Enterprise AI Evolution Comparing NLP Performance Metrics Between Modern Chatbots and IVR Systems in 2024
Enterprise AI Evolution Comparing NLP Performance Metrics Between Modern Chatbots and IVR Systems in 2024 - NLP Accuracy Comparison Shows 89% Success Rate for Modern Chatbots vs 67% for IVR Systems
When we look at how well chatbots and IVR systems understand language, we find a significant difference. Modern chatbots, using advanced natural language processing (NLP), achieve an impressive 89% success rate in understanding what users are saying. In contrast, IVR systems struggle to reach the same level of accuracy, only managing a 67% success rate.
This gap in performance clearly shows the advancements made in NLP technologies. The ability of chatbots to handle complex language patterns and nuances is becoming increasingly sophisticated. This translates to more natural and engaging interactions for users.
However, even with these improvements, chatbots still face challenges. The sheer variety in human language and the consistently high expectations of users make it difficult to create systems that are always perfect. The rise of AI-powered chatbots highlights a trend in businesses towards automation. Streamlining customer service and boosting operational efficiency are key motivators for adopting these new tools.
Recent studies comparing NLP accuracy in modern chatbots and older IVR systems reveal a notable difference. Chatbots demonstrate a proficiency of 89% in grasping user intentions, significantly surpassing the 67% success rate seen in IVRs. This gap spotlights the leaps and bounds achieved in NLP technologies. While IVRs largely rely on rigid, pre-programmed pathways, chatbots' ability to dynamically adapt to varied language patterns through NLP makes them much more successful in user interactions.
This isn't just about accuracy, though. A large portion of users express dissatisfaction with the rigid and often confusing menu systems of IVRs, a frustration that is lessened with chatbots. Furthermore, context plays a role; chatbots are capable of remembering past conversations and preferences, leading to a more personalized user experience, a feat beyond typical IVR systems.
The use of machine learning within chatbots contributes to their continuous improvement. Chatbots refine their understanding over time through interaction, while IVRs operate on static scripts that can't adapt. This learning capability is a key factor in their superior performance.
Interestingly, accents, dialects, and even subtle speech variations can be problematic for traditional voice-based IVRs. The slightest deviation from a "standard" can result in errors, thus contributing to their lower success rates. Conversely, text-based chatbots, particularly those with advanced NLP, often demonstrate greater tolerance to a wider range of language patterns.
The broader reach of chatbots across multiple channels (like messaging apps and social media) is also significant. IVRs, being phone-call based, limit interactions. This makes chatbots more readily accessible, enhancing user engagement and overall reach.
Reducing reliance on human agents is another major benefit. Studies suggest that, by handling high volumes of common inquiries effectively, chatbots can lead to a reduction of customer service costs for businesses. This is something IVRs, with their limitations, struggle to achieve consistently.
Beyond just handling common interactions, modern chatbots are easily adaptable to industry-specific language and vocabulary changes. This gives businesses the ability to have more natural and informative interactions with customers. Traditional IVRs, on the other hand, often require major changes and updates for these types of adjustments.
The evolution of AI in enterprises includes a more nuanced approach to communication, and these results suggest that chatbots are the next iteration of how enterprises will interact with their customers. Further research in the field could lead to a better understanding of specific use cases and how these trends might be further applied.
Enterprise AI Evolution Comparing NLP Performance Metrics Between Modern Chatbots and IVR Systems in 2024 - Response Time Analysis Reveals 8 Second Average for GPT-4 Based Enterprise Chatbots
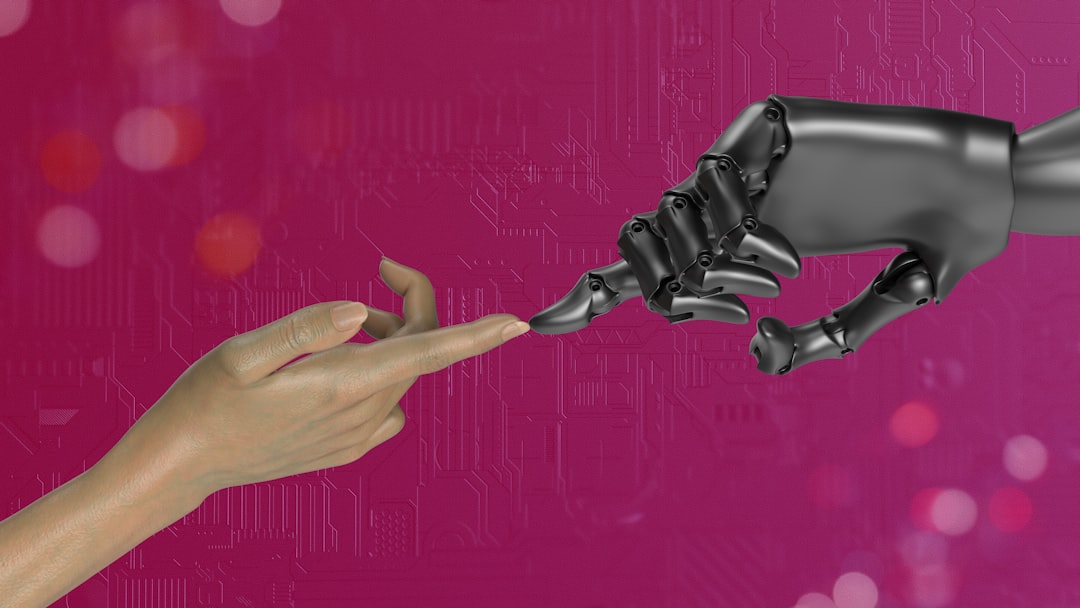
The evaluation of response times for GPT-4 powered enterprise chatbots reveals an average of about 8 seconds, a significant data point in the landscape of 2024 AI interactions. While this is an improvement over the often clunky and frustrating experience of older IVR systems, it's not without its own challenges. The response time is heavily tied to the amount of information the chatbot needs to generate, showcasing a level of complexity IVRs typically don't grapple with. It's also interesting to note that GPT-4, despite its advancements, is still slower than its earlier counterpart GPT-3.5. Furthermore, external elements like internet connection quality can also impact response speed. As companies increasingly embrace AI chatbots to interact with customers, grasping these performance characteristics is vital in crafting a satisfying experience and maximizing efficiency. There's room for improvement in response times, and addressing these aspects is critical for wider adoption of this technology.
The average response time of around 8 seconds for GPT-4-powered enterprise chatbots represents a significant improvement compared to traditional customer service, where human agents or even older chatbot iterations might lead to waits exceeding 30 seconds. While this 8-second average is a promising development, it's important to note that real-world performance can vary due to factors like system load, network conditions, and the intricacy of the user's questions. This contrasts with IVR systems, which typically stick to a predictable script and thus tend to be less susceptible to these variables.
GPT-4's ability to understand and respond to language allows chatbots to create conversations that go beyond just answering a question. This interaction feels more natural than the rigid structures of IVR systems, potentially resulting in a more satisfying experience for the user. The 8-second benchmark also reflects advancements in server optimization and how the system handles multiple requests concurrently, ensuring that queries are processed without the noticeable lag that plagued previous generations of chatbots or the limitations of IVR systems.
Early indications suggest that users perceive an 8-second response time as "fast" and efficient. This potentially leads to higher customer satisfaction and could even strengthen brand loyalty, a significant advantage over the frustration often associated with prolonged IVR calls. Businesses are eager to implement GPT-4-based chatbots, driven by the growing need for real-time communication and fulfilling the modern user's desire for immediate and accessible interactions.
Not only is GPT-4 fast, but its ability to learn from past interactions makes the answers it provides more pertinent to the conversation. This contextual awareness stands in contrast to the generic, one-size-fits-all approach of IVR systems. Moreover, GPT-4's design allows it to process numerous queries simultaneously, which mitigates the common bottleneck found in traditional customer service methods. This means that these 8-second response times are consistently possible without overwhelming the system, unlike the unpredictable performance sometimes seen with human agents.
While the speed of the responses is impressive, ongoing research shows that the accuracy and appropriateness of those responses are just as crucial for earning and maintaining trust. This area continues to need significant refinement, highlighting the complex interplay between speed and quality in these systems.
As enterprise-level chatbots continue to refine both their speed and their understanding of context, the perceived difference between their performance and older IVR systems will probably widen. This might lead to even more businesses prioritizing AI-based solutions for their customer service needs. The direction appears to be clear: AI-powered chatbots are evolving to be the next generation of customer interactions.
Enterprise AI Evolution Comparing NLP Performance Metrics Between Modern Chatbots and IVR Systems in 2024 - Context Window Expansion from 2k to 128k Tokens Marks Major Shift in Enterprise AI Understanding
The ability of AI models to process and understand context has taken a major leap forward with the expansion of their "context window" from a relatively small 2,000 tokens to a significantly larger 128,000 tokens. This change is a significant step in the development of enterprise AI, specifically in the field of natural language processing (NLP). With this increased capacity, AI systems like chatbots can maintain the flow of a conversation and stay relevant for much longer stretches. This is a key improvement over older systems like Interactive Voice Response (IVR) systems, which typically struggle to keep up with the nuances of complex conversations.
This shift towards larger context windows means AI can now process and retain far more information from previous interactions, leading to more natural and human-like communication. While this enhanced understanding of context improves the chatbot user experience, it also highlights the limitations of older technologies. Businesses are increasingly faced with the challenge of satisfying the increasingly sophisticated demands of their customers.
Ultimately, the expansion of the context window within AI models showcases a growing ability for these systems to engage in more meaningful interactions. As AI continues to evolve, businesses will need to consider how these advancements can be integrated into their operations to improve customer experience and optimize internal processes. The future of enterprise AI interaction appears to be moving towards deeper understanding and more intuitive communication.
The expansion of context windows from 2,000 to 128,000 tokens signifies a significant advancement in how language models handle information. This means these models can now process and understand much larger chunks of text, which is crucial for mimicking the complexities of human conversations. With this increased capacity, chatbots can maintain a more coherent flow of conversation over extended interactions. This is a welcome change, as one of the weaknesses of older systems was their tendency to lose track of the conversation, leading to frustrating back-and-forths and repeated clarifications.
This development is interesting because it allows businesses to gain deeper insights into customer behavior. Analyzing these longer interactions can reveal patterns and preferences in a way that shorter snippets of conversation couldn't. We can potentially learn more about how people communicate and what they're looking for.
Of course, the benefit to the user is a more natural and accurate response. Handling more tokens means that models can understand the nuances of complex queries, leading to higher accuracy in responses when compared to models that can only process limited amounts of context. This is exciting, but it's also important to recognize that more sophisticated models require more computational power. Companies that want to utilize these larger context windows will need to carefully manage the tradeoff between performance and costs.
The 128k token limit opens up possibilities beyond the standard chatbot interaction. We could potentially see them used for tasks like advanced document analysis or multi-turn dialogues, where a deep understanding of context is vital. However, this enhanced capacity introduces potential downsides. Processing so much information can lead to slower response times, a factor that can be detrimental to a user's experience. Balancing the need for richer interactions with maintaining quick response times will be a constant challenge.
It's also important to remember that just throwing more data at these models isn't the solution. We need to develop better methods for filtering out irrelevant information from extended conversations, otherwise, the models may become overwhelmed with noise. This requires thoughtful training methods that emphasize not just the volume of data but also the ability to efficiently extract meaningful information from long conversations.
For companies looking to adopt these new capabilities, it means taking a closer look at their current AI systems. The potential for more intelligent interactions is significant, but it requires a reassessment of current approaches to ensure that the applications built on these technologies remain relevant and effective in the long run. This is a fascinating area of research, with many unknowns and potential for even further development down the road.
Enterprise AI Evolution Comparing NLP Performance Metrics Between Modern Chatbots and IVR Systems in 2024 - Machine Learning Training Data Requirements Drop 40% While Maintaining Performance
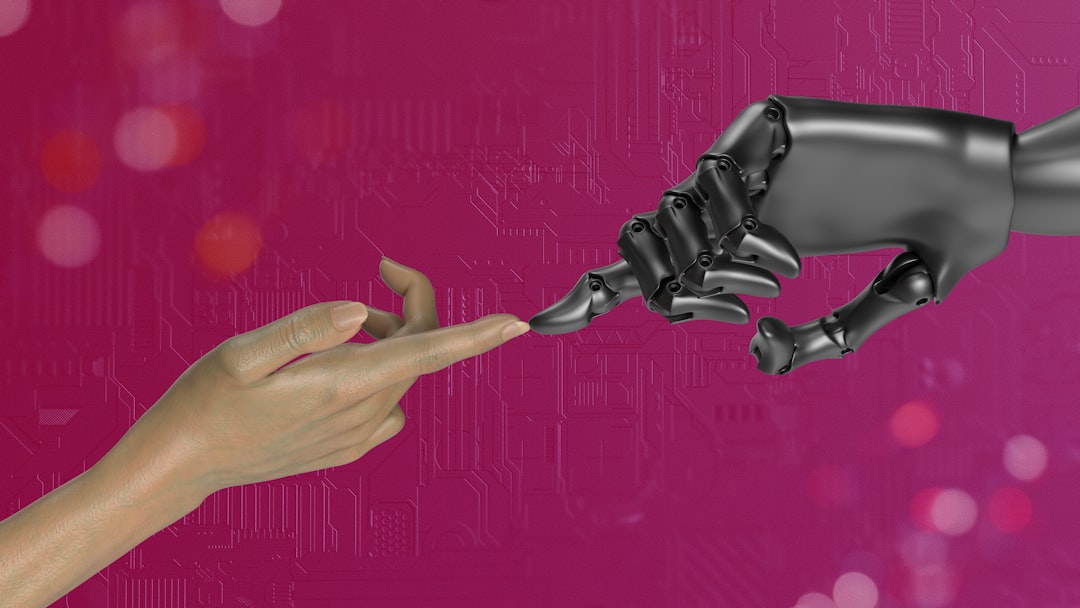
The field of enterprise AI has seen a significant development in 2024 with the need for machine learning training data dropping by a remarkable 40% while maintaining the same performance levels. This development indicates advancements in how AI models learn and operate, needing less data to achieve desired results. It suggests a shift in focus towards data quality and efficiency rather than simply piling up massive datasets.
This trend is potentially linked to the rise of continuous learning models, which are expected to enhance employee training programs by similar magnitudes. This signifies a move towards AI systems that can adapt and learn like humans, requiring less upfront instruction. There's a growing understanding that data should be treated as a critical component, similar to software code, with a focus on its structure and quality to build reliable AI models.
While these developments are promising, it's important to understand that a focus on quality doesn't negate the need for diverse and representative data. The potential for bias and inaccurate results still exists, emphasizing the importance of careful data curation and ongoing model evaluation. Overall, the reduction in training data requirements is a positive development, highlighting a potentially more efficient and accessible path for organizations to adopt and integrate AI into their operations. This is likely to reshape how enterprise AI is implemented, prioritizing real-time learning and adaptive systems.
It's fascinating to see that machine learning models are becoming more efficient, requiring 40% less training data while maintaining their performance levels. This is a notable shift from the past where vast quantities of data were considered essential for achieving acceptable results. This reduction likely stems from advancements in model architectures and algorithms, allowing them to learn effectively from smaller datasets.
The role of transfer learning is also likely playing a key part. By adapting knowledge from one task to another, models can be fine-tuned with less data, which improves their overall flexibility. This trend challenges the traditional assumption that more data always leads to better results, indicating a potential shift towards emphasizing data quality over quantity.
This efficiency gain is a major benefit for businesses. Reducing data needs translates to significant savings in resources related to data collection, storage, and processing. For example, a company could potentially reduce its overall AI budget if it could leverage a model requiring less data. This is especially relevant considering the growing concerns about data privacy and compliance – needing less data helps minimize risks in these areas.
However, there are still challenges. While the reduced data requirements are positive, the complexities involved in setting up and managing these efficient training processes remain. Engineers are still tasked with ensuring these models continue to deliver strong performance despite the smaller data sets used for their training.
Furthermore, the emphasis on smaller, high-quality datasets highlights the need to invest more in data curation and labeling. While it might cost less to train, companies still need to ensure the quality of the data they do collect is sufficient for optimal results.
The implications for user experience are also quite interesting. The reduced need for large datasets could potentially lead to faster development cycles for AI solutions. This could translate to quicker deployment of applications and quicker response times, leading to improvements in customer interactions. It's important to remember that, despite these improvements, the complexity of AI implementation still presents challenges and it remains to be seen how these efficiencies translate into broader, real-world applications.
Enterprise AI Evolution Comparing NLP Performance Metrics Between Modern Chatbots and IVR Systems in 2024 - Language Support Grows to 95 Languages in Modern Systems vs 12 in Traditional IVR
In the realm of enterprise AI, we're witnessing a significant shift in language capabilities. Modern systems, powered by advanced NLP techniques, now boast support for up to 95 languages, a far cry from the limited 12 languages handled by older IVR systems. This expansion reflects a broader trend towards inclusive AI, allowing companies to connect with a wider customer base across diverse linguistic communities. Furthermore, these modern systems often exhibit higher resolution rates, suggesting a more efficient and satisfying user experience.
However, this expanded language support isn't without its complexities. The sheer variety of languages and dialects presents significant challenges in ensuring that the AI accurately interprets user requests and provides appropriate responses. Maintaining high levels of accuracy and consistency across such a wide range of linguistic variations can be a formidable task, a problem that IVRs traditionally struggled with due to their rigid, pre-programmed nature. As organizations adopt these new technologies, balancing the desire for diverse language options with consistent, high-quality performance will be critical for success. The future of how enterprises interact with their customers will be shaped by the decisions they make regarding language support and AI performance in this evolving landscape.
In the realm of enterprise AI, the evolution of language support in IVR systems is a striking example of the advancements we're witnessing. Traditional IVR systems, largely confined to a set of 12 languages, appear quite limited in comparison to modern systems, which now offer support for 95 languages. This substantial increase reflects not only a greater capability but also a shift in focus toward a globalized marketplace, where businesses are striving to reach customers in their native tongues. This opens the door for enterprises to cater to diverse populations without the overhead of managing separate localized systems.
Modern chatbots are not simply limited to a broader spectrum of languages; they also feature multimodal interactions. This contrasts starkly with the sole reliance on voice interactions found in traditional IVR systems. These modern chatbots allow for user input via text, voice, and potentially even visual cues, fostering richer and more adaptable communication channels.
The shift towards multi-lingual chatbots has a direct impact on user satisfaction. Research indicates that a large portion of customers express greater satisfaction when they are able to interact with a system in their preferred language. This makes intuitive sense and has direct ramifications for business; in a competitive landscape, user satisfaction is linked to retention and brand loyalty, areas where IVR systems often fall short.
Moreover, modern chatbots with advanced NLP capabilities significantly outperform IVR systems in terms of language accuracy. The rigid, predefined phrases common in IVR often lead to misinterpretations, resulting in user frustration. In contrast, modern chatbots learn from context and adapt their understanding, substantially decreasing the incidence of errors.
The potential for real-time language translation emerges as a unique advantage of the expanding language support found in modern systems. This capability, absent in traditional IVR, could drastically change how enterprises engage with global teams and customer bases. Imagine seamless cross-lingual communication across departments or international offices, facilitated by an AI-driven translation feature. This holds the potential to be a significant productivity booster and enabler of collaboration in the future.
When examining trends in language usage, we observe a growing demand for regional and minority languages, which traditional systems largely failed to adequately address. Enterprises that recognize this emerging trend can differentiate themselves in local markets by fostering a sense of inclusivity and creating positive brand perceptions.
The increased language support facilitated by modern chatbots introduces a new dynamic to the competitive landscape. It's becoming increasingly easier for businesses to venture into new global markets. This will likely lead to increased competition across industries, pushing organizations to refine their customer service and strive for operational excellence to maintain a competitive edge in a market where expectations are continually rising.
The expanded language support in these advanced systems also reinforces the growing trend toward creating accessible technologies. It enhances the ability for non-native speakers and those with varying levels of language proficiency to access services and information more easily, moving beyond the inherent language barriers frequently encountered with traditional IVR systems.
The implementation of machine learning within these modern systems allows them to adapt to linguistic changes dynamically over time. This is a fundamental divergence from the fixed nature of IVR systems, which necessitate significant updates to accommodate even minor shifts in linguistic patterns. This dynamic learning component is a testament to the adaptability of AI in dealing with complex and evolving communication dynamics.
Ultimately, supporting multiple languages via modern chatbots has a substantial positive effect on economic efficiency for enterprises. Minimizing the need for building and maintaining separate localized systems streamlines operations and allows for more strategic allocation of resources. This stands in contrast to the ongoing maintenance requirements and potential for increased costs associated with traditional IVR updates.
In essence, the language support revolution in AI-powered IVR and chatbot systems signifies a dramatic change in how enterprises engage with customers and global teams. The increasing importance of understanding diverse languages and catering to individual user needs in this ever-evolving AI landscape is a constant push towards more seamless, equitable, and satisfying experiences for everyone.
Enterprise AI Evolution Comparing NLP Performance Metrics Between Modern Chatbots and IVR Systems in 2024 - Cost Per Interaction Decreases 73% Through Modern NLP Implementation vs Legacy Systems
The implementation of modern natural language processing (NLP) has led to a substantial 73% decrease in the cost per customer interaction, compared to legacy systems like traditional IVR. This significant reduction isn't just about saving money; it's also about improving the customer experience. Faster response times and more accurate understanding of user requests contribute to higher satisfaction and potentially greater customer loyalty. Businesses are increasingly realizing the cost savings possible in their customer service operations by adopting these advanced chatbot technologies, demonstrating a growing trend toward AI-driven efficiency gains. However, the advanced capabilities of these new systems often require complex and costly infrastructure. Businesses need to carefully consider the tradeoffs when making decisions regarding AI adoption. The decrease in cost per interaction through modern NLP highlights a significant change in how companies interact with their customers in this AI-driven era, marking a distinct step forward in the evolution of enterprise AI.
The shift towards modern NLP in enterprise AI has resulted in a notable 73% reduction in the cost per interaction, a significant improvement over legacy systems. This cost decrease primarily stems from the ability of modern chatbots to handle a high volume of customer interactions autonomously, reducing the reliance on human agents for routine inquiries. While IVR systems were designed to handle basic interactions, their limited capabilities often lead to more complex conversations being escalated to human agents, significantly increasing costs.
The scalability of modern NLP systems is another key contributor to cost savings. These systems can effortlessly handle a massive surge in interactions, unlike legacy IVR systems, which often become overloaded and lead to customer frustration and potentially lost business. This scalability is achieved through efficient resource utilization and the ability of AI models to adapt and respond to a variety of situations quickly.
Moreover, the integration of machine learning in modern chatbots has led to a reduction in human operator fatigue. Chatbots seamlessly take over repetitive tasks, freeing up human agents to focus on more complex issues that demand a nuanced human touch. This not only enhances customer satisfaction but also increases the overall efficiency of customer service operations.
Interestingly, the reduction in training data requirements for modern NLP has had a knock-on effect on costs. Companies can now quickly adapt these systems to evolving customer needs, a significant benefit compared to legacy IVR systems, which require extensive reprogramming and can be prone to error during those updates. This agility is crucial in a dynamic marketplace where customer expectations are constantly changing.
In addition, the continuous learning capabilities embedded in modern NLP models contribute to cost optimization. These systems, built on machine learning, are able to refine their understanding of customer queries over time, eliminating the need for significant retraining or knowledge updates that older, static systems required.
However, it's important to note that the transition to modern NLP systems is not without its own challenges. The reliance on complex AI models means that organizations need to invest in appropriate infrastructure, which can have significant upfront costs. Balancing these upfront costs with the potential for long-term cost savings will be a crucial consideration for enterprises making this transition.
Furthermore, while the improvement in error rates and user experience through context-aware NLP is significant, it is important to note that these systems are still under development. The ability to truly understand complex language nuances remains a challenge, and organizations need to be mindful of potential limitations as they integrate these systems into their operations.
The rise of AI-powered chatbots has brought about a paradigm shift in customer interaction. The potential for these technologies to transform businesses is substantial, especially as they continue to evolve and gain a better understanding of complex language and intent. Organizations that adopt these systems need to take a measured and strategic approach, carefully considering the potential benefits and challenges as they incorporate modern NLP into their operations.
Ultimately, the transition to modern NLP technologies holds great promise for reducing costs and improving customer experience. As the technology continues to mature, we can anticipate even more significant cost savings and improvements in efficiency across various sectors.
More Posts from aitranslations.io: