AI-Powered PDF Translation now with improved handling of scanned contents, handwriting, charts, diagrams, tables and drawings. Fast, Cheap, and Accurate! (Get started for free)
AI Translation Trends Navigating Seasonal Mood Fluctuations in Language Processing
AI Translation Trends Navigating Seasonal Mood Fluctuations in Language Processing - AI-Powered OCR Enhances Multilingual Document Processing
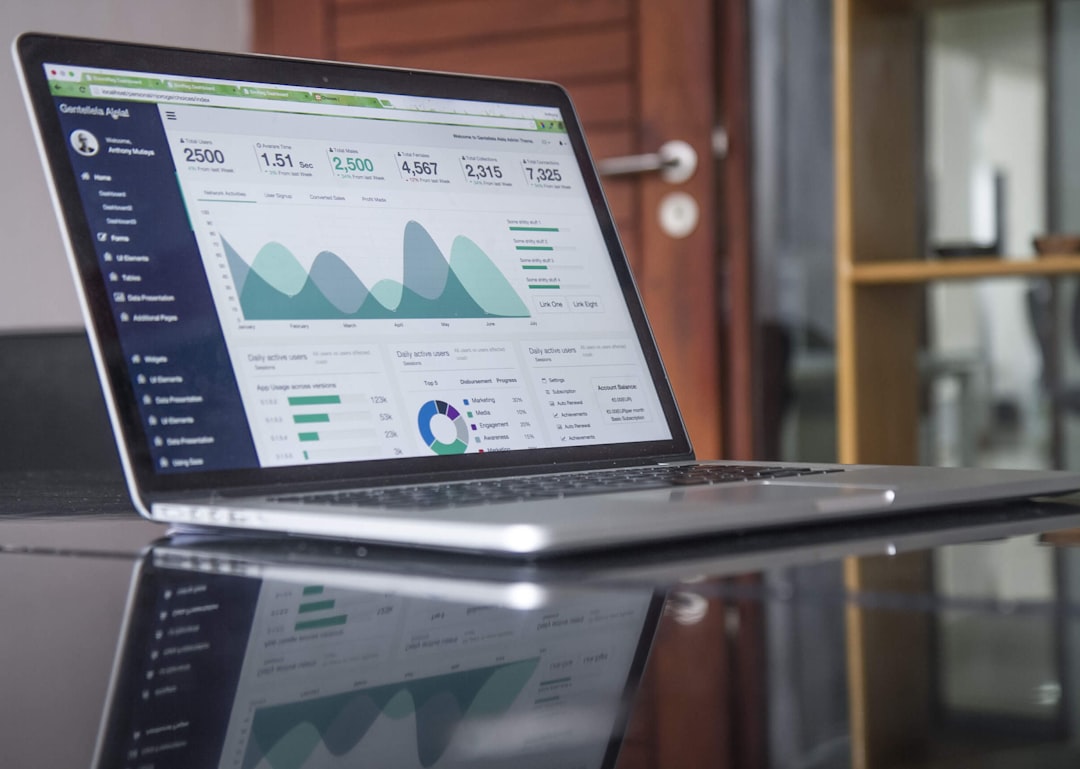
AI-driven OCR is reshaping how we handle multilingual documents, making it easier to extract and organize text from diverse sources. This capability is especially valuable in areas like e-commerce and retail, where swiftly processing orders and managing inventory are paramount. Combining AI and OCR empowers automated document analysis, using techniques like natural language processing and deep learning to understand the content more accurately and rapidly. While this combination shows great potential, fully automating document processing remains a hurdle. The ongoing development of AI suggests its impact on areas like localization and global communication will continue to expand, creating a need for ongoing adjustments and attention. The path forward will likely involve balancing the strengths of AI-powered OCR with the nuances and complexities of human language across numerous languages.
AI-powered OCR has opened new avenues for handling documents in a multitude of languages. It can now decipher text from over a hundred languages, minimizing the time-consuming manual intervention that was once the norm for translation. Interestingly, integrating AI into OCR seems to drastically boost accuracy, pushing it beyond 98% in certain scenarios. This is especially important for dealing with documents in sectors like law and finance, where accuracy is paramount. However, it's important to note that the source document's quality remains crucial. High-resolution scans or images produce better results, highlighting the need for clear input, a factor amplified when dealing with multiple languages.
Further, these AI systems, trained on a broad spectrum of languages, are becoming adept at recognizing variations within languages, such as dialects and regional speech. This adaptable quality is essential in locations where language shifts can be quite rapid over small distances. Moreover, with advancements in neural networks, AI-driven OCR has moved beyond just printed text; it can now decipher handwritten documents as well. This capability unlocks the potential for digitizing a much wider array of materials, encompassing historical documents and personal notes, expanding the reach of multilingual processing.
While the positive effects of AI on OCR are evident, it’s important to consider the ongoing need for human oversight in some areas. Implementation of AI-powered OCR seems to lead to cost reduction through automated processes. Companies have reported a significant drop in labor hours related to data entry for translation, leading to greater efficiency. Also, many OCR systems are incorporating machine learning approaches that learn from human corrections, refining the accuracy of both recognition and translation over time through a feedback loop.
The marriage of OCR with NLP allows for a more in-depth understanding of language. This combination empowers translations that account for the nuances of language, especially in idiomatic expressions, enhancing the overall quality of translated content. There's also been a surge in OCR tools that can translate in real-time, often within a fraction of a second. This rapid processing is invaluable for time-critical situations such as live translation at events or emergency communication. The increasing use of cloud-based OCR solutions democratizes access to sophisticated multilingual processing, requiring less initial investment in infrastructure and making powerful translation tools available even to smaller companies. While progress in OCR is notable, there are still challenges regarding the total automation and streamlining of manual document processing that hinder the complete transformation of the field. The role of AI in language processing continues to expand, and advancements in multilingual document analysis create opportunities for businesses to navigate high-volume transactions and improve client experience.
AI Translation Trends Navigating Seasonal Mood Fluctuations in Language Processing - Seasonal Language Shifts Impact Neural Machine Translation Models
Seasonal shifts in language, influenced by cultural and social factors, can create difficulties for neural machine translation (NMT) models. These models, often trained on large datasets, may struggle to adapt to the subtle variations and nuances that emerge during different periods of the year. This can lead to inaccuracies and a decline in the overall quality of translations. The challenge stems from the mismatch between the fixed nature of the training data and the dynamic evolution of language.
Furthermore, the rapid development of AI translation technology often focuses on widely used languages, leaving many lesser-known languages behind. This creates a disparity in the level of translation quality available across languages, emphasizing the need for greater inclusivity and equal access to advanced translation tools. While progress in AI translation is undeniable, there's a growing awareness of the need to balance the pursuit of efficiency and scalability with a deeper understanding of how languages evolve across seasons and cultures. Future advancements in AI translation should incorporate more nuanced and adaptive approaches that better account for these dynamic shifts. This will help ensure that AI-driven translation systems remain relevant and effective across the diverse spectrum of languages used globally.
AI translation models, while impressive, are still developing, especially when dealing with the nuanced shifts in language that come with the seasons. Research suggests that vocabulary and sentence structures can change depending on the time of year, potentially causing a decrease in translation accuracy for models trained on static datasets. This is particularly important because if the model's training data doesn't adapt, the translation's quality can suffer.
We're finding that the variations in language caused by seasonal trends are more pronounced in some languages than others, especially those with many dialects. This creates challenges when trying to design AI models that work across various cultures. It raises questions about whether the models can generalize well enough to be useful for a wide range of languages. It's also fascinating to consider how idiomatic expressions and local slang can pop up during particular times of year. AI models that aren't exposed to these language changes might not understand them well, leading to less effective translations.
One intriguing possibility is to use human feedback to help AI translation models adjust to seasonal language shifts. It could involve creating interactive systems that learn from real-time data, allowing the translation tools to adapt much faster. However, the challenge lies in designing efficient systems that can keep up with rapid changes in how people speak and write. We've also observed that slang and informal language tend to increase during cultural events like holidays or festivals. This increase in informal language could be a real challenge for AI models that are not regularly updated with the latest language trends.
To improve these models, a focus on high-quality annotations in the training data becomes even more important during seasonal transitions. Capturing the subtle nuances that emerge in language during specific times of year requires detailed and accurate annotation, leading to more robust AI translation models. There's also an interesting possibility that we could use advanced NLP and machine learning techniques to predict how language might change with the seasons. This prediction could prepare translation systems for expected changes in language patterns. However, this predictive ability is computationally intensive and requires more research to be practical.
It's also crucial to consider the broader implications of these seasonal shifts. How people use language online is influenced by seasonal trends, and this can affect AI models that analyze digital communication data. For example, language usage patterns around seasonal products can change due to increased searches for those products. The impact of these changes extends further as seasonal mood fluctuations may also impact consumer behavior, and AI translation models could benefit from being able to adjust their output accordingly. It's conceivable that future translation models could be designed to consider sentiment in their output, enabling better marketing strategies that match the emotional tone of the season. This area offers fertile ground for ongoing investigation.
AI Translation Trends Navigating Seasonal Mood Fluctuations in Language Processing - Fast Translation Tools Adapt to Cultural Nuances in Real-Time
Fast translation tools are rapidly incorporating cultural nuances into their processes, driven by AI advancements. These tools are striving to move beyond basic word substitutions and understand the subtle ways language differs across cultures. AI-powered systems now utilize large language datasets and machine learning techniques to better adapt to variations in how language is used at different times of the year, in various social settings, and during specific cultural events. However, the full integration of cultural context into translation remains an ongoing challenge. Many systems still rely on a fixed set of training data, which can fail to encompass the dynamic nature of language and its diverse manifestations. The future of accurate and effective global communication will depend on AI's ongoing ability to navigate these intricate cultural layers. While the potential for improvement is significant, overcoming the limitations of static datasets and building truly adaptable translation tools is a complex endeavor.
Global communication necessitates increasingly sophisticated translation systems, and fast translation tools are now adapting to incorporate the subtleties of cultural nuances in real time. AI-powered translation is moving beyond simple word-for-word substitutions, leveraging advanced algorithms and neural networks to understand context and meaning within specific cultures. These tools are now pulling from cultural databases and utilizing machine learning to improve accuracy, recognizing that direct translations aren't always adequate, especially when dealing with idioms or slang.
There's a growing focus on bridging the gap for under-represented languages with initiatives like "No Language Left Behind" aiming to extend AI capabilities to a wider range of languages, addressing the needs of those often overlooked. While progress is encouraging, there are still obstacles, especially when dealing with nuanced cultural differences in communication. A key challenge is ensuring accuracy, as cultural interpretations can differ significantly.
We're seeing a significant shift in the translation landscape. Tools are getting faster, with real-time translation becoming more commonplace, particularly helpful for events like live conferences and urgent communications. The ambition of these tools is to create more seamless understanding across global communities. However, we also need to acknowledge the complexities of language, as language is constantly evolving, impacted by social media, seasonal influences, and pop culture. This dynamic nature requires these translation systems to be continuously learning and adapting to remain relevant.
AI-powered tools are becoming better at recognizing language families and related dialects. This helps improve accuracy, particularly for those languages with fewer readily available resources. Furthermore, many tools are now incorporating feedback loops, utilizing human input to correct errors and refine translation quality. This constant refinement process is crucial to enhance accuracy, especially when dealing with regional variations in language.
Interestingly, some AI translation models are now incorporating social media data into their algorithms, allowing them to better understand current trends in language usage, including slang and informal speech. This is quite important as slang and informal language usage can vary significantly with seasons and events. We might see future translation models incorporate sentiment analysis as well. This could lead to more nuanced and impactful translations that consider the overall emotional context of the text, something that might be especially valuable for marketing materials. There is still a need for human oversight, but these technological advancements are steadily making translation more efficient and accessible. Overall, while the potential is there, the field of AI translation is still evolving, with a clear need for constant refinement and adaptation to address the challenges of cultural and linguistic variations.
AI Translation Trends Navigating Seasonal Mood Fluctuations in Language Processing - Balancing Cost and Quality in AI-Driven Language Services
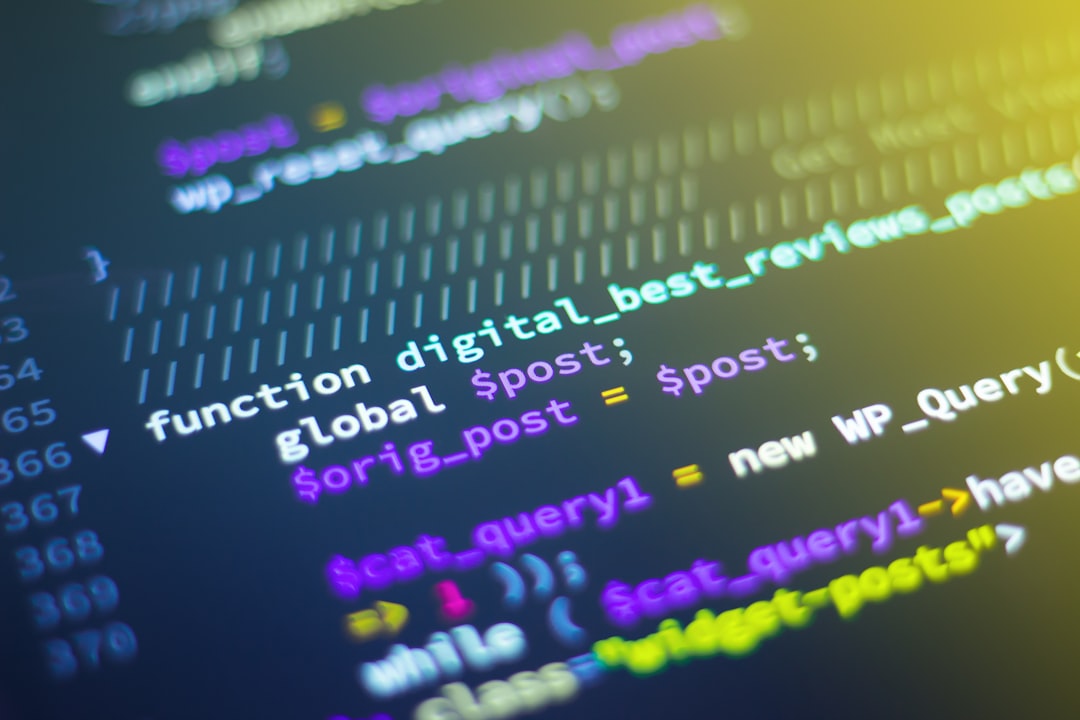
The push for swift and accurate language services using AI is driving a growing focus on balancing cost and quality. AI-powered translation, especially with generative models, can deliver translations much faster than traditional methods, leading to reduced costs. However, this speed often comes at a potential expense to the quality of translation, particularly when it comes to capturing subtle meanings and cultural contexts. While machine translation tools can greatly improve efficiency, they still require human intervention to maintain contextual accuracy and cultural sensitivity in the final output. As the landscape of AI translation continues to shift, the ability to successfully manage this tension between cost-efficiency and high-quality output will be crucial to meeting the complex needs of global communication. Finding this equilibrium is a continuous challenge within the translation field.
The pursuit of affordable AI-driven language services often leads to compromises in quality. Simpler AI models, trained on smaller datasets, might offer cheap translations but often lack the depth to handle complex sentences or understand cultural context well. This is particularly evident when dealing with idiomatic phrases or slang, which can be crucial in conveying accurate meaning. While we see rapid improvements in real-time translation, tools still stumble on those tricky expressions during live events or urgent communications where quick comprehension is key.
The quality of the source document is a significant factor determining the success of AI-powered OCR. Low-resolution scans or poorly formatted text can drastically impact accuracy. This becomes even more critical when dealing with multilingual documents, highlighting the importance of careful preparation. This isn't just an issue with obscure languages. Even well-known languages have dialects and variations that can throw off simpler models.
We see a clear trend toward prioritizing high-volume languages in the development of AI translation. Languages spoken by fewer people often get overlooked, lacking comprehensive training data and context that leads to lower quality translations. Keeping AI models up-to-date with language shifts throughout the year requires a continuous training cycle fueled by vast computational resources. This becomes a significant expense, particularly for smaller organizations trying to maintain competitiveness.
The way a document is formatted can have a dramatic effect on how well OCR works. Well-organized and clearly laid out documents offer the best results. On the other hand, messy or inconsistently formatted documents can seriously impede accuracy. Human oversight is critical for refining these AI systems. Systems that incorporate user feedback and learn from corrections have a tendency to perform better over time, especially when it comes to identifying subtle changes in language patterns.
Interestingly, even small geographical differences can cause language variations. These dialectal differences introduce a whole new layer of complexity that simpler models sometimes fail to recognize. Fast translation tools are attempting to adapt to cultural nuances. However, successfully interpreting the sociolinguistic context in real-time for different cultures is a constant challenge, especially when it comes to marketing or cross-cultural communications.
Despite the leaps and bounds made in AI translation, many fast tools still report error rates exceeding 20% for complicated sentences or less common dialects. This reveals the ongoing struggle to find the balance between speed, cost, and truly high-quality translations. It suggests that achieving truly cost-effective solutions while maintaining accuracy remains a complex challenge.
AI Translation Trends Navigating Seasonal Mood Fluctuations in Language Processing - Navigating Data Privacy Challenges in Global AI Translation Projects
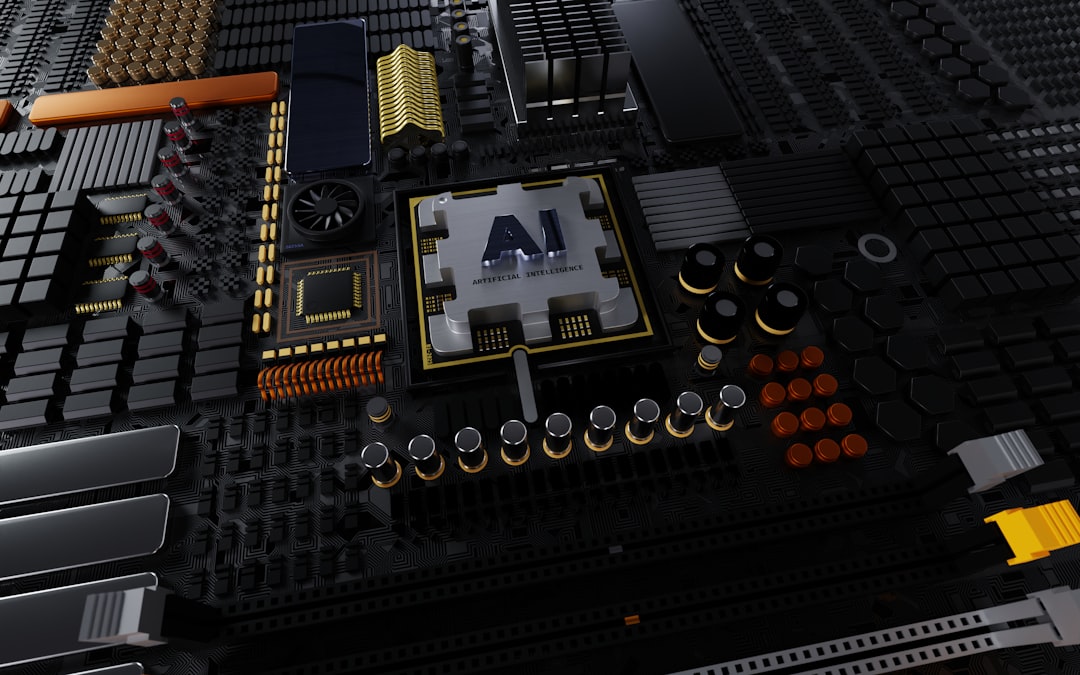
The expanding use of AI in global translation projects brings with it a set of complex data privacy concerns that demand careful consideration. Balancing the desire for efficient translation with the need to protect sensitive information requires organizations to strictly adhere to existing data privacy standards and develop strong ethical guidelines. This is particularly important given the nature of translation work, which often involves handling personal or confidential data. Moreover, cultural sensitivities must be addressed, as AI models may not always capture the subtle nuances within languages and cultures, potentially leading to inaccurate or insensitive translations. The threat of data breaches is also a significant factor to consider, prompting a need for stronger safeguards to prevent unauthorized access to the vast amounts of data used to train and power AI translation tools. The challenge moving forward will be to integrate robust privacy measures into the design and development of these AI systems, ensuring they are both effective and respectful of user privacy and the diversity of global languages and cultures.
Navigating the global landscape of AI translation presents unique challenges related to data privacy, particularly given the diverse regulatory environments and the nature of the data involved. Data privacy regulations vary wildly from one nation to another, creating a complicated patchwork of rules for AI translation projects. For example, the European Union's General Data Protection Regulation (GDPR) sets a very high bar for how data is handled, but in other parts of the world, the legal landscape is more fragmented, making it hard to implement a single standard.
AI translation models typically rely on massive datasets of text in multiple languages to learn and improve. Gathering and using this data, while staying compliant with privacy laws, creates ethical questions about who owns the data and whether proper consent was given. It's a tightrope walk between getting the necessary data and respecting individuals' privacy rights.
Another crucial aspect is anonymization. Stripping out personally identifiable information from datasets used to train AI translation systems isn't as simple as it sounds. Even after removing obvious identifiers, the remaining context can sometimes be enough to identify individuals, potentially leading to privacy violations. It's a tricky balancing act, finding a way to protect individuals' privacy while retaining enough context for the translation model to be accurate.
Many fast translation tools rely on cloud-based systems to handle the massive amounts of data involved. However, this approach raises issues about data vulnerability because it can expose sensitive information to various jurisdictions. If a company is unaware of the data protection standards in a particular region where their data is stored, they might unintentionally expose user information to less stringent rules, putting people's privacy at risk.
AI models trained on data generated by users, like social media content, can sometimes inherit biases embedded in that data. These biases can be both cultural and linguistic, leading to errors in translations that may misrepresent or even offend certain groups. It highlights the complexities of dealing with language and culture in a sensitive way using AI.
Interestingly, the drive to comply with data privacy requirements can sometimes lead to 'data scrubbing', where the model over-filters for sensitive content and loses the nuances of the original text. This highlights the difficulties in creating AI systems that can meet both privacy and accuracy goals simultaneously.
Real-time translation poses particular problems for privacy assessment. The swift pace of processing doesn't allow enough time for careful review of how the data is being handled and stored, leading to possible gaps in privacy protection.
The growing reliance on AI for translation services might lead to decreased focus on rigorous data handling practices. As we become more accustomed to automated translation, it's important to avoid complacency when it comes to ensuring compliance with data privacy rules.
OCR, used to translate documents scanned into a digital format, can introduce its own data security risks. Scanning and storing sensitive documents without proper encryption or context filtering creates vulnerabilities, and care is needed to ensure data safety and user privacy.
Seasonal changes in language usage can affect the content of user-generated data. Specific times of year may see increased use of sensitive or culturally specific phrases, making continuous monitoring of datasets for AI training important to ensure compliance with data privacy standards.
These issues highlight the importance of carefully considering ethical implications of AI translation tools, including the potential for misuse of personal information. The future of AI in global communication hinges on developing responsible AI systems that respect user privacy while continuing to provide accurate and useful translations.
AI Translation Trends Navigating Seasonal Mood Fluctuations in Language Processing - Human-AI Collaboration Remains Crucial for Contextual Accuracy
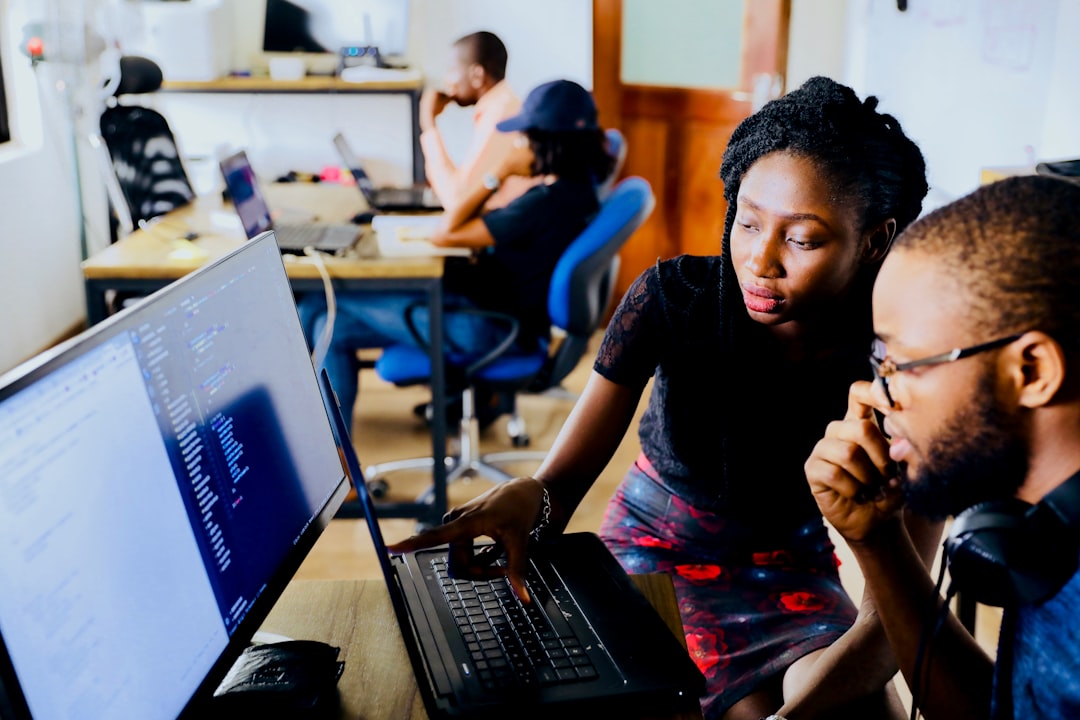
The importance of human-AI collaboration in translation is becoming increasingly evident, especially when aiming for accurate contextual understanding. AI translation tools, while offering faster and more accessible translations, often fall short when it comes to grasping the subtle nuances and cultural contexts vital for effective communication. Though AI has advanced in its ability to handle varied language styles through natural language processing and machine learning, it still needs human intervention to fully understand complexities like idiomatic phrases and regional dialects. As AI translation continues to evolve, this partnership between humans and AI will become more crucial for achieving high-quality translations that resonate with intended audiences, especially during times when language itself shifts due to seasonal influences. This highlights the necessity of blending human intelligence with AI-powered systems to create a deeper level of understanding in the ever-expanding world of global communication.
While AI translation has undeniably accelerated the speed and accessibility of translations, particularly with the rise of tools focused on fast and cheap translation, the need for human-AI collaboration in ensuring contextual accuracy remains paramount. AI, especially models like OpenAI's GPT-4, is revolutionizing language technologies, but it still struggles to capture the subtle nuances and cultural contexts that are crucial for effective communication, particularly during seasonal shifts in language patterns.
Research suggests that AI models trained on static datasets can falter when confronted with the dynamic nature of language, including seasonal shifts in vocabulary and expressions, leading to potentially nonsensical translations. The reliance on static data becomes especially problematic in languages with numerous dialects, as AI systems often struggle to differentiate and translate these variants accurately. While impressive advancements have been made in dialect recognition, achieving perfect parity with human comprehension in this area remains an ongoing challenge.
Interestingly, the introduction of feedback loops, where humans correct AI errors and provide input, has demonstrated a significant impact on translation accuracy. By learning from human corrections, AI models are better able to adapt to language evolution and nuanced changes. However, the ongoing question persists: how can we best leverage these feedback loops to ensure that AI remains aligned with evolving cultural contexts and adapts swiftly to seasonal changes?
The quality of the input data, particularly when utilizing OCR technology, has also been shown to significantly impact AI's ability to translate accurately. Clear, high-resolution scans produce vastly superior results, emphasizing that the success of the entire process hinges on the preparation of the source document. This is not a trivial matter, especially given the increased use of AI for processing diverse languages, including those with fewer available resources for training data.
The translation industry is, therefore, moving towards a hybrid approach, where human translators and AI work together. This collaborative environment leverages the strengths of both: AI's rapid processing and human's capacity for nuanced understanding. It has become apparent that solely relying on AI for translation, without human oversight, can lead to errors and a disconnect with the intended cultural message.
Another interesting aspect of this trend is the increased focus on incorporating cultural sensitivities into AI translation models. Algorithms are being developed to leverage cultural databases and adapt translation output accordingly, minimizing the risk of inadvertently creating insensitive or inaccurate translations in specific contexts. While this is an encouraging trend, it requires careful monitoring and development to prevent biases from creeping into the training data and further impacting the accuracy of the AI translation.
Despite these improvements, the resource requirements for continuous model training to adapt to seasonal and dialectal variations are substantial, particularly for the smaller organizations hoping to benefit from cheap and fast translation services. Moreover, the ongoing challenge of ensuring data privacy and ethical use of the vast language datasets powering AI translation remains a crucial topic of research and discussion. The diverse regulatory landscape of data privacy adds another layer of complexity, highlighting the need for careful consideration of ethical implications in the development and application of AI translation tools. It's a continuous balancing act between the quest for cheap and efficient translation tools and the requirement to safeguard sensitive data and uphold ethical standards, particularly in a globalized world with a plethora of different languages and customs.
AI-Powered PDF Translation now with improved handling of scanned contents, handwriting, charts, diagrams, tables and drawings. Fast, Cheap, and Accurate! (Get started for free)
More Posts from aitranslations.io: