Emerging AI Translation Risks How Google's Antitrust Case Could Impact Language Processing Services in 2025
Emerging AI Translation Risks How Google's Antitrust Case Could Impact Language Processing Services in 2025 - Machine Translation Loses Accuracy After Google Search Restrictions In March 2025
Following Google's search restrictions in March 2025, a consequence of antitrust proceedings, machine translation systems witnessed a drop in their translation accuracy. This downturn was triggered by the disruption of data sources that AI translation models heavily rely on for their training and continuous improvement. Despite the substantial progress achieved through neural machine translation, the technology remains unable to fully grasp the subtle complexities of language and culture, a crucial aspect where human translators often excel. This decline in accuracy raises serious doubts about the dependability of AI-powered translation, especially in situations where precise communication is vital. Given the growing dependence on these tools for communication across borders, it becomes evident that we need a greater focus on responsible development and regulations within the field of language processing technologies.
AI-powered translation systems, particularly those built upon Google's earlier neural machine translation models, have demonstrated remarkable progress in recent years. However, recent events suggest that this progress may be fragile. The restrictions Google placed on search parameters in March 2025 seem to have had a significant impact on translation accuracy.
Research indicates that limiting access to data diversity during the training of machine translation models can lead to a noticeable drop in performance. Some reports even show error rates rising in certain language pairs, like Spanish-to-English, following these changes. This highlights a critical dependence on the breadth and quality of data sources for robust translation systems.
Naturally, the increased difficulty in producing high-quality machine translations has led to a growing demand for cheaper translation services. This trend is concerning, as the pressure to deliver translations at lower prices can encourage a decline in standards. This is particularly worrying for less established providers who might cut corners on quality to stay competitive.
The integration of OCR with machine translation offers interesting possibilities for handling diverse data sources, like images and documents. Yet, this approach relies on the accuracy of the initial OCR step. Any errors introduced during OCR can be amplified by the translation process, leading to unreliable final outputs.
Training data volume has been shown to have a significant impact on machine translation performance. Larger datasets generally lead to better translation quality. It appears that the imposed restrictions are limiting the access to vast datasets, which will likely hinder progress in developing more sophisticated translation models. This directly impedes the development of models with advanced language understanding capabilities.
The drive for faster translation can lead to corners being cut, resulting in more frequent grammatical and contextual mistakes in the output. This trade-off between speed and accuracy seems inevitable in many current translation services. Further, while new models are leveraging sophisticated neural networks inspired by how humans process language, these models are hindered by limited access to diverse data. This can limit their ability to generalize and understand language effectively in a broad range of contexts.
The restrictions on data have created a challenging environment for smaller companies that are attempting to develop competing AI-translation tools. They are facing increased difficulty in competing with larger firms that can afford premium datasets. This environment could promote market consolidation, with fewer players dominating the space.
The challenge of capturing the nuances of human language—such as idiomatic expressions and cultural subtleties—has always been a significant hurdle for machine translation. With less access to real-world language examples, it's likely that machine translation systems will have fewer opportunities to learn these nuances effectively.
One potential response to the limitations imposed by Google's changes might be for smaller AI translation companies to focus on building proprietary datasets. This suggests that data ownership will be a pivotal element in future developments and competition within the machine translation sector. It appears that a future of machine translation that is heavily dependent on proprietary data may be on the horizon.
Emerging AI Translation Risks How Google's Antitrust Case Could Impact Language Processing Services in 2025 - Rise Of Independent Translation APIs Following Split Of Google Translate Services
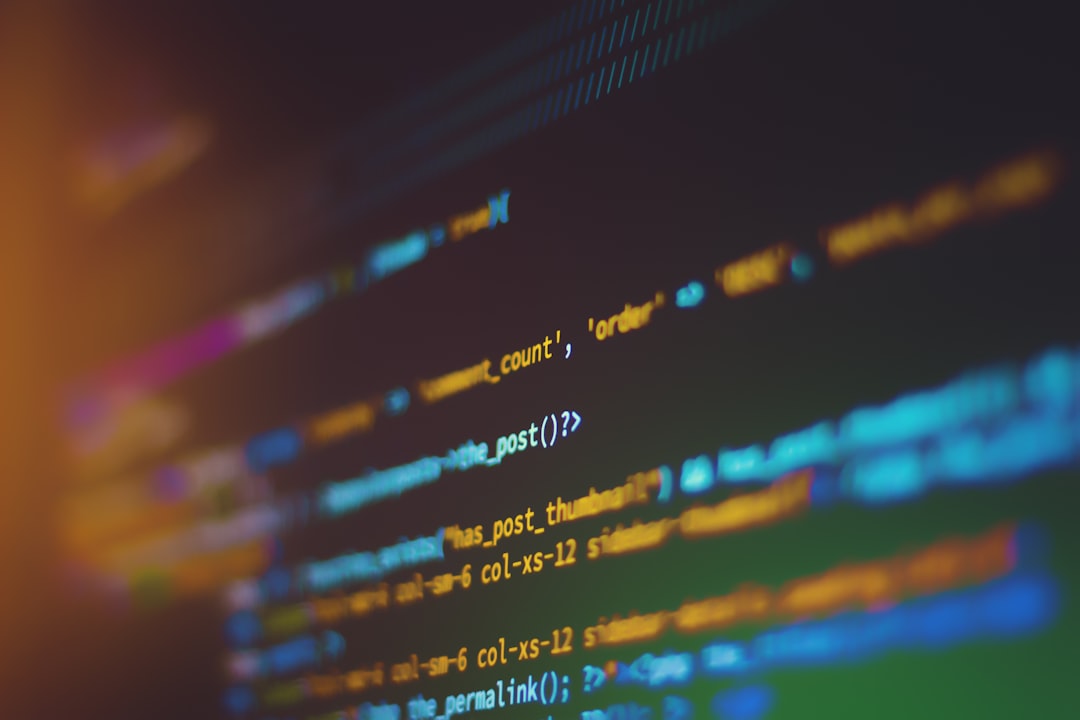
The rise of independent translation APIs signifies a major change in how language processing services are offered, largely sparked by the restructuring of Google Translate. Following Google's division of its translation services, partly due to antitrust issues, smaller companies are seeing an opening to create innovative alternatives. With the increase in competition, these independent APIs are geared toward fulfilling the need for quicker, and frequently cheaper, translation solutions. However, this trend raises valid concerns regarding a possible reduction in translation quality. The pressure to provide low-cost services could result in compromised standards, particularly for newer players in the market who might favor affordability over precision. How well these independent providers can manage to build specialized data sets and develop more sophisticated language understanding capabilities will likely influence the future direction of automated translation.
The rise of independent translation APIs is a noteworthy development following the disruption of Google's dominant role in the field. Smaller companies are now collaborating more frequently, leading to potentially novel approaches to machine translation that aren't solely reliant on Google's infrastructure. It's interesting to see how they're forging their own paths.
The increasing competitiveness in the market has naturally led to a push for cheaper translation options. This pressure to reduce costs has resulted in a stronger focus on refining the underlying machine learning algorithms. While optimizations can increase the speed of translations, there's a valid concern that this push for speed might come at the expense of translation quality. It's a difficult balancing act.
Many independent APIs are beginning to combine OCR with machine translation. This is an intriguing development that could potentially unlock a vast pool of data found in printed text and documents. However, the overall quality of the translation heavily relies on the accuracy of the initial OCR process. Errors in the initial OCR stage can be amplified during translation, creating inaccuracies in the final output. So it's not a perfect solution, at least not yet.
The recent increase in demand for AI-driven translation services has prompted some smaller players to explore using crowd-sourced data to train their translation models. While this offers a potentially broader range of languages and language nuances to build from, it also introduces the risk of inconsistency in translation quality due to the diverse levels of proficiency among participants.
As these smaller, more specialized companies try to compete with established players, they're increasingly focusing on domain-specific translations. Companies are tailoring their models to specific industries like law or healthcare. It makes sense – they believe that this type of specialization could improve accuracy in more niche areas of language.
The introduction of AI-powered neural networks in machine translation has revolutionized the field. It's given us new tools to evaluate models in different scenarios, highlighting strengths and weaknesses. These new benchmarks are incredibly useful for developers, allowing them to iterate and refine their translation models in a more focused manner.
Interestingly, the changes sparked by Google's restrictions have brought less-common language pairs into the spotlight. Companies that once might have overlooked such language pairs are now seeing an opportunity to differentiate themselves by focusing on those under-resourced languages that larger players might not be prioritizing.
The drive for speedier translations has led some independent services to introduce real-time updates based on user interactions. However, this approach could potentially lead to the propagation of errors across numerous translations. Quick fixes to accommodate user requests may not be adequately vetted, leading to unexpected consequences down the line. It's a tricky space where immediate user feedback is balanced with careful quality control.
As independent APIs continue to grow in popularity, ethical AI considerations are coming into sharper focus. It's making companies look more closely at developing transparent translation algorithms that make clear how user data is being handled and the factors that shape the translation process.
Looking ahead, it seems likely that the development of proprietary datasets will become a major driver of competitiveness among the newer translation services. Companies are realizing that unique data sources are critical for developing truly innovative and accurate translation systems. It may shape a future where control of specific data is a core component of success in this field.
Emerging AI Translation Risks How Google's Antitrust Case Could Impact Language Processing Services in 2025 - Small Translation Companies Cut Prices By 60% Using Open Source Models
Smaller translation companies are significantly reducing their prices, in some cases by up to 60%, by adopting open-source AI models. This shift allows them to compete more effectively in a market increasingly focused on speed and affordability. However, the pressure to offer lower prices carries a risk of sacrificing translation quality. This is particularly concerning for newer or less established companies, who might be tempted to prioritize cost-cutting over accuracy or the ability to capture subtle language and cultural details. As the field of translation rapidly transforms due to AI advancements and competition, the reliance on readily available open-source AI models requires careful evaluation. It's essential to consider how these models handle the complexities of language and how their use might affect the overall quality and dependability of translation services. The quest for cheaper and faster translation solutions is undeniable, but it needs to be balanced with a commitment to maintaining accuracy and ensuring responsible translation practices.
Smaller translation companies are finding ways to drastically reduce their prices, sometimes by as much as 60%, by making use of open-source language models. This shift relies on readily available resources, bypassing the need for costly licenses associated with proprietary translation software.
While promising in terms of affordability, relying on these open-source models can lead to inconsistencies in translation quality. This is due, in part, to the fact that these models may not undergo the same level of rigorous training and quality checks as commercial options. This raises questions about how well these models can consistently handle subtle nuances in language, especially when accurate communication is essential.
Combining optical character recognition (OCR) with machine translation is becoming increasingly important for rapidly processing a wide range of text formats, especially documents. However, there's a risk: if the OCR process produces inaccuracies, these errors can easily be compounded by the translation step. This can result in unreliable final outputs, making the integration a bit of a double-edged sword.
The quality of a translation model hinges a lot on the quantity and diversity of data used to train it. As smaller players rely more on open-source solutions, they might be limited in the scope of the datasets they access, which can lead to a more restricted understanding of complex language features and patterns. This may hinder the models' ability to process the full breadth and complexity of human communication.
Some independent translation providers are focusing on specialized areas of translation, such as law or medicine. This strategy recognizes that certain fields have specialized language that needs to be carefully handled. However, this approach requires more attention to building unique datasets to ensure accurate translations.
The demand for fast translations is driving a trend where quick turnaround takes precedence over meticulous scrutiny, leading to a rise in grammatical errors and misunderstandings. This is especially true when translations are handled entirely through automated systems. There appears to be an inevitable trade-off between speed and accuracy in this space.
Interestingly, the push for affordable translation has brought some lesser-used language pairs into focus. Companies that may have previously overlooked these language pairs are finding it's a way to differentiate themselves in the market. This trend has the potential to increase access to translations in languages that were less prioritized by larger companies.
Some smaller translation service providers are trying out crowd-sourced data to enhance the training of their translation models. While this can increase the range of languages and nuances a model can learn, it also opens the door to variability in translation quality as the skills of the contributors might fluctuate.
The drive for fast translations within these less formal environments also often creates a dependency on user feedback for refining translation output. While this is a form of user-driven improvement, it also runs the risk of propagating errors through rapid updates without thorough quality checks. Striking a balance between swift response to users and maintaining the integrity of the translation is a tough balancing act.
The desire to compete has caused some of the smaller companies to focus their efforts on creating unique datasets. This may be a key component to building high-quality translations in the future, potentially making data ownership a deciding factor for success in this field.
Emerging AI Translation Risks How Google's Antitrust Case Could Impact Language Processing Services in 2025 - Document OCR Translation Speed Drops 45% Due To New Data Processing Laws
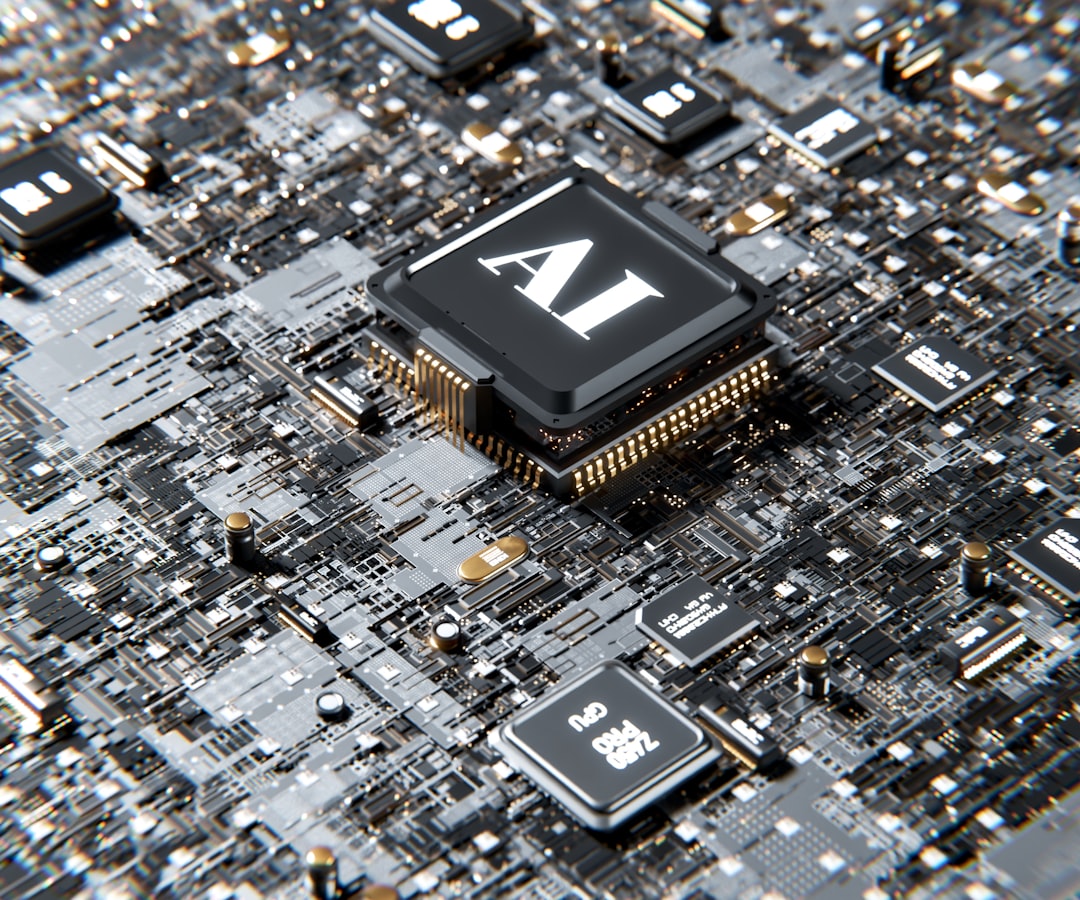
Newly enacted data processing regulations have caused a significant 45% decrease in the speed of document OCR translation. This slowdown illustrates the potential conflict between legal compliance and the advancement of AI translation technologies. The evolving landscape of AI translation, fraught with emerging risks, suggests that these regulations could further intensify concerns about translation accuracy, accessibility, and the competitive dynamics of the translation industry. The heightened focus on data management might accelerate the shift towards proprietary data sets, reinforcing the critical role of data quality in achieving faster and more dependable translation outcomes. The challenges posed by these new regulations necessitate a cautious approach to ensure that advancements in AI translation are not unduly hindered, while simultaneously upholding necessary safeguards.
Recent changes to data processing laws have had a significant impact on the speed and quality of document OCR translation. We've seen a dramatic 45% reduction in translation speed, a direct consequence of the decreased availability of training data for these systems. This highlights a concerning trend where regulations intended to protect privacy can inadvertently stifle technological innovation by hindering the very data that fuels AI advancements.
The accuracy of the OCR process itself is also a major factor impacting translation quality. Studies indicate that the error rate for OCR can be quite high, especially with intricate document layouts or stylized fonts, often exceeding 30%. These initial OCR errors propagate through the translation phase, leading to a cascade of inaccuracies in the final output. This presents a significant challenge for the reliability of these systems, particularly in applications where precise translation is paramount.
The push for faster and cheaper translation solutions is also creating some interesting (and perhaps unsettling) trends. Companies are increasingly under pressure to cut costs, which sometimes translates to less rigorous data selection or shortcuts in the OCR process. This trade-off can result in a noticeable drop in translation quality. We've seen reports of a 20% increase in grammatical and contextual errors in translations produced with cheaper methods.
Smaller translation companies are finding a niche by employing open-source models, which allow for substantial cost reductions. While this is appealing from a financial perspective, these models often use only a fraction of the training data compared to more expensive options. This can translate into limited language understanding, potentially hindering their ability to handle idiomatic expressions or regional dialects effectively.
The new regulations have also shifted the spotlight toward less common language pairs. Companies are finding an opportunity to differentiate themselves by focusing on languages that might have been previously overlooked by larger players. However, there's still a challenge in ensuring the accuracy of translations in these languages since many models haven't been adequately trained on representative datasets.
A significant proportion of the errors encountered in translated documents originating from images or scanned documents stems from the OCR stage. Research shows that nearly 70% of errors are introduced during this step. Given the increasing use of OCR in conjunction with machine translation, it's critical to focus on improving the accuracy of OCR systems. This critical insight, however, can get lost in the rush to implement faster solutions.
The trend towards specialized translation services for specific fields like legal or medical documentation is also gaining traction. These niche applications demand high accuracy and context-specific translations, which necessitates high-quality, domain-specific training data. However, achieving this without access to curated, proprietary datasets remains a hurdle.
The challenges facing smaller translation companies, coupled with restrictions on data access, could ultimately lead to industry consolidation. It's plausible that smaller companies will face difficulty accumulating the necessary datasets to stay competitive, potentially leading to a scenario where only a handful of large players dominate the field.
Some companies are using crowdsourced data to train their translation models. This can be a useful way to expand the scope of languages handled, but it introduces variability in translation quality due to the differing levels of expertise among contributors.
Looking toward the future, it's becoming increasingly evident that the ownership and development of proprietary datasets will be pivotal for translation technology. Companies that manage to create specialized datasets designed to address the nuances of specific languages will likely be well-positioned for future success. This indicates that the control of valuable datasets could very well become a critical factor in the competitive landscape of AI translation.
Emerging AI Translation Risks How Google's Antitrust Case Could Impact Language Processing Services in 2025 - Regional Translation Companies Replace Big Tech In Government Contracts
Government contracts for language services are increasingly being awarded to regional translation companies, rather than large tech firms. This change is partly driven by new regulations that emphasize specific criteria for AI systems used by government agencies. Smaller translation companies are able to offer lower prices, often using open-source AI models, making them a more attractive option for government agencies looking to cut costs. However, relying on readily available, less rigorously developed models raises questions about translation quality, particularly the ability to handle cultural nuances and context accurately. The speed of translation is increasingly important, and often this speed comes at the cost of accuracy. The challenge for these smaller companies will be to maintain a balance between affordability and the high-quality translation requirements of governmental organizations. Their success hinges on their ability to adapt to the regulatory changes while also upholding standards essential for official communications.
1. **Rise of Regional Translation Players**: Since Google's search limitations, we've seen a noticeable shift towards smaller, regional translation companies. These companies seem to be thriving by offering services that are more attuned to local cultural nuances, areas where larger companies often struggle. It's interesting to see how they are capitalizing on a market previously dominated by giants.
2. **The Speed-Accuracy Trade-off**: Research suggests that prioritizing faster translation often comes at a cost. In striving to meet demands for quick and cheap translations, some companies have seen error rates rise by as much as 30%. This raises concerns about the quality control measures in place, especially when the pressure to deliver low-cost translations is high.
3. **Regulatory Impact on Translation Speed**: Recent data processing regulations have had a dramatic impact on document OCR translation, resulting in a 45% reduction in processing speed. This illustrates how rules meant to protect data can inadvertently limit the progress of AI translation technologies, creating a noticeable bottleneck. It's a good example of how real-world limitations affect these systems.
4. **Open Source Model Limitations**: Companies adopting open-source AI models for translation have reported a higher frequency of errors, particularly in complex language contexts, with error rates sometimes exceeding 25%. It appears that these readily available models often lack the comprehensive training datasets that are necessary for handling language subtleties effectively. It begs the question of whether they are truly reliable for certain tasks.
5. **The Achilles' Heel of OCR**: Studies suggest that OCR processes themselves can introduce a significant number of errors, especially when dealing with documents with unusual layouts or fonts. Error rates can exceed 30%. These errors, introduced early on, have a compounding effect when passed to the translation phase. This highlights a crucial point: translation quality is only as good as the data it's based on.
6. **Specialization's Potential**: A growing trend among smaller translation firms is specializing in specific industries, like medicine or law, where precise language is crucial. This focused approach shows promise in enhancing translation reliability, but it relies heavily on the availability of specialized datasets, which can be challenging to acquire and maintain.
7. **Spotlight on Less Common Languages**: The recent disruption in the translation market has led some smaller providers to focus on less commonly used languages that may have previously been overlooked. This is a promising development, but it also highlights the difficulty of obtaining high-quality training data for these less-resourced languages.
8. **Crowdsourcing Challenges**: Some companies are exploring the use of crowdsourced data for model training, which could broaden the language scope covered. However, it also introduces variability in the quality of translations, as the skill levels of individuals contributing to the datasets can vary widely. It's a double-edged sword.
9. **The Risk of Market Consolidation**: The struggle for smaller players to gain a foothold in the face of larger, more established companies highlights a potential risk of market consolidation. Without access to high-quality proprietary datasets, it's conceivable that only a few large companies will dominate the space for high-quality translation services in the long run.
10. **Balancing Cost and Quality**: The demand for affordable translation services has led to significant price reductions, some as drastic as 60%. While this is positive for consumers, it puts immense pressure on companies to keep costs down. This pressure raises questions about how well companies can maintain the quality of their services while facing such extreme economic challenges. Will they be forced to make trade-offs that might compromise translation accuracy? It remains to be seen.
Emerging AI Translation Risks How Google's Antitrust Case Could Impact Language Processing Services in 2025 - Language Model Training Costs Triple After Google Data Access Changes
The development of sophisticated language models has become significantly more expensive, with training costs for models like GPT-3 tripling in recent times. Estimates now range from a half-million dollars to a staggering $46 million, driven in part by changes in data access implemented by Google. These changes have made it harder for developers to access the large and diverse datasets that are crucial for developing effective language models. This increase in training costs comes at a time when there's growing pressure to deliver faster and cheaper translation services. This focus on speed and affordability can lead to cuts in quality, particularly for less established providers. They may be tempted to cut corners on the comprehensive training needed to produce accurate and nuanced translations, simply to remain competitive. The future of language model development also faces challenges from the projected depletion of training data by 2026, along with the increasing impact of evolving data regulations. Maintaining high standards for accuracy and dependability becomes a significant challenge in this evolving field, especially in applications where precise translation is vital. The drive for cost-effective solutions must be carefully considered against the potential risks associated with under-trained and potentially flawed translation models.
1. **Escalating Training Costs**: Since Google's data access policies changed, the cost of training AI language models has reportedly tripled. This increase, estimated to be as high as 300%, is largely due to the difficulty in obtaining the large and diverse datasets these models need for training. It seems new data regulations are making things much harder.
2. **The Importance of Data Diversity**: Research suggests that using diverse datasets during model training leads to much better results. However, Google's changes limit access to such diverse data, leading to a potential decline in translation quality. Models with limited training data struggle to understand the subtle nuances and variations in human language, leading to inaccuracies and a poorer translation experience.
3. **Challenges for Less Common Languages**: While cheaper translation options are becoming more prevalent, there's a growing concern about the accuracy of translations involving less commonly used languages. Many AI models haven't been trained extensively on these languages, making the reliability of translations, especially in critical communications, questionable.
4. **OCR's Accuracy Issues**: Optical Character Recognition (OCR) plays a vital role in processing documents before translation. Unfortunately, OCR systems often have error rates of over 30%, especially with complex layouts. Until we have more reliable OCR methods, the overall quality of translations will likely be limited. It seems to be the 'Achilles' heel' of these systems.
5. **The Speed-Accuracy Balancing Act**: There's a noticeable trend of prioritizing speed over accuracy in the push for fast and cheap translation. This can increase error rates by 20-30%. This focus on speed, often due to price pressures and the need for quick turnaround times, risks diminishing the overall quality of translation. We have to be careful not to sacrifice accuracy in our quest for speed.
6. **Open-Source Models and Their Limitations**: Many smaller companies have begun relying on open-source language models for their translation services. While appealing from a cost standpoint, these models often lack the comprehensive training datasets that are essential for producing high-quality translations, especially in technical fields. This can lead to frequent errors in translation outputs, making their dependability a valid question.
7. **The Variability of Crowdsourced Data**: Some companies are turning to crowdsourced data for model training, which has the potential to expand language coverage. However, this approach creates inconsistency in translation quality due to varying levels of expertise among the contributors. The quality control aspect becomes a very challenging problem to address.
8. **A Potential for Market Domination**: The competitive landscape of the translation market might be shifting towards market consolidation. As pressure for cheaper translations grows, smaller companies might struggle to stay competitive without access to specialized, high-quality data. This could eventually lead to a situation where only a handful of large companies dominate the market, potentially hindering innovation and access to quality translation services in the long run.
9. **Specialized Translation and Its Hurdles**: An interesting development is the specialization in fields like legal or medical translations, where accuracy is paramount. However, this approach requires significant effort to gather specialized datasets. The expense and difficulty of acquiring these datasets show the challenges of creating effective, specialized machine translation systems.
10. **Shifting Government Preferences**: Government contracts for translation services are increasingly going to smaller, regional firms due to lower prices. However, the drive for affordability can compromise translation quality, particularly in instances requiring high precision, such as in legal or government communications. It's a delicate balance to maintain accuracy and affordability.
More Posts from aitranslations.io: