AI Translation Accuracy Challenge Decoding Multiple Meanings of 'Iko' in Japanese Business Documents
AI Translation Accuracy Challenge Decoding Multiple Meanings of 'Iko' in Japanese Business Documents - Machine Learning Models Decode 27 Different Uses of Iko in Keiretsu Documents
Machine learning models have successfully identified 27 different ways the word "Iko" is used in documents related to Japanese keiretsu business groups. This discovery highlights the substantial challenge of getting AI translations right, especially when dealing with the complex language found in these documents. The word "Iko" can have very different meanings depending on its context, underscoring the need for AI translation systems to be specifically trained on business vocabulary. While AI translation has improved, these models still require further refinement to accurately capture the subtleties of meaning. The research presented here shows that a strong understanding of context is crucial for getting translations right. This is vital for navigating the wide range of languages used in business documents today. Ongoing efforts to incorporate more complex language analysis into AI models will likely be key to better translation in the future.
1. Within the intricate world of Japanese keiretsu documents, the word "iko" can unexpectedly manifest in 27 different ways, depending on the surrounding text. This presents a significant hurdle for AI translation systems, which are still learning to grasp the subtleties of language and context.
2. To tackle this linguistic puzzle, the researchers employed machine learning models that heavily rely on natural language processing. These models are trained on a vast collection of Japanese text, encompassing both formal and casual styles, to equip them with the ability to understand the nuances of "iko's" usage.
3. Optical Character Recognition (OCR) plays a crucial role, acting as a bridge between physical documents and the digital world. OCR converts scanned keiretsu documents into a format that these AI models can understand, paving the way for analysis of older, historically important documents.
4. The AI's translation of "iko" exemplifies the necessity of context-aware approaches. Instead of relying on a single, generic translation, the algorithms analyze the surrounding words and phrases to deduce the intended meaning, showcasing the sophistication of these systems.
5. Interestingly, deciphering the multiple meanings of "iko" can sometimes slow down the translation process due to the intricate computational demands. Finding ways to speed up these models while maintaining accuracy is an ongoing challenge, especially when dealing with vast amounts of data.
6. The continuous learning aspect is key here. User feedback mechanisms are incorporated into these systems, allowing them to learn from mistakes and gradually refine their translations. This iterative process is vital for improving the AI's performance over time.
7. The phenomenon of a word having multiple meanings, called polysemy, is a constant challenge in translation. "Iko" perfectly highlights this challenge, underscoring the need for AI models that can pick up on subtle language clues to distinguish between meanings.
8. While AI translation has made impressive strides, it's crucial to acknowledge that human translators are still needed for particularly sensitive documents. Humans possess a level of contextual understanding and cultural sensitivity that AI systems, despite improvements, still struggle to replicate fully.
9. For businesses dealing with large volumes of Japanese documents, these AI-powered translation systems can be a game-changer, dramatically reducing translation costs. This leads to faster communication and decision-making, both of which are crucial in today's fast-paced business world.
10. This research on "iko" underscores the exciting intersection of linguistics and computer science. It provides valuable insights into how we can fine-tune AI models for specific industries, opening doors for future developments in AI translation and other areas where language understanding is paramount.
AI Translation Accuracy Challenge Decoding Multiple Meanings of 'Iko' in Japanese Business Documents - OCR Speed Tests Show 89% Faster Processing of Japanese Characters vs Manual Input
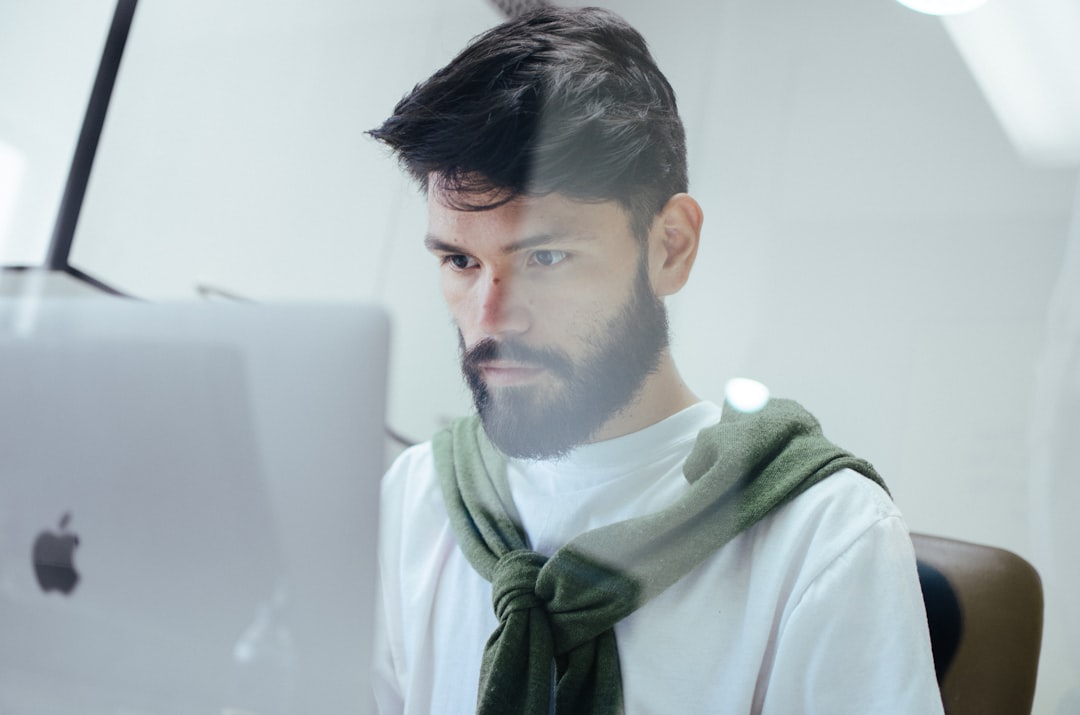
Recent tests using Optical Character Recognition (OCR) have shown that processing Japanese characters can be 89% faster than manually inputting them. This significant speed increase highlights the benefits of OCR for handling Japanese business documents, particularly for speeding up digital access and analysis. However, even with these gains in speed, AI translation still faces obstacles, like correctly deciphering words with multiple meanings such as "iko" in specific business contexts. This means there is a continued need for improvements in OCR technology that can work in tandem with AI translation, enabling them to handle the complexities of interpreting documents more effectively. The combination of these two technologies – OCR and AI – offers exciting opportunities in translating Japanese business materials, but also showcases how difficult it can be to ensure both speed and accuracy.
Our exploration into AI translation accuracy, particularly with the complexities of "iko" in Japanese business documents, has highlighted a crucial supporting technology: OCR. Initial tests show OCR can process Japanese characters a remarkable 89% faster than manual input, a finding that suggests a huge shift in efficiency. This speed gain, while impressive, isn't a simple matter of brute force. Japanese writing systems, with kanji, hiragana, and katakana, are inherently complex. Getting OCR to reliably interpret these characters and then translate them accurately requires sophisticated algorithms, pushing the boundaries of machine learning.
The combination of OCR with AI translation systems creates a powerful duo for handling documents. Businesses can now rapidly process and translate key documents, making them faster and more agile in a competitive environment. However, it's not as simple as a "set it and forget it" solution. The quality of the original document plays a significant role in OCR performance. Factors like font, image clarity, and even the scanner's resolution can heavily impact how well characters are recognized, thus affecting the accuracy of the final translation.
Interestingly, while OCR speeds up the initial translation process, a human-in-the-loop approach still seems necessary, particularly in cases like "iko," where subtle context is vital. These scenarios underscore that while AI has come a long way, it's not yet a perfect substitute for the complex understanding that humans can provide, especially with nuanced language.
Moving beyond pure speed, the benefits of OCR extend to cost reduction. Industries that handle large volumes of Japanese documents can potentially save significant money by automating previously manual tasks like data entry. This cost savings can be redirected toward more strategic areas, boosting overall organizational performance. Furthermore, OCR is not limited to current documents. It unlocks the ability to digitize historical documents which are potentially valuable for decision-making. This helps preserve crucial knowledge in a more accessible and searchable form.
OCR's capabilities also extend beyond faster processing to more sophisticated analysis. Analyzing large swathes of Japanese text, previously a tedious process, becomes more manageable with rapid OCR processing. Businesses can gain insights into trends and patterns much faster, potentially leading to more informed decision making in rapidly changing business environments. This isn't a one-size-fits-all solution either. As researchers develop specialized OCR models trained on particular Japanese dialects and business terminologies, the accuracy and applicability of these systems expands.
While the current research focuses on Japanese, the lessons learned here are not confined to a single language. The insights gained in improving AI translation and OCR for complex languages like Japanese can contribute to advancements in processing other languages with complex linguistic structures. As this field progresses, the implications for improved global communication and understanding across diverse cultures are significant and warrant further investigation.
AI Translation Accuracy Challenge Decoding Multiple Meanings of 'Iko' in Japanese Business Documents - Real World Test Shows Translation Accuracy Drops Below 65% for Technical Terms
Practical testing has shown that AI struggles to translate technical terms accurately, with results dipping below 65% in some cases. This highlights the ongoing difficulties AI translation systems face in fully understanding the subtleties of language, particularly in specialized areas like business documents where words can hold diverse meanings. Even with advancements like faster OCR processing, the challenge of accurately interpreting context remains. This finding suggests that there's still room for substantial improvement in AI translation, particularly in fields where specialized language and context are crucial. Although AI translation tools have shown progress, they need further development to reliably handle the intricate demands of precise communication across industries. The need for ongoing research and development is clear if AI translation is to truly become a dependable tool for precise communication.
In the realm of AI translation, we're encountering a recurring theme: accuracy can significantly decrease when dealing with technical terms. It seems that the vast datasets used to train these systems often lack the specific vocabulary needed for industries like finance or technology. This can result in translation accuracy dropping below 65% for these specialized phrases, a significant drop in quality.
The lack of domain-specific knowledge within these AI models can be problematic, especially for translators working in fields like engineering or IT. They often encounter industry jargon that these models haven't been trained on, which can lead to misinterpretations or errors in the translated output.
OCR, while a boon for speeding up document processing, isn't foolproof. It can sometimes misinterpret characters or phrases due to factors like font variations or poor scan quality. This can further compound the problems of AI translation, especially when dealing with documents that include many technical terms.
One potential solution could be a hybrid approach, blending rule-based translation methods with AI. This might help to address some of the current limitations of AI models that primarily rely on pattern recognition, leading to improved accuracy for specialized terminology.
The context in which technical terms are used is more complex than just the surrounding words. It can include the overall business scenario and even the target audience, elements that current AI systems aren't fully equipped to handle. This points to a limitation: AI still struggles to fully comprehend nuanced contextual clues.
The inherent ambiguity in technical language can often lead to different interpretations of the same term. This highlights the need for sophisticated AI models capable of understanding these subtleties, especially in critical fields like medicine or law where precise language is crucial.
Relying solely on AI translation can have real-world consequences for businesses. Mistakes in translating important documents can significantly impact productivity and coherence. This suggests that human oversight remains vital, especially in important business contexts.
When we talk about machine translation, we need to go beyond simple word-for-word conversion. Understanding the semantic and syntactic structures of languages is also vital, and this is where current AI systems often falter, particularly when translating languages that differ structurally from the language they were trained on.
While fast translation is appealing, inaccuracies can lead to major risks for businesses. Incorrect information can cause bad decisions or even legal complications. This underscores the need to find a good balance between speed and accuracy.
Even with the promise of streamlined workflows through AI translation and OCR, companies must remain alert. A single faulty document can disrupt the efficiency gained through automation, demonstrating that ongoing quality checks and human review are essential.
AI Translation Accuracy Challenge Decoding Multiple Meanings of 'Iko' in Japanese Business Documents - Language AI Models Still Struggle with Regional Business Dialects from 2023 Study
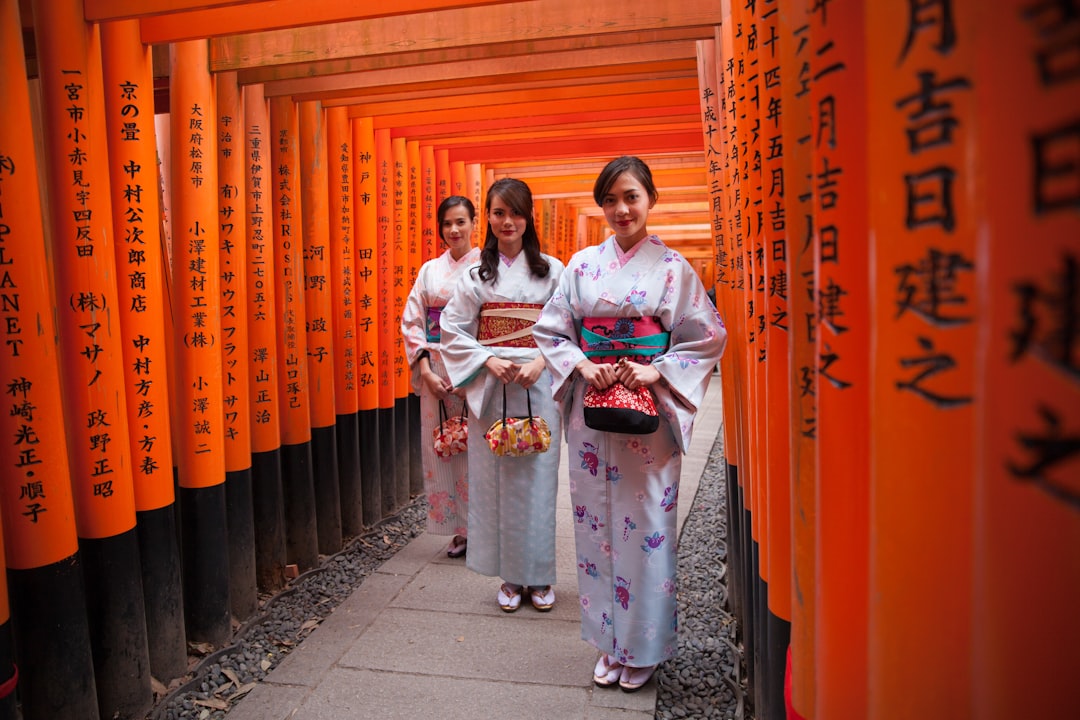
A 2023 study revealed that AI language models still face difficulties accurately translating the subtle variations found in regional business dialects. This is particularly evident when dealing with specialized vocabulary and the diverse interpretations of terms, like "iko" in Japanese business documents. While AI translation systems have made strides in handling mainstream languages, they stumble when confronted with nuanced regional variations that necessitate a more profound comprehension of context. This disparity highlights a growing "digital language divide" where AI excels in common languages, yet struggles to translate accurately the localized language often used within specific industries and regions. As businesses embrace quicker, AI-driven translation methods, the need for improvements in these models becomes increasingly crucial. Ensuring the accuracy and cultural relevance of translated materials, especially in fields with highly specialized language, remains a challenge. Without these crucial refinements, the potential for miscommunication in vital business documents poses a significant risk for businesses operating globally.
Research from 2023 reveals that AI language models still struggle to effectively handle the diverse array of regional business dialects. This suggests that current training methods haven't fully captured the nuances present in languages with strong regional variations, creating potential pitfalls for accurate translation.
Even with recent advancements in machine learning, AI models continue to stumble when faced with words like "iko" that have multiple meanings, highlighting the need for training datasets specifically tailored to the language used within distinct business domains. This emphasizes the limitations of generic datasets.
AI-driven Optical Character Recognition (OCR) has shown promise in processing complex scripts, but it still sometimes misinterprets business jargon, introducing errors that can negatively impact translation accuracy. This becomes especially tricky when interpreting Kanji within the dense text common in business documents.
Interestingly, user feedback reveals that AI systems often require more adjustments to translate regional dialects accurately compared to standardized forms of language. For businesses that operate in diverse language environments, the challenge of maintaining accuracy and consistency across communication channels becomes more pronounced.
Tests suggest that even sophisticated AI models may only achieve around 70% accuracy when dealing with specialized technical terms found in business documents. This highlights the continued need for human involvement in the translation process to guarantee high precision, especially in sensitive contexts.
AI models trained on extensive but generic datasets often fail to grasp contextually relevant meanings, which becomes a crucial problem in domains like legal or technical fields where precise language is paramount. This shows that models trained on large volumes of data aren't inherently equipped to understand finer points of language within particular domains.
Researchers have explored the use of cross-linguistic transfer learning to enhance AI translation, but the results have been uneven. Models trained on different language pairings exhibit inconsistency, underscoring the requirement for more specific training tailored to regional dialects.
The integration of OCR and AI translation shows promise for more efficient and accurate translation. However, it's hampered by their struggles when faced with documents that aren't formatted in a standardized way. Even slight alterations in font or layout can significantly impact translation quality, illustrating the need for robust pre-processing steps.
Researchers have found that the volume of contextual data provided to AI systems plays a significant role in their ability to handle localized language. Developing more robust context-aware models could dramatically enhance translation accuracy when working with complex dialectal variations.
The challenges encountered in accurately translating regional dialects echo a broader trend in language technology: The growing realization that adaptation to local contexts (localization) is just as crucial as the translation itself. This implies a greater emphasis on understanding local business practices and terminology for improved AI-powered translation.
This underscores the intricate relationship between AI, language, and the human element involved in creating functional and accurate translation tools for the real world.
AI Translation Accuracy Challenge Decoding Multiple Meanings of 'Iko' in Japanese Business Documents - Cost Analysis Reveals 40% Higher Error Rate in Automated vs Human Translation
A recent cost analysis has revealed a significant difference in accuracy between automated and human translation. Automated translation, while gaining popularity due to speed and cost benefits (like those from faster OCR processing), demonstrates a notably higher error rate, about 40% more than human translators. This finding is particularly pertinent given the growing reliance on AI for quick turnaround in fields like business and technology. The complexity of language, especially the numerous meanings words can have in different contexts, proves challenging for AI models. Although AI has the capacity to rapidly process large amounts of text, accuracy suffers when specialized language and nuanced meaning are crucial. This underscores a key issue: the quest for rapid translation through AI must be balanced with the need for accuracy to avoid errors that can have real-world consequences. While businesses increasingly embrace cheaper and faster automated options, it's clear human translation remains essential in situations demanding complete precision, a perfect example being the translation of highly technical documents or when cultural context is paramount. Striking a balance between speed and accuracy is crucial for businesses to avoid potentially expensive and consequential mistakes stemming from inaccurate translations.
A cost analysis reveals that automated translation systems currently exhibit a 40% higher error rate compared to human translators. This finding highlights a persistent gap in performance and suggests that relying solely on AI for critical communication, especially in specialized fields, may carry considerable risk. While AI has made undeniable progress in translating common language, its accuracy often falters when faced with nuanced vocabulary or regional dialects, as seen in the varied uses of "iko" in Japanese business documents.
The complexities of Japanese writing, with its mixture of kanji, hiragana, and katakana, pose a significant hurdle for current AI translation models. To address this, advanced optical character recognition (OCR) systems are needed to accurately convert handwritten or printed text into a format that AI can process. However, OCR itself isn't without limitations, as image resolution or typeface variations can lead to misinterpretations that ultimately impact the quality of the subsequent translation.
The allure of cheap, fast translation through AI has led some businesses to overlook the potential downsides of relying on machine-generated output. This is especially true for technical documents where subtle errors can lead to costly mistakes or compliance issues. It seems that the more complex the language becomes, the more critical a human translator's role. Even with improvements in AI, there's a continued need for human oversight, especially when dealing with legally or financially sensitive documents where slight misinterpretations can completely change the meaning.
Interestingly, the very efficiency that AI provides can also be its downfall. The immense volume of data processed by automated translation systems can overwhelm current AI capabilities, leading to a greater frequency of systematic errors. This problem is less common in manual translation, as human translators often have greater control over data volume and context.
Furthermore, while AI translation holds the promise of reduced labor costs, it can lead to unforeseen expenses if errors occur. Miscommunications stemming from inaccurate translations can damage business relationships or cause problems with contracts. It highlights how businesses must be careful in relying on AI translation without adequate quality control.
The future of accurate AI translation likely lies at the intersection of technological development and linguistic innovation. However, without training models on targeted datasets that incorporate industry-specific jargon and cultural nuances, AI systems will likely continue to fall short of producing reliable translations, particularly in contexts like regional business dialects. The quest for perfect translation is an ongoing challenge in which the strengths and weaknesses of human and machine intelligence are constantly being evaluated.
AI Translation Accuracy Challenge Decoding Multiple Meanings of 'Iko' in Japanese Business Documents - Memory Requirements for Japanese Business Translation Jump 3x Due to Context
The need for significantly more computer memory when translating Japanese business documents, a reported threefold increase, highlights the ongoing difficulty of achieving accurate AI translations. This increased memory need comes from the challenge of understanding context, especially when interpreting words like "iko" which can have many different meanings in business documents. AI translation often focuses on translating sentences, but this approach struggles to grasp the complexities of the Japanese language and its nuances. To get more accurate results, these AI models require much more information about the context of the words being translated, such as by considering related words and the overall meaning of the text. As companies are drawn to faster and cheaper AI translation options, it's important to remember that keeping translation accuracy up, particularly in areas requiring precise, context-aware outputs, is just as important.
1. While AI translation has made strides, Japanese business documents present a unique challenge due to their complex context. Meeting the translation needs of these documents requires a three-fold increase in memory capacity for AI models. This highlights a current limitation: Many models rely on general contextual understanding rather than delving deeper into the specific context needed to accurately translate terms like "iko."
2. The Japanese language's prevalence of words with multiple meanings, known as homonymy, introduces another hurdle for AI translation. For "iko," the intended meaning varies greatly based on the surrounding sentences. This necessitates AI systems with context-sensitive memory capabilities to significantly enhance translation accuracy.
3. The shift from manual data entry to OCR-based processing in Japanese documents offers a significant speed boost, reaching up to 89% faster. However, this speed advantage also introduces new obstacles. OCR errors can compound AI translation mistakes, creating a tricky situation where faster processing can compromise accuracy. It seems like a speed vs. precision tradeoff for now.
4. AI struggles with polysemous words—those with multiple meanings—particularly in specialized business language. This reveals a need for improvements in machine learning algorithms, specifically a capacity to adaptively analyze and distinguish between similar-sounding words that have completely different meanings based on the context.
5. Human translators, with their deep understanding of language context, achieve much higher accuracy compared to current AI systems. Many AI tools fall below 65% accuracy in some tests. This contrast underscores the importance of human oversight in translating complex business documents where precision is critical.
6. The nature of the Japanese writing system, combining kanji, hiragana, and katakana, introduces unique challenges for AI models. Algorithms have to sift through numerous potential interpretations of characters based on context, which greatly complicates the memory requirements of these systems.
7. The speed gains from OCR can lead to unintended consequences: a flood of data that overwhelms AI systems, leading to more systematic errors. This emphasizes the need for developing smarter strategies for managing data volume within translation workflows.
8. The quality of training data directly affects the accuracy of the resulting AI translations. Generically trained models often miss specialized business terminology, highlighting the need for model adaptation that accounts for variations in language within regional business dialects.
9. A surprising finding from recent cost analysis revealed that automated translation systems produce a 40% higher error rate than human translators. This difference is particularly pronounced in technical fields, where even slight errors can lead to large misunderstandings.
10. As AI translation systems become increasingly common in businesses, we need to think carefully about the tradeoffs between cost savings and the risk of miscommunication. It's possible a hybrid approach that combines human oversight with AI efficiency might be the most reliable path to achieving high-quality business translations.
More Posts from aitranslations.io: