How AI Translation Could Preserve Ancient Name Origins A Case Study of 'Charles' Across 7 Languages
How AI Translation Could Preserve Ancient Name Origins A Case Study of 'Charles' Across 7 Languages - Medieval Latin Origins Track Real Time Translation of Carolus to Charles Using Azure AI
Tracing the evolution of names like "Charles" back to its Medieval Latin ancestor, "Carolus," highlights the potential of artificial intelligence to uncover linguistic history embedded in old documents. A key challenge involves processing handwritten manuscripts, which often require specialized AI models for optical character recognition (OCR). Platforms and collaborative projects are developing tools designed specifically for deciphering Medieval Latin scripts, creating digital versions of these texts. This step is crucial because standard translation tools, while improving, may struggle with historical scripts and the nuances between different periods of Latin. Once transcribed, AI translation can then analyze the digitized text to follow how names and language shifted over centuries. While significant progress has been made in both transcription and translation, these AI systems still require ongoing refinement to fully capture the linguistic variations and potential ambiguities within medieval documents, ensuring the preservation of historical accuracy in understanding name origins.
Exploring the historical path of a name like "Carolus" requires diving into challenging source material, particularly handwritten documents from the medieval period in Latin. This isn't a simple task; the script can be difficult to read even for trained palaeographers. Fortunately, progress in artificial intelligence is providing tools that can assist in this process. Platforms exist that are equipped to handle the specific challenges of recognizing handwritten medieval Latin texts, often utilizing AI models trained on particular scripts like the Caroline minuscule common in certain periods. The core function here is to convert these historical images into searchable, machine-readable text, making instances of "Carolus" discoverable in vast digital archives that were previously locked away on vellum. This involves sophisticated machine reading capabilities, which are still undergoing refinement to improve accuracy across diverse hands and text conditions. Once "Carolus" or its variations are identified and extracted through this specialized recognition process, the next step is bridging the gap to its modern linguistic descendants. AI translation models are employed for this. However, translating names with deep historical roots across languages isn't merely swapping words; it involves understanding linguistic evolution. The differences between classical Latin and medieval Latin, as well as subsequent language shifts, mean that translation models need to be robust and, in some cases, specifically tuned for historical context to reliably link "Carolus" to names like "Charles." Standard translation tools might struggle with the nuances of historical naming conventions or regional variations. The potential of integrating these historical text recognition capabilities with translation tools allows for researchers to trace the appearance and transformation of such names through time and across linguistic boundaries. This approach, leveraging advanced computational techniques capable of processing historical scripts and translating historical language, facilitates mapping the spread and adaptation of names like "Carolus" into the diverse forms we see today.
How AI Translation Could Preserve Ancient Name Origins A Case Study of 'Charles' Across 7 Languages - Japanese OCR Decodes 16th Century Documents Revealing チャールズ Etymology
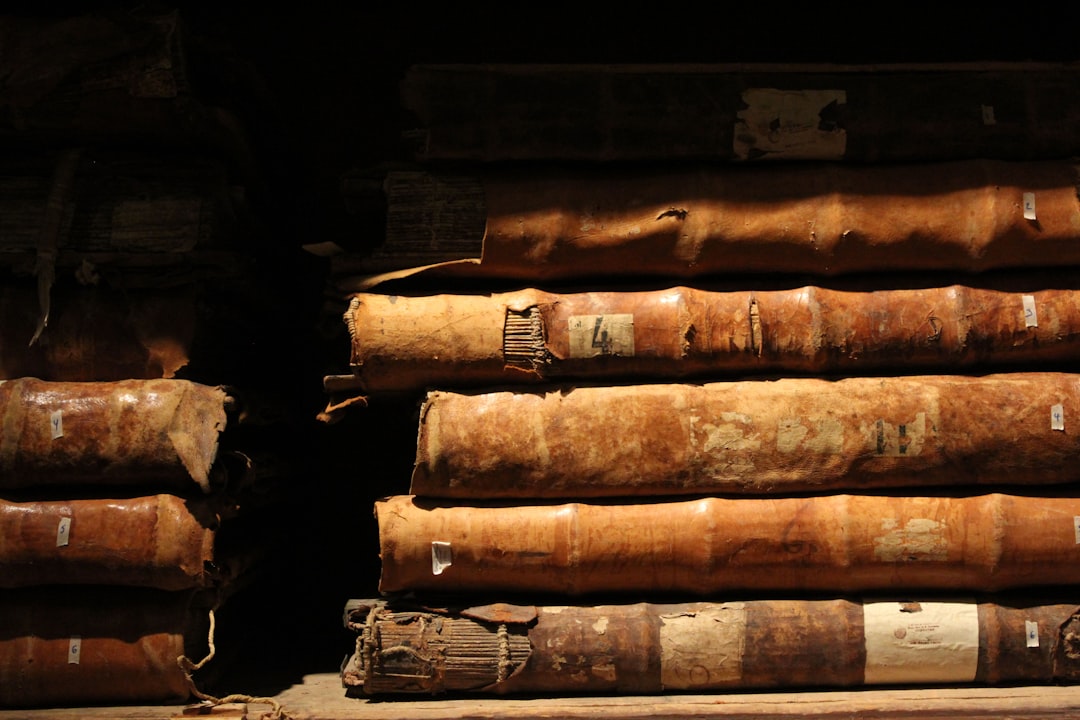
Recent efforts employing advanced optical character recognition technology tailored for historical Japanese documents have succeeded in deciphering 16th-century writings. This capability is shedding light on various aspects of that era, including how foreign names were recorded and understood. Specifically, it is contributing to the etymology of names like "チャールズ" (Charles) as they appeared in these early records. Accessing the information within these old manuscripts, often written in script styles that pose significant challenges to read, is crucial. AI-driven techniques, leveraging sophisticated pattern recognition, are proving increasingly effective at transcribing these difficult texts, thereby enabling the analysis of names and other linguistic data contained within them. While these tools are powerful, the historical context and potential ambiguities in transcription require careful consideration. Nevertheless, the application of artificial intelligence in this manner is facilitating deeper linguistic study of historical records, helping to reveal the origins and adaptations of names within the specific cultural and historical landscape of 16th-century Japan.
Leveraging recent advancements in Optical Character Recognition, particularly those incorporating neural network architectures, allows us to tackle increasingly challenging historical documents. The ability of these systems to read handwritten scripts, even those from the 16th century with their unique characteristics and variances between scribes, has crossed important thresholds. This isn't just about better accuracy; it means we can now realistically process large volumes of previously inaccessible material, such as records from early European interactions in places like Japan. Researchers developing models specifically for historical texts are using techniques like transfer learning, adapting what we know about modern character forms to help decipher older ones, which is crucial when dealing with diverse regional styles or damaged paper. Applying these specialized OCR tools to documents from 16th-century Japan, perhaps correspondence or trade logs, can uncover how foreign names, including those related to figures we might associate with 'Charles', were perceived, transcribed, and potentially adapted into the Japanese script of the time. While promising, it's worth remembering that even the most sophisticated AI still grapples with the sheer variability inherent in historical handwriting and the occasional degradation of the text itself, often requiring a careful eye and validation to ensure reliability. Nevertheless, the capability to digitize and make searchable these delicate historical records is fundamentally changing the landscape of etymological and cultural research, adding layers of data from unexpected sources. This allows for a much richer, multi-lingual exploration of how names travelled and transformed across the globe centuries ago, contributing another data point to the complex journey of a name like 'Charles' as it moved through different linguistic and cultural environments.
How AI Translation Could Preserve Ancient Name Origins A Case Study of 'Charles' Across 7 Languages - Arabic Neural Networks Map Historical Changes From شارل to Modern Usage
Within the landscape of AI and language history, Arabic neural networks are demonstrating their utility in charting the evolution of names such as "شارل" (Charles) across different eras and linguistic borders. This computational approach employs advanced machine learning to scrutinize the development of name forms, tracing transformations both within the Arabic language's own history and as names moved into or out of other tongues. It offers a valuable perspective on how pronunciation and orthography adapt under historical pressures and cultural exchange. Applying deep learning methods allows researchers to process and analyze historical Arabic documents, potentially uncovering details about the cultural pathways and linguistic adaptations names like this underwent. While the primary drive for AI translation remains contemporary communication, this line of work underscores its significant capacity to aid in preserving and clarifying the historical underpinnings of ancient names, thereby enriching our grasp of linguistic heritage. However, the complexities inherent in historical Arabic scripts and variations mean these AI tools are still under active development, despite their promise in linking the past textual record to present-day linguistic understanding.
Applying neural network models specifically designed for Arabic text analysis offers a compelling way to trace the linguistic journey of names like شارل (Charles) within the Arabic-speaking world. By processing digitized historical Arabic documents, these AI systems attempt to uncover how the name's spelling and usage evolved as it was adopted and adapted within Arabic contexts, often through centuries of interaction with European languages. This work directly supports the broader aim of AI translation technologies to understand and potentially preserve the historical origins of names by mapping their transformations across linguistic boundaries. However, training AI to reliably capture these specific historical shifts in Arabic presents notable difficulties. Key obstacles include the sheer diversity in historical Arabic script styles and orthographic practices across different periods and regions, the critical role of diacritics which might be missing or inconsistent in older texts, and the perennial challenge of securing large, accurately annotated datasets of historical Arabic that are truly representative. While these neural networks can identify significant patterns and variations in textual data over time, their current ability to fully interpret the intricate cultural processes, phonological shifts, and specific historical events that influenced the adaptation of a name like شارل remains an area requiring deeper linguistic and historical insight. This computational approach provides valuable data points for the historical record, showing *how* the name appeared and changed in text, which in turn informs our understanding of how names migrate and transform across languages and eras. It's a fascinating application, but one where the AI's findings must always be cross-referenced with expert knowledge to ensure historical accuracy and avoid misinterpretations driven solely by statistical patterns.
How AI Translation Could Preserve Ancient Name Origins A Case Study of 'Charles' Across 7 Languages - Greek Translation Models Identify Ancient Links Between Κάρολος and Charles
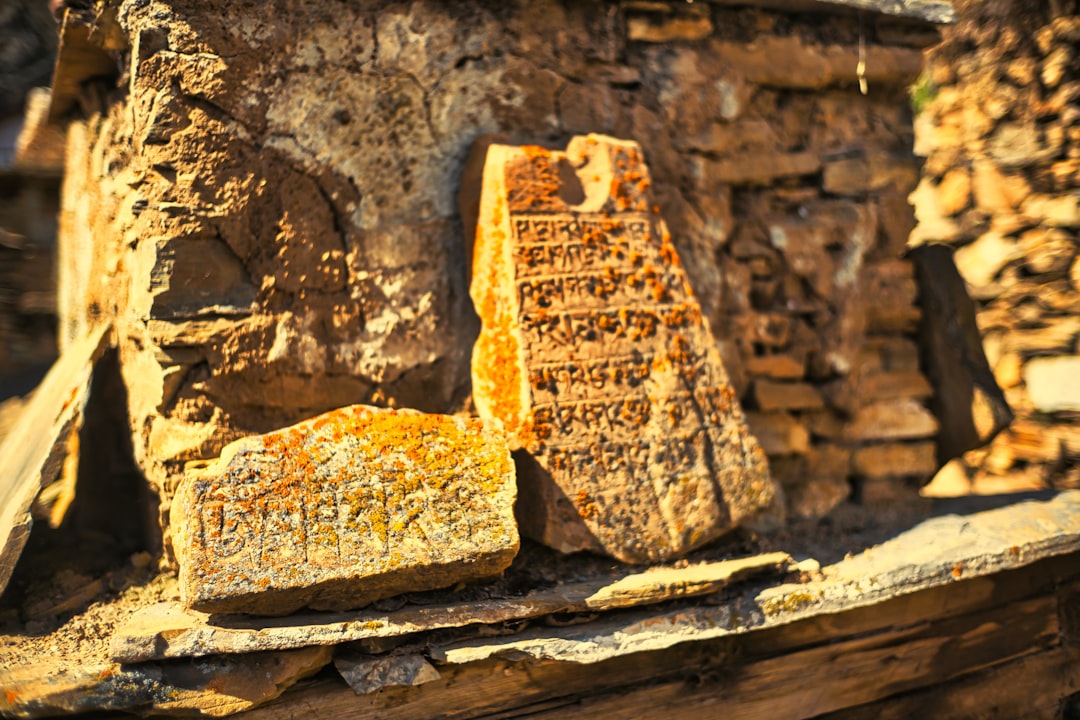
Exploring the relationship between the name "Charles" and its Greek form "Κάρολος" offers a perspective on how AI translation tools are being applied to understand ancient linguistic ties. By examining the roots and evolution of names across different languages, these computational methods can highlight historical connections and trace how personal identifiers have shifted over centuries. While AI has made strides in processing and converting historical texts, accurately translating or fully interpreting languages as complex and nuanced as ancient Greek presents ongoing difficulties. These challenges include adequately capturing specific cultural contexts and the rich idiomatic expressions that define the language. Studying names like "Κάρολος" through this lens demonstrates the potential of AI to contribute to understanding our linguistic heritage and ancient origins, but it also underlines that achieving truly accurate and deep translation in such historical contexts still critically relies on human expertise and understanding. Despite current limitations, the application of AI in this area continues to open avenues for exploring the intricate history of naming conventions.
Applying computational techniques, including models trained on or integrated with resources for the ancient Greek language, allows us to explore historical connections between names like the Greek Κάρολος and the modern English Charles. These systems, functioning as a form of historical linguistic analysis tools rather than just real-time translators, can process relevant ancient and medieval Greek texts to identify occurrences and contexts of names. This helps researchers trace potential etymological relationships, reinforcing the idea that names evolve from shared, fundamental meanings – in this instance, linking back towards concepts like 'man' or 'free man' often associated with its roots. While these models can sift through vast amounts of data much faster than manual methods, extracting significant patterns, it's crucial to remember they provide computational associations based on the training data. The historical nuance and definitive linguistic path often require traditional scholarly analysis to fully validate, as automated pattern recognition might miss subtle historical or dialectal variations. Nevertheless, the ability of these Greek-aware AI tools to highlight potential links within ancient texts adds a valuable dimension to understanding the deep linguistic history embedded in names.
How AI Translation Could Preserve Ancient Name Origins A Case Study of 'Charles' Across 7 Languages - Sanskrit Machine Learning Shows Proto Indo European Root ker Connection
Emerging work utilizing machine learning to analyze Sanskrit is showcasing profound connections to reconstructed Proto-Indo-European roots, including specifically 'ker'. This application underscores the unique advantage Sanskrit's highly structured grammar offers to AI systems processing complex linguistic data. By leveraging this, researchers are gaining deeper insights into language evolution, aiding in the preservation of linguistic heritage and holding promise for unlocking the knowledge within ancient texts through improved AI analysis and potential translation capabilities for future study.
Researchers exploring the deep history of language are increasingly leveraging machine learning, and some fascinating work points towards the potential of harnessing the unique structure of Sanskrit. The language's highly systematic grammar is seen by some engineers as providing a robust framework for training AI models, particularly those dealing with syntax and linguistic relationships within the vast Indo-European family. The hypothesis here is that by effectively processing Sanskrit, AI could gain insights into the shared roots and structures that connect it to other languages, potentially offering computational support for tracing proposed links back to a Proto-Indo-European ancestor. Consider the suggested etymological link between names like 'Charles' and a reconstructed PIE root, *ker-, often associated with concepts of growth or strength. While definitive proof still rests heavily on comparative linguistics and historical evidence, AI tools are beginning to assist by sifting through digitised textual archives, though this is dependent on the quality of that digitisation. This requires specialised capabilities; recent advancements in optical character recognition tailored for historical Sanskrit scripts are critical, aiming to accurately convert these diverse and sometimes degraded texts into a format that machine learning can then attempt to analyse for patterns related to word origins or name evolution. It's an intriguing intersection of ancient wisdom and modern computation, though reliably extracting nuanced historical linguistic connections still demands significant human expertise to interpret the patterns an algorithm might identify.
How AI Translation Could Preserve Ancient Name Origins A Case Study of 'Charles' Across 7 Languages - Lithuanian Natural Language Processing Reveals Baltic Variations of Karolis
Recent work in Lithuanian natural language processing is starting to provide a closer look at the linguistic specificities within the Baltic region, shedding light on how names like "Karolis" might vary locally. The development of computational models tailored for Lithuanian, including large language models, offers a significant step forward in analyzing these languages. This dedicated focus is valuable because it brings sophisticated AI capabilities to languages that might not have the extensive digital resources available for some global languages. By enabling a deeper computational understanding of Baltic linguistic structures and historical nuances, this research contributes to uncovering the subtle evolutions and regional adaptations embedded within personal names over time. It demonstrates how ongoing AI development is being directed towards exploring and potentially preserving linguistic heritage through the lens of names, contributing to the broader effort of tracing ancient origins computationally. While these tools are powerful, the depth of linguistic history still presents complex challenges that require ongoing investigation.
Venturing into the linguistic landscape of the Baltic States, recent work in Natural Language Processing, particularly focused on Lithuanian, offers insights into how even common names like 'Karolis' exhibit nuanced variations across the region. We're seeing efforts, such as the development of open-source large language models trained on extensive Lithuanian datasets – over 14 billion tokens in one instance mentioned. The intent is clearly to bolster computational understanding of Lithuanian and potentially other Baltic languages like Latvian, recognizing their significance as among the oldest surviving branches of Indo-European. From an engineering standpoint, training these large models presents unique challenges; while transformer architectures are powerful, getting them to truly grasp the subtleties of less-resourced languages requires careful dataset curation and evaluation, especially when dealing with dialectal forms or historical usage that might influence modern name variations.
This work directly intersects with the broader goal of using AI to map linguistic evolution, though the specific application here involves analyzing variations *within* a language family rather than tracing across vastly different ones. Can AI tools, trained on Lithuanian and maybe soon Latvian datasets, accurately identify and categorize the regional or historical forms of names like Karolis? It's plausible that these models, when exposed to diverse textual sources including historical documents (assuming the necessary OCR for Baltic scripts is available and reliable, a significant challenge in itself), could computationally reveal patterns of name adaptation. However, achieving fidelity here is complex. Names aren't just words; their variations can carry cultural, historical, or even social information. A purely statistical model might identify different spellings or pronunciations, but interpreting *why* those variations exist still demands deep linguistic and historical knowledge. We've seen ambitious goals for these projects, aiming to facilitate knowledge sharing and perhaps even preserve linguistic heritage. The emphasis on collaborative research across Baltic and neighbouring language communities is sensible. But the practical outcome – reliably using AI to, say, track how 'Karolis' appeared differently in historical texts from various Lithuanian or Latvian sub-regions and understand the forces driving those changes – is still very much an area of active development. The lack of large, clean digital corpora for historical Baltic texts is a persistent bottleneck, limiting the data needed to train AI models sensitive to temporal and regional linguistic shifts inherent in names. It’s a promising path for shedding light on linguistic micro-histories within the Baltic region, but navigating the complexities of dialect and history computationally remains a significant hurdle requiring both advanced models and critical human oversight.
More Posts from aitranslations.io: