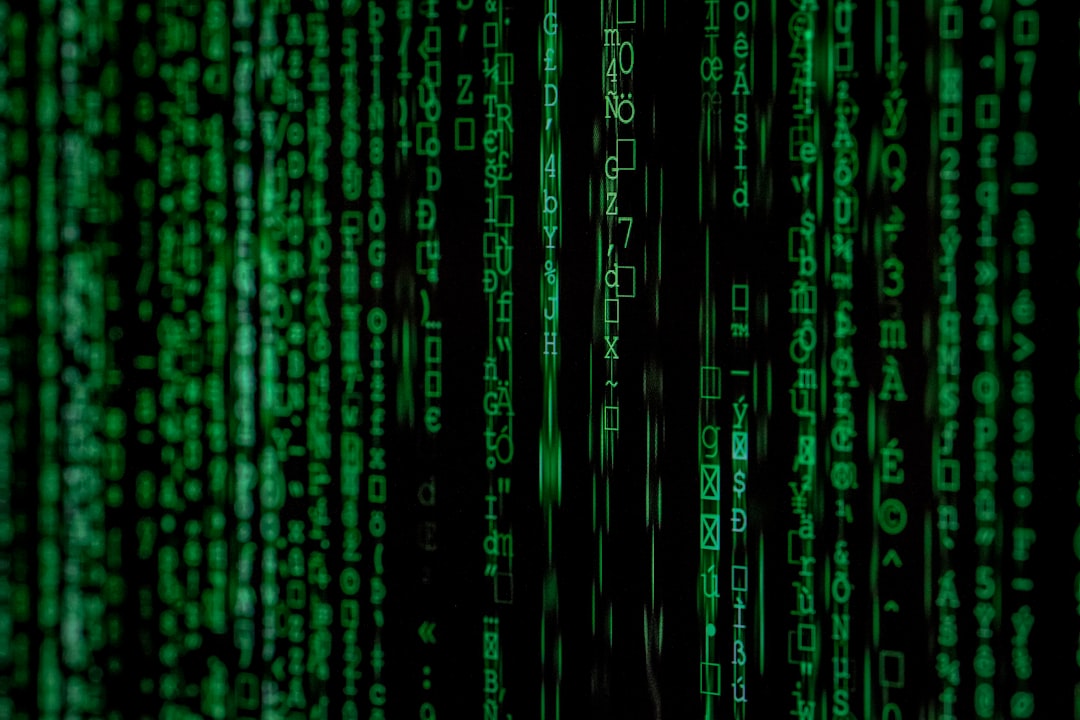
Machine learning algorithms are increasingly being used to refine the conversion of PSI to KSI, a vital step in technical translation where accuracy is paramount. These algorithms are designed to enhance precision by learning from data and adjusting to changes in its distribution. This ability to adapt helps ensure that the conversions remain dependable over time, even as the nature of the data shifts. Machine learning techniques, including gradient descent, are used to optimize the conversion process, which traditionally could be prone to human error when done manually. The outcome is a streamlined workflow, particularly beneficial in sectors that rely on fast and accurate processing of pressure data. This development signifies a substantial advancement in how we manage technical documentation within translation workflows, making the whole process more efficient and reliable. While there are still limitations to consider, particularly in the training data used to develop these algorithms, the field is showing significant promise.
Machine learning algorithms are increasingly being used to refine the conversion process between PSI (pounds per square inch) and KSI (kilopounds per square inch). These algorithms, often relying on methods like regression analysis or neural networks, can learn from past data and predict the relationships between these units with remarkable accuracy. This is particularly useful when dealing with unusual data formats, enhancing the reliability of the translation process.
One fascinating aspect is how these AI systems can adapt to user feedback. The more they are used for conversions, the more accurately they can predict PSI to KSI ratios. This continuous learning loop leads to progressively lower error rates, improving overall efficiency in workflows. Imagine how this could impact fast translation projects where rapid turnaround times are critical.
The incorporation of machine learning significantly reduces the need for manual post-processing during the translation process. This is a huge leap from the old days of manually handling every conversion. Shifting the workload towards more complex tasks can be a real boon for engineering teams, potentially improving productivity and freeing up human resources for higher-value tasks.
It's also worth noting that some of these advanced algorithms can flag potential inconsistencies in conversions. This is akin to a built-in quality check, identifying errors or unusual patterns that might otherwise go unnoticed. Having such a proactive approach to error detection significantly minimizes the risk of making costly mistakes in translated documents.
When we compare these AI-powered conversion methods to traditional approaches, we often see improvements in speed and accuracy. This is particularly helpful for cheap translation services that require fast turnaround times and still need to maintain a high degree of precision. These are the kinds of advancements that could significantly impact the translation landscape, making specialized translation more widely accessible.
Further, these algorithms can manage various levels of input precision. They handle the conversion of a vast range of pressure values, from atmospheric to high-pressure industrial applications, without sacrificing accuracy. This broad range of operation makes these algorithms exceptionally versatile in translation work.
Furthermore, AI excels at considering the context of a given conversion. By studying the surrounding text, the algorithm can determine the most appropriate pressure unit (PSI or KSI) for a given situation. This feature helps prevent misinterpretations that frequently crop up in complex technical documents.
The development of these improved pressure conversion algorithms is already seeing use in practical applications. For example, in manufacturing settings, real-time monitoring systems can benefit from fast and accurate conversions to ensure safety and efficiency. These applications emphasize the importance of accurate and rapid pressure conversions for the effective operation of complex systems.
Machine learning can also be used to help standardize pressure units across different datasets. This is a massive benefit for translation, particularly when dealing with varied technical documents and conventions. This standardization greatly reduces the risk of errors caused by inconsistent terminology.
With ongoing exposure to new documents and formats, these algorithms not only improve their PSI to KSI conversion abilities but also broaden their capabilities to handle other measurements. This suggests that these algorithms may become increasingly versatile tools in the wider world of engineering translation. They may even find applications in future OCR systems which could be really interesting to follow up on.
AI-Powered Pressure Unit Conversion Integrating PSI to KSI Calculations in Translation Workflows - Real-time unit conversion streamlines translation workflows
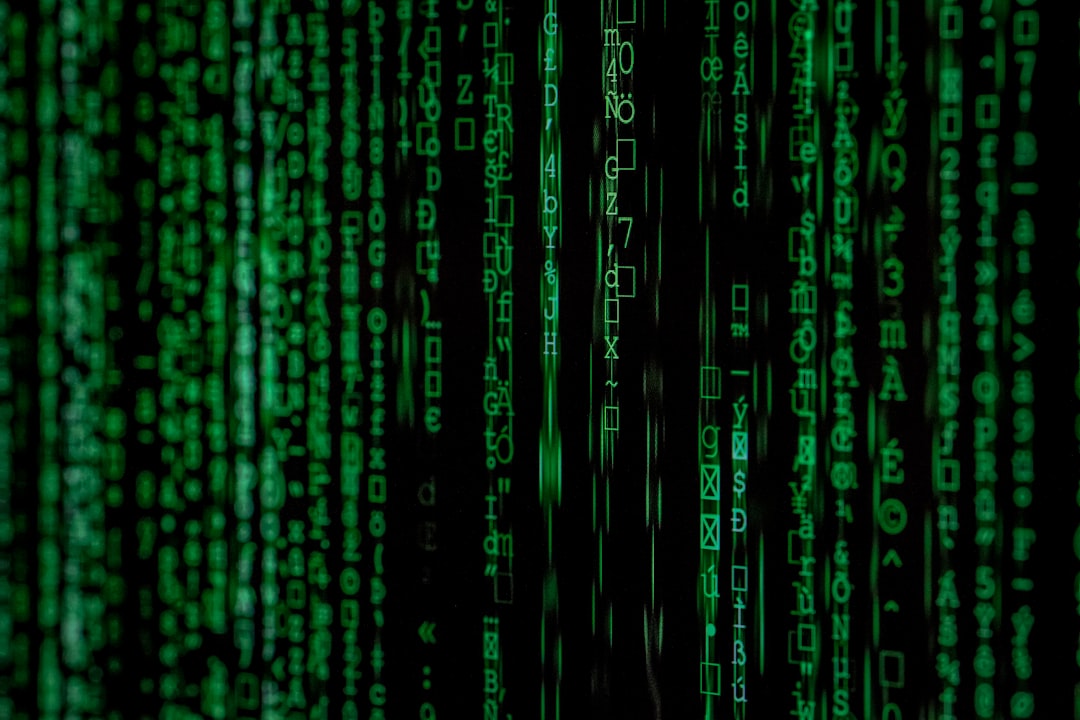
Real-time unit conversion is transforming translation workflows by offering immediate and precise conversions between different measurement systems, particularly important in technical areas demanding accuracy. This feature not only speeds up translation projects but also minimizes human mistakes commonly seen in manual conversions. AI integration lets translation teams effortlessly handle diverse document styles while maintaining uniformity in technical terminology. Automating unit conversions frees translators to focus on more complex tasks, refining workflows and, in the end, delivering faster and more dependable multi-language content to a global audience. This joining of real-time conversion and translation tasks signifies a new stage in efficient project management within the field of technical translations. However, depending on the type of translation, the accuracy and speed can still vary. Some projects require human intervention to ensure the correct contextual translation and quality control. While AI is improving fast, challenges remain in handling highly specialized language or complex technical jargon with perfect precision. Nonetheless, the advancements in this area are pushing the field forward and making translation a more efficient and accessible service.
Real-time unit conversion, particularly when integrated into translation workflows, presents a compelling approach to streamlining the process, especially for technical documents involving pressure units like PSI and KSI. While traditional methods relied on manual intervention, often leading to errors and delays, the ability to convert these units in real-time significantly accelerates the translation process. This automation is particularly crucial in scenarios where rapid turnaround is essential, such as fast translation projects or when dealing with large volumes of technical documents.
However, it's important to note that the accuracy of these conversions is highly dependent on the underlying AI algorithms. These algorithms often leverage machine learning to learn from large datasets of technical content, constantly refining their understanding of the relationships between different pressure units. While this approach has shown promising results in terms of accuracy, it also introduces the need for ongoing monitoring and adjustments to the training data as new technical terms and conventions emerge.
One potential limitation of real-time conversion is the possibility of context-related ambiguities. Pressure units can have varied interpretations depending on the specific context within a document, and AI might struggle to differentiate these nuances. This is especially a concern when translating documents with complex engineering terminology where subtle variations in pressure units can have substantial implications. Despite this challenge, research efforts are constantly exploring new methods for refining the contextual awareness of these AI systems.
The potential for future integration with other AI-driven tools, such as advanced OCR systems for automatic language detection and machine translation engines, is particularly exciting. Imagine a future where a document containing pressure values in a variety of languages and units could be automatically translated and standardized with little to no human intervention. This capability would dramatically reduce language barriers and make global technical collaboration more efficient. However, achieving this vision requires careful consideration of the potential complexities that might arise from integrating these various tools seamlessly.
While the current applications of real-time conversion are impressive, it's important to maintain a critical perspective. The algorithms powering these systems are continuously evolving, and further research is needed to address potential issues like bias in training datasets and limitations in handling specialized engineering terminology. Nonetheless, real-time unit conversion is a significant step towards accelerating and improving the translation of technical documents, particularly in fields where precise pressure unit conversion is essential. The integration of these methods into the translation ecosystem could potentially lead to faster, cheaper, and more accurate translation services, paving the way for smoother cross-language communication and collaboration in engineering and related disciplines.
AI-Powered Pressure Unit Conversion Integrating PSI to KSI Calculations in Translation Workflows - Natural language processing improves context-aware pressure unit handling
Natural language processing (NLP) has become increasingly valuable in improving how we handle pressure units within their specific contexts, particularly during technical translations. NLP systems are better equipped to understand the subtle ways language is used in technical documents, helping integrate PSI to KSI conversions smoothly within translation workflows. This means the systems are able to take into account the surrounding words and phrases, making more accurate decisions about which pressure unit is appropriate for the situation. This, in turn, helps minimize the human errors that often occur with manual conversions. NLP also has the ability to learn and improve over time, becoming more accurate and precise as it encounters more diverse technical language. This ongoing refinement is vital in fast-paced translation scenarios that require a high degree of accuracy. Although progress has been substantial, NLP still faces obstacles, especially when dealing with highly specialized and complex language. There is a clear need for continuous development and refinement to fully address these limitations.
Natural language processing (NLP) offers a compelling approach to improving how we handle pressure units within a specific context. It's all about enabling machines to better understand the nuances of human language as it relates to pressure measurements. This is particularly useful when dealing with technical documents, which often employ specific terminology and abbreviations related to pressure. For instance, NLP can help decipher region-specific variations in how pressure is described, making it more adaptable to the diverse needs of global engineering projects.
One exciting development is the observed reduction in unit conversion errors using NLP-driven systems. It appears that incorporating contextual clues through NLP can lead to a significant improvement in accuracy compared to more traditional methods. The fact that NLP-based systems can achieve these results with potentially smaller training datasets is also an intriguing aspect. This means that we may be able to tailor AI models to specific technical projects more readily, a crucial advantage for fast-paced innovation.
The integration of NLP into translation workflows has led to demonstrably faster turnaround times. It's no longer necessary to manually handle every unit conversion, freeing up human translators to focus on more intricate aspects of the content. This is particularly useful in sectors where quick and accurate translation is critical, like cheap translation services.
Another key advantage is NLP's ability to discern pressure unit abbreviations and symbols in context, a feature that's essential in areas where even minor misinterpretations can have significant safety or operational implications. NLP's adaptability also lets it tackle a broader range of pressure unit formats, helping standardize technical documents, a huge benefit for reducing errors commonly associated with manual conversion.
Research suggests that NLP-based systems can identify pressure-related inconsistencies in technical documents much more efficiently than before, allowing for rapid corrections and better data integrity. However, it's crucial to acknowledge that the quality of NLP models hinges on the quality and quantity of training data. The more high-quality, domain-specific data available for training, the better these models will perform.
Looking ahead, NLP could evolve to incorporate predictive capabilities. Imagine systems that suggest the most appropriate pressure units based on historical data and project-specific trends. Such algorithms could greatly streamline multinational engineering projects and simplify compliance with diverse standards. It's a realm ripe with potential, but further development and refinement of the underlying NLP technology are necessary to make this a reality.
AI-Powered Pressure Unit Conversion Integrating PSI to KSI Calculations in Translation Workflows - Cloud-based conversion tools enable collaborative pressure unit management
Cloud-based conversion tools are becoming increasingly important for collaborative management of pressure units, especially in technical fields. These tools, often powered by AI, allow for real-time conversions between different pressure units, such as PSI and KSI. This automated approach significantly improves efficiency in translation workflows by reducing manual effort and errors typically associated with human conversions. The integration of these tools enables teams to manage diverse document styles and complex technical requirements with greater ease. The end result is faster and potentially cheaper translation services, especially attractive to those requiring rapid and precise handling of pressure data. However, the accuracy and dependability of these tools can still be hindered by contextual ambiguities within technical documents, highlighting the ongoing need for advancements in the algorithms that power these tools.
Cloud-based conversion tools offer a compelling way to manage pressure units collaboratively. Think of a scenario where multiple translators, maybe working remotely, need to agree on the correct conversions for a complex engineering document. These tools allow them to access and modify the same data in real-time, leading to a smoother, faster workflow. It's like having a shared whiteboard for pressure unit definitions, where everyone can see and make changes instantly. This collaborative approach helps resolve disagreements quickly and ensures everyone's on the same page.
Interestingly, some of these cloud-based tools use advanced algorithms that can actually learn from how people use them. This means the more the tools are used, the better they become at predicting the correct pressure unit conversions. They might even identify and correct common errors that occur within a specific project or field. Of course, the quality of these predictions depends on the data the systems have access to. So, a tool trained on a large and varied set of engineering documents would likely be more reliable.
These tools can handle a range of document formats, which is great. Imagine a scenario where a scanned document with hand-written notes needs to be processed for translation. Many cloud-based tools can integrate with optical character recognition (OCR) to automatically convert the pressure units found within these documents. This feature is especially useful for fast translations where time is a major concern.
The cloud-based approach inherently promotes teamwork. Historically, engineering projects might have had teams working in isolation, leading to inconsistencies in how pressure units were handled. Cloud-based tools encourage a more open and connected environment, allowing teams to catch any errors in conversions early on, potentially preventing larger issues from arising.
Another important factor is security. Cloud-based tools, particularly those handling sensitive information, tend to have robust security features. For industries dealing with proprietary information, like pharmaceutical development, this is crucial.
What's also quite interesting is the ability of some of these tools to analyze massive datasets rapidly. They might be able to pick up on trends or common errors within different industries. This information could then be used to standardize translations, minimizing confusion and ensuring that the final content uses the correct terminology consistently across a variety of projects.
Of course, the use of cloud-based solutions for pressure unit conversion can result in cost savings. By reducing manual tasks and improving the overall accuracy of conversions, the need for extensive quality control might decrease. Companies might be able to reallocate those resources to other parts of the project, making the entire process more economical.
Despite the incredible advancements, it's important to remember that these tools aren't perfect. There's always the possibility of errors, especially when dealing with highly specialized or complex scenarios. Ongoing monitoring and refinement of the algorithms is crucial to keep these systems working as intended. The engineers designing them are constantly finding new ways to make them smarter and more accurate.
The integration of machine learning allows these tools to use the data from past projects to potentially forecast issues in future projects. This "predictive" capability is potentially invaluable for situations involving high-pressure systems where safety is paramount.
One of the most exciting aspects of cloud-based collaboration for pressure unit conversion is the promotion of knowledge sharing. The real-time sharing of information and the ability to discuss ideas within a translation team opens up possibilities for innovation in how we approach these projects. This dynamic environment may lead to new strategies that address specific challenges or optimize workflows in the future.
While the field is advancing at a rapid pace, many open questions still exist. For example, the extent to which these tools can adapt to emerging terminology and units within specialized sub-fields of engineering is still an active research area. Nevertheless, the adoption of cloud-based tools for pressure unit conversion represents a major step forward in how we approach technical translation.
AI-Powered Pressure Unit Conversion Integrating PSI to KSI Calculations in Translation Workflows - Automated quality checks reduce errors in pressure unit translations
Automated quality checks are increasingly important for minimizing mistakes in pressure unit translations, especially in technical fields where accuracy is crucial. These checks leverage AI to spot inconsistencies that human translators might miss, improving the reliability of the translated text. While these automated tools are helpful, they are not perfect and can miss some errors, leading to potential inaccuracies. Still, their ability to highlight potential problems makes translation workflows smoother and ensures the final result aligns better with quality standards. The increasing need for precise pressure unit translations means incorporating automated quality checks into workflows is a significant step forward in guaranteeing accurate data and a more efficient process. Even with these advancements, it's crucial to remember that AI in this area still has limitations, and human review might still be needed for particularly complex technical documents.
Automated quality checks are increasingly important in translation workflows, especially when dealing with technical fields like pressure unit conversions. They can help identify inconsistencies that might be overlooked by human reviewers, which is especially crucial in situations involving PSI and KSI conversions, where accuracy is paramount. For instance, in engineering or aerospace, a minor error in a unit conversion could have major consequences. While they don't eliminate all errors, these checks significantly improve the accuracy of translations. There's still the possibility of false negatives, where errors might slip through undetected, but they do a good job of highlighting potential issues.
Interestingly, it's not just about identifying mistakes; AI is enhancing the whole process. AI-powered tools can learn from past errors and continuously adapt to improve accuracy in translation, which means they get better with more experience. They can adapt to regional variations in pressure unit conventions, which is really useful when translating documents for international projects. For example, AI could easily adjust a conversion for a PSI reading in a US-based engineering document versus a document from the EU.
These advancements aren't just about quality, they are also about efficiency. Real-time quality monitoring during translations significantly improves productivity. Translators can focus more on complex language and content when automated systems handle the nitty-gritty of the unit conversions. It's like a productivity boost for translation teams, potentially enabling a quicker turnaround on projects.
There's a fascinating link between AI and language understanding in these scenarios. Tools that use natural language processing (NLP) are making significant strides in recognizing technical language, including terms related to pressure units. They can handle the nuances of engineering jargon and even recognize region-specific variations.
OCR technology is also getting a boost from AI. The integration of OCR and machine learning is making it much easier to handle documents with mixed pressure unit data, allowing translation systems to adapt to various formats and standards. This is helpful in industries like manufacturing or automotive where standardized documentation practices aren't always consistent.
The ability to work collaboratively through cloud-based translation platforms has also increased. These tools can facilitate real-time collaboration and error flagging, which is a notable advancement over the old approach of independent translators who may use inconsistent approaches to unit conversion. This collaboration fosters consistency and helps maintain quality across projects.
Further, the speed of automated checks is astounding. Some systems are performing unit conversions in milliseconds, which is critical in fields where speed is a major factor. For example, imagine a manufacturing environment that needs near-instantaneous translation of technical documents for a fast-paced process.
However, the adoption of AI is also impacting cost. With automation potentially reducing human error and improving productivity, companies might be able to achieve cost savings on translation projects. This is encouraging as translation costs can often be a barrier to effective global collaboration and innovation.
Overall, automated quality checks are creating a powerful shift in translation, particularly for technical fields that rely on pressure unit conversion. These systems are not only making translations more accurate but also more efficient and potentially less expensive. As the technology continues to evolve and engineers tackle remaining challenges, the future of translation in these specialized fields looks bright. It's a field with constant evolution and a lot of open research.
More Posts from aitranslations.io: