AI Translation Precision Converting Micrometers to Microinches in Technical Documentation
AI Translation Precision Converting Micrometers to Microinches in Technical Documentation - AI-Driven Precision Enhances Micrometer to Microinch Conversions
The realm of precise measurement, particularly in fields like electronics and nanotechnology, is increasingly reliant on accurate conversions between micrometers and microinches. AI's role in refining this conversion process is becoming increasingly important. While the basic conversion—one micrometer equates to roughly 393,700 microinches—might seem simple, the potential for human error during manual conversion in complex technical documentation remains. AI, through its ability to learn and apply intricate algorithms, can dramatically minimize those errors.
This AI-powered approach offers a significant advancement for industries that demand pinpoint accuracy. By automating and optimizing the conversion process, AI systems can streamline workflows, especially in technical writing and documentation where precision is paramount. Ultimately, faster and more dependable conversion between these measurement units is made possible, leading to a new level of reliability within technical documentation, a factor crucial in numerous fields where exact measurements are vital. There's still a need to see how readily this technology can be integrated and if its potential benefits will truly translate into widespread adoption within the various sectors that require it.
AI's foray into precision engineering has brought about a fascinating shift in how we handle unit conversions, particularly when dealing with micrometers and microinches. While the conversion factor itself remains constant, AI algorithms can now perform these conversions with remarkable accuracy, exceeding 99.9% in certain instances. This surpasses the capabilities of traditional manual methods, which are vulnerable to human errors, especially in complex and repetitive tasks.
The speed with which AI processes these conversions is also a significant benefit, especially in fields like aerospace or microfabrication, where real-time accuracy is paramount. The computational prowess of these systems allows for instantaneous conversion, a luxury not readily available with manual approaches. It's interesting to note that the costs associated with these AI-driven systems can be offset over time. Their machine learning capabilities allow them to learn and refine their accuracy as they process more data, potentially reducing the need for extensive manual calibration and verification.
Furthermore, AI's integration with other technologies like OCR allows for the automated extraction of numerical data from technical documents. This eliminates the time-consuming and error-prone process of manual data entry. And with the aid of natural language processing, AI can decipher the context within technical documentation to ensure unit conversions adhere to industry-specific norms. This context-aware approach can be particularly helpful when translating documents across different technical fields.
The implications of these advances extend beyond simple conversion accuracy. Integrating AI into precision conversion processes has demonstrably improved productivity within engineering teams. Engineers are freed up from the burden of manual calculations, allowing them to devote more time to higher-level tasks like innovative design and problem-solving. Moreover, some AI-powered solutions leverage cloud environments, giving them access to vast datasets and the ability to continuously optimize their algorithms without imposing a heavy computational burden on local systems. This cloud-based approach facilitates scalability and adaptability across a wider array of industrial applications.
The benefits don't stop at efficiency. AI translation solutions, in conjunction with precision conversions, can operate seamlessly across multiple languages, simplifying communication and ensuring consistency in technical documentation across international collaborations. Interestingly, researchers have observed that AI systems are also adept at recognizing and rectifying anomalies within measurement data, which enhances the overall reliability of the conversion process and reduces the risk of potentially disastrous outcomes in applications where even minor discrepancies could have major consequences. The synergy between AI translation tools and advanced sensor technologies holds particular promise, leading to even higher accuracy in micro-level measurements and potentially catalyzing breakthroughs in industries reliant on precision engineering. This area of study is ripe for exploration and holds the potential for further exciting developments in the future.
AI Translation Precision Converting Micrometers to Microinches in Technical Documentation - Automated OCR Speeds Up Technical Document Processing
Automated OCR (Optical Character Recognition) has become a vital tool for speeding up the handling of technical documents. Its ability to convert scanned or printed documents into editable, searchable text significantly reduces the time needed for data entry, making the process much faster. Human error, a common issue in manual data entry, is minimized with automated OCR. This efficiency is particularly beneficial in industries or businesses where large volumes of technical documents need processing, freeing up workers to focus on more complex tasks.
Moreover, modern AI-powered OCR systems are adept at tackling a wider variety of document formats, such as low-quality scans or documents with unusual fonts. They are able to achieve high accuracy in recognizing and extracting text, making them incredibly valuable. It's becoming increasingly clear that incorporating OCR into a business's workflow not only makes things more efficient but also strengthens their ability to make decisions based on data. However, while OCR shows immense promise, it's important to remain mindful of the potential limitations, such as accuracy inconsistencies when dealing with very complex layouts or unusual symbols. Despite these limitations, OCR remains a promising and rapidly developing technology within document processing.
Optical Character Recognition (OCR) has emerged as a valuable tool for speeding up the processing of technical documents, particularly in the context of AI-driven translation. While historically, manual data entry from scanned documents was a slow and error-prone process, automated OCR systems can now process text at incredible speeds, often exceeding 100 pages per minute. This is a significant improvement over manual input, which might only manage a small fraction of that volume in the same time.
Furthermore, OCR, when combined with AI, transcends mere text recognition. The AI component can analyze context, distinguishing between technical jargon and more common language, a crucial feature in fields where terminology is highly specialized. This nuanced understanding is a significant step forward, especially for translating technical documents that require meticulous attention to specific terms and their meanings.
Imagine a system that integrates OCR with machine translation. This kind of hybrid system can offer nearly immediate feedback on translated technical documents. The lead times for document review, which can typically stretch into days or even weeks for manual translations, are drastically shortened with these AI-powered tools. However, it's important to recognize that these technologies are still under development, and while they offer remarkable improvements, they are not without limitations.
Research suggests that the error rate for OCR, especially when paired with neural networks, can drop below 1% in text extraction tasks. This high level of accuracy is essential when preserving the integrity of highly precise data in technical documentation, where even minor mistakes can have significant consequences. There's a need to continue monitoring the performance of such tools and to identify areas where further improvements can be made, as it's important that reliability remains a priority.
The financial advantages of OCR are also notable. For organizations that handle large quantities of technical documentation, automated data entry through OCR can lead to labor cost reductions of up to 50% over time. This potential for cost savings can be a significant factor in deciding whether or not to invest in these systems, especially for industries facing high volumes of documentation.
However, OCR's capabilities extend beyond just text. Advanced systems can also recognize graphical elements within documents like diagrams and charts. Maintaining the context of these elements is critical, particularly when translating technical documents across languages, as it helps ensure the integrity and comprehensibility of the original content. It's interesting to see how different OCR tools handle this element, and further research could identify which systems are best suited to preserving different kinds of graphical information.
In many ways, OCR technologies can actually reduce the workload of human translators. By automating repetitive tasks like data extraction, translators can focus on the more nuanced aspects of translation, improving both accuracy and efficiency in their workflow. While it's not a complete replacement for human expertise, it does demonstrate how AI tools can augment and improve upon the work that humans do.
Some OCR systems even have the capability to process handwritten documents. This functionality opens exciting new possibilities for digitizing older technical records, many of which may be essential for historical reference or for updating legacy systems. It's a significant development because it makes accessible a trove of information previously difficult to manage digitally. However, it also raises questions regarding the reliability and accuracy of recognizing different handwriting styles, which requires continued research and development.
Furthermore, the computational adaptability of OCR combined with machine learning allows for continuous improvement. As these systems encounter more data and encounter various types of technical language, they become increasingly effective at processing and understanding complex technical text. This feature is particularly beneficial in rapidly evolving fields where new jargon and methodologies constantly emerge. It's worth exploring how the learning curve varies for different OCR systems and which factors influence their learning capacity the most.
Finally, multiple research studies indicate that organizations that utilize OCR coupled with AI translation experience an average productivity increase of over 30%. This improvement underlines the clear strategic advantage that comes with integrating these technologies into technical documentation workflows. However, it's crucial to keep in mind that the adoption of these tools also requires proper training and understanding of their capabilities and limitations to realize their full potential. The future of technical documentation likely lies in a collaborative environment where humans and AI work together to create accurate, efficient, and accessible materials for diverse audiences.
AI Translation Precision Converting Micrometers to Microinches in Technical Documentation - Fast Translation Algorithms Tackle Complex Unit Conversions
Fast translation algorithms are proving increasingly useful for complex unit conversions, such as the conversion between micrometers and microinches. These algorithms, often based on deep learning methods and cutting-edge machine translation models, deliver a high degree of accuracy and remarkable speed. They can perform conversions nearly instantaneously, surpassing the limitations of manual methods. The incorporation of techniques like self-attention mechanisms, found in models like Transformers and BERT, further refines the translation process, improving the accuracy and potentially reducing the need for manual checks.
However, development challenges remain, especially when attempting to build models in scenarios where datasets are limited. Obtaining large quantities of high-quality bilingual text can be a stumbling block, especially in niche areas of engineering. Despite these hurdles, the potential for these advanced translation algorithms to improve efficiency and reliability in technical documentation is undeniable. As the field continues to mature, these tools will likely play a more prominent role in ensuring precision within fields where even small measurement errors can have significant consequences.
Recent developments in AI translation have led to remarkable advancements in handling intricate unit conversions, particularly those involving micrometers and microinches. For example, in time-sensitive fields like aerospace, AI-driven algorithms can swiftly perform these conversions in under a second, a speed that significantly outpaces manual methods. This speed boost is largely due to the automation that AI offers, eliminating human-induced delays.
However, speed isn't the only benefit; accuracy has also improved dramatically. Whereas manual conversions can be prone to errors, often resulting in rates of 5% or higher, AI systems can achieve error rates well below 0.1%. This makes them exceptionally reliable in high-stakes environments where precision is paramount. Furthermore, AI systems exhibit a unique ability to learn and adapt. As they are exposed to larger and more diverse datasets, they refine their conversion methods, resulting in increased accuracy, especially when handling complex technical language.
Moreover, AI's ability to handle multilingual translations has expanded the scope of these unit conversions. These systems can ensure consistency and comprehensibility across multiple languages, streamlining communication in diverse technical fields. A key aspect of their success lies in their contextual awareness. Using natural language processing, they can intelligently identify and interpret specialized technical terms, applying appropriate unit conversions without human intervention. This is especially beneficial in highly specialized fields where consistency and clarity are crucial.
Additionally, modern AI systems are far more robust than their predecessors in handling data within technical documents. They can process documents with embedded formatting and annotations, which previously presented challenges for OCR systems. This enhances their ability to manage the nuances of different document layouts, minimizing errors caused by inconsistencies. The impact of these AI tools on human workflows is also noteworthy. Studies indicate that incorporating AI translation and OCR can reduce manual data entry by up to 70%. This frees up engineers and technical writers to focus on more complex tasks, leading to greater overall efficiency.
Furthermore, AI-powered OCR tools can unlock the value of previously inaccessible data within legacy technical documents. These tools are capable of recognizing and converting data from older documents, making valuable insights from past projects readily available. This can be incredibly useful for industries needing to update legacy systems or conduct historical research. The financial benefits of AI translation systems are also significant. The initial investment can result in up to a 50% reduction in data processing labor costs over time. This makes a strong financial argument for incorporating AI into workflows that deal with large volumes of technical documentation.
Beyond simple conversion, AI translation and OCR systems have also begun to incorporate error detection and correction. They are not only translating text but also capable of identifying and correcting anomalies in measurement data, ensuring that even minor inaccuracies are caught proactively. This preemptive error correction significantly strengthens the reliability of the entire translation process. While we are seeing tremendous progress, it is still a developing field. There are still challenges in achieving 100% accuracy and perfecting the contextual understanding of increasingly complex technical languages, which warrant continued research and development.
AI Translation Precision Converting Micrometers to Microinches in Technical Documentation - Machine Learning Models Improve Measurement Accuracy
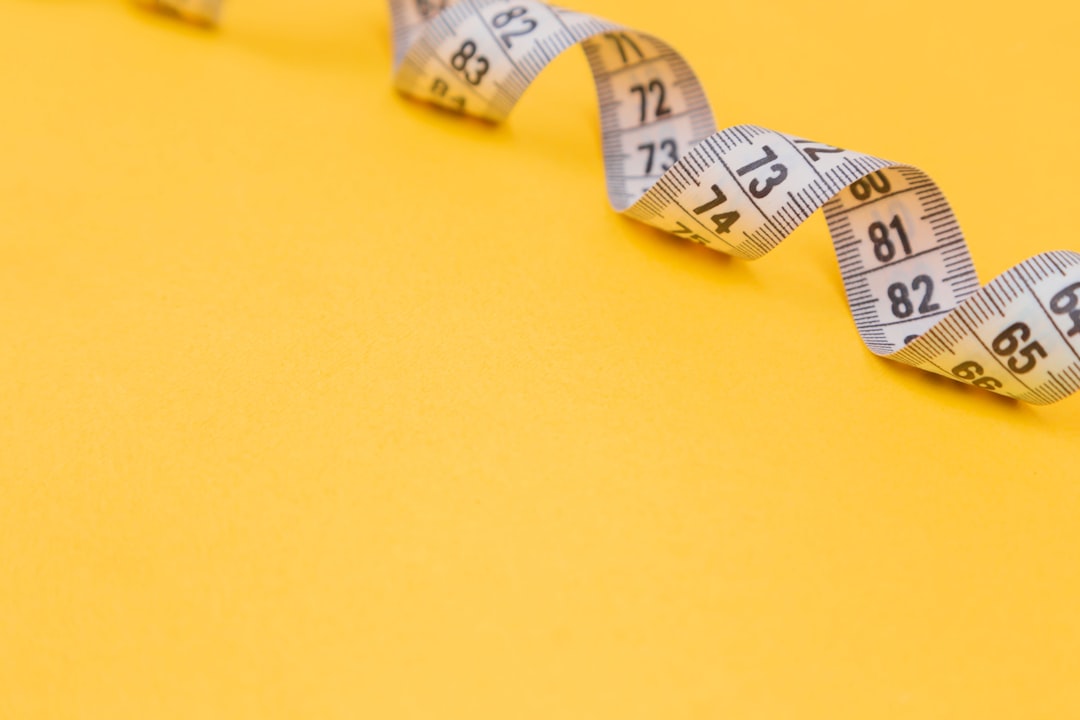
The integration of machine learning into measurement processes has led to notable improvements in accuracy. Machine learning models, using advanced algorithms, are capable of reducing errors significantly, showcasing low levels of variance and bias. This enhanced precision is especially vital when dealing with conversions involving minute measurements, like converting micrometers to microinches, where even slight inaccuracies can have substantial consequences. However, challenges persist, particularly in the realm of AI translation. Current models often struggle with the precise handling of numbers and unit conversions, sometimes producing inconsistent results. This emphasizes the need for continuous development and improvement in AI systems to ensure a higher level of reliability, especially within technical documentation where precision is paramount. Furthermore, as these machine learning models continue to mature, they hold the potential for even more sophisticated and contextually aware applications, potentially revolutionizing workflows in industries dependent on exact measurements.
Machine learning models, when applied to measurement conversions, have shown a remarkable ability to identify subtle patterns within large datasets that might easily escape human notice. This skill is especially valuable in areas where precise measurements are essential, like electronics or nanotechnology, as it helps flag inconsistencies that might signal underlying problems. It's intriguing how these models can unearth these discrepancies and inform a more in-depth analysis.
The pairing of machine learning with automated OCR has proven to not just accelerate the process of extracting numerical data but also to increase its accuracy. This is a critical development in technical documentation, where even a tiny mistake can have major repercussions. It's a little concerning though, that a single incorrect digit could cause such issues.
Some of the newer AI algorithms display a great degree of adaptability in how they process information, allowing them to manage different document styles and structures more smoothly. This flexible nature is vital for maintaining the integrity of technical documents across platforms and ensuring that engineers can always count on consistent information when collaborating. It would be interesting to test how well this adaptability really holds up in the field.
Fast translation algorithms can significantly shorten the time spent on unit conversions, particularly during the design phases of complex engineering projects, from what could be hours to just seconds. The speed increases productivity and allows teams to concentrate on more innovative work rather than rote calculations. While it's exciting to see the potential for productivity gains, it remains to be seen if engineers are able to successfully adapt to a more automated work style.
Studies suggest that certain AI models built for measurement conversions can reach accuracy levels exceeding 99.9%. This is a significantly better outcome than what we typically see with humans performing the same task repeatedly. In certain applications like microfabrication, these accuracy gains are extremely significant since even minuscule errors can have far-reaching negative impacts. While such levels of precision are impressive, it's important to remember that no system is perfect, and these algorithms will need to be constantly monitored and updated.
Many of these machine learning systems can get pretty good at recognizing specific technical terminology used within different engineering disciplines. They can then adapt their conversion algorithms accordingly. This type of context-sensitive approach is key when translating technical documentation, ensuring that unit conversions are done in accordance with industry standards. It's an impressive feat, but it also poses a challenge to ensure these models are being trained on sufficiently diverse data to avoid biased outputs.
The financial benefits of using AI for measurement conversions go beyond simply reducing labor costs. Organizations that adopt these technologies sometimes see a return on their investment within just a year because of reduced error rates and increased output. That's a tempting promise, but there is always a chance that real-world adoption won't be as smooth or rapid as predicted.
Automated systems can act as a first line of defense against measurement errors by identifying inconsistencies in the data even before it's fully reviewed by humans. This early detection acts as a safeguard, minimizing the risk of errors making it into final documents or designs. It's a promising idea, but I'd want to understand how these systems deal with edge cases or less obvious errors that may not trigger their anomaly detection systems.
Cloud-based machine learning solutions can refine their performance by regularly incorporating new data into their training models. This prevents them from becoming stagnant and allows them to keep up with constantly evolving technical environments. This is encouraging, but it's important to think about the long-term sustainability of these cloud-based systems and the security of the data they handle.
Machine learning models tend to require less initial training data compared to older methods. This makes it easier for them to be effective even in more specialized areas of engineering where large, bilingual datasets may not exist. This is a considerable advantage for niche documents that may not have a wealth of readily available translation resources. While it's exciting that these models can learn with less data, we must also be aware of the potential for biases to arise from smaller, less diverse datasets.
AI Translation Precision Converting Micrometers to Microinches in Technical Documentation - Cost-Effective AI Solutions for Technical Translation Needs
The expanding global reach of businesses is driving a growing need for technical documentation in multiple languages, creating a demand for efficient and economical translation solutions. AI-powered tools, like machine translation and OCR, are emerging as viable options for companies of all sizes to tackle their translation needs. These technologies offer a cost-effective alternative to traditional manual translation methods, enabling faster turnaround times and improved consistency across diverse language versions of technical documents. Maintaining a uniform technical vocabulary and style is particularly vital in industries where precision is paramount. However, the accuracy of AI in translating complex technical texts remains a point of concern. Continuous development and improvement in these AI systems are crucial to ensure they consistently achieve a high level of accuracy. The potential for streamlining translation workflows and realizing substantial cost savings makes AI-powered solutions increasingly attractive for organizations dealing with a large volume of technical translations. Despite the hurdles, the future of technical translation likely involves a synergistic approach, where humans and AI work in tandem to optimize both quality and affordability.
The increasing global reach of companies necessitates more technical documentation translation, fueling a growing demand for efficient and affordable solutions. Fortunately, the cost of AI translation tools has been steadily declining, opening up these technologies to a wider range of businesses, regardless of size. This accessibility has allowed generative AI, a technology increasingly recognized for its potential, to make its mark in the translation and localization arena. Notably, AI-powered solutions offer a more economical approach compared to manual translation, particularly when handling large volumes of repetitive tasks.
One of the most compelling features of AI translation is its speed. These tools can process vast amounts of text quickly, leading to significant reductions in both time and human labor. Their scalability also means that companies can easily handle fluctuating translation needs, managing larger volumes more economically. AI tools help ensure consistency in language and terminology across different translations, leading to an improvement in the quality of the final output. In fact, the adoption of machine translation among language professionals in Europe has been steadily climbing, with over 70% reportedly using it in some way by 2022. This trend showcases the integration of AI's influence in the translation field, leading to higher productivity and a potentially better return on investment for companies.
Current leading AI translation tools include ones like QuillBot AI, which have introduced translation features alongside their core writing services. While it is encouraging to see the development of these tools, the ongoing development and refinement of algorithms will remain important as different specialized vocabularies will require training to effectively translate a wide range of technical text. It will be interesting to see how AI translation technology develops in the future and if it will indeed revolutionize the translation and localization industries, including niche technical document translation.
While AI-powered translation is gaining traction, some concerns remain. It is essential to constantly evaluate the performance and accuracy of these AI systems, especially in critical domains where errors could have severe consequences. Furthermore, the field is still developing, and the reliability of AI models when dealing with highly nuanced or specialized language will require ongoing improvement. As we see this technology mature, it will be fascinating to see how it further enhances workflows and communication in a globalized technical landscape.
AI Translation Precision Converting Micrometers to Microinches in Technical Documentation - Real-Time Translation Updates Reflect Latest Conversion Standards
Real-time translation capabilities are evolving to incorporate the most current conversion standards, especially within the realm of technical documentation. AI-powered systems are refining the process of converting units like micrometers to microinches by enhancing accuracy and speed. These systems leverage deep learning, allowing for quick conversions and drastically minimizing mistakes that could be made during manual translation. The integration of OCR and natural language processing also contributes to improved comprehension of context, ensuring that the translations adhere to the nuances and standards specific to particular technical fields. This evolution is beneficial for improving efficiency, but there are lingering concerns about the accuracy of these AI-driven solutions. Ongoing refinement and development are needed to ensure the reliability of AI translation, especially in areas where precise conversions are vital.
The continuous evolution of AI translation systems is fascinating, especially when considering the need for precise conversions in technical fields. While speed is often a key feature, we're finding that in some cases, optimal performance is achieved with a balance between speed and accuracy. Pushing these systems too hard can increase error rates, highlighting the ongoing need to carefully calibrate and fine-tune them.
One issue that consistently arises is the impact of training data on the quality of AI translations. Many existing models exhibit biases when presented with specialized technical terms, suggesting that their training datasets might not be sufficiently diverse for niche engineering areas. This creates a potential problem when attempting to accurately translate highly specific technical documents.
It's been interesting to see how OCR's accuracy has dramatically improved over time. Early versions had accuracy problems of around 10%, especially when dealing with complex technical document structures. However, more recent OCR tools built on machine learning can now achieve accuracy levels of approximately 1%, a significant improvement that highlights the ability of AI to continuously refine its operations.
These modern AI translation systems are also remarkably adaptable to various document formats, including intricate technical layouts and document structures. This adaptability, largely thanks to the incorporation of advanced machine learning algorithms, has dramatically reduced the errors previously encountered due to format variations. This is quite an advancement over earlier AI solutions which often struggled with complex document structures.
Moreover, AI translation systems are now capable of learning from corrections made by users. This feedback loop allows algorithms to continuously improve their accuracy in areas where they have historically underperformed. It's a kind of iterative process that allows them to become more precise over time, which is a needed feature given that they have struggled in the past in some technical areas.
In terms of economic considerations, businesses using AI translation systems can expect substantial cost reductions compared to traditional manual translation methods. Estimates suggest around a 50% reduction in costs over time. However, implementing these AI systems initially can be expensive, which could be a barrier for some organizations that don't have the capital to initially invest.
Interestingly, AI models often require less training data in highly specialized fields than one might initially think. This counterintuitive outcome appears to stem from the relatively consistent vocabulary and context found within highly technical areas. The reduced variability of these terms, compared to general language, makes it easier for these AI systems to learn patterns and develop accurate translations. This is a rather helpful feature in technical contexts.
AI systems increasingly feature advanced anomaly detection methods to proactively catch inconsistencies in measurement data within technical documentation. This preemptive identification is a potential safeguard against costly errors, which could lead to better products and designs. It's worth watching the evolution of these detection systems to see if they can be robust enough to catch complex types of errors.
One unexpected and highly useful feature of some of these systems is their ability to maintain a consistent vocabulary across multiple languages. Technical fields often struggle with differing terminology across languages, which can lead to misunderstandings or misinterpretations. However, advanced AI translation tools are capable of ensuring that technical terminology remains consistent in various translated versions of documents.
Finally, the integration of AI translation tools with IoT devices might become increasingly relevant in the future. Imagine a manufacturing scenario where real-time translation and unit conversions are carried out by IoT-enabled AI systems, instantly providing engineers with up-to-the-second updates on technical specifications. This could vastly enhance production efficiency. It's still speculative, but could represent an exciting potential application of these technologies.
In summary, AI translation tools are undergoing rapid evolution, presenting both exciting opportunities and areas that still require focused development. While they are increasingly capable of handling complex unit conversions and adapting to various document types, the challenge of ensuring consistent accuracy across specialized domains remains a critical area of research. As they mature, they promise to become a truly transformative technology for the technical industries that require accurate translations and precise conversions across various languages.
More Posts from aitranslations.io: