AI-Powered PDF Translation now with improved handling of scanned contents, handwriting, charts, diagrams, tables and drawings. Fast, Cheap, and Accurate! (Get started for free)
AI Translation Tools for Cross-Cultural Spotify Playlist Descriptions A Technical Guide
AI Translation Tools for Cross-Cultural Spotify Playlist Descriptions A Technical Guide - OpenAI Whisper Translation Now Works With Brazilian Portuguese Playlists On Spotify Backend
OpenAI's Whisper technology is now working with Brazilian Portuguese playlist descriptions within Spotify's infrastructure. This integration is intended to provide more contextually appropriate translations for Portuguese-speaking users, aiming to enhance how content connects with local audiences. The system uses Whisper's capabilities for text processing to automate translation, moving beyond manual methods. While AI translation tools like Whisper are powerful, their performance isn't always flawless; there have been reports of variable accuracy depending on the input quality or complexity, a factor to consider when relying on automated translation for nuanced content like playlist descriptions. Ultimately, the goal appears to be reducing language friction in content discovery.
The deployment of OpenAI's Whisper technology reportedly within Spotify's backend focuses on addressing the challenge of translating playlist descriptions, specifically targeting Brazilian Portuguese content. The intent behind this integration appears to be the pursuit of translations that are both technically accurate and better aligned with the cultural nuances expected by listeners in Brazil and other Lusophone communities. Utilizing a system like Whisper for this task is presumably aimed at preserving the intended meaning and local flavor often embedded in such descriptions, a perpetual hurdle in automated cross-cultural communication systems. The underlying process likely involves piping the original description text through the Whisper model to generate a translated version, intended to enhance the overall user experience by making discovery easier and more relevant for this significant market segment. Focusing efforts on Brazilian Portuguese underscores the scale of the potential audience and the practical necessity of lowering language barriers for accessing content.
AI Translation Tools for Cross-Cultural Spotify Playlist Descriptions A Technical Guide - OCR Translation For K-Pop Playlist Descriptions Now Available Through Spotify API
The availability of OCR (Optical Character Recognition) translation for K-Pop playlist descriptions through the Spotify API represents a notable technical step towards making global music content more accessible. This capability means the system can process and translate text that might not originate from standard keyboard input, potentially assisting with text presented as part of or alongside the description. Leveraging AI translation tools via the API appears designed to foster a more inclusive environment where users can more readily understand and engage with a wider variety of musical content, moving beyond language barriers. While this technology offers efficiency in bridging linguistic divides, it's worth acknowledging that automated translation, particularly for creative or culturally specific language common in music contexts, sometimes faces challenges in fully conveying the original nuance and intended tone. Ultimately, this move signifies an ongoing effort to employ artificial intelligence to facilitate smoother content distribution across linguistic boundaries.
The arrival of OCR translation capabilities specifically for K-Pop playlist descriptions, accessible potentially through the Spotify API, presents an intriguing technical pathway. From an engineering perspective, this capability suggests mechanisms for pulling not just the standard text descriptions but also potentially processing associated visual elements, like cover art or promotional banners sometimes linked or embedded within playlist contexts, where descriptive text might reside not as searchable Unicode but as part of an image file. The integration via an API implies a programmatic method for developers or internal systems to interact with this content, extracting the image, applying OCR to convert the embedded text, and then feeding that extracted text into an AI translation engine. This could be particularly relevant for K-Pop, where highly stylized fonts or text integrated into complex graphics are common in fan-created content or promotional materials. However, the accuracy of OCR on such varied and often non-standard visual inputs is a known challenge; busy backgrounds, unusual typefaces, or low-resolution images can significantly degrade performance, potentially leading to nonsensical or partially captured text that is then impossible to translate meaningfully. It appears the goal is to make more aspects of the playlist experience globally understandable, moving beyond just the music itself to the surrounding descriptive and visual context, though reliably extracting and translating text from diverse image sources at scale remains a non-trivial task, susceptible to errors at multiple points in the pipeline.
AI Translation Tools for Cross-Cultural Spotify Playlist Descriptions A Technical Guide - Automated Subtitle Generation For Multi Language Music Video Descriptions At 002 USD Per Word
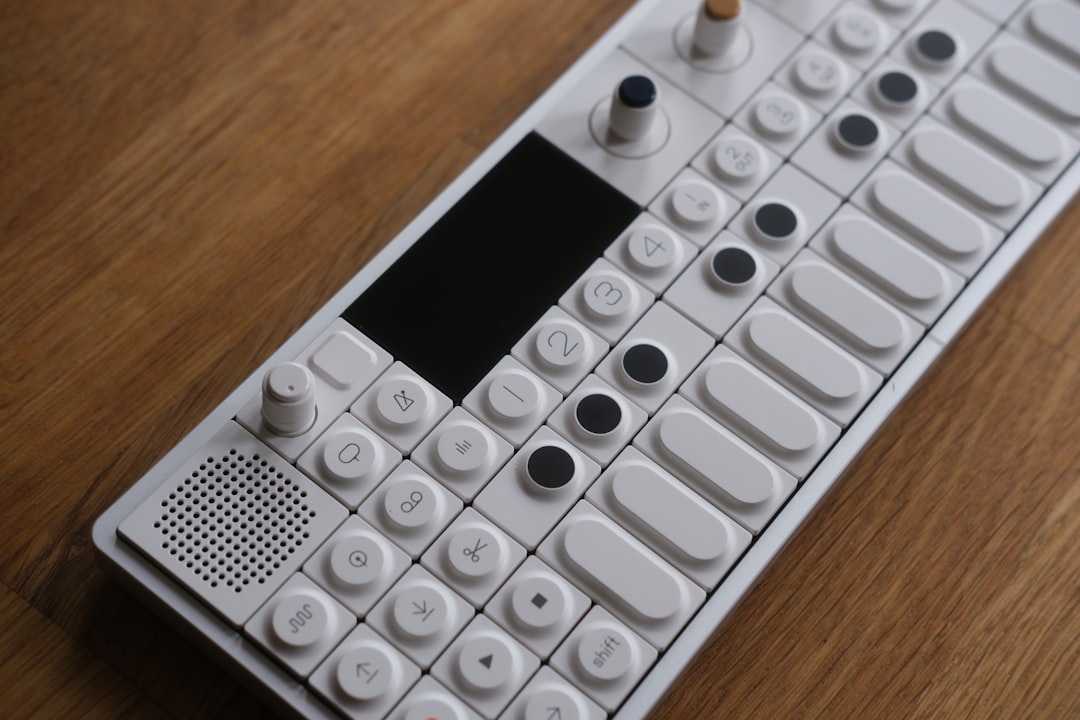
The development of systems for automatically creating subtitles for multilingual music video content at a price point reported around 0.02 USD per word marks a notable evolution in making media more widely understandable. These technologies employ sophisticated speech recognition and automated transcription capabilities to convert spoken or sung audio into text. The aim is to streamline the production of captions across different languages, reducing the significant effort traditionally needed for manual subtitling or translation of audiovisual material. This capability not only speeds up workflows dramatically but also opens up video content to viewers globally, potentially increasing viewership and engagement by overcoming language barriers directly within the video stream. However, generating subtitles, especially for creative or performance-based content like music videos, presents unique challenges; capturing lyrical subtleties, translating cultural references, or even accurately segmenting spoken dialogue in complex audio tracks isn't always seamless. While the efficiency gains are substantial, the output still warrants review to ensure fidelity to the original artistic intent and accurate representation of nuance, as automated systems aren't yet perfect interpreters of human expression, particularly in a creative medium. This automation undeniably contributes to a more connected media environment, lowering the practical hurdles to sharing music videos across diverse linguistic communities.
Exploring the technical avenues for enabling multi-language access to media like music videos and their surrounding descriptions reveals several interesting developments in automation. Generating subtitles and translating related textual elements automatically appears increasingly viable, achieving speeds where thousands of words can be processed in surprisingly short periods, a noticeable departure from the paced workflow of human translators.
The economic proposition is significant; we're seeing claims of costs dropping to figures like $0.002 per word for certain automated processes. While perhaps not universally applicable or maintaining perfect fidelity, this cost point drastically shifts the calculus for scaling localization efforts compared to typical human translation rates, which are orders of magnitude higher.
Optical Character Recognition (OCR) is integrating more deeply into these automated pipelines. This allows systems to pull text not just from standard fields but potentially from imagery associated with the music content, like overlaid text on a music video frame or text embedded in album art used in a description context. It's an effort to capture a more complete picture of the textual information surrounding a track or video.
There's an underlying belief that these AI systems possess a capacity for refinement. The idea is that by observing numerous translation tasks or potentially incorporating feedback signals, the models can iteratively improve their output, theoretically leading to better accuracy or more natural phrasing over time for recurring translation patterns.
However, a persistent challenge lies in capturing the subtle nuances of language, particularly the creative or idiomatic phrasing common in music-related descriptions and lyrics. Automated systems, while efficient at structural translation, can still produce outputs that feel stiff, culturally misaligned, or miss the intended tone, underscoring the potential need for human review layers.
Leveraging neural architectures supports handling content in bulk. Applying translation or subtitling processes across large sets of music videos or descriptions concurrently through batch processing streamlines workflow and reduces the delay in making translated content available across various linguistic markets.
The scope of what's being targeted for translation is expanding. Beyond just primary text descriptions or embedded subtitles, there's movement towards multimodal processing – potentially analyzing and translating related audio commentary or text appearing within video content, aiming for a more holistic localization approach.
Reliably extracting text using OCR, particularly from diverse and potentially low-quality visual sources common in user-generated or less formal content, remains a technical hurdle. Varied fonts, complex backgrounds, or image compression artifacts can easily degrade OCR performance, leading to errors that propagate downstream into the translation output.
Some systems incorporate automated checks to flag potential issues post-translation. These are useful as preliminary filters but often lack the discernment needed to identify more subtle errors related to cultural context or artistic expression, suggesting they serve better as complements to, rather than replacements for, human judgment when accuracy is critical.
The underlying technology is often designed with portability in mind. The core AI models and techniques developed for tackling translation or subtitling for one set of languages are frequently adaptable, allowing for expansion into new language pairs by retraining models on relevant datasets, offering a scalable foundation for global reach.
AI-Powered PDF Translation now with improved handling of scanned contents, handwriting, charts, diagrams, tables and drawings. Fast, Cheap, and Accurate! (Get started for free)
More Posts from aitranslations.io: