AI-Powered PDF Translation now with improved handling of scanned contents, handwriting, charts, diagrams, tables and drawings. Fast, Cheap, and Accurate! (Get started for free)
AI Translation Embracing New Languages with Open Arms
AI Translation Embracing New Languages with Open Arms - NLLB200 Model Translates 200 Languages with High Accuracy
The NLLB200 model represents a major stride in AI's capacity to bridge linguistic divides, achieving high-accuracy translation across an impressive 200 languages. This development is particularly notable for its focus on languages often overlooked by traditional translation systems, ensuring that speakers of less-common tongues are not left behind. The model's proficiency underscores the power of advanced AI in language processing, offering a potential solution to the growing need for fast and accurate cross-lingual communication. However, the effectiveness of such models in real-world scenarios with their complex nuances and dialects remains an area that needs continued research and refinement. Nevertheless, projects like NLLB200 illustrate the ongoing shift towards more inclusive and accessible AI translation, a trend that promises to reshape how we communicate across the globe.
The NLLB200 model is a notable advancement in machine translation, boasting the capability to translate between 200 languages. One intriguing aspect is its ability to deliver relatively high accuracy, even for languages with limited available data. Historically, these "low-resource" languages have been a challenge for AI translation systems, often resulting in less accurate results. This model tackles that limitation using a variety of clever techniques.
It employs a method called multilingual training, where the AI model learns from multiple languages concurrently. This approach allows it to recognize and utilize common patterns across different language families, leading to improved general translation quality. Further bolstering its performance, NLLB200 incorporates techniques like back-translation and data augmentation, essentially creating synthetic training data. This helps the model better capture the nuance and context within the text.
Beyond that, the model showcases a capacity to process more casual language, something that is often problematic for standard AI translation systems. Think slang, internet language, or regional dialects. This makes it more applicable to genuine everyday interactions. Furthermore, the model does a better job than its predecessors of capturing sentence structures and grammatical patterns specific to different languages. This means it's not just spitting out a literal word-for-word translation but one that makes sense in the target language, taking context into account.
Speed is also a focus. For tasks requiring real-time translation, the NLLB200 model can deliver outputs in a matter of milliseconds. This opens up applications in fields like automated customer service or live captioning events across a broad range of languages. Interestingly, its development also seems to have minimized some of the biases that had plagued older models. This is thanks to refinements in training that prioritize fairness and equal representation.
Looking forward, one fascinating possibility is its ability to integrate with Optical Character Recognition (OCR). This means the model could potentially translate text captured from images. Imagine the possibilities for accessing and translating historical documents, official records, or even everyday signs and notices. It's important to remember though that this is an ongoing area of development and refinement. The model is able to learn and refine its translations with user feedback, which is crucial in today's world of rapid linguistic changes.
While it's impressive in its current capabilities, NLLB200 is also a reminder that translation is still a complex field. The challenge of handling idiomatic expressions and cultural nuances that don't have direct equivalents across languages remains. This points to the ongoing need for research and innovation in the world of natural language processing.
AI Translation Embracing New Languages with Open Arms - FLORES200 Dataset Measures AI Translation Performance
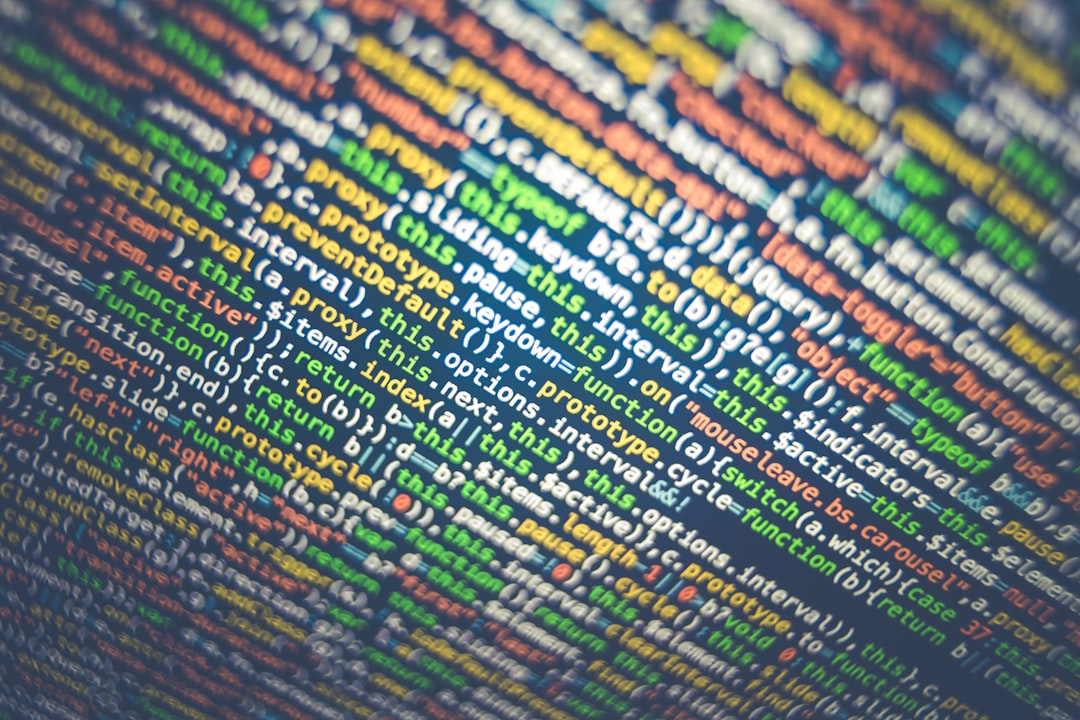
The FLORES200 dataset represents a substantial step forward in gauging the quality of AI translation, especially for systems dealing with multiple languages. It significantly expands upon its earlier iteration, FLORES101, by encompassing a much broader range of languages, including those with less formalized structures. This broadened scope allows for a more comprehensive assessment of AI's capabilities across 40,000 different language combinations. It's particularly valuable for evaluating the performance of AI in translating what are often called "low-resource" languages, which are known to be challenging for current translation systems.
The dataset's utility is clearly demonstrated when used alongside the NLLB200 model. The results have shown noteworthy gains in translation accuracy, particularly for languages spoken in parts of Africa and India. This underscores the ongoing progress in AI's ability to make translation more accessible and sophisticated. Despite these advancements, the intricate details of dialects and cultural contexts continue to be areas requiring further investigation and development. While AI translation is demonstrably making strides, fully grasping the nuances of human communication remains a complex goal.
The FLORES200 dataset is a valuable tool for evaluating how well AI models translate across a wide range of 200 languages. It's quite important because most existing datasets tend to focus on a smaller group of common languages, making FLORES200 essential for improving translations in less commonly used languages.
A key feature of FLORES200 is the use of high-quality human-translated text as a benchmark. This gives us a much more accurate way to assess AI-generated translations, highlighting areas where models struggle with specific language pairs that are often missed by older datasets.
Beyond simply translating words, FLORES200 prioritizes understanding context and nuance. It recognizes the challenges posed by idiomatic expressions that often confuse traditional translation tools, which makes the translation models trained on FLORES200 more suitable for real-world use.
The dataset includes a diverse mix of text types, from formal documents to informal chats, forcing AI models to adapt to various communication styles. This pushes developers to build more versatile systems, rather than those solely trained on formal language.
Developers who created FLORES200 also put a lot of effort into reducing bias in translations, which is important as previous models sometimes unintentionally reinforced stereotypes or inaccuracies. This focus on fairness is crucial for the responsible development of AI translation.
Furthermore, FLORES200 lets researchers test language pairs that haven't received much attention from translation technology in the past. This can spur the development of specialized machine learning techniques for these languages, bringing a wider range of people into the global communication sphere.
When it comes to speed and efficiency, models trained using FLORES200 are often evaluated on their quick output times, a critical factor for applications like real-time customer support or live translation at public events.
The dataset's primary focus on low-resource languages is important because it provides a standard for improving translations that are usually hindered by limited data availability. This is vital for ensuring that people from different linguistic groups have equal access to communication technology.
With its structured approach to measuring AI translation performance, FLORES200 has become a benchmark for future datasets. This encourages more rigorous testing of machine translation systems and highlights areas that need improvement.
Lastly, the ability to use FLORES200 with various machine learning frameworks creates opportunities for collaborative research. It allows developers to more effectively innovate and refine algorithms for different contexts and languages.
AI Translation Embracing New Languages with Open Arms - SeamlessM4T Released Under Research License for 100 Languages
Meta's SeamlessM4T is a new AI model that can translate and transcribe between nearly 100 languages. It's a significant step forward in AI translation, handling various tasks like translating speech to text, speech to speech, and text to both speech and text. It's been trained on a massive dataset of over a million hours of audio and text, giving it a solid foundation for accurate translations. Importantly, Meta has made it available under a research license, encouraging other researchers and developers to experiment and improve upon its capabilities. Reports indicate it outperforms existing translation systems in certain areas, suggesting a major improvement in the speed and accuracy of AI translation. SeamlessM4T's potential to become a versatile, unified translation tool is promising, though it's still early days in determining how well it handles the complex nuances of real-world language interactions. This release signals Meta's ongoing effort to enhance AI's ability to facilitate communication across a wider range of languages, hopefully leading to more accurate and accessible communication globally.
Meta's recent release of SeamlessM4T, a multilingual and multitask AI model, under a research license is quite interesting. It can handle a wide range of tasks, including speech-to-text, speech-to-speech, text-to-speech, and text-to-text translation across nearly 100 languages. This broad support is particularly notable as it potentially addresses a major gap in the field: ensuring translation access for less-common languages.
What makes it stand out is its ability to work across different modalities – speech and text – which could significantly reshape how people interact and communicate across language barriers. It's encouraging to see an attempt to address the challenge of underrepresented languages, often overlooked in past AI translation projects. SeamlessM4T uses a huge dataset with a blend of human-labeled and automatically labeled data, likely using innovative self-supervised learning techniques to maximize its learning. This could potentially lead to better and more refined translations, especially in those languages that traditionally have limited training data.
A key consideration is its claimed real-time performance, which is critical for applications like live interpreting or instant translation during video calls. Interestingly, it seems to achieve this while being designed to be sensitive to cultural nuance and context. It has also been touted as potentially capable of working with OCR, which could be huge for applications involving historical documents or image-based translation. That said, it remains to be seen how well it handles the complexities of dialects, idioms, and cultural specificities that often make language translation a thorny challenge.
The researchers have also acknowledged the risk of bias in AI translation models and appear to have implemented measures to reduce its impact in SeamlessM4T. It's also worth highlighting that it can learn and refine its translation abilities over time using user feedback. Finally, the rigorous evaluation and benchmarking approach used with this project are likely to pave the way for creating more robust and sophisticated translation quality benchmarks in the field.
SeamlessM4T represents an ambitious step in the evolution of AI translation and offers a glimpse into the future of cross-lingual communication. While its efficacy needs to be tested and refined in diverse contexts and scenarios, it offers a valuable resource for researchers and engineers hoping to push the boundaries of AI's capacity to comprehend and bridge language differences.
AI Translation Embracing New Languages with Open Arms - AI Addresses Gap in Popular Translation Tools for Lesser-Known Languages
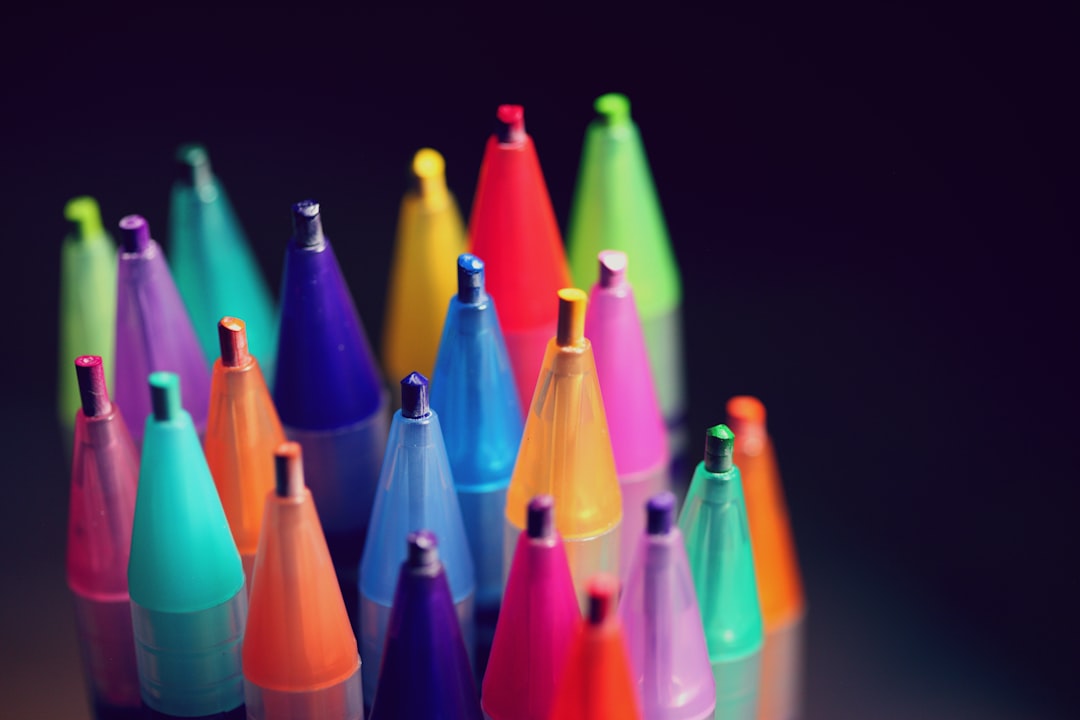
AI is increasingly adept at tackling the limitations of traditional translation tools when it comes to lesser-known languages. The advancements in machine learning and natural language processing have allowed AI systems to bridge the gap, particularly for languages that historically lacked sufficient data for accurate translations. These AI systems are leveraging large datasets that include a wider range of languages, enabling them to learn and adapt to the nuances and complexities of less common tongues. The emphasis on capturing cultural nuances and dialects is vital for creating more accurate and contextually relevant translations. This effort is often fueled by collaboration with communities, incorporating local knowledge into AI model development. Furthermore, the increasing investment from tech companies in this area suggests a broader movement towards creating more inclusive translation technology. This trend is fostering global communication by ensuring that individuals who speak less common languages have better access to translation tools, potentially leading to a more equitable communication environment. While there are challenges that remain in accurately representing the intricate aspects of languages, the progress in AI translation is promising for the future of global communication.
AI's ability to translate languages spoken by even a few thousand people is significantly expanding access to communication for smaller linguistic groups that were largely ignored by earlier translation tools. This increased scope is due to advancements in AI that enable models to simultaneously handle translation tasks for diverse languages, leading to faster processing and a better grasp of context.
A clever trick being used is the creation of artificial training data for languages with limited readily available data. Through techniques like back-translation, AI systems can generate synthetic data that enhances the accuracy of translations. This is particularly useful for languages with small speaker populations.
Furthermore, we're now seeing AI translation in real-time applications like international conference calls. This facilitates immediate communication across different languages without frustrating delays. There's also the exciting potential of combining AI translation with Optical Character Recognition (OCR) for deciphering printed materials like ancient documents or signs on the street. Imagine being able to understand any sign in your native tongue instantly!
Researchers are working to make AI translation more culturally sensitive. This involves designing algorithms that take into account the complex cultural contexts surrounding language, potentially minimizing miscommunication that can result from a purely literal translation. These models are rigorously tested using comprehensive datasets that include a wide variety of dialects and variations in speech. This ensures that the models don't just handle textbook language but can also cope with the dynamic and ever-evolving nature of communication.
A fascinating aspect of these newer systems is their capacity to learn from user feedback in real-time. This continuous improvement process allows them to adjust to changing linguistic trends and improve accuracy over time. Moreover, there's an increasing awareness of the potential for bias within these models, which has sparked efforts to design them in a way that minimizes potential unfairness, especially for translations of culturally sensitive topics.
These developments are creating new challenges for AI researchers. We now need benchmarks and metrics that accurately capture a model's performance across the vast array of languages and their specific nuances. Developing these evaluation frameworks that truly reflect the complexities of linguistic diversity is vital for the field to progress. This push to translate languages with as few as a thousand speakers is a testament to the growing understanding of the crucial role that AI can play in promoting linguistic inclusivity and global communication.
AI Translation Embracing New Languages with Open Arms - M2M100 Enables Direct Translation Between 100 Language Pairs
M2M100 represents a noteworthy step forward in AI translation because it can directly translate between any two of its 100 supported languages without needing to go through English as an intermediary. This model, developed by Facebook AI, deviates from the usual English-focused approach in machine translation. The result is a significant boost to multilingual communication with the ability to handle almost 10,000 different language pairs. Its open-source design encourages a wider range of people to experiment and improve the model, which is especially helpful for languages not often included in translation technology. However, M2M100's performance can vary based on the amount of high-quality training data available for a given language. This raises questions about its overall consistency in practical use cases. The ongoing development of models like M2M100 highlights the continuous push for more accessible and inclusive translation tools, but it also highlights the challenging nature of perfectly capturing the intricate details of diverse linguistic and cultural environments.
M2M100, initially unveiled in 2020, is a significant development in AI translation, focusing on direct translation between 100 language pairs. Instead of relying on English as an intermediate step—a common practice in many translation systems—M2M100 can translate directly from one language to another, potentially leading to more accurate and nuanced results, especially in scenarios where context is critical. This model, created by Facebook AI, is a departure from the traditional English-centric approach, aiming to democratize translation for a wider range of languages.
One of the key aspects of M2M100 is its ability to adapt quickly to new languages, even those with limited readily available data. It achieves this using techniques like transfer learning, effectively leveraging what it's learned from other languages to gain an initial understanding of new ones. Furthermore, to address the challenge of scarce training data for less common languages, M2M100 utilizes a fascinating approach of creating synthetic data. Techniques like back-translation are used to generate artificial sentences, essentially expanding the training pool and allowing the model to improve its understanding of these languages.
Interestingly, M2M100's architecture is geared towards speed, making it potentially suitable for real-time applications. This is important because the demand for instant translations is growing rapidly in areas like live events, customer interactions, and international collaboration. It's not simply about translating words; M2M100 is designed to consider cultural context and idiomatic expressions. This is crucial for avoiding misinterpretations that can arise from literal translations.
The creators of M2M100 were conscious of the biases that have been documented in older translation systems. Consequently, they incorporated methods to identify and mitigate potential biases within the model itself. This is a crucial step towards ensuring fairer translations, particularly when dealing with culturally sensitive subjects. Another intriguing aspect of M2M100 is its potential to be integrated with Optical Character Recognition (OCR) technologies. This capability could unlock a wide range of applications, such as translating historical documents, images, and signs.
The model is built for ongoing learning and refinement. It can adapt and improve based on feedback from users, ensuring the accuracy of its translations increases over time as the model is exposed to new examples and linguistic changes. To ensure its performance meets certain standards, it can be tested against high-quality human translations, allowing researchers to identify specific areas for improvement. The model's architecture is also designed to scale efficiently, meaning that it can handle expanding datasets and an increasing number of languages without losing performance. This is important as the demand for translation expands globally.
Overall, M2M100 stands out as a forward-looking initiative, offering a new approach to AI translation. While further research and refinements are likely needed, it's exciting to see an attempt to build a system that can directly support a large number of languages without relying on a dominant language as a bridge. This approach, combined with the model's adaptability, speed, and bias-mitigation capabilities, could contribute to a more inclusive and globally accessible communication environment.
AI Translation Embracing New Languages with Open Arms - Google's 1,000 Languages Initiative Aims to Expand AI Translation Reach
Google's ambition to expand AI translation to encompass 1,000 languages is a significant development in the field. Their "1,000 Languages Initiative" highlights a push towards wider accessibility in communication, with a recent milestone being the addition of 110 new languages to Google Translate. This expansion leverages the advanced capabilities of their PaLM 2 language model, which employs sophisticated AI techniques for improved translation accuracy and nuance. The initiative seeks to make translation tools more inclusive by bringing in a wider array of languages, including ones like Afar, Cantonese, and Manx. This reflects a commitment to breaking down language barriers that can often hinder communication across diverse communities. While this is a positive step, the effectiveness of these AI models when dealing with intricate cultural context and various dialects remains an ongoing challenge. The quest for refining AI translation to capture the full spectrum of human communication is a complex endeavor that necessitates continual development and refinement. Ultimately, Google's efforts are part of a broader movement aimed at making information more accessible and fostering communication across the world's languages.
Google's 1,000 Languages Initiative is a significant undertaking aimed at making AI-powered translation accessible for a vast majority of the world's languages, including many that have historically been underserved by traditional translation systems. This is particularly interesting because AI translation models have struggled in the past with languages that have a limited digital footprint. It's a big step toward making communication more inclusive for communities that haven't always had access to these technologies.
The technical challenges involved are immense, given the vast differences in language structure and available data. To address this, Google has adopted a range of sophisticated machine learning techniques. These approaches include transfer learning, which enables the AI model to learn from data in more common languages and adapt it to those with less training data. They're also utilizing various unsupervised learning methods that allow AI to extract meaning from language even when explicit labels aren't readily available. This approach is crucial for those languages where the readily available digital resources are limited.
Beyond just increasing the number of languages, they're also placing a lot of emphasis on the speed of translation. The goal is to develop models that can translate languages nearly instantaneously, making them usable in real-world situations like online meetings or live events where rapid communication is critical. This is a major advancement compared to traditional methods, which were much slower.
Perhaps one of the more unexpected elements of the initiative is its integration with Optical Character Recognition (OCR). This adds the capability of translating text from images, such as scanned documents or even signs and notices on the street. This potential to access a wide variety of visual content opens up a whole new avenue for applications.
One of the key challenges is the scarcity of data for many of the languages targeted by this initiative. Google has implemented a clever strategy for this using a technique called data augmentation. They generate artificial data to enhance the training process and build more robust language models. This is crucial to ensure that translations are accurate and reliable for even those languages that are underrepresented online.
Another significant factor in this area is addressing potential bias within AI models. Biases can lead to skewed or inaccurate translations, particularly when it comes to culturally sensitive topics. Google is aware of this risk and has taken steps to minimize it, focusing on creating a more equitable communication experience. They're paying attention to the diversity of the training data they utilize to minimize the risk of any unfair biases that might unintentionally emerge in the translations.
This initiative isn't just limited to text translations either. Google's ambition is to support multimodal translation capabilities as well, covering both written and spoken languages. This allows for more intuitive and complete interaction in a diverse range of languages.
Finally, ongoing learning and improvement are essential to keep up with the dynamism of language use. Google plans to integrate feedback loops where users can contribute to refining the AI models, allowing for continuous adaptation and improvement over time.
The practical applications of this technology are wide-ranging. From facilitating international business deals to easing communication in emergency situations, this initiative has the potential to make a big difference in how people across the globe connect with each other. The goal of supporting real-time translation in such a diverse linguistic landscape offers the possibility for significant impact across numerous fields, including global health, humanitarian aid, and international diplomacy. These potential uses indicate the broad influence of AI translation in our interconnected world. While challenges remain in accurately representing all nuances of human communication, Google's ambitious initiative stands as a testament to the potential for AI to break down language barriers and promote a more interconnected world.
AI-Powered PDF Translation now with improved handling of scanned contents, handwriting, charts, diagrams, tables and drawings. Fast, Cheap, and Accurate! (Get started for free)
More Posts from aitranslations.io: