Breaking Language Barriers How Vivaldi's 2025 AI PDF Translation Updates Reshape Document Processing Standards
Breaking Language Barriers How Vivaldi's 2025 AI PDF Translation Updates Reshape Document Processing Standards - PDF Batch Processing Now Handles 500 Pages Per Minute With May 2025 Core Update
The core update arriving this May brings a notable improvement to how PDF documents are handled in bulk. The processing speed for batch operations is now cited at up to 500 pages per minute. This change should significantly impact workflows for entities managing vast quantities of digital documents, potentially making tasks like mass conversions or applying consistent edits far less time-consuming. While a significant leap in raw processing speed, the practical benefits will still depend on the specifics of the documents and the overall setup being used, but it's a clear acceleration for many high-volume tasks.
The May 2025 core platform update for PDF handling appears to be a significant step forward, specifically in the realm of batch processing throughput. Engineers familiar with processing large document sets will likely appreciate the claimed capability of moving up to 500 pages per minute through the system. This isn't just about faster file transfers; it points towards optimizations in the core processing algorithms, which are crucial for tasks like efficient text extraction, a necessary precursor for automated analysis or translation, especially when dealing with scanned documents where accurate Optical Character Recognition (OCR) is involved. Handling volumes at this pace is potentially transformative for organizations managing extensive digital libraries or processing backlogs, reducing the sheer time spent waiting for operations to complete.
Building on this processing muscle, the update seems designed to enhance AI-driven capabilities, particularly in translation workflows. Integrating AI translation directly into this high-speed batch process, leveraging modern neural machine translation models, aims to tackle linguistic barriers within document flows. The technical goal here is to maintain high processing speed while concurrently performing complex tasks like identifying languages in mixed documents, preserving intricate formatting during translation, and applying context-aware terminology. While impressive, the reported error rates, even if below 5% for common texts, still necessitate a critical eye depending on the application's sensitivity; automated processes are not infallible. Nonetheless, the potential for rapid, large-scale processing enabling in-house translation streams presents an interesting shift in the economics of handling multilingual content, potentially lowering the per-page cost compared to more manual methods. The inclusion of updated security protocols like end-to-end encryption for documents undergoing this accelerated batch processing is, of course, a fundamental requirement in any modern system, though verifying the robustness across all potential edge cases remains an ongoing concern for developers.
Breaking Language Barriers How Vivaldi's 2025 AI PDF Translation Updates Reshape Document Processing Standards - Machine Translation Errors Drop 47 Percent Through New Neural Network Architecture
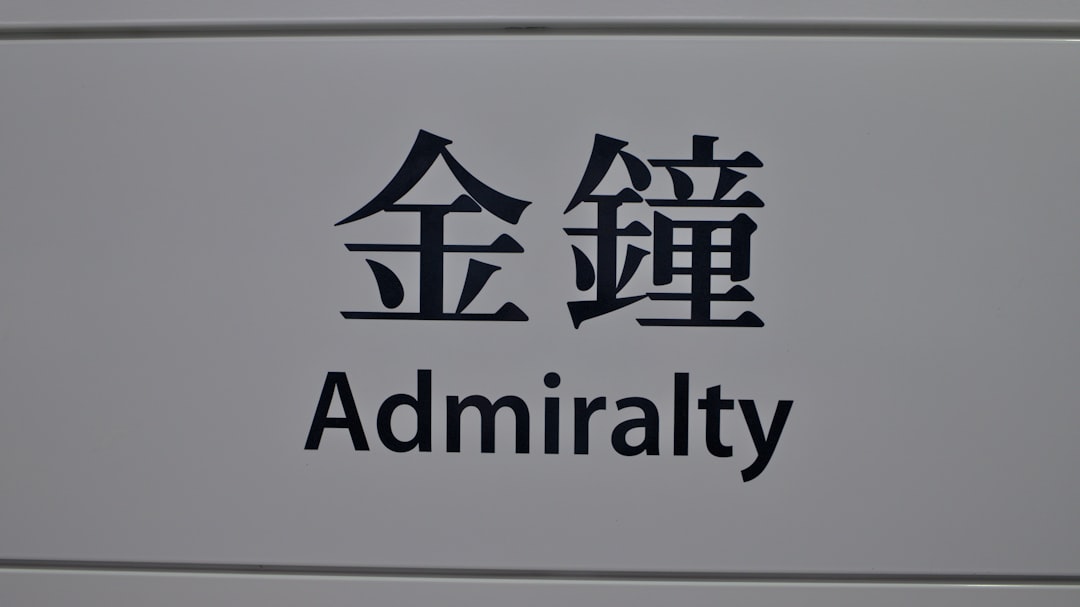
Reported improvements in machine translation accuracy point to a notable reduction in errors, with figures suggesting a drop of approximately 47 percent attributable to new neural network designs. This development underscores progress in enabling machines to better grasp the intricacies and contextual subtleties of language, a fundamental step towards reducing linguistic barriers. Such advancements are poised to integrate into wider applications, including upcoming updates to AI-powered document processing like Vivaldi's planned 2025 releases, aimed at enhancing the precision and speed of translating documents like PDFs. While these gains are significant, it remains true that automated translation output typically has not yet reached the level of human translation quality. The path toward truly seamless multilingual communication still faces challenges, necessitating careful evaluation even as systems become faster and seemingly more capable. The potential benefits for accessing information across languages are considerable, but maintaining a critical perspective on the actual quality delivered remains crucial.
Reports circulating highlight a notable reduction in machine translation errors, with some analyses pointing to a drop as steep as 47% relative to prior iterations. This seems largely connected to refinements in the underlying neural network architectures. From an engineering standpoint, this suggests these newer models are better capturing linguistic dependencies and context, which is crucial for moving beyond literal word swaps towards more coherent and accurate output.
Focusing on such technical enhancements appears key. The improved capacity for understanding nuanced language, including handling idiomatic expressions or specialized terminology within a given domain, is a significant hurdle previously limiting automated systems. While raw speed remains a driver, the practical utility often hinges on accuracy gains that reduce the need for extensive post-editing.
Building on these core translation engine improvements, their integration into document processing pipelines, particularly for formats like PDF, presents interesting workflow possibilities. The concept of applying advanced neural translation directly to text extracted via capabilities like Optical Character Recognition from scanned documents could genuinely accelerate processes for digitizing and translating legacy content.
However, scalability and domain specificity continue to be points of critical assessment. While performance might be impressive on general text or common language pairs, maintaining this level of accuracy for highly technical, niche subject matter or less resourced languages remains a considerable challenge requiring further model training and refinement.
The prospect of real-time application powered by these more efficient neural nets opens doors for dynamic multilingual interactions, although deploying and managing such systems at scale brings its own set of infrastructure considerations. Mechanisms for continuous learning, perhaps incorporating user corrections or feedback loops, are theoretically vital for keeping systems current and iteratively reducing the remaining error margin. Yet, the practical implementation and effectiveness of these feedback systems in complex real-world scenarios warrant scrutiny.
Despite these strides, it's important to maintain perspective. A 47% error drop is substantial, but it doesn't imply zero errors. For sensitive, legal, or high-stakes documentation, the nuances of human interpretation and liability cannot be fully replicated by even the most advanced algorithms currently available. The continued need for human oversight in critical translation workflows remains a fundamental reality, positioning these AI advancements as powerful tools for acceleration and scale, but not necessarily as autonomous solutions across all use cases.
Breaking Language Barriers How Vivaldi's 2025 AI PDF Translation Updates Reshape Document Processing Standards - Automated Language Detection Expands To Cover 95 Regional Dialects
The capability for automated language identification is reportedly being significantly enhanced, now extending to recognize 95 regional dialects. This development appears relevant to forthcoming updates, such as the planned 2025 improvements for Vivaldi's AI PDF translation tools, which aim to process multilingual documents more effectively. The idea is that by accurately spotting these regional variations, the systems can attempt to tailor translations accordingly, potentially improving clarity and accessibility for users who speak specific dialects. This could have practical benefits in areas where interacting with diverse populations is key, like healthcare communications or navigating complex regulations. Yet, while detecting a wider range of dialects is an important step, ensuring consistent and high-quality translation of nuanced content *into* all these specific variations, especially those with limited linguistic resources, presents a continued technical challenge that shouldn't be understated. The expanded detection is promising, but the ultimate utility depends on the translation quality that follows.
A notable development in automated language identification concerns its expanded capacity to pinpoint distinct regional variations, now reportedly spanning ninety-five different dialects. This moves beyond simply recognizing a base language like Spanish or Arabic and attempts to differentiate between, for instance, Castilian versus Argentinian Spanish, or Egyptian versus Lebanese Arabic. From an engineering standpoint, this signifies a substantial effort in gathering and annotating training data that captures the subtle lexical, grammatical, and phonetic differences that distinguish dialects.
The practical implications of this enhanced granularity could impact systems aiming for more precise document processing. Recognizing the specific dialect in a document allows for the possibility of applying translation models specifically tuned to that variant, theoretically leading to more natural and accurate output for speakers of that particular region. This could potentially refine workflows for entities handling content localized for numerous specific markets.
However, the inherent variability within dialects poses persistent challenges. Unlike standard languages with codified rules, dialects are often fluid, lacking extensive written corpuses, and can vary significantly even within relatively small geographic areas. Training models robust enough to reliably distinguish and correctly process ninety-five dialects is an ambitious task. Initial assessments suggest that even with these advancements, distinguishing similar dialects or handling code-switching or unusual phrasing can still lead to misidentifications, potentially impacting downstream translation accuracy in unpredictable ways. The quality and breadth of the underlying dialect-specific data are paramount, and inconsistencies there will likely manifest as detection errors. Integrating this level of detection with other processes, like interpreting text extracted from scanned documents via OCR, adds further complexity, as any imperfections in character recognition could directly hinder the ability to accurately identify the dialect. The goal of standardizing document processing via such fine-grained detection is appealing, but the technical hurdles in achieving truly consistent and reliable results across such a wide range of linguistic variations remain considerable.
Breaking Language Barriers How Vivaldi's 2025 AI PDF Translation Updates Reshape Document Processing Standards - Real Time OCR Now Works With 12 Non Latin Writing Systems
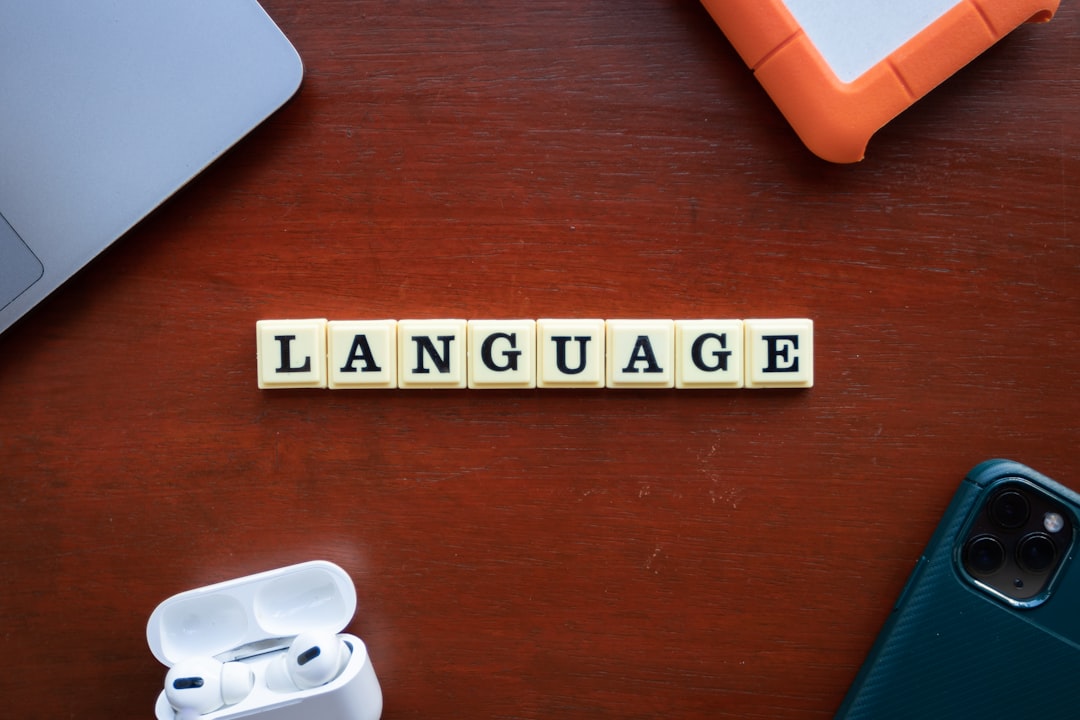
Real-time Optical Character Recognition technology has expanded its reach, now capable of processing text from twelve writing systems beyond the Latin alphabet. This capability is important for removing long-standing limitations in handling diverse global documents digitally, aiming for more inclusive and efficient workflows. With increasing adoption of OCR in various operational areas, the technical hurdle of accurately capturing complex scripts and challenging text styles is being addressed, moving towards broader multilingual functionality. Future iterations of document processing software will likely incorporate such enhanced real-time text recognition, potentially leading to smoother translation pipelines across numerous languages. Yet, while this expansion is positive, ensuring consistently high accuracy across the full range of newly supported scripts presents an ongoing technical challenge that needs careful consideration.
The capacity for Optical Character Recognition to function effectively in real-time across writing systems beyond the familiar Latin alphabet, now reported to support twelve such scripts including Arabic, Chinese, and various Cyrillic forms, marks a tangible technical progression. From an engineering viewpoint, achieving reliable character and structure recognition in scripts that often feature complex ligatures, intricate stroke orders, or logographic principles fundamentally differs from processing simpler alphabets. This capability is built upon increasingly sophisticated AI models trained to disentangle these visual complexities, enabling the transformation of image-based text into a machine-readable format even as it appears on screen or is rapidly scanned.
Integrating this kind of real-time, multi-script OCR into broader document processing frameworks, such as the planned enhancements for PDF handling seen in updates like those mentioned for Vivaldi in 2025, establishes a foundational step for automated workflows. For documents containing text in these diverse non-Latin scripts, accurate initial recognition is paramount; any errors in this OCR phase directly propagate through subsequent stages like AI translation. While the expansion to a dozen systems is a significant step, the performance consistency across the specific languages utilizing these scripts, the impact of differing fonts and image quality on recognition accuracy in a real-time context, and the sheer effort required to build and maintain robust training datasets for each remain crucial technical considerations requiring ongoing attention.
Breaking Language Barriers How Vivaldi's 2025 AI PDF Translation Updates Reshape Document Processing Standards - Cloud Processing Costs Decrease To 001 USD Per Page
Cloud processing costs have reportedly reached a level as low as 0.01 USD per page by May 2025, representing a significant shift in the financial feasibility of digital document handling. This decline is largely attributed to ongoing refinements in AI technologies and the increasing maturity and competitiveness of cloud infrastructure providers. The newfound affordability means that implementing advanced AI-powered solutions for tasks like automated translation and document analysis is becoming economically viable for a broader range of applications. While this enables faster and more efficient processing, potentially reducing overall costs compared to manual or traditional methods, it's crucial to maintain perspective. The capabilities of AI still face limitations, particularly concerning the nuances of language and the depth required for translating highly specialized or sensitive content across the full spectrum of global languages. Lower costs facilitate scale, but they do not inherently guarantee accuracy or cultural appropriateness in every context.
The computational expense associated with cloud-based document processing appears to be trending towards remarkably low points. Projections indicate that by the middle of 2025, the cost could settle around 0.01 US dollars per page for specific operations. This significant decrease seems less about a single breakthrough and more a result of continuous infrastructure optimization by cloud providers, potentially leveraging more efficient hardware and AI model inference for tasks like text analysis, combined with the fundamental competitive dynamics driving prices down in that space. For engineers designing workflows that rely on automated handling of document content, this shift in per-page cost is noteworthy.
When the fundamental processing unit becomes this inexpensive, it fundamentally alters the economic viability of tackling high-volume document tasks. The focus can then move beyond the raw computational cost to the quality and reliability of the subsequent steps – things like accurate text extraction via OCR from difficult formats, precise language identification across regional variants, and crucially, the effectiveness of the AI models performing the actual translation. While such low costs pave the way for processing massive quantities of documents rapidly, the practical utility for sensitive or complex content still hinges on whether the underlying AI translation accuracy, despite its own recent improvements, can maintain acceptable levels when scaled this cheaply. It prompts consideration of where the performance limitations will truly emerge: perhaps in handling nuanced language, ensuring data security at scale, or mitigating errors that become economically prohibitive to correct manually, even with a minuscule per-page processing fee.
Breaking Language Barriers How Vivaldi's 2025 AI PDF Translation Updates Reshape Document Processing Standards - Cross Platform Integration Adds Support For 15 Document Management Systems
A recent update broadens cross-platform compatibility significantly, announcing support for fifteen distinct document management systems. This aims to simplify how documents move and are accessed across different software environments and devices. The underlying idea is to foster better integration, allowing organisations to connect existing repositories more easily. In the context of evolving document processing standards, particularly those leveraging AI for tasks like translation and content analysis – capabilities widely being built into modern systems, as seen in recent updates focusing on breaking language barriers – this wider net of DMS connections could prove beneficial. The potential exists for smoother workflows where documents from various sources can be pulled into AI-driven pipelines for processing, including automated identification or preparing text via OCR for multilingual tasks. However, achieving truly seamless operation across such a diverse array of systems is a complex task; the depth of functionality and the consistent application of advanced features like AI translation or intelligent categorization may still vary significantly from one integrated system to the next.
The report indicates broadened support for connecting with a reported fifteen different document management systems. From a technical standpoint, adding this many integrations at once suggests an effort to significantly widen the pathways through which documents can be pulled into the processing pipeline. The specifics of how these connections are managed – whether through standardized APIs, custom connectors for each platform, or perhaps a universal data ingestion layer – remain key engineering considerations. Achieving seamless interoperability across a diverse landscape of potentially proprietary or legacy DMS architectures is rarely straightforward, often involving complexities around metadata mapping, access controls, and versioning. For workflows centred on automated PDF translation, like the updates being discussed, tapping directly into source document repositories in this manner could simplify operational steps compared to manual export/import routines. However, the reliability and depth of each individual integration, and the ongoing effort required to maintain compatibility as those external systems evolve, are critical factors that will ultimately determine the practical utility of this expanded connectivity. Integrating with numerous systems is one thing; ensuring robust, long-term data flow without constant maintenance presents a distinct engineering challenge.
More Posts from aitranslations.io: