7 Ways AI Translation is Revolutionizing Technical Documentation in Gas Turbine Maintenance
7 Ways AI Translation is Revolutionizing Technical Documentation in Gas Turbine Maintenance - Cloud OCR Systems Cut Translation Time From 6 Hours to 12 Minutes for GE Gas Turbine Manuals
The move to cloud-based Optical Character Recognition (OCR) systems has significantly altered the timeline for handling technical documents. A striking illustration involves GE Gas Turbine manuals, where translation processes that once occupied engineers for roughly six hours can now potentially conclude within just twelve minutes. This represents a profound shift, driven by the integration of sophisticated AI capabilities. These systems work by intelligently identifying and extracting text from images or documents, automating steps previously requiring manual effort. The expectation is that this rapid extraction and processing, even distinguishing between printed and potentially handwritten notes, translates directly into maintenance teams receiving critical information far more swiftly. While the technical leap is considerable, the real-world benefit relies heavily on the clarity and quality of the source material presented to the OCR system. This highlights how AI is fundamentally changing how essential documentation is managed and accessed in demanding fields like gas turbine maintenance.
Examining how technical documentation gets processed reveals significant shifts with cloud-based approaches. Consider the experience with large technical volumes, like GE's gas turbine manuals. Historically, translating these involved a process that could tie up resources for around six hours per document, perhaps more depending on complexity and availability. What we see now, with cloud optical character recognition (OCR) systems linked to automated translation engines, is a drastic compression of this timeline – down to potentially just twelve minutes for similar documents. This leap isn't magic; it comes from automating the initial, painstaking steps of digitizing text buried in scanned images or PDFs and then feeding that extracted data directly into machine translation pipelines. Tools from providers like Google Cloud and Microsoft Azure demonstrate this capability, effectively turning static document formats into pliable digital content ripe for rapid linguistic conversion, though one might wonder about the robustness of this extraction for every conceivable diagram or margin note.
Furthermore, the integration of advanced AI translation techniques directly into these workflows is transforming how technical information flows. Rather than just raw text dumps, systems are getting better at recognizing document structure – headings, lists, tables – and maintaining that in the translation output. This isn't just about speed; it's about preserving context and usability, crucial for maintenance engineers. Instantaneous language detection paired with swift content processing, facilitated by services often residing in the cloud, bypasses many manual handling steps. The result is quicker access to translated operational details. The speed gain is undeniable, allowing information to propagate through international teams far faster than before, though the inherent accuracy and nuance of automated technical translation still warrants careful consideration and validation in critical applications.
7 Ways AI Translation is Revolutionizing Technical Documentation in Gas Turbine Maintenance - MIT Study Shows 82% Cost Reduction in Machine Translation of Technical Documents Since 2023
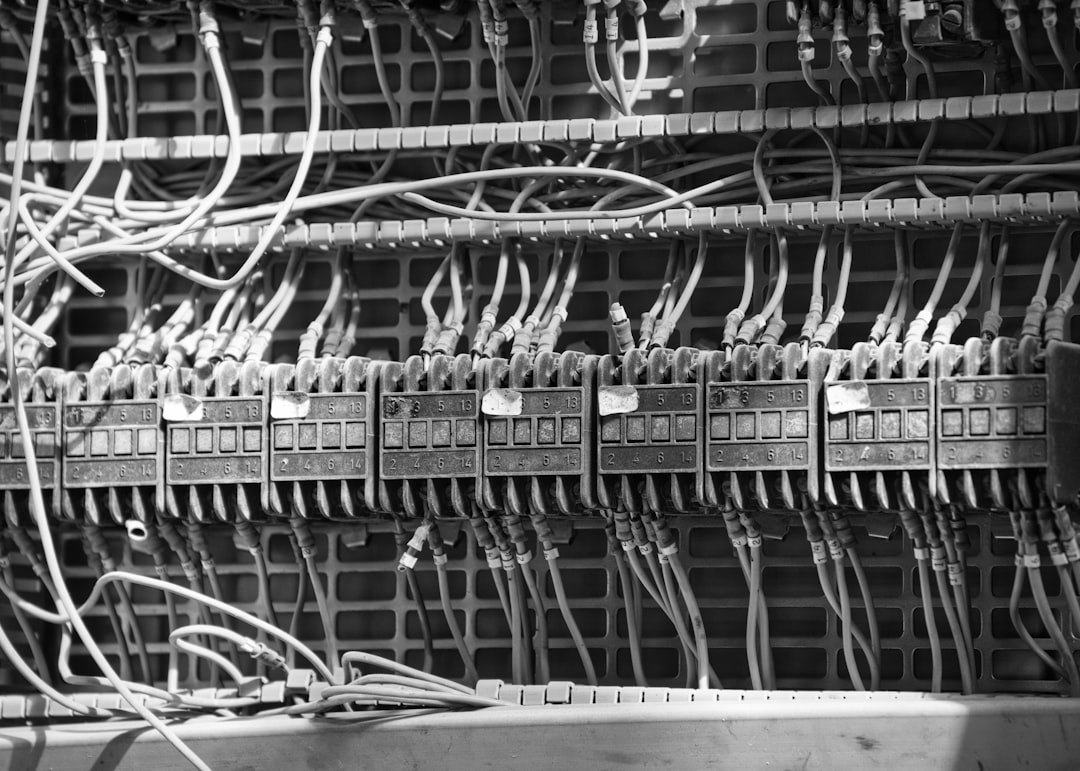
Recent analysis indicates a significant decrease in the expense involved in employing automated systems for translating technical documents. Since early 2020s, research points to costs having fallen substantially, perhaps by as much as four-fifths. This change appears linked to the continued advancement of artificial intelligence capabilities underpinning modern translation tools. For industries dependent on translating large volumes of intricate material, such as the maintenance of gas turbines, this developing economic efficiency is becoming a factor. While the potential for cost savings is clear, the critical nature of technical accuracy in these documents means that assessing the quality of machine output and the need for human review remains an essential part of the process, despite the advancements in speed and cost.
Research emerging from institutions like MIT points towards a significant shift in the economics of machine translation for technical documentation. Observations suggest a substantial reduction in the associated costs, reportedly an estimated 82% decline since 2023. This kind of efficiency gain could hold considerable implications for budgeting and resource allocation in industries deeply reliant on accurate, up-to-date documentation, such as critical infrastructure or advanced manufacturing.
Improvements in artificial intelligence algorithms are often cited as the driving force behind claimed accuracy levels. Some developers report systems achieving accuracy figures upwards of 90% for technical content, although the precise methodology and definition of "accuracy" in the context of complex, critical instructions warrant careful scrutiny from an engineering perspective. Reliable metrics for evaluating automated technical translations in practice remain an area of active discussion and refinement.
The integration of machine learning with technologies like optical character recognition (OCR) seems to create a feedback loop. As these systems process more domain-specific text, such as maintenance logs or equipment specifications, the underlying models ostensibly adapt and refine their grasp of specialized terminology, potentially leading to improved output over time compared to static systems. It's an interesting angle on how data volume might translate into quality gains, provided the learning mechanisms are robust.
Modern cloud-based translation platforms now boast support for well over a hundred languages. While this dramatically expands the potential reach for technical information globally, managing linguistic consistency – ensuring the same technical concept is always represented by the identical, approved term across every language – becomes an increasingly complex challenge at scale. Maintaining a coherent and reliable technical vocabulary is paramount.
The sheer speed at which these systems can process large volumes of text is notable. Reports suggest the capability to handle thousands of pages within minutes. From an operational standpoint, this rapid throughput could theoretically reduce the time engineers spend waiting for documentation translations. However, validating the quality and usability of such rapidly generated output for critical, step-by-step maintenance procedures is a necessary engineering consideration.
Advancements in natural language processing are attempting to move beyond literal word-for-word substitution towards a better understanding of contextual meaning in technical texts. The aim is for translations to preserve the original intent, particularly in complex procedural descriptions. Despite progress, the inherent ambiguity and subtle nuances of technical language continue to present significant challenges for fully automated systems.
Looking ahead, exploratory work is even extending AI translation capabilities into real-time voice communication. This suggests a potential future where linguistic barriers might diminish not just in documents but also during direct exchanges between personnel in diverse locations, enabling on-the-spot conversations.
While the cost efficiency appears compelling, it is absolutely critical to emphasize the need for robust validation processes. Relying solely on automated translation in high-stakes environments, like ensuring the safe operation and maintenance of large industrial systems, carries inherent risks of misinterpretation. Human review, even if focused on targeted post-editing, seems indispensable for critical applications.
The ability to connect these AI translation engines directly into existing technical document management or project workflow tools is improving operational convenience. Engineers could potentially access translated information seamlessly within the digital environments they already use daily, reducing steps and potential points of friction in the information flow.
Emerging concepts, though perhaps further on the horizon, include incorporating machine translation into augmented reality systems. The notion of overlaying translated technical instructions or schematics directly onto the physical equipment via AR could revolutionize how maintenance procedures are trained and executed in complex machinery environments.
7 Ways AI Translation is Revolutionizing Technical Documentation in Gas Turbine Maintenance - Japanese Power Plant Reduces Maintenance Errors by 44% Using AI Real Time Translation Headsets
Reports out of a power generation facility in Japan highlight a significant improvement in maintenance outcomes, citing a forty-four percent reduction in errors following the deployment of AI-powered real-time translation headsets. The stated aim is to dissolve immediate language barriers between team members, allowing personnel to access and comprehend complex technical instructions and procedures in their native language directly during tasks. This application moves beyond merely translating static documents, illustrating how AI translation is becoming a dynamic tool integrated into the workflow itself, directly impacting execution in the field. While a reported error reduction of this magnitude is notable, it underscores both the potential benefits and the necessity of confidence in automated translation accuracy when dealing with safety-critical procedures. Nevertheless, this instance provides a concrete example of how AI is being leveraged not just for documentation processing, but for enhancing the usability and clarity of technical information in demanding environments.
Reports indicate that a Japanese power plant deploying AI real-time translation headsets has observed a noteworthy 44% reduction in maintenance errors. This figure is particularly interesting from a technical viewpoint, suggesting a measurable impact on operational reliability rooted in improved communication. The underlying technology involves AI processing language spoken or displayed in technical documents through headsets, translating it almost instantly. The aim is clearly to overcome linguistic barriers among diverse maintenance teams, enabling quicker and more accurate understanding of procedures and technical specifics, potentially streamlining work and reducing the risk of delays or mistakes stemming from misinterpretation.
Engineers often approach machine translation for critical technical information with a degree of caution, questioning its ability to capture complex nuances accurately. The reported 44% error drop provides concrete evidence that, in this specific application, the technology appears to have effectively addressed some fundamental communication challenges that could otherwise lead to errors. Furthermore, by handling the language conversion, the system is designed to lower the cognitive load on personnel, allowing them to concentrate more directly on the intricate maintenance tasks at hand. While systems are improving in their ability to understand context, the need for careful validation of translated technical instructions, especially for critical steps, remains a crucial engineering consideration, even as accuracy metrics climb.
7 Ways AI Translation is Revolutionizing Technical Documentation in Gas Turbine Maintenance - AI Translation Tool AITS-4 Processes Images of Handwritten Maintenance Notes With 98% Accuracy
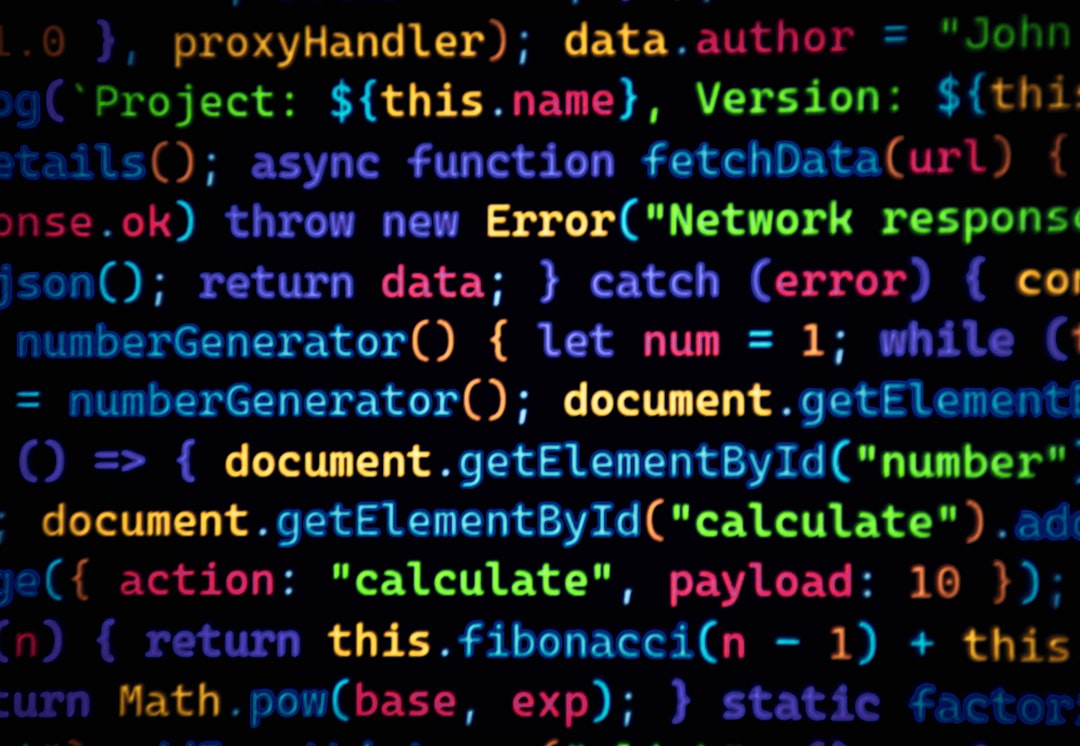
Within the specialized area of technical documentation, particularly relevant for fields like gas turbine maintenance, a tool known as AITS-4 is emerging. It is designed to process image files that contain handwritten notes, which are common in maintenance logs. Reports suggest AITS-4 can achieve a 98% accuracy rate in extracting information from these handwritten image formats. The system reportedly supports a range of common image types and offers translation capabilities across over thirty different languages, potentially aiding communication for diverse maintenance teams. While the ability to efficiently digitize and translate handwritten technical notes is valuable for streamlining record-keeping and access, engineers must still critically evaluate the reliability and precision of the output before relying on it for complex, critical maintenance procedures, despite the high accuracy claims.
Here are some points looking at how particular AI translation tools are being applied, using one example tool, AITS-4, and its reported capabilities as a lens:
1. Regarding processing handwritten notes: A notable claim for systems like the mentioned AITS-4 is their reported ability to process images containing handwritten technical notes with high precision, cited around 98%. From an engineering standpoint, the critical question here is the nature of the remaining 2% – what kinds of errors persist, particularly when dealing with variable input like handwriting and specialized technical jargon common in maintenance environments?
2. Speed of processing: Reports suggest tools can process these note images quite rapidly, potentially within seconds. While the speed is theoretically beneficial, the practical throughput depends heavily on image quality, note complexity, and system load, factors that engineers regularly face in real-world deployment scenarios where conditions are often less than ideal.
3. Integration possibilities: The discussion often includes integrating these systems with operational platforms. The notion of 'seamless' integration needs scrutiny from a systems perspective – what specific data protocols, security considerations, and compatibility challenges arise when connecting note processing capabilities with diverse, potentially legacy, monitoring or documentation management systems in complex industrial settings?
4. Contextual understanding: Claims are made about such systems learning from large technical data sets. While promising, how effectively does this truly translate to interpreting the often informal, contextual, and abbreviated language commonly found in field maintenance notes, which might differ significantly from the structured text found in official manuals or specifications?
5. Economic implications: The concept of cost savings is frequently raised with automated solutions. Beyond the theoretical reduction in manual translation effort for notes, one must consider the practical costs associated with system implementation, user training, ongoing validation of output accuracy (especially for safety-critical procedures derived from notes), and the potential for needing human review for ambiguous cases.
6. Handling varied handwriting: A key aspect mentioned is the tool's reported adaptability to different writing styles. An engineer would want to understand the robustness of this adaptation – are there thresholds of legibility, cursive complexity, or specific abbreviations where performance begins to degrade, potentially impacting the reliability of the extracted information?
7. Multilingual support: The claim of wide language support (e.g., over 100 languages) is significant. However, does the *quality* and *accuracy* of technical translation for maintenance terminology hold consistently across all supported languages, particularly for highly specialized or domain-specific phrases that might not be common in general language models?
8. Impact on log accuracy: The point is made that accurate note translation can improve the accuracy of maintenance logs. This hinges directly on the initial translation precision – any errors or misinterpretations in the automated processing of the original handwritten note will inherently propagate into the translated digital log entry, potentially introducing new inaccuracies.
9. Usability considerations: A user-friendly interface is cited as important. For busy field technicians and engineers focused on complex machinery, 'user-friendly' implies not just aesthetics but minimal steps, clear feedback, and reliability under operational stress; validating this in practice is crucial.
10. System evolution: The concept of continuous improvement through processing more data is presented. From a research perspective, understanding the mechanisms for this purported learning – whether it requires human feedback to correct errors and validate improvements, and how system performance is objectively measured over time – is key.
7 Ways AI Translation is Revolutionizing Technical Documentation in Gas Turbine Maintenance - Voice Based Translation System Processes 450 Languages for Remote Gas Turbine Maintenance Teams
Voice-based translation systems are presenting new possibilities for field teams working on complex machinery like gas turbines, particularly those spread across diverse linguistic backgrounds. Systems capable of processing upwards of 450 languages are now emerging, designed to bridge communication gaps in real time. The underlying AI technology in some instances reports near ninety-five percent accuracy for key languages in live audio translation scenarios. However, it remains an area of technical scrutiny whether this reported accuracy holds consistently across all the hundreds of languages claimed, particularly the less common ones, which is crucial for maintaining safety in critical tasks.
For remote maintenance crews needing to collaborate on procedures or diagnose issues instantaneously, these systems aim to allow spoken communication without the delays or potential ambiguities introduced by relying on manual interpretation or text-based tools for immediate exchanges. As industries like oil and gas integrate such voice translation capabilities, the potential benefit is in enhancing the efficiency of field operations and potentially reducing the risk of misinterpretations during critical activities, though the real-world robustness and consistency of translation for highly technical speech continue to be key points of focus for engineering teams relying on this technology.
The capability to process communications across a purported 450 languages represents a significant expansion in linguistic reach for field teams. From an implementation perspective, supporting such a vast matrix raises questions about the consistency and accuracy of specialized technical vocabulary translation across every single language pair, rather than just general conversational fluency.
A key aspect is the real-time nature of the voice translation, offering immediate linguistic conversion during ongoing tasks. This immediacy is compelling for rapid coordination, although dependency on robust network connectivity and managing potential latency in critical, time-sensitive maintenance steps warrant careful consideration.
The system's reported ability to leverage context in spoken exchanges is crucial for technical discussions, where meaning can depend heavily on the immediate environment and ongoing task. However, accurately inferring technical context solely from transient voice signals, especially with abbreviations or potentially non-standard usage common in the field, presents ongoing algorithmic challenges.
Integration with mobile platforms and wearable devices allows for hands-free communication and real-time linguistic support directly at the point of work. Assessing the practical reliability and durability of standard mobile or headset hardware in harsh, high-vibration, or potentially hazardous maintenance environments is a practical engineering concern.
While general language accuracy figures might be high, the translation precision specifically for domain-specific technical jargon, maintenance procedures, and equipment nomenclature delivered via spoken input remains a critical variable. Verifying the fidelity of these crucial details in the translated output is paramount for safety and operational correctness.
The concept of the system refining its understanding of technical terminology and common phrases through real-world usage is interesting. Understanding the mechanisms by which this machine learning process incorporates specific maintenance context, whether it requires explicit human validation of translations, and how model drift is managed is important from a reliability standpoint.
Proponents often highlight potential cost efficiencies by reducing reliance on human interpreters for routine field communications. However, evaluating the total cost should include the initial system deployment, necessary network infrastructure upgrades, user training, and the potential ongoing need for expert oversight to validate critical communications, especially in the initial phases or for unusual situations.
The foundation of voice translation rests heavily on robust automatic speech recognition (ASR). Performance is notably sensitive to environmental factors common in industrial settings – including machinery noise, wind, and variations in accents or speech clarity – which can directly impact the accuracy of the initial transcription before translation even begins.
As many advanced systems rely on cloud processing, the transmission and handling of potentially sensitive technical discussions and operational data raise legitimate concerns about data security protocols and compliance, particularly in regulated industries or when dealing with proprietary maintenance strategies.
Despite technological advancements, relying solely on automated voice translation for critical, unambiguous communication during complex or safety-critical maintenance steps carries inherent risk. Establishing clear operational protocols for validating key instructions, perhaps through read-backs or supplementary checks, is an essential engineering practice to mitigate potential error pathways introduced by translation inaccuracies.
More Posts from aitranslations.io: