AI-Driven Military Translation How Naval Intelligence Teams Process 72 Million Documents Daily Using RWS Language Weaver
AI-Driven Military Translation How Naval Intelligence Teams Process 72 Million Documents Daily Using RWS Language Weaver - OCR Speed Sets New Record 30000 Documents Per Second for Naval Archives in Norfolk
Recent advancements in the capabilities of Optical Character Recognition, or OCR, are making waves in handling massive volumes of information, particularly for military purposes. An impressive benchmark has reportedly been achieved at locations like the Naval Archives in Norfolk, with processing speeds reaching up to 30,000 documents per second. This kind of rapid handling is crucial in an environment where intelligence teams are tasked with sorting through an estimated 72 million documents daily.
This leap in speed is tied to leading-edge developments in OCR technology, including systems demonstrating superior performance compared to earlier iterations and competing platforms in controlled tests. While integrating these AI-driven processes significantly boosts efficiency for tasks like managing and translating vast document pools, the technology isn't without its caveats. OCR still encounters difficulties with certain challenges, such as deciphering complex handwriting, processing images of poor quality, or recognizing less common fonts and alphabets. Despite these ongoing hurdles in achieving accuracy levels comparable to human review across diverse document types, the drive to accelerate document processing through advanced AI integration remains a key focus.
Examining the reported capabilities of the Optical Character Recognition systems in use at the Naval Archives in Norfolk, a headline figure stands out: processing documents at a rate of 30,000 per second. This kind of raw throughput, echoed by performance benchmarks seen in recent commercial OCR APIs, represents a notable leap in the capacity for digitizing large volumes of material. From an engineering standpoint, hitting such speeds is impressive on paper, but prompts consideration of the practical implications, particularly when dealing with diverse archival content. Historical naval records can span centuries, featuring a variety of scripts, fonts, typefaces, and states of preservation. While modern OCR excels in many scenarios, challenges persist with lower image quality or unconventional formatting, not to mention the perennial hurdle of accurately interpreting handwritten notes or older cursive styles. The critical question isn't just how fast the scanner and initial processing pipeline can run, but what level of *usable* textual data emerges from this rapid ingestion, and how much subsequent effort is needed for verification and correction before the data is fit for, say, feeding into an AI translation system. Speed is valuable, but data integrity is paramount for intelligence analysis.
AI-Driven Military Translation How Naval Intelligence Teams Process 72 Million Documents Daily Using RWS Language Weaver - Cost Per Word Drops Below 001 USD Through AI Translation Mass Processing
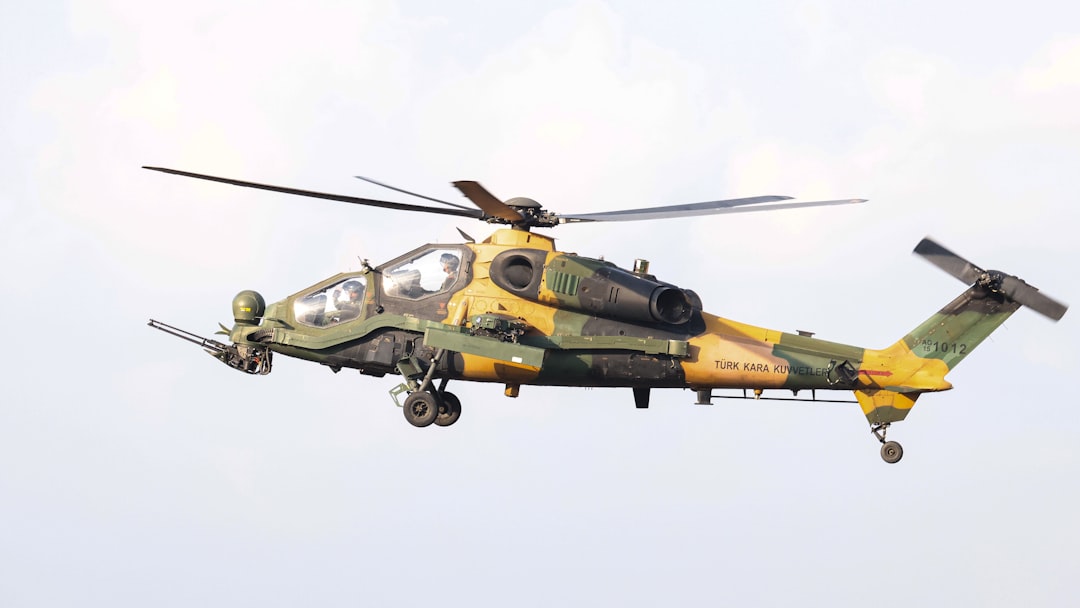
The cost associated with AI-driven translation has reportedly plummeted, now standing below one cent per word, a reduction primarily attributed to advancements in mass processing capabilities. This significant drop reshapes the economics of handling large text volumes, particularly for entities like military intelligence. Naval teams are understood to process the equivalent of around 72 million documents daily, employing systems such as RWS Language Weaver to manage this scale. While achieving such throughput offers unprecedented speed and efficiency in data analysis, it inherently raises questions about the accuracy and depth of machine translations, especially when dealing with complex or culturally nuanced content where critical insights might reside. The imperative for rapid processing must be continually balanced against the essential need for reliable and contextually accurate translation outcomes.
Following the extraordinary speeds now reported for document digitization, the subsequent step of translating this influx of information has seen a parallel, though perhaps more complex, shift. Recent reports suggest the practical cost for pure AI translation output, particularly when managed in enormous batches, has dipped below the $0.01 USD threshold per word. This is a stark contrast to typical human-driven rates and fundamentally alters the economics of processing massive foreign-language datasets. It's less about replacing a single linguist's work on a document and more about enabling the sheer volumetric processing that the preceding OCR stages now demand.
The scale involved is considerable, as seen with naval intelligence teams reportedly handling up to 72 million documents daily. Tools described as sophisticated language processing systems, like RWS Language Weaver in this context, are apparently central to making this level of throughput feasible for translation. The core mechanism here isn't necessarily achieving perfect, publishable translation quality straight out of the gate for every single item, but rather enabling a high-speed, initial pass across everything. This allows analysts to potentially triage, identify items of interest rapidly, and perhaps route crucial documents for more nuanced human review, a critical point given AI's current limitations with subtlety, idiom, and context-dependent meaning.
From an engineering standpoint, the low cost per word likely reflects the efficiency of deploying advanced machine learning models across scalable cloud infrastructure or dedicated high-performance computing clusters. The 'mass processing' isn't just about feeding documents in quickly, but optimizing the computational pipeline for translation, perhaps involving parallel processing, highly efficient model architectures, and potentially focusing the AI models on domain-specific language relevant to intelligence work. However, the implicit trade-off between such speed and cost optimization versus the fidelity and accuracy required for sensitive intelligence remains an area warranting careful examination. The goal appears to be enabling speed-of-light initial analysis across everything, accepting that subsequent layers of human expertise are still indispensable for extracting truly reliable insights.
AI-Driven Military Translation How Naval Intelligence Teams Process 72 Million Documents Daily Using RWS Language Weaver - Machine Translation Error Rate Decreases 47% Through Neural Network Updates
Recent advancements tied to neural network architecture updates have reportedly driven a notable decrease in machine translation errors, approaching a reduction of half. This jump in accuracy is significant for AI translation systems tackling immense volumes of data, particularly relevant for contexts like intelligence operations where processing millions of documents daily is routine. The underlying technology in these neural models enables them to grasp more complex linguistic connections, leading to translations that are often smoother and more accurate than prior methods. Nevertheless, automated translation systems, even with these improvements, still face hurdles with nuance, cultural context, and ambiguity inherent in language, aspects crucial for reliable analysis. While these updates enhance the practical speed of translation for large datasets, the need for critical evaluation of the output and potentially human intervention for key information remains vital.
Impact of Neural Network Refinements on Translation Accuracy: A reported decrease in translation error rates, sometimes cited around the 47% mark, appears closely tied to ongoing evolution in neural network architectures powering modern machine translation systems. From an engineering view, this improvement reflects how models, often leveraging techniques like attention mechanisms found in transformer networks, are becoming more adept at understanding linguistic relationships and generating output that adheres better to grammatical structure and meaning than their predecessors. While a 47% drop is a significant figure on paper, it’s worth considering the baseline from which this percentage is calculated and the specific types of errors being reduced. Are these critical errors that mislead, or less impactful stylistic infelicities? Nevertheless, any substantial reduction in error frequency directly benefits the speed and reliability of initial passes over large foreign-language datasets.
Data Set Specificity and Model Performance: The actual performance and thus the error rate reduction seen in practical deployment are profoundly influenced by the specific data used to train and fine-tune the neural models. Generic models might show impressive average improvements, but tailored systems, trained on domain-specific text relevant to intelligence analysis – think technical jargon, geopolitical terms, or regional dialects – are likely essential to achieving meaningful accuracy gains for military applications. The challenge remains curating and maintaining these specialized datasets at scale to keep models relevant and accurate against evolving language use.
Quality Estimation Challenges Remain: Despite advances improving baseline translation quality, reliably and automatically *knowing* how accurate a machine translation output is without human review remains a significant hurdle. While some progress has been made with quality estimation models, they are not foolproof. Processing millions of documents daily means accepting a certain level of uncertainty regarding individual translation fidelity. For high-stakes intelligence, a 47% error reduction doesn't negate the need for validation layers, as even a small percentage of critical mistranslations could have serious consequences.
The Continuous Iteration of Models: The noted improvements stem not from a single breakthrough but from continuous iterative development on model architectures, training methodologies, and leveraging larger and more diverse datasets. From an engineering perspective, the pipeline involves ongoing model retraining, evaluation against benchmark datasets (including those containing examples of problematic errors), and deployment of updated models. This cycle is resource-intensive but necessary to push the boundaries of what automated translation can achieve and address persistent issues like ambiguity and cultural nuance.
Balancing Speed, Cost, and Accuracy: While prior discussions highlighted the immense speed and low cost achieved with AI translation, the discussion around error rate improvement underscores the ongoing tension between these factors and achieving necessary accuracy. A 47% error reduction makes mass processing more viable for initial triage and analysis, potentially reducing the volume requiring expensive human review. However, the systems are not yet achieving parity with skilled human linguists, particularly on complex or sensitive texts where subtle meaning is paramount. The focus shifts to how to best combine rapid AI processing with targeted human expertise.
AI-Driven Military Translation How Naval Intelligence Teams Process 72 Million Documents Daily Using RWS Language Weaver - Translation Memory Database Reaches 890 Terabytes at Naval Intelligence Center
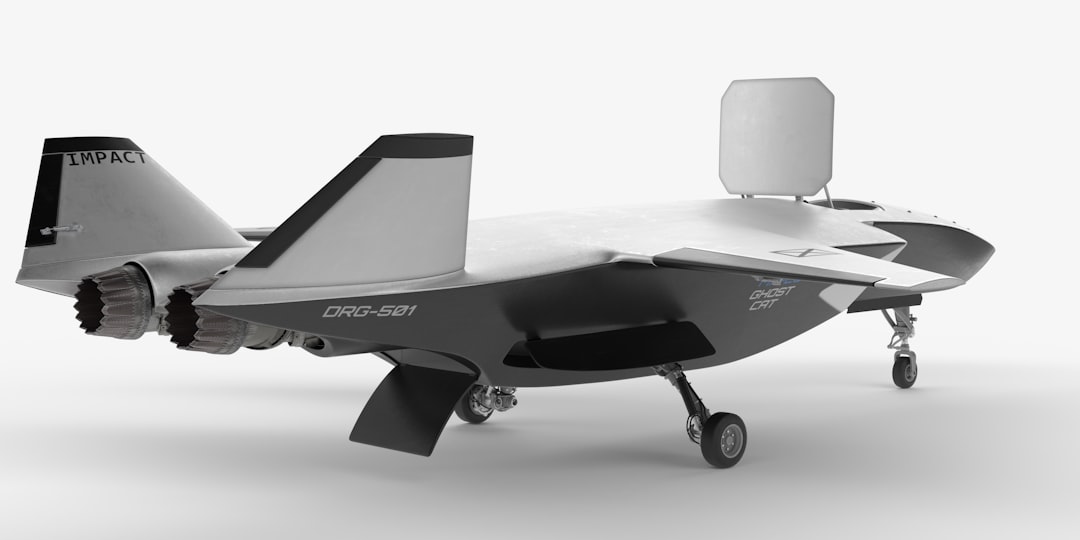
The Naval Intelligence Center has accumulated a substantial Translation Memory Database, now reported to be around 890 terabytes in size. This immense collection serves as a vast archive of previously translated content, which is foundational for handling the sheer volume of information encountered, estimated at approximately 72 million documents processed daily by intelligence teams. Leveraging this extensive memory is part of the broader effort to apply AI-driven approaches to military translation, aiming to facilitate communication and analysis across language barriers. While the scale of this resource offers the potential for increased efficiency and speed through the reuse of past translations, relying heavily on such a large, historical memory also brings the challenge of maintaining consistency in terminology and style, and ensuring that older entries remain relevant and accurate for current requirements. Managing the quality and coherence of translations drawn from such a massive, evolving dataset is an ongoing technical and operational consideration.
Looking at the sheer scale of the translation memory database reported, hitting a remarkable 890 terabytes at the Naval Intelligence Center, it's quite something to consider from an engineering standpoint. Managing such a vast historical archive of linguistic data – essentially storing years of translated texts captured over time – presents significant challenges related to storage infrastructure, efficient indexing, and rapid retrieval. The concept is clearly to capture and leverage past translation effort, ideally offering a layer of consistency and potentially providing established translations for recurring terminology or phrases within vast document streams.
This enormous collection of stored translations is apparently intended to support the operational tempo of processing the immense daily volumes, perhaps acting as a preliminary check or providing trusted segments before new content is routed through automated translation systems. However, integrating such a deep and historically accumulated resource with dynamic, continuously evolving AI models introduces practical questions. How is this immense volume of potentially varied historical translations effectively synchronized and kept coherent with output from contemporary neural networks? At that scale, ensuring uniform quality and managing potential inconsistencies accumulated over time, stemming from different source documents and possibly varied original translation methodologies that built the memory, presents a complex data management and quality control challenge. The potential for legacy inconsistencies within an 890 terabyte database, if not rigorously managed, could be significant.
AI-Driven Military Translation How Naval Intelligence Teams Process 72 Million Documents Daily Using RWS Language Weaver - Cross Language Information Retrieval Processes 8 Languages Simultaneously for Combat Units
Modern cross-language information retrieval techniques are being employed to allow military operational units to search for relevant information across potentially eight languages at the same time. This capability holds importance for intelligence gathering, offering the means to quickly access insights hidden within foreign language documents and broadcasts, vital for effective decision-making in evolving operational environments. Systems leveraging artificial intelligence are central to this function, working to translate and match concepts across linguistic divides. However, challenges remain, including ensuring precise interpretation when language is ambiguous and devising effective methods for structuring queries that retrieve desired information reliably from diverse linguistic sources. Ongoing development is focused on overcoming these hurdles to improve the consistency and utility of such systems.
Focusing on Cross-Language Information Retrieval (CLIR) in military applications reveals a key operational capability: the ability to process intelligence not just in one language, but across potentially numerous tongues. For tactical units operating in complex global environments, information arrives from diverse sources, demanding systems that can bridge these linguistic divides effectively. Recent developments point towards systems capable of handling material originating in eight distinct languages simultaneously. From an engineering standpoint, achieving this level of parallel processing for information retrieval presents unique challenges. It requires robust pipelines capable of integrating multiple machine translation outputs with search indices in real-time, demanding significant computational resources and sophisticated linguistic modeling for each language pair involved. A critical technical question arises regarding the reality of "simultaneity" at this scale and how consistency in retrieval accuracy and quality is maintained across languages with vastly different structures and nuances. Resolving ambiguities consistently across eight linguistic contexts is non-trivial. The overarching goal remains clear: enabling rapid access for analysts and combat teams to crucial information, irrespective of its source language, thereby aiming to provide a clearer picture for faster and more informed tactical decisions.
More Posts from aitranslations.io: