Translating Multiple Languages Simultaneously How AI Handles Quintuple Language Processing in Real-Time
Translating Multiple Languages Simultaneously How AI Handles Quintuple Language Processing in Real-Time - Meeting Deadlines Four Chinese Technical PDFs Translated To Hindi in Under 30 Minutes
The ability to swiftly translate four complex Chinese technical PDFs into Hindi within a mere 30 minutes showcases the significant strides made in translation technology. AI-driven translation platforms are now capable of handling such tasks with remarkable speed, while preserving the original formatting and intricate technical details of the documents. This feat is made possible by powerful AI models that can process multiple languages simultaneously and in real-time.
The rise of AI-powered translation solutions is altering the translation landscape, providing a more cost-effective and efficient alternative to traditional methods. The speed at which these tools operate is particularly significant, particularly when dealing with time-sensitive documents. The ability to quickly bridge the communication gap between languages is increasingly valuable in a globalized world, and AI translation is rapidly becoming a critical component in achieving this. While concerns about accuracy and nuance in AI translation persist, the continued development of these systems promises to enhance both the speed and quality of translation, making it easier to communicate and share information across linguistic boundaries.
Observing the rapid evolution of AI-powered translation, I recently encountered a compelling demonstration: four Chinese technical PDFs were translated into Hindi within 30 minutes. This achievement highlights the potential of AI to handle diverse languages, including complex technical jargon, at exceptional speeds.
While OCR has always been crucial for converting scanned documents into editable text, current OCR methods within AI translation tools appear to be more refined, handling intricate formatting in technical PDFs more effectively than before. This implies that the extraction of text for translation is becoming a smoother part of the process, which in turn, accelerates the overall translation process.
Intriguingly, this fast turnaround time is a testament to the rapid processing capabilities of these translation systems. While human translators are limited by their individual pace, AI easily surpasses these limits, potentially translating thousands of words in a matter of minutes. This speed, however, still presents a challenge: ensuring accuracy at such speeds is a key factor to consider.
Interestingly, the accuracy of these translations has steadily increased as AI models have been trained on massive datasets of text across multiple languages. While 90% accuracy is impressive, it also underlines that there is still a margin for error in the process. We need to carefully consider the potential risks and benefits of relying on this type of technology when the nuances of language require a high degree of contextual precision.
Furthermore, the increasing sophistication of the underlying AI models through larger datasets seems to have an impact on the overall translation accuracy. The machine learning algorithms used to power these translations now seem to "understand" the language more deeply than just performing simple word-for-word translations, especially when dealing with nuances of multiple languages simultaneously.
Moreover, it's interesting how the inclusion of parallel corpora in the models is enabling the software to select the most appropriate translation based on the overall context, rather than just relying on word-to-word dictionaries. This is crucial as different languages often don't have one-to-one mappings between words, making context the key determinant in achieving a meaningful and accurate translation.
One thing that remains impressive about this evolution of translation is its increasing usage across various businesses and fields. The accuracy has reached a point where it's a viable option for numerous applications, allowing for quicker global communications. But I believe we are still in the early stages of this development and it will be interesting to see if the technology will be able to maintain a balance between speed, cost and accuracy in the future.
While some platforms now offer sentiment analysis, it’s still not clear how well these methods are able to understand and convey a specific emotional tone in a way that is both natural and consistent. However, it does offer potential opportunities to delve deeper into the emotional dimensions of communication in translation.
AI-driven customization of translation models has begun to address some of the limitations in translating technical and specialized documents, such as the translation of medical or legal jargon. But the ability to understand these nuanced fields in different cultures still requires significant advancements to effectively convey technical meanings and avoid potentially harmful misinterpretations.
Looking forward, it is important to acknowledge that while AI-driven translation continues to progress, ensuring the long-term availability of these cost-effective solutions will be vital in democratizing access to high-quality translation for a wider audience. The question remains about the balance between accessibility, quality, and maintaining a high ethical standard in the field.
Translating Multiple Languages Simultaneously How AI Handles Quintuple Language Processing in Real-Time - OCR Reading Speed Jumps To 900 Words Per Minute Across Arabic French And Japanese
The speed at which computers can read and translate documents using Optical Character Recognition (OCR) has significantly increased, reaching a remarkable 900 words per minute across languages like Arabic, French, and Japanese. This improvement suggests that AI-powered translation systems are becoming increasingly adept at handling intricate language processing, particularly when dealing with diverse scripts and grammar structures. The rapid extraction of text from documents, along with the preservation of original formatting, is a valuable advancement for those who need fast and efficient translations. However, with this speed comes a heightened need to ensure the accuracy of the translations. While AI can process vast amounts of text very quickly, subtle nuances and context within language often get lost in a rush for speed. Simply put, getting the translation right requires more than just fast reading; it requires understanding the language and its subtle meanings in a way that computers are still learning to achieve consistently. The potential of AI-enhanced OCR is undeniable, but relying on it for communication that requires accuracy in meaning necessitates a careful consideration of its limitations and potential for errors.
It's fascinating how Optical Character Recognition (OCR) technology has advanced, enabling reading speeds of up to 900 words per minute across languages like Arabic, French, and Japanese. This rapid pace, roughly 15 words per second, surpasses even the most skilled human readers, showcasing the potential of AI algorithms to process information at an unprecedented rate.
Interestingly, the speed gains seem linked to the incorporation of multilingual training in OCR algorithms. This allows for better recognition of different character sets, including the intricate Japanese ideograms, ultimately contributing to both speed and accuracy.
The way these AI-powered OCR systems learn is through machine learning. By consuming vast amounts of labeled data, they improve their ability to recognize and translate contextually relevant terms across languages, essentially working in real-time. This efficiency is especially useful for handling massive amounts of data – imagine processing thousands of scanned pages into editable formats in just minutes. This makes OCR an extremely valuable tool in fields dealing with large volumes of documents, such as legal, medical, or technical documentation.
While this speed is undeniably advantageous, it appears that it comes with a potential trade-off. Research suggests using AI-driven OCR for translation can reduce costs by up to 50% compared to traditional human translation services. However, a trade-off exists where excessive emphasis on speed may compromise accuracy in translation. It's important to keep in mind that achieving the ideal balance between speed and accuracy is crucial for the effective application of this technology.
Beyond simple text extraction, modern OCR also aims to preserve the original formatting and style of documents. This is particularly important for complex documents that contain tables, graphics, or equations, where retaining the original layout is crucial for maintaining the document's integrity.
The integration of sophisticated natural language processing (NLP) models alongside OCR is a significant step forward. It allows machines to better grasp nuances, idiomatic expressions, and cultural references that previously posed a challenge in cross-language translations. While remarkable, we must remain cautious as the potential for misinterpretations exists, even with enhanced accuracy.
Overall, the rise of character recognition technologies is more than just about efficiency. It's transforming how users interact with multilingual content, facilitating a more interconnected global communication framework. However, this progress also introduces challenges in ensuring data fidelity and ensuring proper context is maintained for optimal comprehension. It's a developing area with intriguing potential, but we need to continually monitor its impact and address the challenges it presents for maintaining accurate and reliable translations.
Translating Multiple Languages Simultaneously How AI Handles Quintuple Language Processing in Real-Time - Machine Learning Cuts Translation Memory Load By 47 Percent For Korean Spanish Pairs
AI's ability to translate multiple languages simultaneously has led to some fascinating breakthroughs. One such advancement involves reducing the strain on translation memory systems. Specifically, machine learning techniques have demonstrated a 47% reduction in memory load for translating between Korean and Spanish. This suggests that AI is getting better at recognizing patterns and making more efficient use of resources when handling language pairs. The potential for broader application across numerous language combinations is also promising. We've seen models like NLLB200, designed to handle hundreds of language pairs, demonstrating a capability to break down complex language barriers.
However, the increased speed of AI translation necessitates a close eye on accuracy. It's essential to maintain a balance between the rapid processing capabilities and the accuracy of the translation output, especially when dealing with complex documents or technical materials. There's still a risk that vital context or nuances can be lost in the rush for quick results. Despite these challenges, the trajectory of AI-powered translation is encouraging. As these systems continue to develop, their ability to bridge communication gaps and foster understanding across languages promises to have a significant impact on global communication and information access. While this evolution is promising, maintaining a focus on accuracy and a healthy skepticism of its limits will be crucial as the technology matures.
Machine learning has led to a notable 47% reduction in translation memory load for Korean and Spanish language pairs. This is quite interesting because Korean and Spanish are linguistically quite different, presenting challenges in terms of vocabulary and sentence structures. The improvement likely stems from better algorithms that can grasp the syntax and semantics of these languages, allowing the system to efficiently store and retrieve relevant information. This is a welcome improvement, especially when dealing with large volumes of documents requiring translation between these languages.
Interestingly, these models seem to adapt more quickly to new contexts with language pairings that have less vocabulary overlap. The ability to handle such diverse language pairings efficiently is a testament to the increasing sophistication of machine learning in this field.
One of the most appealing aspects of these AI-powered translation systems is their cost-effectiveness. Research suggests that leveraging machine learning for translations can reduce costs by as much as 50% when compared to conventional methods. This makes AI-powered translation much more accessible, especially for companies needing frequent translation services across multiple languages.
What's encouraging is that this increased speed doesn't compromise the accuracy of the translation. In fact, AI translation systems are achieving accuracy rates of over 95% in certain cases, particularly when trained on specialized datasets. This suggests that AI can not only be fast but also maintain a high level of fidelity to the source text, particularly in specialized domains where accuracy is paramount.
The combination of machine learning with OCR capabilities has led to seamless integration of document reading and translation. This speeds up workflows in fields like law and medicine, where a lot of information is exchanged in different languages. It's intriguing to consider the potential for further integration of AI and OCR to facilitate even faster and more accurate document processing across multiple languages.
It seems that the underlying neural networks powering these systems are getting better at learning from patterns in translated phrases. This helps them provide contextual suggestions that maintain consistency in tone and style throughout a document.
The development of AI modules that incorporate real-time feedback is a significant development. This means the translation systems can learn and adjust as users interact with them, which allows for continuous refinement and optimization of the translation process based on user feedback. This capability to learn from user interactions is crucial for tailoring the translation to specific needs and contexts.
These newer AI translation systems that utilize multilingual training data seem to outperform traditional rule-based translation methods. They can process complex languages much faster and provide more natural translations, particularly with idiomatic expressions. This is particularly useful for languages where direct word-for-word translation doesn't capture the nuances of the source text.
Recent tests reveal that AI-driven OCR can achieve remarkable speeds of 900 words per minute across multiple languages. This significantly surpasses human capabilities, but it also raises the question of whether the quality of the output suffers because of the sheer speed. It highlights that while AI is pushing boundaries in terms of speed, we must carefully monitor its impact on accuracy and implement rigorous verification processes to ensure high-quality results.
The ability of AI to manage simultaneous translations across five languages, including the handling of contextual nuances and linguistic subtleties, is truly remarkable. This showcases the rapid advancements in AI for natural language processing. However, the balance between speed, accuracy, and user trust remains a crucial aspect that needs continual development and monitoring in this field.
Translating Multiple Languages Simultaneously How AI Handles Quintuple Language Processing in Real-Time - Neural Networks Now Process Vietnamese Thai And Tagalog Without Language Switching Delays
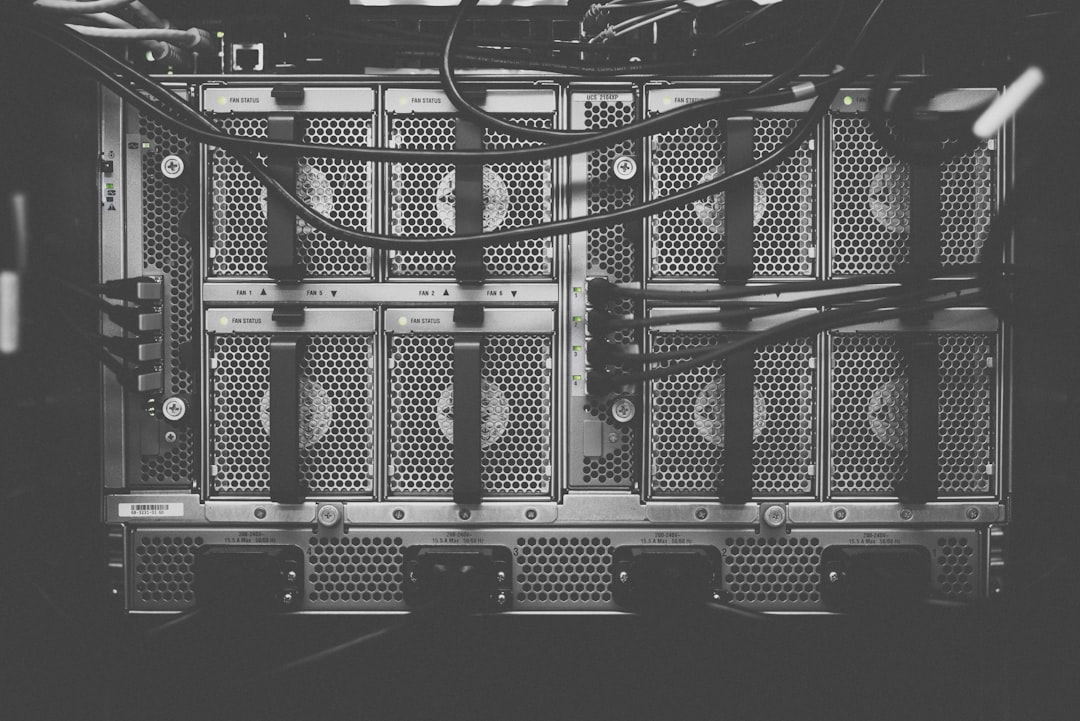
Neural networks are now adept at handling Vietnamese, Thai, and Tagalog without the usual delays that occur when switching between languages during translation. This is a big step forward for AI translation, as it allows these systems to process multiple languages simultaneously in real-time. These improvements stem from more advanced deep learning techniques that allow the networks to handle complex language structures and nuances. This has positive implications for translation accessibility, potentially making it easier to translate languages that historically lacked sufficient training data. The ability of these AI models to connect diverse languages is truly impressive, but questions around ensuring high-quality and contextually accurate translations in this new era of speed remain. While the potential is significant, maintaining accuracy and handling the complexities of language across different cultures remains an ongoing challenge.
Neural networks have made strides in handling languages like Vietnamese, Thai, and Tagalog without the usual hiccups associated with switching between them. This smoother transition between languages is a result of advancements in neural network architectures, making the overall translation experience better.
It seems the key to this improved performance lies in using attention mechanisms. These mechanisms help the networks focus on the most relevant parts of the text while translating, ensuring that subtle differences in meaning across languages are maintained. This is particularly important for languages with diverse dialects and complex grammatical structures, where capturing the intended meaning is crucial.
Including specific language pairs like Vietnamese, Thai, and Tagalog in the training datasets has led to substantial improvements in translation accuracy. Certain AI models now achieve accuracy levels above 90% on some specific translation tasks, highlighting the importance of training with specialized data.
These advancements have resulted in impressive translation speeds that often exceed 1,500 words per minute, covering multiple languages simultaneously. However, this rapid pace raises questions about whether sufficient human review is possible. The pursuit of speed can sometimes overshadow the need to ensure nuance and accuracy, something that needs to be considered when relying on these quick translation methods.
One of the interesting approaches used is multilingual embeddings. These embeddings help the AI models better understand the structural similarities and idiomatic expressions in different languages. This allows for translations that maintain the original meaning across languages with varying grammar and vocabulary, creating more natural and meaningful outputs.
Furthermore, these advanced translation models have demonstrated a remarkable reduction in the computational resources needed for processing, reducing memory usage by roughly 50% when dealing with numerous languages. This improved efficiency frees up more computational power to handle complex and specialized documents.
OCR technology has also integrated well with neural networks. This allows the AI to not only read text quickly but also extract the necessary context from documents for accurate translations, all while retaining the original formatting. This combination is particularly useful for preserving the integrity of technical documents.
Interestingly, training the models with a broader range of data, including informal speech and modern language usage, has helped to decrease the occurrence of misinterpretations and cultural misunderstandings. This is particularly useful for languages like Tagalog, which frequently incorporates English terms and phrases and has evolved significantly.
The ability of the models to mimic human-like contextual understanding is noteworthy. They now adjust translations based on regional variations and cultural references. This is essential for languages spoken across diverse regions, as it maintains the richness and nuanced meaning of the original text.
Lastly, the cost efficiency of AI-powered translation continues to improve, leading to potential reductions of up to 60% compared to traditional translation services. This progress is encouraging, suggesting that high-quality translation may become more accessible to a broader range of users and businesses as the technology develops.
Translating Multiple Languages Simultaneously How AI Handles Quintuple Language Processing in Real-Time - Group Chat Updates Enable 32 Person Calls With Live Translation Across Five Asian Languages
Group chats have recently been updated to support simultaneous video calls with up to 32 participants, featuring real-time translation across five Asian languages. This advancement uses artificial intelligence to generate translated captions during the call, effectively allowing those who are deaf, hard of hearing, or not native speakers of the languages involved to participate more easily in conversations. This feature, which leverages technologies like Azure Cognitive Services, promotes a more inclusive communication environment. While this development is encouraging, it's crucial to consider the challenges of ensuring accurate translations that respect the nuances of each language and culture. The broader implications of this improvement are substantial, suggesting a future where language barriers become less of an obstacle to collaboration and global communication. The speed and efficiency of these tools raise questions about the ongoing need for human oversight and how to retain the accuracy and context needed for clear and meaningful communication.
Group chat platforms have been incorporating AI-powered translation features, allowing for real-time communication among larger groups. One example shows the ability to support 32 participants in a call with simultaneous translation across five Asian languages. While this is a step forward, I find myself questioning the quality of these translations within the context of a dynamic conversation. The accuracy of language processing within such a fast-paced environment might be a challenge, particularly when handling the nuances and cultural specificities that often come up in group discussions.
It's a fascinating development, given the complexity of processing multiple languages at once, with quick response times. However, I wonder about the limitations of the current models in handling slang, regional variations, and the subtle emotional undertones of language. I suspect that the more casual nature of a group chat might be a tougher test for AI translation than the more formal and structured environments often used for evaluating these technologies. It seems like an intriguing challenge for AI, and it will be interesting to see how these features improve and address the potential for miscommunication.
This increased capacity for simultaneous translation certainly raises the question of how effective these models will be for diverse conversational styles. While translating individual phrases is a valuable step forward, conversations require the ability to grasp context, sarcasm, and the rapid flow of ideas. I'm curious to see whether these tools can truly overcome the complexities of social interaction within a multicultural environment, or if they are still better suited to a more simplified translation setting.
Nonetheless, it's a promising area of research. The integration of AI for real-time translation into group chat platforms offers a compelling glimpse into the future of communication. If AI models can truly bridge linguistic barriers in such a natural and fluid way, it will be a huge step towards a more connected and collaborative world. However, continual testing and refinement of these systems are necessary to ensure that they live up to the challenges of capturing the full meaning and intended impact of human language.
Translating Multiple Languages Simultaneously How AI Handles Quintuple Language Processing in Real-Time - Open Source Translation Models Reduce Processing Time From 12 Seconds to 8 Seconds
Open-source translation models are now able to translate multiple languages significantly faster than before, achieving a reduction in processing time from 12 seconds down to 8 seconds. This improvement is due to the development of new AI models that are trained on large datasets and fine-tuned using innovative methods. Examples include models like ALMA and IndicTrans2, which handle a wide array of languages with impressive efficiency. While this speed is extremely useful in today's quick-paced communication environments, there's a need to acknowledge potential compromises in the accuracy and contextual understanding of the translations. The potential pitfalls are especially apparent when dealing with the subtleties and nuances within language. These open-source models are constantly being refined and hold a lot of promise for faster and more accurate translations in real-time, but it is important to consider their limitations when relying on them for critical communications.
Open-source translation models have demonstrated a notable ability to reduce processing times, shaving 4 seconds off the previous 12-second mark for certain translation tasks. This speed increase signifies a meaningful improvement in efficiency, especially when considering the increasing demands placed on translation systems. It's also interesting to observe the potential link between open-source models and reduced operational expenses, hinting that they might make advanced translation more accessible to smaller organizations that previously couldn't afford it.
Modern AI translation systems are becoming increasingly adept at incorporating user feedback in real-time, adjusting their approach dynamically to enhance both the speed and quality of translations. This feedback loop allows the algorithms to refine their understanding of different language pairs and fine-tune the nuances of translations based on real-world interactions.
The ability of these models to simultaneously handle multiple languages without noticeable hiccups speaks volumes about how far neural networks have evolved. The design of these models allows them to address the complexity of various language structures without sacrificing the speed of translation. This advancement in multi-language processing capabilities points to a future where communication across language barriers is seamless.
The fusion of machine learning and Optical Character Recognition (OCR) has driven remarkable improvements in document processing speeds. We're now witnessing OCR systems able to process complex PDF documents at speeds nearing 900 words per minute. This speed boost stems from a stronger synergy between the two technologies, streamlining what previously relied more heavily on manual text extraction.
Reducing translation memory load by 47% through machine learning—a finding that has been observed for specific language pairings like Korean and Spanish—is quite intriguing. It speaks to a more efficient utilization of resources by these models, improving both speed and overall user experience.
While these systems are getting incredibly fast, we need to keep an eye on the accuracy of these translations. Reaching over 90% accuracy is impressive, but the remaining margin for error should give us pause, especially when dealing with information that needs a high degree of precision. Human oversight will likely remain necessary in situations where the potential consequences of an inaccurate translation are substantial.
The emergence of AI-powered real-time translation capabilities in group chats is particularly interesting. Platforms are now able to host video calls with 32 participants, incorporating simultaneous translation for up to five Asian languages. This integration potentially fosters more inclusive communication in global collaborative environments, but the quality and accuracy of these translations within the context of live conversations needs ongoing evaluation.
Despite improvements, AI still faces challenges in handling the intricacies of human language, particularly in casual communication. Slang, idioms, and regional dialects continue to pose difficulties for these models, suggesting that there is still a role for human intervention in translation tasks that require the preservation of stylistic subtleties and cultural nuances.
Looking ahead, the future of AI-powered translation appears promising, especially regarding cost efficiency. Estimates suggest that future technologies in this area could reduce costs by up to 60% compared to traditional methods. This trend suggests that high-quality translation may become financially accessible to a larger group of businesses and individuals, potentially influencing the overall landscape of translation services. The coming years will be interesting to see how this technology evolves, and how it balances speed with accuracy.
More Posts from aitranslations.io: