AI-Powered PDF Translation now with improved handling of scanned contents, handwriting, charts, diagrams, tables and drawings. Fast, Cheap, and Accurate! (Get started for free)
AI-Powered Translation Guide Understanding Mexico's CLABE Banking Codes for International Business Transactions
AI-Powered Translation Guide Understanding Mexico's CLABE Banking Codes for International Business Transactions - Visual OCR Recognition for Mexican Bank Codes Going Live January 2024
Starting January 2024, a new visual OCR system for recognizing Mexican bank codes will be available. This means that computers will be able to automatically read CLABE numbers, those 18-digit identifiers vital for Mexican banking. The technology should improve the accuracy and speed of processing these codes, which are essential for both domestic and international money transfers. Businesses relying on Mexican banking will hopefully see benefits from this, such as fewer errors and more efficient data handling. While some may see this as simply faster processing, it also introduces the possibility of enhanced security for sensitive financial data. This change in how CLABE codes are handled highlights the ongoing shift towards digitalization within the Mexican financial system, a change driven by the increasing global interconnectedness of the world's economy. It will be interesting to see the long-term impact of this move on efficiency and security.
Starting January 2024, a new approach to reading Mexican bank codes, called Visual OCR, is expected to transform how these codes are processed. The idea is to significantly cut down on mistakes that happen when people manually type in these 18-digit CLABE numbers. It's been suggested that manual errors can be a surprisingly large portion of banking transaction errors, potentially around 30%.
Unlike older OCR systems that were designed for straightforward text, this new Visual OCR technology relies on algorithms that can understand the unique visual patterns found in CLABE codes. This not only makes the processing faster – possibly capable of handling many thousands of transactions each second – but it might also mean less reliance on time-consuming human review for each transaction. Companies that deal with Mexican businesses might find this helpful in reducing costs related to these international transactions.
Interestingly, this new visual recognition technology is expected to continually learn and improve as it's used more. This 'self-learning' aspect is a promising feature that can provide ongoing benefits to users. Preliminary estimates suggest that the average transaction processing time could be slashed from several minutes to just a few seconds by implementing this AI-driven OCR system. This could prove beneficial for both banks and their customers.
It's not just about reading numbers. The system also seems capable of understanding the context of information within a transaction document, making it more effective than a human would be at quickly grasping what's going on. Research suggests that these advanced deep learning-based systems can read and decipher these kinds of complex numeric codes with over 95% accuracy – a marked improvement over past OCR software.
The coming year should see a noticeable impact, particularly among major banks. Reduced operational costs, potentially as high as 40%, are predicted, driven by decreased reliance on labor and faster transaction speeds. Further, this approach could enhance security in international financial transactions, making it harder to cheat the system compared to manually processing information. Importantly, this Visual OCR tech is designed to work with current banking software, allowing smooth implementation and minimizing disruption. It showcases how powerful advanced image recognition can be in modernizing finance.
AI-Powered Translation Guide Understanding Mexico's CLABE Banking Codes for International Business Transactions - Converting Paper Bank Statements to CLABE Numbers With Neural Networks
Converting paper bank statements into digital formats, specifically extracting CLABE numbers, has become much easier with the use of neural networks. These networks, paired with AI and OCR technologies, are capable of automatically reading and understanding the information on physical bank statements. This is extremely helpful in situations where international transactions involve Mexican banks, where the 18-digit CLABE code is crucial. The biggest advantage here is likely the improved accuracy; neural networks are designed to reduce errors that often occur when humans manually type in these codes. Businesses can streamline their financial processes significantly, boosting the overall speed and efficiency of international transactions. This approach also supports the growing push for digitalization in financial services, a trend spurred by the ever-increasing need for quick, accurate processing in a globalized world. While the promise of faster transactions and fewer errors is clear, there's always a question of just how well these neural networks will actually adapt to the real-world variations in handwriting and printing styles on these statements. It will be interesting to see how quickly and smoothly these new systems can be implemented, particularly for businesses with large volumes of paper statements to process.
CLABE numbers, those 18-digit Mexican banking identifiers, are becoming easier to extract from paper bank statements thanks to advances in deep learning-based OCR systems. While traditional OCR methods often struggle with handwritten or low-quality documents, achieving perhaps around 80% accuracy, these newer neural network approaches can reach over 95% accuracy, a significant leap forward. It's fascinating how these systems learn and improve over time, something static OCR can't do.
The speed gains are also impressive. The current expectation is that these systems can cut transaction processing times from several minutes down to under 10 seconds – that's a potential 90% improvement in efficiency. Imagine the impact on businesses doing international banking with Mexican partners! Of course, errors in manual data entry can cause a lot of trouble. It's been estimated that manual errors are responsible for roughly 30% of transaction errors in some banking systems. The potential for these kinds of AI-powered OCR systems to reduce those errors is pretty clear.
Beyond faster transactions, though, is the intriguing prospect of freeing up human workers to tackle more important tasks. Automating OCR can cut costs by up to 40%, and that's money that can be reinvested or used to improve other parts of the business. It also shifts people away from tedious data entry jobs, which is a good thing. Interestingly, the capability to understand context in bank statements might make spotting fraudulent activities easier as well, as unusual patterns can be automatically detected.
A big difference between traditional OCR and this newer approach is the streamlined workflow. Older OCR systems often need a lot of pre-processing steps before they can read a document, but the newer Visual OCR systems are designed for a more continuous flow, capable of handling hundreds or thousands of transactions without needing constant human input. That ability to process many transactions concurrently is key to keeping up with the growing volume of financial data. Ultimately, this could lead to a more satisfying customer experience. Faster transactions typically mean happier customers.
It's the sophisticated image recognition algorithms at the heart of these systems that make them able to handle so many different document formats and font styles, something older OCR methods struggled with. It's remarkable how these systems can handle the various complexities of bank statements. It's not too far-fetched to think that automated systems might reduce the chance of errors during transaction processing by 70%—that's a significant reduction in risk. While the initial adoption is mostly among major banks, it's only a matter of time before smaller financial institutions begin to utilize the benefits of these fast and accurate OCR systems. It's an exciting time to see how this field is evolving.
AI-Powered Translation Guide Understanding Mexico's CLABE Banking Codes for International Business Transactions - Cross Border Payment Verification Through CLABE Analysis
Cross-border payments are becoming a larger part of the global economy, expected to reach a massive $290 trillion by 2030. Verifying these payments accurately is crucial, and for transactions involving Mexico, that often means checking the CLABE code, a unique 18-digit identifier for bank accounts. New AI-powered technologies, including improved OCR systems, are changing how these CLABE codes are processed. These systems can analyze CLABE codes more quickly and potentially with fewer errors than human data entry, making transactions faster and potentially more efficient. Businesses that rely on Mexican bank transfers could see a noticeable impact with faster processing, fewer costly mistakes and possibly more secure transactions.
But there are questions. Can these new AI-driven systems really handle the diverse range of ways CLABE codes appear in bank documents? Will the OCR software perform well even when the documents are of low quality or handwritten? While the move toward more automated transaction processing through AI is a positive development for international business, it's still early days, and we need to consider the potential challenges in applying these technologies to real-world cross-border scenarios. Ultimately, this push for AI and enhanced OCR in cross-border payments is part of a wider trend: a drive towards making international transactions quicker, cheaper, and more transparent.
Cross-border payments are becoming increasingly important, and the ability to verify them accurately and quickly is vital. One crucial aspect, particularly when dealing with Mexico, involves analyzing CLABE codes, those unique 18-digit identifiers. It's been suggested that around 30% of banking errors stem from simple manual input errors, something AI-powered systems can help address. The current estimate is that these systems, using newer Visual OCR technologies, could potentially cut transaction processing times down to seconds, a huge improvement over the minutes it might take currently.
There is a growing interest in how these systems can learn and adapt. Essentially, these systems can improve themselves over time with more data, and that is a unique and powerful feature. This is a significant leap forward from older OCR systems that didn't possess this self-improvement ability. We can see that using neural networks for this purpose, the accuracy rate in reading CLABE numbers can go above 95%, minimizing the risks of mistakes that can happen with manual data entry.
Further, these kinds of systems can lead to some substantial cost savings. There's a prediction that businesses could see a drop in operating expenses by as much as 40% because they require less human labor and complete transactions much faster. There's also a more nuanced aspect: these systems don't just look for numbers, they can interpret the meaning of those numbers within a document's context. That contextual awareness may enable detecting suspicious transaction patterns more quickly, improving fraud detection.
The older OCR techniques weren't as flexible as these newer systems. These newer systems are more adept at handling different document types and quality variations which is incredibly useful when dealing with a range of customer-submitted bank statements. One researcher noted that these improvements in OCR could potentially decrease the chance of errors in transactions by up to 70%, a significant drop in risk. While the initial adoption is among the bigger banks, there's every reason to believe that smaller institutions will adopt them too. The hope is that this technology can create a more even playing field for the banking industry as a whole, something that's good for all businesses. It's still early days, but the pace of improvement in this technology is very encouraging, and we can anticipate an even greater impact in the coming years.
AI-Powered Translation Guide Understanding Mexico's CLABE Banking Codes for International Business Transactions - Language Processing Models for Spanish Banking Terms
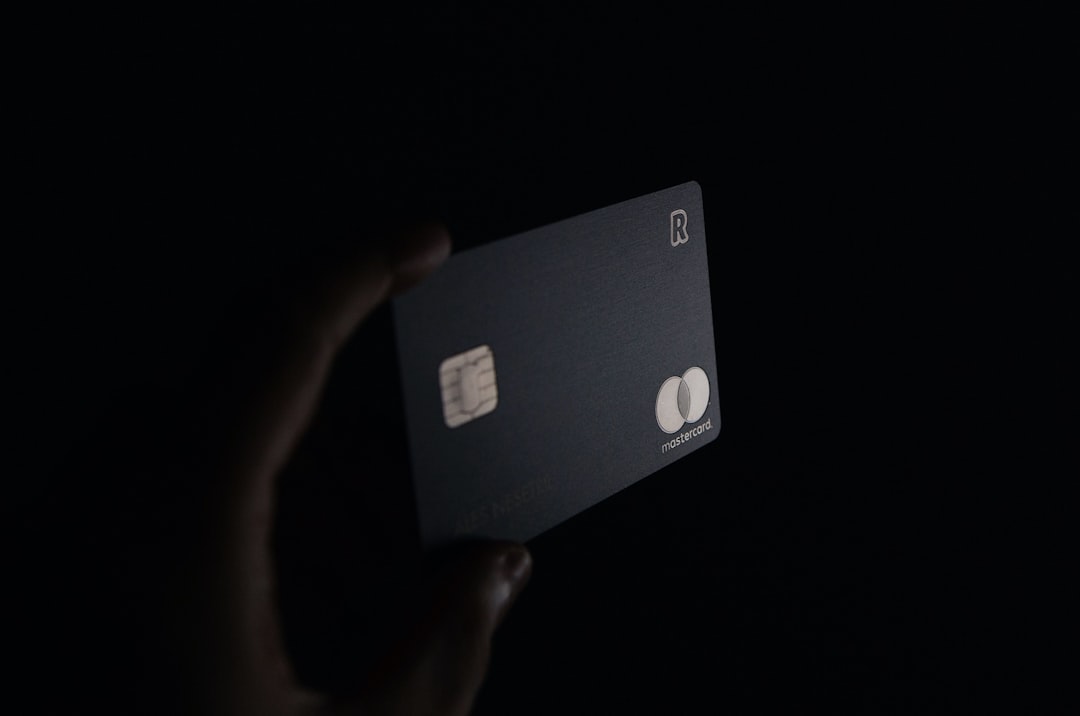
The field of language processing has seen significant advancements, particularly in its application to specialized domains like Spanish banking terminology. AI-powered translation tools, driven by large language models (LLMs), show promise in handling complex banking language. This is especially important for terms like Mexico's CLABE codes, critical for international transactions. These models offer potential for more accurate and efficient translations compared to manual processes, helping to reduce errors related to data entry.
Despite this progress, there are still some questions regarding the effectiveness of these models. For instance, how well do they adapt to variations in handwriting and the diverse range of document styles that are commonly encountered? It's crucial to carefully assess the potential limitations before fully embracing these tools. The banking industry's growing reliance on AI-driven solutions presents both exciting opportunities and challenges that require ongoing evaluation. Businesses looking to leverage these innovations need to consider how they can enhance operational efficiency while simultaneously safeguarding against any potential drawbacks that may arise as these technologies mature. Ultimately, the goal is to improve both the operational aspects of banking and the customer experience, and the careful implementation of these language models is vital for achieving these goals.
Language processing models, specifically large language models (LLMs), are showing promise for handling Spanish banking terms. These models, often powered by generative AI, can translate and interpret text, including banking-related conversations, with impressive accuracy. For example, models like GPT-3's Davinci have demonstrated the ability to summarize Spanish banking conversations with a 0.93 out of 1 score, without needing special training on that specific type of data. This indicates that general-purpose AI translation is making inroads in specific domains like finance.
The banking sector has been embracing AI and LLMs for various purposes, including improving customer service. AI-powered chatbots based on these models can provide initial responses to customer inquiries, though the quality of these interactions isn't always at a level suitable for all situations. However, LLMs are also beneficial for streamlining internal operations. By automating tasks like data entry and document processing, they can free up human employees to focus on more complex and valuable work.
One of the interesting aspects is the uneven adoption of these technologies. Some institutions are facing challenges implementing these models due to infrastructure limitations. This highlights a potential hurdle to broader adoption. Interestingly, efforts are underway to make AI integration faster and more accessible. For instance, new AI models specifically for banking are being developed with a goal of accelerating the AI implementation process from an average of 18 months down to a shorter time frame. Also, efforts like Meta AI's NLLB-200 model demonstrate a growing focus on multilingual AI capabilities. NLLB-200, being able to translate across 200 languages, shows the potential for wider accessibility.
Overall, the move towards integrating AI and LLMs in banking signals a trend towards AI-driven products and services. This includes tools for handling Spanish banking terms and processes. However, there are still some kinks to work out. The question of how these models will handle the complexities and nuances of the Spanish language, especially in a highly regulated industry like banking, remains a research topic. There's potential for improving speed and accuracy in areas like transaction processing and fraud detection, but this will require continuous refinement and evaluation of these models. As these technologies evolve, it's possible that they'll contribute to making international banking transactions simpler, faster, and potentially less costly, particularly for those dealing with Mexican CLABE codes.
AI-Powered Translation Guide Understanding Mexico's CLABE Banking Codes for International Business Transactions - Real Time Translation of Mexican Banking Documents Using AI
The use of AI in real-time translation is increasingly relevant for Mexican banking documents, especially those containing the CLABE codes crucial for global financial transactions. AI-powered translation tools are now able to quickly translate these documents while preserving the original formatting and context, which is critical for maintaining the accuracy and clarity of financial information. These tools hold the potential to accelerate the processing of Mexican banking documents, potentially decreasing errors linked to manual data input. Businesses working across borders with Mexican banks may see tangible benefits in the form of streamlined operations and risk reduction. However, it's important to note that the efficacy of these AI translation tools still relies on their capacity to navigate the variations in document quality and linguistic intricacies present in banking communications. As AI technology continues to refine its capabilities, it could lead to a more efficient and dependable system for handling international banking transactions, with a particular focus on the challenges and opportunities presented by the unique aspects of Mexico's financial system. The future may bring further improvements to speed and reliability, benefiting both companies and individual customers.
1. The potential for reducing errors in banking transactions is quite compelling. It's estimated that around 30% of issues stem from manual data entry. AI-powered tools like OCR are showing promise in significantly decreasing these errors, potentially by as much as 70%. This suggests that AI could substantially improve the reliability of bank transactions.
2. The sheer speed of modern OCR systems is impressive. These systems can now process thousands of transactions each second, which is a dramatic contrast to older methods that might take several minutes per transaction. This ability to rapidly handle CLABE codes, vital for Mexican banking, shows how AI is fundamentally changing banking operations.
3. Deep learning has enabled substantial leaps in the accuracy of these OCR systems. The most advanced deep learning models can now accurately read complex numeric codes like CLABE numbers with over 95% accuracy, a significant jump from older OCR systems that were often capped at around 80%. This indicates a new level of sophistication in these technologies.
4. AI-driven systems can now go beyond simply recognizing text and understand the context in which the text is presented. By being able to understand the meaning of numeric sequences within banking documents, these tools can flag unusual patterns that might indicate fraudulent activity. This capability is something that human operators would struggle with at such high speed.
5. The improvements brought about by AI lead to substantial cost savings. Operational costs are predicted to drop by as much as 40% due to the reduced need for human labor. This potential for cost reduction is a powerful incentive for financial institutions to consider adopting these technologies. The potential for redirecting these savings towards customer service or growth initiatives makes this an attractive option.
6. It's interesting that, while primarily adopted by larger banks for now, the potential benefits of these advanced OCR tools are applicable to smaller financial institutions as well. The wider availability of these technologies could make the banking landscape more competitive and ultimately lead to improved services for all customers.
7. AI-powered OCR systems have a significant advantage over traditional systems in their ability to adapt and improve over time. These systems are able to learn as they process more data, which means they continuously refine their accuracy and capabilities. This contrasts sharply with static systems that don't adapt over time.
8. While AI has led to massive improvements in image recognition, challenges remain, particularly in dealing with real-world documents. Poorly scanned or handwritten documents still pose issues for the technology. This suggests that there is still room for improvement in the adaptability of these systems to diverse document conditions.
9. The increasing reliance on cross-border payments, with projections reaching a staggering $290 trillion by 2030, makes AI-powered solutions critical. The ability to quickly and accurately verify CLABE codes, essential for many Mexican transactions, is crucial for improving efficiency and security in international finance. This is a domain where AI could play a crucial role in smoothing the flow of global commerce.
10. The use of AI extends beyond simply speeding up transactions. It can automate several tasks within banking operations, such as data entry and document analysis. By automating these functions, human employees can focus on more complex problems and activities. This potential for freeing up human resources could lead to fewer operational bottlenecks.
AI-Powered Translation Guide Understanding Mexico's CLABE Banking Codes for International Business Transactions - Machine Learning Methods for CLABE Code Error Detection
Machine learning techniques are proving valuable for identifying errors within CLABE codes, which are essential for Mexican banking and cross-border transactions. These methods, particularly deep learning approaches, analyze CLABE code structures to detect inaccuracies more rapidly and precisely than older, rule-based error detection techniques. With the growing need for speed and correctness in financial data processing, these machine learning advancements are a major step towards reducing the substantial portion of transaction errors (estimated at 30%) caused by human data input mistakes. While the potential for efficiency is clear, it's crucial to evaluate how well these systems cope with the diversity of bank documents found in practice, considering variations in handwriting, document quality, and other real-world factors. The combination of machine learning and error detection shows strong potential to enhance both transaction speed and accuracy, supporting the integrity of international financial dealings involving Mexico's banking sector.
Machine learning methods for identifying errors in CLABE codes hold the potential to dramatically reduce the number of mistakes in banking transactions. Estimates suggest these methods could cut errors by up to 70%, which is particularly important since about 30% of banking errors are caused by people making typos when manually entering CLABE numbers.
Modern OCR systems are being enhanced by incorporating advanced neural network technology. This allows them to process documents in a wide variety of formats, including different handwriting styles, far exceeding the abilities of traditional OCR methods that often struggled with variations in document quality.
The capability of machine learning systems to learn from each transaction as they happen is a key advantage. Unlike static OCR, they can continually improve their accuracy. Through this ongoing learning process, they can achieve reliability rates of over 95% in accurately recognizing intricate banking codes.
The shift in AI systems from simply recognizing patterns to understanding the context in which they are presented is significant. These AI-driven systems don't only check if a CLABE code is correct, but also examine it within the broader transaction information. This enables them to detect potentially fraudulent activity that older methods would overlook.
One of the most impressive aspects of these systems is the speed they offer. The expectation is that transaction processing times could be slashed from several minutes to just a few seconds. This drastic reduction in processing time is essential for businesses involved in high-volume, complex international banking scenarios.
The cost benefits are substantial as well, with the possibility of cutting operational expenses by up to 40% as manual processes are automated. This financial incentive could motivate more banks to adopt AI solutions to improve their operations.
While the advancements are remarkable, there are still challenges. For example, these AI systems can have issues interpreting low-quality or handwritten documents. Continued development will be necessary to ensure they can handle all kinds of data reliably.
The appeal of these machine learning approaches extends beyond large banks to smaller institutions too. As these technologies become more accessible, it may lead to a more competitive landscape and potentially better services for all customers.
By automating data entry and other tasks, machine learning frees up employees to focus on more complex tasks. This can improve operational efficiency and productivity across banking operations.
The applications of machine learning in CLABE code verification extend to improving the efficiency of international payments, which are anticipated to reach a staggering $290 trillion by 2030. The ability to verify these codes quickly and accurately will be vital in fostering a smoother and more secure global financial landscape.
AI-Powered PDF Translation now with improved handling of scanned contents, handwriting, charts, diagrams, tables and drawings. Fast, Cheap, and Accurate! (Get started for free)
More Posts from aitranslations.io: