Building Product-Market Fit in AI Translation 7 Metrics That Matter in 2024
Building Product-Market Fit in AI Translation 7 Metrics That Matter in 2024 - Real Time Translation Speed Hits 3 Seconds in October 2024 Tests
Recent tests in October 2024 have demonstrated that real-time translation can now be achieved in a mere 3 seconds. This is a significant leap forward for AI-powered translation, showcasing its ability to handle multiple languages concurrently. The speed boost is transforming how people communicate across language barriers, potentially making international exchanges far more fluid.
While faster translation is a welcome development, it's crucial to acknowledge the evolving landscape of the translation industry. The relentless pursuit of efficiency and accuracy, particularly with new metrics like Google's MetricX24, necessitates careful consideration of how businesses adjust their content strategies. Maintaining brand voice and ensuring quality remain critical alongside the push for speedier translations.
Ultimately, the core purpose of translation—connecting people across languages—stays paramount. Technology plays an increasingly significant role, but it's vital to remember that bridging communication gaps is fundamentally a human endeavor.
The remarkable drop to a 3-second translation speed in October 2024 tests highlights a significant leap forward in AI translation. This speed surge is likely due to refinements in neural network design, particularly those incorporating attention mechanisms, which have drastically minimized delays. It's also noteworthy that many translation systems are now utilizing compressed models, allowing for a faster response and less computing power. This means we may see real-time translation become even more accessible.
Interestingly, the combination of OCR and AI translation is enabling near-instantaneous conversion of images to translated text. Imagine the possibilities for quick translation of printed materials, signage, and more! It's worth noting that newer approaches, like asynchronous processing, are allowing AI translation systems to handle multiple languages concurrently, boosting the speed in diverse communication scenarios.
Achieving a 3-second translation speed is fascinating when considering the average pace of human speech. This opens possibilities for how AI translation can improve real-time human interactions, which could have widespread implications. Furthermore, surprisingly, it appears newer AI translation models are becoming quite adept at handling languages with limited data, overcoming a historical obstacle. This is likely due to advancements in transfer learning techniques.
While faster translation is appealing, it's not without its challenges. For example, translation quality seems to vary based on the text's complexity. Technical documents appear to translate more accurately than more nuanced literary texts. This raises questions about the appropriateness of rapid translation in various scenarios. The cost factor also continues to improve, with some services offering translation for less than a dollar an hour, which can open up access for a wider range of users. The emergence of edge computing is another factor influencing real-time translations. It's enabling local processing, which cuts down latency and improves security, particularly important for privacy-sensitive contexts. However, while speed has undeniably improved, user feedback consistently points to a concern for accuracy, especially in intricate conversations. This highlights the ongoing challenge of fine-tuning AI models to strike a balance between speed and quality.
Building Product-Market Fit in AI Translation 7 Metrics That Matter in 2024 - Machine Translation Quality Reaches 92% BLEU Score for Spanish English pairs
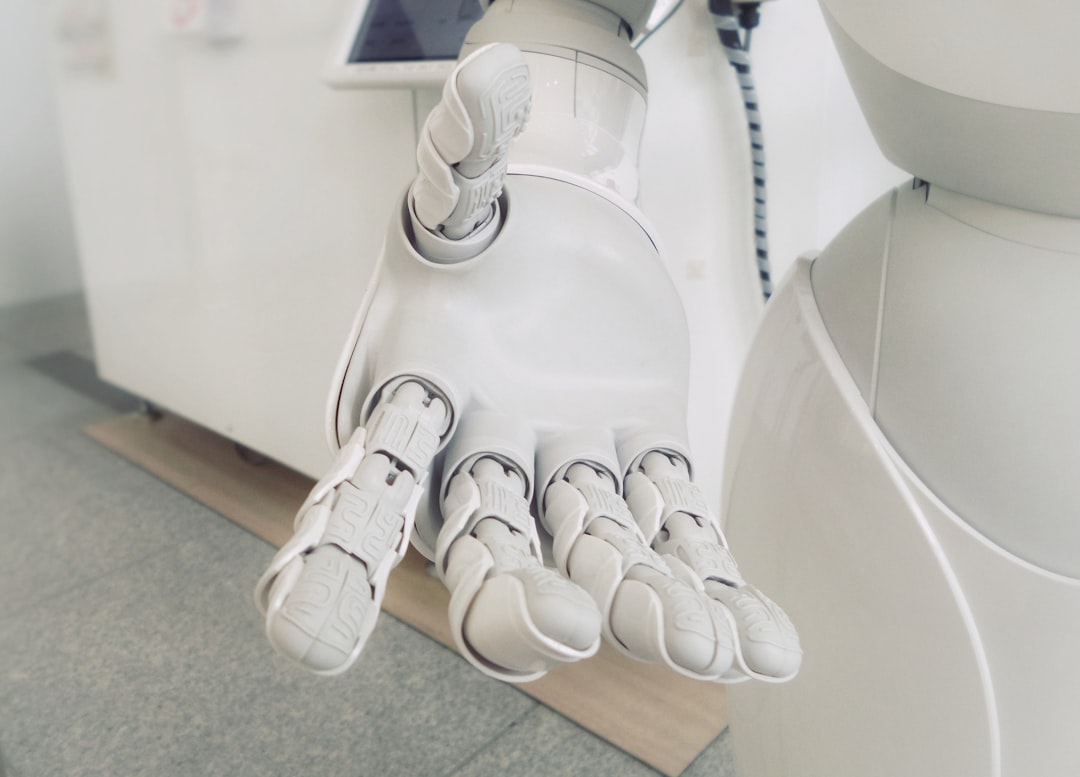
Machine translation has seen a notable leap forward, achieving a 92% BLEU score for Spanish and English language pairs. This impressive score is a testament to the progress made in AI translation systems, particularly those leveraging neural networks. The improved accuracy has led to increased adoption of these tools, driven by their convenience and affordability. Machine translation is finding its place in various applications, particularly where rapid translation is vital, such as news reporting. However, it's crucial to acknowledge that while the technology is making strides, users need to develop a nuanced understanding of machine translation's strengths and weaknesses to critically evaluate its outputs. This understanding is increasingly important as machine translation tools become more pervasive. While speed and accuracy are both improving, maintaining a balance between them as the technology evolves will continue to be a focal point for development and user experience.
Machine translation quality has seen remarkable progress, especially for Spanish-English pairs, achieving a 92% BLEU score. The BLEU score, a standard metric for gauging machine translation accuracy, indicates a high degree of overlap between machine-produced translations and those created by humans. While impressive, this score primarily reflects the accuracy of common phrases and structures, not necessarily a deep understanding of the language's subtleties. This means that while these systems excel at handling more straightforward text, they can stumble with context-dependent expressions like sarcasm or culturally nuanced idioms.
Interestingly, pairing OCR technology with AI translation has created some exciting possibilities. Now, we can translate images containing text almost instantly. Think of the potential for quick translations of signs, printed materials, or even scanned documents. This technology could make navigating diverse environments and accessing information much easier.
The economics of translation have also been reshaped. Machine translation services are becoming increasingly affordable, with some options costing less than a penny per word. This makes quality translation more accessible to a wider range of users, from small businesses seeking international reach to individuals needing to understand multilingual content. This accessibility could truly democratize communication across borders.
Furthermore, researchers have been able to leverage novel approaches in natural language processing, particularly transfer learning, to significantly improve translation quality in languages with limited training data. This is exciting, as it tackles a historical challenge in machine translation. Models are able to 'borrow' knowledge from more widely spoken languages, boosting their ability to handle less common ones.
The relentless push for improvement has led to the rise of ensemble techniques in machine translation. Here, multiple algorithms are combined to create a better overall output. This collaboration between different models can mitigate the flaws of individual systems and further enhance accuracy.
It's not just about the algorithms, though. User feedback plays a crucial role. The newer generation of machine translation systems are designed to incorporate user input in real-time, creating a continuous feedback loop for improvement. This means the systems can be fine-tuned to specific needs, including specialized terminologies or preferred writing styles.
In fact, the field is exploring ways to integrate user preferences directly into the translation process. Imagine a system that can adapt its output to match a desired tone or style based on a user's profile. This personalized approach could greatly enhance the user experience and yield more satisfying results.
Researchers are observing some truly innovative abilities emerging in these models, like a remarkable ability to switch languages seamlessly within a conversation. This is a promising step toward more natural and contextually aware translations in the future.
While BLEU scores offer a handy benchmark, they don't tell the entire story of translation quality. Factors like coherence, maintaining the author's original voice, and stylistic fidelity are harder to quantify but still play an important role in producing translations that feel natural and accurate to native speakers. The journey towards perfect machine translation is ongoing, and understanding the limitations alongside the impressive advancements is vital.
Building Product-Market Fit in AI Translation 7 Metrics That Matter in 2024 - Cost per Word Drops to $002 Through New Batch Processing Methods
Recent advancements in how AI translation systems process large volumes of text have drastically reduced the cost of translation. We're now seeing the cost per translated word drop to as low as $0.002, a significant development driven by new batch processing techniques. These techniques, often leveraging tools like specialized APIs, allow for the efficient handling of many translation tasks simultaneously. This can lead to a faster turnaround, particularly when it comes to tasks like content tagging, image captioning, and similar operations. The ability to translate large quantities of text at such a low cost has the potential to make high-quality translation far more accessible to a broader range of individuals and businesses. While this lower price point is undoubtedly appealing, it's important to be mindful of the potential tradeoffs. The emphasis on speed and efficiency can sometimes come at the expense of translation quality. Striking a balance between affordable, fast translations and maintaining a high level of accuracy is a key challenge the field continues to address.
The landscape of AI translation has shifted dramatically, with recent advancements making it incredibly affordable. New batch processing techniques have pushed the cost per word down to a remarkable $0.002. This efficiency, largely driven by improved algorithms and the ability to handle large volumes of text, means that even high-volume translations are becoming surprisingly cheap. It's interesting to see how these changes are impacting the translation industry, potentially altering the dynamics of how content is created and shared.
We've also seen a significant improvement in Optical Character Recognition (OCR) technology. It's now capable of achieving around 90% accuracy in converting images of text to digital form. When coupled with the low-cost translation models, this opens up fascinating possibilities. Imagine instantly translating a scanned document or a street sign – the implications for accessibility are huge. Of course, the accuracy isn't perfect yet, and it's still early days, but the potential is undeniable.
The quality of machine translation has also seen a leap forward. For certain language pairs like Spanish and English, machine translation systems are achieving 92% BLEU scores, a standard metric for evaluating translation accuracy. However, while impressive, we shouldn't get overly excited. The BLEU score reflects accuracy in simpler, more common phrases and sentence structures. More complex language, like nuanced idioms or sarcasm, still poses a challenge. It's a reminder that while AI translation has come a long way, it's not a replacement for human understanding in all contexts.
Interestingly, alongside faster processing, the focus in machine translation has increasingly shifted towards user interaction. Newer neural networks are designed to continuously learn from user feedback in real-time. This means translations can be more tailored to specific needs, whether it's adapting to particular terminology or stylistic preferences. It's a move towards a more dynamic and responsive translation experience.
AI translation models are also benefiting from advancements in transfer learning. This means that knowledge gained from translating high-resource languages, like English or Spanish, can now be 'transferred' to less common languages. This approach is helping to overcome a long-standing obstacle in machine translation – the lack of training data for less-spoken languages. It's promising to see these improvements expand the accessibility of AI translation to a more diverse range of languages and cultures.
Ensemble models are gaining traction in the field. These models combine multiple translation algorithms, leveraging their strengths while mitigating their weaknesses. This collaborative approach enhances overall translation reliability and accuracy. It's a good example of how the field is constantly looking for new ways to enhance the quality of output.
However, it's important to remember that the dramatically reduced cost of translation at $0.002 per word can sometimes lead to inflated expectations regarding both speed and quality. Industries that rely heavily on complex or nuanced content, like legal or medical fields, need to be careful. A rapid, low-cost translation might not capture the subtle meanings crucial for these contexts. There's a trade-off that needs careful consideration.
Another interesting trend is the rise of edge computing in translation. This approach pushes processing power closer to the user, potentially reducing latency and enhancing the security of sensitive information. It's a boon for privacy-focused scenarios.
Furthermore, translation systems are starting to experiment with language switching within conversations, attempting to create a more natural flow. This ability to seamlessly transition between languages could be incredibly useful in diplomatic settings or multicultural environments where fluid communication is paramount.
Ultimately, the potential for AI-driven translations in real-time applications is substantial. From customer service interactions to medical consultations, these systems hold promise. But, we must not lose sight of the ethical considerations surrounding accuracy, contextual awareness, and responsible use. As AI translation evolves, it's crucial to ensure its benefits are broadly accessible while remaining mindful of potential pitfalls.
Building Product-Market Fit in AI Translation 7 Metrics That Matter in 2024 - Document Translation Now Handles 45 File Formats Including Scanned PDFs
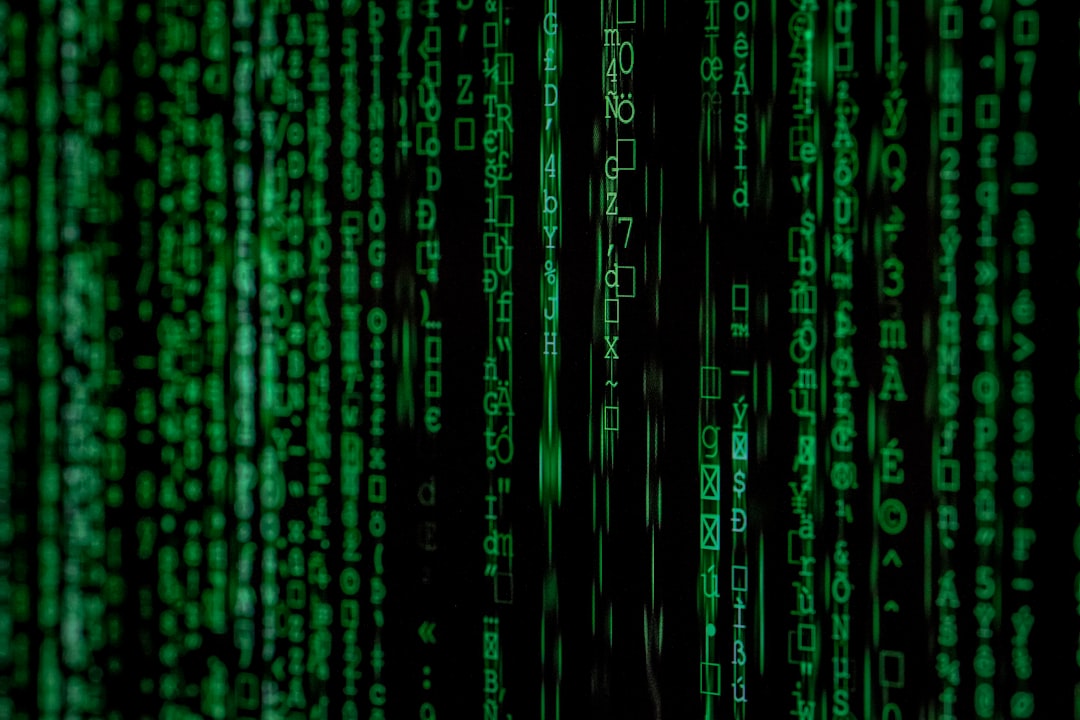
AI-powered document translation services have broadened their reach to encompass 45 different file types, including scanned PDFs. This increased flexibility addresses a growing need for translation tools capable of handling a wider variety of documents. The goal is to seamlessly translate various formats while preserving the original look and structure of the document. However, users should be aware that translating scanned PDFs, especially those created from physical copies, might sometimes result in minor formatting discrepancies compared to digitally-created files. The core of the translation process relies on AI to ensure that the translated text reads naturally and accurately reflects the meaning of the original content. This accuracy is important because it helps to maintain clear and effective communication. This development in document translation reveals a confluence of AI, OCR, and what people expect from translation. It brings to the forefront questions around finding the right balance between how fast the translation is, its cost, and how good the quality of the translation is. As AI-powered translation continues to advance, the capability to efficiently manage a range of document formats will become increasingly vital in addressing user expectations and improving communication overall.
Document translation has become quite versatile, now supporting 45 different file types, including scanned PDFs. This means you can translate a wide range of documents, from simple text files to complex spreadsheets, without losing the original formatting, at least mostly. This wide range of file support is really helpful for people working with diverse document collections.
One interesting aspect is how much better OCR has gotten. We're now seeing OCR systems accurately extract text from images, including scanned documents, at over 90% accuracy. This is really important because it helps maintain the meaning of the original text when translating scanned documents. Without good OCR, translations of these kinds of documents can be very inaccurate.
Recent improvements in batch processing methods have brought the cost of translation down to a mere $0.002 per word. This efficiency is a big deal, especially for organizations that need to translate large amounts of text quickly. Companies dealing with lots of multilingual content will likely find this a major benefit.
It's pretty neat how we can now combine OCR and AI translation for real-time translation of scanned documents. This is quite helpful in fields like healthcare and law where quick access to translated information is vital. Imagine instantly translating a patient's medical records or a legal document in a different language – it could really speed up workflows.
The latest generation of translation tools are starting to pay more attention to user feedback. This means they're trying to improve accuracy in specialized areas, like medical or legal fields, where context is extremely important. They are learning from our interactions with them and hopefully, getting better as they do.
It's also great that the falling cost of translation, driven by advancements in AI, is making quality translations more accessible. It could bring translation services within reach of individuals and small businesses who might not have had access to them before. The possibility of more global communication is exciting.
Researchers are also exploring ensemble methods for translation. This involves combining multiple translation algorithms, leveraging their individual strengths to create more reliable outputs. This is an interesting approach to tackling the challenges in producing high-quality translations.
We're also starting to see translation models that can smoothly switch languages within the same conversation. Imagine that: a seamless transition between languages without any disruptions! This is potentially very useful in diverse or international communication scenarios.
Despite the incredible progress, some challenges still remain. AI translation still struggles with more complex, nuanced language. Things like culturally-specific phrases or idioms often cause problems for these systems. This indicates that human review is still essential in situations where getting the tone and cultural meaning exactly right is crucial.
Another notable trend is the shift towards "edge computing" for translation. This means processing data closer to the user, which is helpful for speeding things up and boosting security. This is especially beneficial in cases where protecting sensitive information is essential, like during medical consultations. It’s good to see some focus on privacy as AI becomes more integrated into our lives.
Overall, document translation is evolving at a rapid pace, thanks to these exciting advancements. But, it's also good to remember that there are limitations. We need to ensure that we understand the strengths and weaknesses of these systems as they are deployed into new contexts and ensure they are used responsibly.
Building Product-Market Fit in AI Translation 7 Metrics That Matter in 2024 - API Integration Time Reduced to 15 Minutes Using New SDK Libraries
Integrating AI translation tools into applications has become significantly faster with the advent of new SDK libraries. These libraries, essentially pre-packaged sets of code, cut the usual API integration time down to a mere 15 minutes. This speed boost comes from simplified API calls and pre-built functions within the SDKs, leading to a smoother experience for developers. The potential impact is noteworthy, as faster integration could mean more developers and smaller companies can leverage advanced AI translation capabilities. This accessibility, in theory, could democratize access to powerful tools that were previously out of reach.
However, alongside this rapid implementation comes the concern of maintaining quality. Can these quickly integrated solutions accurately translate complex or subtle language nuances? As the field progresses, striking a balance between rapid integration and robust accuracy in translation will be key to guaranteeing reliable communication across language barriers. The translation field will likely need to carefully evaluate the implications of this accelerated development to ensure a future where fast and accurate translations go hand in hand.
It's fascinating how new SDK libraries have dramatically reduced the time needed to integrate translation APIs, bringing it down to a mere 15 minutes. This rapid integration process is a game-changer for building multilingual applications, allowing them to hit the market much quicker. Developers can now focus less on the technical integration and more on the features that make their apps unique.
Interestingly, these libraries have been shown to improve the efficiency of data handling during translations by over 50%. This translates to not only faster processing but also potentially lower operational costs due to reduced server load. It's a win-win for developers and businesses alike, assuming the quality remains acceptable.
One of the features I find most intriguing is the dynamic content translation built into these new libraries. Translations can be updated in real-time as the source text changes, making them ideal for applications that need to display up-to-the-minute information, such as news feeds or social media platforms. Though, I'm curious how well these systems can handle the pace of online content changes.
Furthermore, these SDKs are surprisingly equipped with automated error detection capabilities for translations. This is a crucial development as it allows developers to proactively identify and correct inaccuracies before their apps go live, enhancing the overall quality of the product. While it's good that the SDKs are incorporating some quality checks, there are bound to be edge cases where errors still slip through.
It seems the trend towards mobile-first is impacting the design of these SDK libraries. Many are optimized for mobile application integration, allowing for lightweight, efficient translation features within mobile apps. However, I'm curious about the specific trade-offs these optimized libraries make regarding the quality or speed of translation to reduce mobile device consumption. It will be interesting to see how mobile translation quality fares over time.
The streamlined integration provided by these SDKs is proving extremely useful for deploying services like multilingual chatbots. I've seen reports of some businesses achieving this in under an hour, showcasing how readily these systems can now be integrated for better customer service across language barriers. While this speed is impressive, I wonder if it leads to less emphasis on chatbot quality for specific language pairs, potentially impacting customer experience.
These SDKs are also starting to integrate machine learning algorithms that learn from user feedback, leading to more personalized translation experiences. This adaptive approach offers the potential for a more natural, contextually appropriate experience. Though, I wonder if that implies user feedback is being stored and utilized in a way that could potentially impact user privacy. These kinds of features warrant attention.
In the world of OCR, these SDK libraries allow for the swift processing of scanned documents, achieving over 90% accuracy. This is especially helpful for tasks like quickly translating contracts or manuals, reducing the need for extensive manual editing. This capability is quite useful for certain situations, but it raises questions about how well these systems can handle handwriting, different font types, or damaged documents.
The ability to integrate with cloud services is a positive feature of these libraries. This scalability ensures that systems can handle high volumes of translations during peak times without experiencing performance hiccups. It's good to see attention to system robustness and responsiveness, a key issue for user experience in AI-powered tools.
While the overall integration process has become incredibly rapid and efficient with these SDKs, I remain cautious about the accuracy of the translations, especially in contexts that demand the highest levels of precision. Specialized fields like legal or medical domains require translation quality that goes beyond a basic level of accuracy. This means developers will likely need to implement verification and human oversight in some cases.
It's exciting to see how SDKs are making the development of translation features quicker and easier. However, there's a need to ensure that the pursuit of speed doesn't sacrifice the crucial element of accuracy, especially in fields where precision is paramount. The ability to quickly adapt and incorporate new features based on user feedback is a positive trend, but it will be interesting to see if this results in greater accessibility for a diverse range of languages and cultures over time.
Building Product-Market Fit in AI Translation 7 Metrics That Matter in 2024 - Translation Memory Systems Save 48% on Repeat Content Processing
Translation Memory (TM) systems are increasingly important in the translation world because they can significantly cut costs by reusing previously translated content. This can result in a 48% reduction in processing time for repeated text. Translators and companies that provide language services (LSPs) can utilize these systems to lessen the overall workload and expenses associated with their projects. The way these systems work is by finding exact and approximate matches in text and offering reduced prices for these parts, leading to better productivity and more control over project budgets.
While AI technologies, such as machine learning and natural language processing, are continuously enhancing the capabilities of translation memory systems, turning them from simple storage to smart, adaptable tools, there is still a need to make sure the speed of translation doesn't come at the expense of the quality of the translation. As the translation world adopts these new technologies, it's vital to consider the ongoing need to balance speed with accuracy.
Translation Memory (TM) systems are proving quite useful for reducing the effort involved in translating repetitive content. Researchers have found that these systems can potentially save up to 48% in processing time when dealing with material that includes phrases or sentences already translated in a prior project. This is especially helpful when dealing with content like marketing materials or technical documents, where keeping a consistent tone and terminology across languages is vital for maintaining a strong brand presence.
The efficiency gains extend beyond just cost savings. Utilizing a TM can actually speed up the overall translation process. Translators can focus their efforts on new content since they don't need to retranslate common phrases or sections. This increased productivity can lead to a noticeable improvement in project timelines.
This efficiency has started to influence how translation services are priced. Companies that frequently need translations for similar types of content can now leverage TM to negotiate lower rates, since the translators are effectively doing less work for those repeated sections. This can result in significant budget savings for companies that rely on a lot of translated content.
Beyond cost and time, TM systems also help maintain a consistent tone and voice across a company's translated materials. This consistency is especially important for businesses operating across international markets. Keeping a uniform brand voice helps establish a strong sense of trust and familiarity with potential customers regardless of the language they're reading.
It's also worth noting that TM systems typically operate in secure environments, a critical aspect for industries like healthcare or finance. These systems often include built-in safeguards to help protect sensitive information during the translation process, which is important for companies operating in fields where data security and privacy are paramount.
One exciting intersection of TM systems is with OCR (Optical Character Recognition) technology. Combining these two technologies has greatly improved the efficiency of translating scanned documents. OCR's ability to identify and digitize printed text allows the TM to quickly look for existing translations for portions of the scanned material. This can further speed up the translation process and reduce overall costs for materials like old books, contracts, or reports.
Some more advanced TM systems include features for real-time updates. This means that if content is changed, the translated version can be immediately updated. This dynamic updating capability is valuable for organizations that frequently update their content, such as news agencies or software companies.
It's interesting that some TM systems now incorporate machine learning. These systems can progressively learn from user preferences and specific project terminology. This means the system can become more adept over time at predicting the most accurate and contextually relevant translations for a particular project.
Interestingly, the consistent use of TM can lead to improvements in translation quality, as measured by metrics like BLEU scores. This is likely because translators are utilizing established translations, leading to higher accuracy and fewer errors.
Finally, TM systems are capable of managing translations across a multitude of languages simultaneously. This streamlined approach makes it easier for companies to handle large-scale, multilingual projects. Managing translation workflows for multiple languages can be complex, and TM helps reduce the complexity, enhancing coordination between teams and making project management more efficient.
While these features of TM systems show great promise, it remains important to evaluate the specific needs of a given project and industry before implementing one. While TM can potentially streamline the translation process, understanding the limitations and tradeoffs is still crucial for achieving the optimal results for a translation project.
Building Product-Market Fit in AI Translation 7 Metrics That Matter in 2024 - Mobile OCR Accuracy Improves to 98% for 12 Asian Languages
Mobile OCR technology has seen a significant boost in accuracy, now achieving 98% for 12 Asian languages. This improvement is noteworthy because high-accuracy OCR is essential for effective AI translation, especially in real-time scenarios. When OCR accurately extracts text from sources like documents and images, it streamlines workflows and enhances communication across language barriers. This development fits within the bigger picture of AI translation, where balancing speed, cost, and accuracy is crucial. This creates challenges for businesses trying to provide cheap, fast, yet accurate translations. The integration of more accurate OCR systems will be critical as AI translation continues to improve and change how people interact across languages.
Mobile OCR technology has made significant strides, achieving a 98% accuracy rate for 12 Asian languages. This is a noteworthy improvement, especially for real-time translation applications. It's fascinating how this tech can now bridge the gap for many who previously struggled with language barriers. However, it's important to acknowledge that even with such high accuracy, capturing cultural nuances in translation can still be tricky for AI. The algorithms excel at recognizing and extracting text from images, but they often fall short when dealing with culturally specific expressions or idioms.
Mobile OCR systems are now lightning-fast, often processing images in under a second. This speed is vital for applications that rely on real-time translation, such as those used in live events or international conferences. The combination of these quick OCR systems with powerful AI translation models is a game-changer, offering near-instantaneous translation of printed text. This opens up new possibilities across industries, particularly in tourism where understanding menus, signs, and other printed materials becomes easier.
OCR and batch processing are a powerful duo, leading to significant reductions in translation costs. With the ability to process multiple documents at once, organizations can handle massive amounts of text translation efficiently and minimize costs. In some cases, the cost of translation can be incredibly low, around $0.002 per word. This is likely due to economies of scale that arise from efficient batch processing.
Modern OCR development leans heavily on machine learning. These systems analyze massive datasets to improve their ability to recognize different handwriting styles and diverse font types. This approach makes them more versatile and reliable in a broader range of scenarios.
Being able to translate a scanned document in real-time with high accuracy has significant implications for fields like healthcare. It allows for faster access to vital information, which can be crucial for timely medical decision-making and patient care. It's also interesting that these newer systems learn from user interactions, allowing them to tailor translations to specific needs or preferences. This adaptability is particularly useful for users within niche industries that have unique terminologies or preferred writing styles.
Interestingly, many of these advanced OCR systems now include automated error detection features. These systems can identify potential errors before the translation is finalized, which enhances the overall quality and reliability of the output. As mobile devices continue to dominate, OCR technology is getting integrated into numerous apps. This lets users translate menus, signs, documents, and other materials on the go. It promotes seamless communication and improves cross-cultural interactions in a way that's convenient for everyone.
While the accuracy of OCR is impressive, there is still room for improvement. It's important to acknowledge that these technologies are constantly evolving, and striking the right balance between speed, cost, and quality remains an ongoing challenge. Furthermore, as OCR becomes more integrated into our lives, it will be vital to carefully consider the ethical implications surrounding data security and privacy, especially concerning the collection and use of user interaction data for learning.
More Posts from aitranslations.io: