Lost in Translation Why AI Language Models Still Can't Challenge Obvious Linguistic Truths (A 2024 Analysis)
Lost in Translation Why AI Language Models Still Can't Challenge Obvious Linguistic Truths (A 2024 Analysis) - Language Models in 2024 Still Miss Basic German Grammar Rules Despite OpenAI Updates
Even with OpenAI's continued efforts to refine their language models, 2024 sees these systems still struggling with rudimentary German grammar. This is surprising given their immense size and the sheer number of parameters they employ. It suggests that simply making models bigger and feeding them more data doesn't automatically translate to a deeper understanding of language. This lack of progress in key areas like grammar raises concerns about the overall approach to improving AI translation. While AI can generate impressive-sounding text, be it poetry or code, the reality is that it often does so through pattern recognition rather than genuine comprehension. This becomes painfully evident when dealing with the basic building blocks of languages. The continued presence of these shortcomings emphasizes the crucial role of human linguistic expertise in the quest for truly accurate and reliable translations. This remains a vital aspect of the field, particularly as AI translation technology evolves. The pursuit of fast and cheap translation shouldn't come at the expense of accuracy, and we must acknowledge that fundamental linguistic understanding still holds a significant advantage over mere statistical prediction.
Even with OpenAI's efforts, language models in 2024 still stumble over fundamental German grammar. Issues like correctly using articles and conjugating verbs persist, often leading to translations that are far from accurate. This creates a disconnect between the AI's apparent fluency and its genuine ability to handle the nuances of a complex language like German.
It seems the focus of recent model updates has been on expanding vocabulary rather than tackling these grammatical complexities head-on. This leaves a considerable gap in the overall accuracy of language models. German, with its intricate case system, poses a particularly challenging example. AI models frequently make mistakes related to grammatical gender and number, undermining their usefulness in academic or professional contexts.
The desire for fast translation in AI tools can often prioritize speed over grammatical accuracy. While this leads to swift translations, the output is frequently far from a faithful rendering of the original. Similarly, cheaper translation services often rely on older, less refined language models, which are especially prone to errors when tackling languages like German.
OCR, when paired with AI for translation, also shows limitations. It's able to recognize characters with precision but struggles to understand the contextual meaning of the text it's processing. As a result, translated outputs, despite having accurate character recognition, still suffer from a lack of consistent grammatical application.
It appears language models often prioritize frequently occurring phrases and patterns over less common, yet grammatically correct constructions. This tendency, while beneficial for common language scenarios, undermines the translation of complex sentences or idiomatic expressions in German. While advancements have been made in translating everyday language, the more complex or nuanced aspects of the language reveal AI's weaknesses.
The core issue lies in developing AI models that don't simply recognize words but fully grasp the underlying rules of grammar. This remains a crucial area for improvement, even for the most advanced AI systems available today.
Lost in Translation Why AI Language Models Still Can't Challenge Obvious Linguistic Truths (A 2024 Analysis) - Machine Translation Creates Artifacts When Processing Japanese Writing Systems
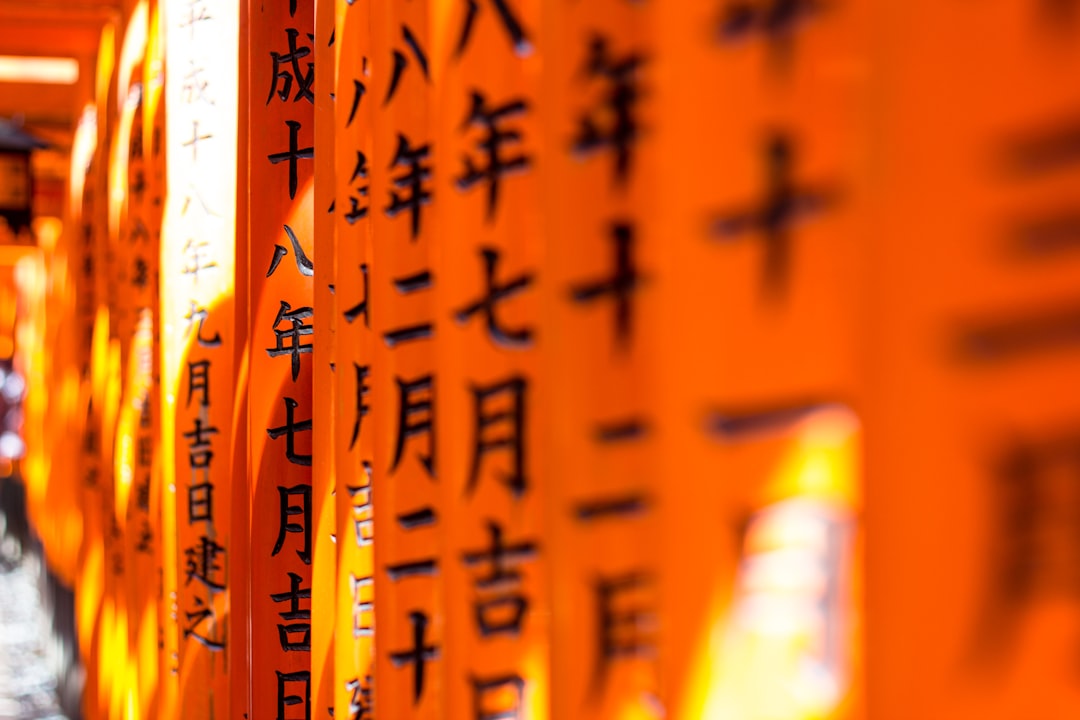
Machine translation faces unique hurdles when dealing with Japanese writing systems. The complexity of Japanese, with its combination of kanji, hiragana, and katakana, often leads to errors or "artifacts" in translated output. Simply put, AI struggles to accurately translate Japanese because of the language's nuances and structure. Unlike languages with more straightforward grammatical systems, Japanese requires a deeper understanding of context and meaning, which current models often miss.
Traditional translation approaches relied heavily on pre-set rules and large dictionaries, but these methods proved inadequate for the inherent ambiguity of Japanese. While advancements in machine learning hold promise, AI still struggles to grasp the full context of Japanese text. It tends to rely more on statistical probabilities and frequently occurring patterns rather than a true understanding of grammar and meaning. This results in translations that might be superficially acceptable but often fall short when dealing with more complex linguistic structures and culturally specific expressions.
To improve translation quality in Japanese, the field needs to shift focus towards developing more sophisticated models. These should be capable of grasping the intricate interplay of the different writing systems and better incorporate cultural context. Relying solely on fast or cheap solutions with outdated methods will continue to produce flawed translations that fail to capture the richness of Japanese language and culture.
Machine translation, even with recent advancements, continues to struggle with the complexities of the Japanese writing system. The use of kanji, hiragana, and katakana, each with unique functions and nuances, presents a significant hurdle. For example, a single kanji can have multiple meanings based on context, making it difficult for AI to consistently choose the correct interpretation. The way Japanese handles foreign sounds using hiragana and katakana also causes problems since AI may not accurately translate the intended pronunciation.
Further complicating matters is the inherent difference in sentence structure. Japanese primarily follows a Subject-Object-Verb order, which clashes with the Subject-Verb-Object order common in many Western languages. AI systems trained predominantly on English and similar languages frequently struggle to adapt and produce accurate translations when the sentence structure differs so fundamentally. And that's not all. Japanese relies heavily on context, often leaving out subjects or employing different levels of formality. AI's difficulty in understanding these subtle contextual clues results in translations that can be ambiguous or incomplete.
Another significant challenge is polysemy, where one word can have multiple meanings. AI often fails to grasp this richness of language, leading to inaccurate translations. Similarly, the various particles like は, が, and を, which play key roles in determining grammatical relationships within sentences, are frequently misinterpreted. This often leads to grammatically incorrect or awkward translations in the target language.
Beyond grammatical challenges, cultural nuances pose additional difficulties. Expressions and idioms specific to Japanese often lack direct equivalents in other languages. Machine translation, often favoring literal translations, fails to convey the underlying cultural significance, resulting in inaccurate or irrelevant outputs.
The inclusion of OCR into the translation process can exacerbate problems. While OCR technology has improved in character recognition, especially for simpler characters, it frequently stumbles when encountering complex kanji or intricate handwriting styles. This further compounds errors in translation.
The constant demand for fast and cheap translation services can also contribute to the issue. In a rush to deliver fast translations, often at lower cost, providers may rely on less sophisticated AI models, which in turn produces inaccurate and unreliable results, especially with a language as complex as Japanese.
Ultimately, while AI translation technology has made considerable strides, it still often requires significant post-editing by humans. This highlights the continuing necessity of human linguistic expertise, even as AI tools become increasingly prevalent. It's a testament to the fact that human language, especially nuanced languages like Japanese, remains incredibly complex, posing a challenge that current AI technologies are still working to overcome.
Lost in Translation Why AI Language Models Still Can't Challenge Obvious Linguistic Truths (A 2024 Analysis) - AI Translation Fails to Bridge Cultural Gaps in Arabic Local Dialects
AI translation, while improving, still struggles to accurately convey the richness and nuances of Arabic, especially its various dialects. This is largely because current AI models, even advanced ones, often miss the mark when it comes to understanding cultural context and idiomatic expressions common in specific Arabic-speaking communities. The resulting translations can be inaccurate and misleading, failing to capture the intended meaning of the original text.
This gap stems from the difficulty AI has in understanding the subtle meanings and cultural context that human translators readily grasp. Furthermore, AI models are often trained on limited datasets that don't adequately represent the diversity of Arabic dialects, further hampering their ability to produce accurate and culturally relevant translations.
Despite advancements, AI translation technology has yet to fully bridge the cultural gap in Arabic. While AI tools can provide fast, cheap translations, those seeking accurate and culturally appropriate communication across dialects often find themselves needing human intervention. The need for human linguists to ensure context and cultural understanding remains a key challenge for future development in this area.
AI translation systems, while showing promise in handling standardized languages, encounter significant challenges when dealing with the diverse range of Arabic dialects. The vast array of dialects across different regions and even within countries presents a complex landscape for AI to navigate, often leading to inaccurate translations. For example, a speaker from Cairo might struggle to fully understand someone from Beirut, highlighting the inherent difficulty in creating a universal translation solution for Arabic.
These systems frequently default to Modern Standard Arabic (MSA) when faced with dialectal variations. While MSA serves as a unifying standard, this approach can result in translations that sound overly formal or fail to capture the nuances of local expressions and idioms. This disconnect between formal and colloquial language can lead to significant misunderstandings and inaccuracies in communication.
Interestingly, some Arabic phrases and idioms can be perplexing even for native speakers when translated literally by AI. This observation further underscores the limitations of current AI in grasping the subtle linguistic complexities that lie beyond the realm of standardized grammar and vocabulary.
The integration of Optical Character Recognition (OCR) into Arabic translation also presents hurdles due to the intricate nature of the Arabic script. The presence of diacritical marks that can alter a word's meaning often poses a challenge for OCR accuracy. This results in frequent misinterpretations and ultimately flawed translations.
Furthermore, culturally embedded references in Arabic dialects frequently elude AI systems, often leading to translations that are not only factually incorrect but also culturally insensitive. This highlights the critical role that cultural context plays in accurate translation, a factor that current AI technologies often fail to adequately address.
The pursuit of affordable translation services can inadvertently compromise translation quality. Many companies, in an effort to offer cheaper rates, utilize less refined AI models, particularly impacting the translation of Arabic dialects where a deeper understanding of cultural nuances is crucial.
Another area of struggle for AI lies in handling the gendered language specific to Arabic dialects. The intricate system of grammatical gender, which differs across dialects, proves challenging for AI models, leading to awkward or misleading translations.
Researchers also suggest that the phonetic diversity between dialects poses a significant challenge for AI-driven translation. Sounds and pronunciations unique to a particular dialect can be difficult to map to other dialects, particularly when dealing with spoken language translation.
Fast translation technologies, prioritizing speed over accuracy, often sacrifice the deeper understanding needed to handle dialectal variations. The need for swift translation frequently leads to overlooking the complex nuances and cultural context that are vital for effective communication.
While advancements in deep learning have been significant, AI still lags in comprehending the pragmatic aspects of language. For truly effective translation of Arabic dialects, AI needs to incorporate not only linguistic knowledge but also a rich understanding of the cultural context. Only then can AI hope to overcome the challenges that remain and deliver truly insightful and accurate translations.
Lost in Translation Why AI Language Models Still Can't Challenge Obvious Linguistic Truths (A 2024 Analysis) - One Model Cannot Handle 7000 Languages The Data Bias in Current Translation AI
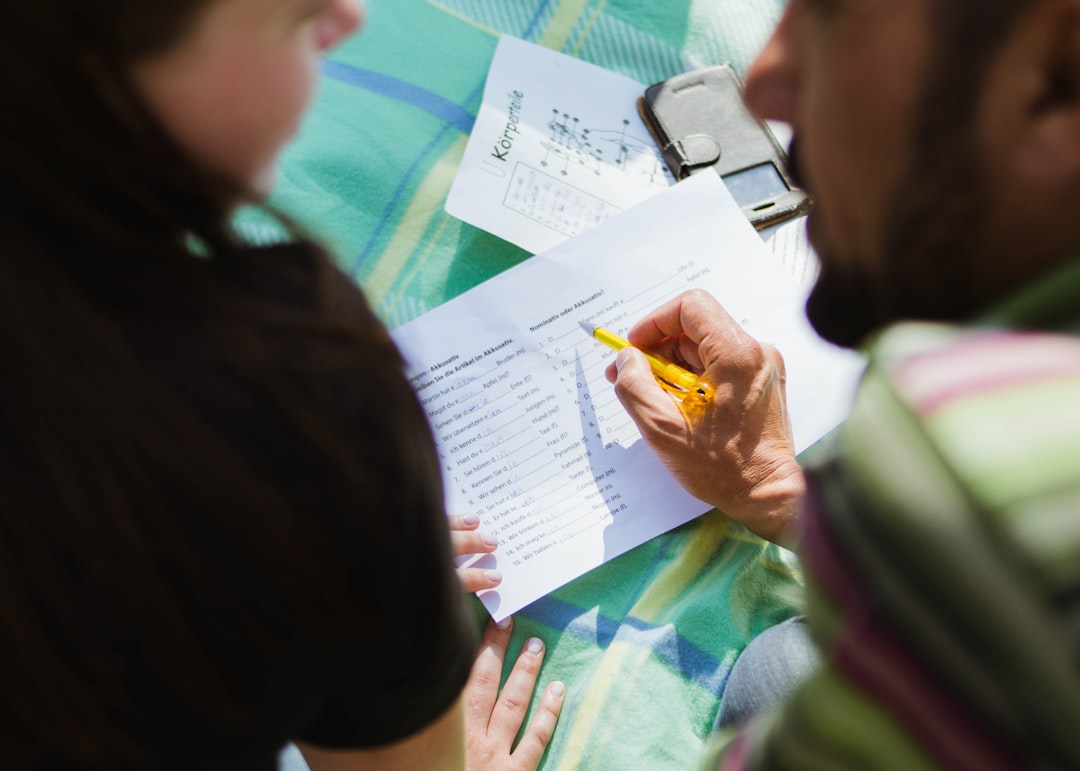
The sheer number of languages spoken globally—estimated at 7,000—highlights a significant shortcoming of current AI translation systems. These systems, often trained on a limited and biased selection of data, struggle to accurately handle the full spectrum of human languages. Many lesser-known languages lack the vast quantities of high-quality textual data needed for effective AI training, leading to translations that can be nonsensical or fail to capture the subtle details of the language. This problem is particularly evident when AI models, primarily trained on major languages like English, encounter less common languages. Their performance suffers as they are unable to process the complexities of the new language and fail to produce meaningful translations.
Furthermore, the over-reliance on automated translation technologies can worsen existing biases. These systems frequently inherit and even amplify harmful biases present in their training datasets, leading to skewed and potentially harmful outcomes in translated materials. This becomes increasingly problematic as demand for quick and affordable translations rises, leading to the need for careful consideration regarding the use of these systems. It emphasizes the continued importance of human expertise in the translation process, especially in ensuring that translations are both accurate and culturally sensitive. Striving for fast and inexpensive translation should not compromise the crucial need for accurate and respectful translation across a variety of languages.
The current landscape of AI translation reveals a significant limitation: its inability to effectively handle the vast diversity of human languages. While impressive in handling major languages like English, these models often falter when confronted with the approximately 7,000 languages spoken globally. This is primarily due to a severe imbalance in the training data. Many languages, particularly those with smaller speaker populations, lack sufficient high-quality textual data to effectively train robust large language models. Consequently, the models end up with a skewed understanding of language, primarily focusing on English and other widely spoken languages.
This data bias has several implications for translation quality. Firstly, the limited data for many languages means the models often fail to capture the full richness and complexity of those languages. They tend to simplify expressions and often miss nuances like idioms and culturally specific terms, resulting in translations that lack depth and authenticity. This problem is particularly evident in languages with complex grammatical structures or intricate writing systems. Take Arabic dialects, for example – the variations in these dialects can be so significant that AI, often defaulting to Modern Standard Arabic, produces translations that don't capture the local flavour.
Furthermore, the emphasis on speed and affordability in the AI translation field has often led to a compromise on accuracy, especially when dealing with less common languages. Many services prioritize speed, employing models trained primarily on easily accessible data like religious texts or Wikipedia entries. While this strategy might work well for common phrases, it often neglects the vast linguistic diversity of the world. Additionally, the use of OCR technology for translation further exacerbates the issue, especially for languages with non-Latin scripts. The intricate nature of these scripts can lead to errors in character recognition, impacting the quality of the translated output.
Finally, the cultural context plays a crucial role in accurate translation, but current AI models struggle to fully grasp this. Cultural references, politeness systems, and social norms that are embedded within a language are often missed by the AI, resulting in translations that can sound unnatural or even offensive. This is especially true for languages with intricate systems of politeness like Japanese and Korean. The limited ability of the models to truly understand these cultural nuances reinforces the importance of human expertise in translation.
While AI translation technology has undeniably made remarkable progress, the sheer diversity of human language remains a formidable challenge. The reliance on limited and often biased training data hinders the ability of AI to accurately capture the subtleties and variations present across languages. The limitations highlighted above suggest that developing AI capable of bridging linguistic and cultural divides requires a more holistic approach, one that goes beyond simple pattern recognition and incorporates a deeper understanding of the nuances of individual languages. Until this goal is achieved, the role of human translators in ensuring the accuracy and cultural sensitivity of translations remains vital.
Lost in Translation Why AI Language Models Still Can't Challenge Obvious Linguistic Truths (A 2024 Analysis) - English Language Training Sets Create Translation Problems for African Languages
The development of AI translation models has been heavily influenced by English-language data, leading to significant problems for African languages. This dominance of English in training sets has resulted in inaccuracies and misinterpretations of the nuanced linguistic features present across the continent's approximately 2,000 languages. While some specialized initiatives, such as GhanaNLP and Lelapa AI, have emerged to address the specific needs of African languages, a crucial gap remains: a lack of native speaker involvement in the AI development process. This limits the ability of these tools to capture the intricate cultural and linguistic nuances that are essential for accurate translation. Attempts to bridge this divide and foster more equitable access to AI translation include efforts like the African AI Network. However, these initiatives face hurdles in achieving true inclusivity, highlighting the need for a deeper understanding and respect for the unique structures and cultural contexts embedded within African languages. Without a more concerted focus on these aspects, the hope for AI-driven translation that effectively facilitates cross-cultural communication across Africa remains largely unfulfilled. The allure of cheap and fast AI translation must be tempered with the awareness that true comprehension of complex languages needs a nuanced approach.
AI translation systems, as they stand in late 2024, face a significant hurdle when it comes to accurately translating African languages. This difficulty arises primarily due to a scarcity of training data specifically focused on these languages. The models often struggle to grasp the subtle nuances of these tongues, which can be problematic in vital areas like education and healthcare where precise communication is paramount.
The sheer diversity of African languages—with thousands of distinct tongues and dialects—presents a major challenge for AI models, which tend to be primarily trained on languages like English. This bias in training data leads to an inherent disadvantage for African languages, making it hard for these models to adequately represent their unique linguistic features.
Compounding this problem is the lack of standardized writing systems for many African languages. This is especially true for languages that are primarily spoken rather than written, or those that have recently transitioned to written forms. The absence of a consistent written representation poses a significant obstacle for AI models, which often rely on textual data for training.
When we use "quick-and-dirty" translation services designed for fast turnaround, we often encounter problems like entire sentences being flipped or parts completely missing. This demonstrates that the quest for speedy translations can unfortunately sacrifice the intricate subtleties of meaning embedded in African languages.
AI's tendency to rely on literal translations often leads to difficulties with the many colorful idiomatic expressions found in African languages. These expressions, when mistranslated or ignored, can significantly change the intended meaning of the original text, creating misunderstandings.
OCR technology, while capable of handling character recognition in many instances, can encounter problems with African scripts, particularly those that don't use Latin characters. This can result in inaccurate character recognition, leading to further issues and compounding existing problems within AI-based translation outputs.
Furthermore, the pursuit of inexpensive translation services often results in the use of outdated or less sophisticated language models that aren't optimized for African languages. This typically leads to a higher number of errors, especially concerning grammatical accuracy and understanding the full context of a passage.
Variations in dialects within a single language pose an added challenge, as AI models may struggle to differentiate between these variations. This often renders a "one-size-fits-all" approach ineffective, potentially leading to confusion and miscommunication.
The issue of data bias, previously discussed, is particularly pronounced when it comes to African languages. Historically marginalized languages often lack representation in AI datasets, exacerbating the existing problems with accuracy and preventing culturally relevant translations.
Ironically, AI translation tools can sometimes contribute to a harmful homogenization effect, potentially diminishing the unique linguistic and cultural identities of African languages. This can result in a loss of the richness and originality inherent in the diverse tapestry of African language expressions and their meanings.
This analysis suggests that there are still significant roadblocks to overcome before AI translation technology can effectively bridge the gap for African languages. A more conscious approach to data collection, model development, and recognizing the importance of dialectal diversity is required to create more accurate and culturally sensitive translations for the many languages of Africa.
Lost in Translation Why AI Language Models Still Can't Challenge Obvious Linguistic Truths (A 2024 Analysis) - Why Low Resource Languages Need Human Translators Until 2030
The landscape of AI translation, despite advancements, still poses significant hurdles for low-resource languages, suggesting that human translators will likely remain crucial until at least 2030. While AI has shown promise in translating widely used languages, its capacity to handle the nuances of lesser-known languages remains limited. This is due in part to a lack of comprehensive training data, hindering AI's ability to grapple with complex grammar, subtle cultural implications, and dialectal variations. The drive towards quick, affordable translations often compromises the accuracy and depth that are essential, further highlighting the need for human input. Particularly for languages at risk of decline, human translators play a critical role in preserving linguistic heritage and ensuring culturally appropriate communication. Until AI systems can effectively bridge this gap in understanding, the expertise of human translators will be vital in ensuring accurate and sensitive translations.
Here are ten points highlighting why, despite advancements in AI, human translators will likely remain essential for low-resource languages until at least 2030:
1. AI models heavily rely on vast amounts of training data, which is simply unavailable for many languages. Thousands of languages have little to no digital presence, making it impossible for AI to learn their intricate structures. This lack of data leads to frequent errors and inaccurate translations.
2. Low-resource languages often exhibit unique grammatical structures that differ significantly from dominant languages used in AI training. The specific grammar rules and markers, like complex tone systems or intricate verb conjugations seen in some African languages, are hard for current AI models to grasp.
3. Cultural nuances and idioms are fundamental parts of language, yet AI tends to struggle with their interpretation. These culturally specific phrases often lose their meaning when translated literally, a problem that human translators can overcome with their knowledge of local contexts.
4. The complex sentence structures and extensive verb conjugations present in several low-resource languages pose a significant hurdle for AI. Languages with rich morphological systems, like Basque, are challenging because small changes can significantly alter the meaning. These intricacies can be tricky for AI to follow consistently.
5. Languages like Vietnamese heavily rely on diacritical marks that influence a word’s meaning. AI models that fail to incorporate these markings can misinterpret entire words, demonstrating a critical area where human oversight is needed to maintain accuracy.
6. Language and culture are intertwined, and human translators possess a deep understanding of this connection. They excel at contextualizing translations, while AI models still struggle, especially in languages where cultural practices and history are closely linked to language use.
7. AI translation systems tend towards literal translations, resulting in awkward phrasing and misinterpretations. Human translators, on the other hand, prioritize contextually relevant translations that resonate with the target audience, preserving the original text’s intended tone and nuance.
8. Many low-resource languages lack standardized writing systems. This lack of formalization makes it difficult for AI to learn from consistent textual data. Human translators can bridge this gap by interpreting spoken dialects and understanding variations not yet codified in written form.
9. Despite progress in OCR, the technology frequently fails to interpret complex scripts or languages that employ unique typesetting. This weakness highlights the ongoing limitations of AI’s ability to effectively process text in low-resource languages that don't have readily available scripts.
10. Humans have a keen ability to recognize ambiguous meanings and underlying tones that AI models tend to miss. This crucial insight plays a vital role in high-quality translation, especially in specialized domains like legal, medical, or literary contexts where precision is paramount.
The continued need for human translators in low-resource languages underscores the limitations of current AI approaches. It highlights the profound complexities of human language that extend far beyond simple word-for-word translation and emphasize the importance of human understanding and cultural sensitivity in creating truly effective and meaningful translations.
More Posts from aitranslations.io: