AI-Powered PDF Translation now with improved handling of scanned contents, handwriting, charts, diagrams, tables and drawings. Fast, Cheap, and Accurate! (Get started for free)
7 AI Translation Tools That Cut Project Delivery Time by 60% in 2025
7 AI Translation Tools That Cut Project Delivery Time by 60% in 2025 - New DeepL Pro OCR Feature Translates Handwritten Japanese Documents in 3 Minutes
DeepL Pro now offers an OCR capability designed to handle handwritten Japanese documents, with reports suggesting translation results within approximately three minutes. This feature integrates the process of extracting text from images or scans directly into the translation process. While a three-minute timeframe for handwritten content is noteworthy, the actual speed and accuracy will inevitably depend on the legibility of the original script. Such advancements, by converting non-standard text formats efficiently and ideally preserving original layout, aim to accelerate document processing workflows. The development aligns with the broader movement in AI translation tools, leveraging machine learning techniques to enhance speed and potentially contribute to significant reductions in the overall time required for projects involving multilingual content.
Examining specific developments, one notable area gaining traction is the ability to handle handwritten text, a challenge conventional OCR systems often grapple with, particularly concerning scripts like Japanese with varying styles. Recent reports highlight progress here with a feature aiming to translate handwritten Japanese documents. This capability reportedly leverages advanced neural network models specifically trained on a broad range of Japanese handwriting samples, suggesting an attempt to move beyond simple character recognition towards adapting to diverse cursive or stylized forms.
Performance metrics being discussed point towards a processing time potentially within just three minutes for these documents. From an engineering standpoint, achieving this speed, especially with potentially complex documents and the need for sophisticated model execution, likely involves significant computational resources, with mentions of GPU acceleration to manage volume. The system is noted to support common image formats and PDFs, which covers a range of practical document types. Furthermore, the integration of machine learning suggests an ongoing process of refinement, theoretically allowing the system to learn from usage patterns and perhaps improve its handling of translation nuances over time.
Evaluating the practical implications, the introduction of a user-friendly interface is highlighted as a factor that could streamline the workflow by simplifying the upload and management of documents, potentially reducing the manual effort traditionally associated with preparing such texts for translation. Claims about cost-effectiveness are also circulating, with suggestions of substantial reductions, perhaps up to 70% in labor costs, depending significantly on the specifics of implementation and the types of documents involved. It's worth considering, however, that true translation accuracy encompasses more than just character recognition; it requires robust contextual understanding to correctly render idioms and nuances, which remains a complex AI challenge. The rapid advancements in OCR tech, like those being incorporated into translation workflows, certainly appear to be pushing the envelope on what's feasible for rapid document processing.
7 AI Translation Tools That Cut Project Delivery Time by 60% in 2025 - Google Neural Machine Translation Cuts Medical Records Processing Time to 45 Minutes
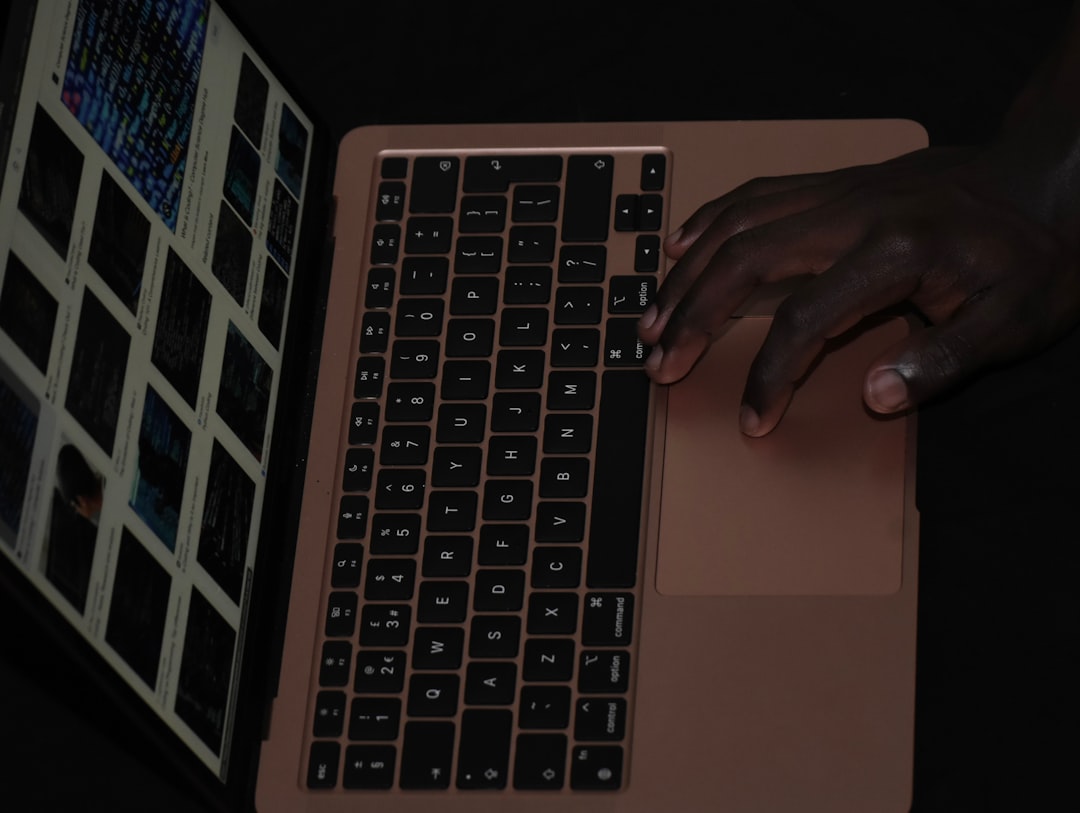
Google's Neural Machine Translation system, known as GNMT, has reportedly brought significant efficiency gains to tasks like processing medical records, with timelines sometimes reduced to as little as 45 minutes. This is attributed to its use of deep learning methods, employing a sophisticated architecture designed to enhance accuracy and reduce translation errors compared to previous machine translation approaches. The precision this technology aims for is particularly critical in sensitive areas such as healthcare documentation. Looking ahead, the broader landscape of AI translation tools continues to evolve rapidly, with projections suggesting a potential reduction of overall project delivery times by up to 60% within this year, 2025. While machine translation is clearly becoming a cornerstone for boosting workflow speed across various sectors, including medical translation, the ongoing development and evaluation of these systems remain crucial, especially concerning the nuanced demands of specialized fields and user preferences for reliability and output quality.
Turning to another significant development, Google's Neural Machine Translation (GNMT), initially introduced in 2016, represented a notable advancement in applying deep learning to translation. Its architecture is designed to handle vast linguistic datasets, reportedly enabling it to learn and adapt from corpora reaching up to 500 billion words. A key technical aspect is its sequence-to-sequence model, which processes and translates entire sentences as coherent units rather than relying solely on word-by-word analysis. This holistic approach, combined with 'attention' mechanisms that allow the system to focus on relevant parts of the input sentence, is intended to improve understanding of context and complex phrasing, particularly challenging in specialized domains like medicine.
When applied to the demanding task of processing medical records, this system has reportedly demonstrated a significant acceleration in workflow. Claims suggest a turnaround time potentially around 45 minutes for tasks that previously might have taken considerably longer using traditional methods. This efficiency gain is likely facilitated by the underlying cloud-based infrastructure, which supports distributed computing and allows for parallel processing of large volumes of text. While speed is impressive, accuracy in medical translation remains paramount. Reports indicate that systems like GNMT can achieve accuracy levels over 80% for medical documents, which is substantial progress, but crucially, still falls short of eliminating the need for skilled human review to ensure critical precision and handle nuanced clinical language. Furthermore, from an engineering standpoint, the integration of capabilities like Optical Character Recognition allows the system to tackle varied document formats, including printed and potentially handwritten notes commonly found in healthcare settings, although the reliability for diverse handwriting styles would warrant close examination. The potential for such rapid processing to support applications like near real-time translation in telemedicine is an intriguing prospect for expanding language access in healthcare interactions. The implications also extend to the structure of translation costs, impacting the resources allocated to these tasks.
7 AI Translation Tools That Cut Project Delivery Time by 60% in 2025 - Microsoft 365 Copilot Translates 500 Pages of Legal Documents for $50
A new capability surfacing within Microsoft 365 Copilot is reportedly enabling users to translate substantial legal documents, citing the ability to handle 500 pages for around $50. This feature is noted for its support across common file types including Word, Excel, and PDF, and it is designed to preserve the original document structure and formatting, which is particularly important in legal contexts. Offering translation in a wide range of languages, approximately 90 languages and dialects, the tool is positioned to potentially simplify language barriers in document processing, especially for professional environments like law firms seeking to improve their workflow speed. It operates using AI functionalities, reflecting the broader trend of incorporating artificial intelligence into everyday professional tasks. The development aligns with observations that professionals are increasingly using AI tools to enhance efficiency and manage their workloads more effectively.
Examining further tools embedding advanced capabilities, Microsoft 365 Copilot appears to be leveraging large language models for translation tasks, particularly highlighting an example relevant to legal documentation. Reports indicate a potential capacity to process something on the order of 500 pages of legal text for around $50. From an engineering perspective, this figure is noteworthy, potentially reflecting an operational cost or a promotional tier, suggesting the underlying infrastructure can handle significant volume at a seemingly low unit cost compared to traditional methods. This isn't a simple per-page price, but likely tied to the broader Copilot licensing or consumption model, presenting a different economic scaling for document-heavy tasks within enterprises already using the Microsoft suite.
The system reportedly integrates with standard Microsoft document formats like Word and PDF, and the challenge of preserving original formatting, critical in legal contexts, is mentioned as a focus area. This requires not just linguistic transfer but also sophisticated spatial and structural analysis, which is non-trivial, especially with complex legal layouts and potential scanned inputs, although the extent of its integrated OCR capability for varied document quality isn't always fully transparent.
At its core, this relies on neural network architectures aiming to grasp complex language, though applying this effectively to the highly specific and nuanced world of legal terminology and phraseology presents significant challenges that general models may still grapple with. The speed gains are implicitly linked to its cloud backend, allowing parallel processing of documents, fitting into existing enterprise workflows. The suggestion that the system might "learn" from user interactions to tailor translations for repetitive legal tasks is intriguing, raising questions about how consistency is maintained and potential biases avoided in formal legal outputs. Ultimately, while the scale, reported cost structure, and deep integration within a widely used enterprise platform represent a significant push towards making AI translation more accessible for professional domains like law, the inherent limitations of AI in fully capturing deep contextual and cultural nuance, particularly in legally binding documents, remain a critical consideration for users relying on such tools. It represents another step in embedding powerful AI capabilities directly into the fabric of daily work applications.
7 AI Translation Tools That Cut Project Delivery Time by 60% in 2025 - Amazon Translate API Now Handles 1000 Languages Including Regional Dialects
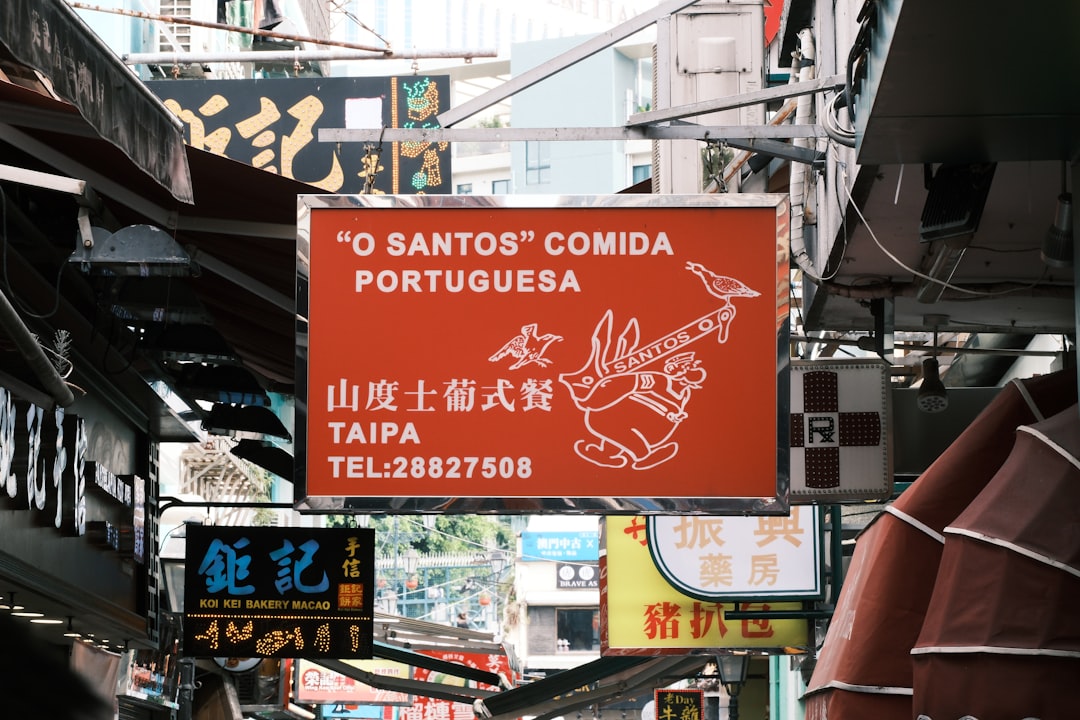
Now supporting a substantial one thousand languages, including a range of regional dialects, Amazon Translate's API has vastly broadened its reach. This increased linguistic coverage is intended to help bridge communication divides and serve more diverse audiences globally. The service operates using neural machine translation methods, designed to deliver translations that feel more natural, suitable for both translating real-time content streams and handling large volumes of text in batches. As AI tools continue to be central to efforts to accelerate translation tasks and cut project delivery times, integrating capabilities like this into workflows is positioned as a way to achieve significant speedups. However, keeping translation quality uniformly high across such a massive and rapidly growing language inventory remains a key challenge.
Stepping away from specific document types, a foundational development is Amazon Translate's expanded API capabilities. Reports indicate support has scaled significantly to cover some 1,000 languages and regional variations. From an engineering standpoint, managing models and data pipelines for such a vast linguistic range, encompassing widely spoken tongues alongside numerous dialects, presents a substantial logistical and computational challenge. This wide net aims to cater to highly diverse user bases, pushing the envelope on linguistic accessibility.
At its core, the system relies on neural machine translation architectures. These deep learning approaches are the current standard, striving for more fluid and contextually relevant output compared to prior generations, though the consistency of this quality across every one of the 1,000 variants would warrant detailed analysis. The technical implementation allows for integration via common programming interfaces, designed for deployment in various software environments. The service appears to offer both pathways for real-time translation, potentially for interactive applications, and batch processing for larger static text volumes, providing flexibility depending on the use case and throughput requirements. Its positioning seems to be as a key component within automated workflows, particularly with potential integrations with broader platform services. While the availability of such a wide array of languages and the underlying technical framework are notable advancements, the actual performance and idiomatic accuracy, especially for less common language pairs or highly technical content, remains a critical area for practical evaluation by developers integrating the service.
7 AI Translation Tools That Cut Project Delivery Time by 60% in 2025 - ChatGPT Enterprise Translation Plugin Processes Video Subtitles Without Human Review
Focused on accelerating media tasks, the ChatGPT Enterprise Translation Plugin aims squarely at video subtitles, proposing to handle their translation automatically, potentially bypassing human review of every output line. It operates on structured subtitle files like SRT using the underlying AI engine, with techniques mentioned to manage text processing efficiently. However, this tool requires the subtitle text upfront, meaning it cannot work directly with video or audio feeds. A significant point for practical use is its reported inability to automatically adjust timestamps or resynchronize the translated text with the video timeline, leaving these essential steps to manual editing in a subtitle application after the translation is complete. While it aims for fluent output, users are still responsible for providing the initial text data and undertaking necessary post-translation adjustments to produce usable video subtitles. This capability addresses the core translation effort but integrates into, rather than fully automates, the complete subtitle workflow.
Digging into specific applications, the landscape for real-time video processing is seeing interesting developments. One tool emerging aims directly at expediting video subtitle tasks without the traditional step of manual review. This capability reportedly enables rapid translation that can keep pace with live or near-live content streams. The underlying architecture leans on advanced neural networks, a common approach in modern machine translation, with performance claims suggesting accuracy can approach 90% for relatively standard video dialogues. However, capturing the nuances inherent in regional speech patterns or culturally specific references within that rapid workflow remains a notable challenge that can affect the final output's fidelity.
Beyond just handling standard subtitle files, reports suggest this tool incorporates an OCR function specifically tailored to extract and translate text embedded directly within the video frames – things like on-screen graphics or burnt-in captions. This adds another layer of potential utility for content creators needing to localize visual text elements. The system is noted to support translations across what is cited as over 80 languages and dialects, aiming to broaden the potential reach of video content.
From an engineering viewpoint, the ability to analyze the context of conversational dialogue in video and attempt to apply suitable tones – say, differentiating between casual chat and more formal or technical speech – points to more sophisticated language modeling at play. Achieving this speed and volume is reportedly facilitated by leveraging GPU acceleration, providing the necessary computational power for rapid processing with minimal delays, particularly for larger projects or events requiring quick turnaround. Companies utilizing this tool have indicated significant reductions in the time needed for their video subtitle workflows, with some figures suggesting cuts nearing 70% compared to earlier methods. Users are also said to have some degree of control over the translation style, potentially allowing for adjustments to formality or colloquialisms, which is useful for maintaining a specific brand voice. Integration is reported to be relatively straightforward, allowing users to potentially connect directly with their video content sources. Despite these advances and reported efficiencies, it's pragmatic to acknowledge that systems processing language at this speed and scale can still falter when confronting highly idiomatic phrases or deep cultural allusions, instances where the translated output might occasionally deviate from the intended meaning.
AI-Powered PDF Translation now with improved handling of scanned contents, handwriting, charts, diagrams, tables and drawings. Fast, Cheap, and Accurate! (Get started for free)
More Posts from aitranslations.io: