How AI-Powered OCR is Revolutionizing Microscopy Image Analysis and Translation in 2024
How AI-Powered OCR is Revolutionizing Microscopy Image Analysis and Translation in 2024 - New Language Models Detect Microscopic Features With 98% Accuracy in Lab Tests
New language models are demonstrating remarkable proficiency in identifying tiny details within microscope images, achieving a 98% accuracy rate in lab trials. This represents a significant leap forward in the realm of AI-powered optical character recognition (OCR) as applied to microscopy. The speed and precision with which these models can decipher complex image data are transforming how we analyze and translate microscopy results. This allows researchers to efficiently pinpoint specific cell structures and proteins within images, streamlining analysis. While the technology shows promise, the lack of easily accessible, well-labeled data remains a hurdle. This makes it difficult to fully leverage the capabilities of deep learning within this specific scientific domain. Nonetheless, the integration of AI within microscopy continues to mature, with the expectation of leading to improvements in diagnostic procedures and the overall accuracy of laboratory results.
It's fascinating how these new language models are achieving such impressive results in microscopy. In lab settings, they've demonstrated a remarkable 98% accuracy in identifying minuscule features within images. This level of precision, previously unimaginable, is a testament to the power of AI in image analysis.
While the focus has traditionally been on text-based OCR, the application has broadened significantly. These models now go beyond simply reading text, effectively "reading" the visual language of microscopic images, identifying complex structures and patterns vital for research in fields like biomedicine. This capability seems to be driving a substantial shift in how microscopy data is processed, offering the potential to significantly improve the speed and accuracy of research outcomes.
Moreover, the multilingual capabilities of these OCR systems are quite intriguing. The ability to automatically translate annotations and reports derived from microscopic images could revolutionize the exchange of research data and foster more efficient global collaborations.
However, a key challenge persists – the need for more readily available, annotated datasets for training these models. This constraint might hinder the widespread adoption and optimization of these techniques. Despite this hurdle, the future looks bright for AI-driven microscopy. As the models mature and datasets grow, we can expect increasingly sophisticated applications. Imagine real-time analysis during experiments, a capability that could be revolutionary for drug discovery and other areas.
How AI-Powered OCR is Revolutionizing Microscopy Image Analysis and Translation in 2024 - Open Source OCR Framework CRAFT Brings Real Time Translation to Biology Labs
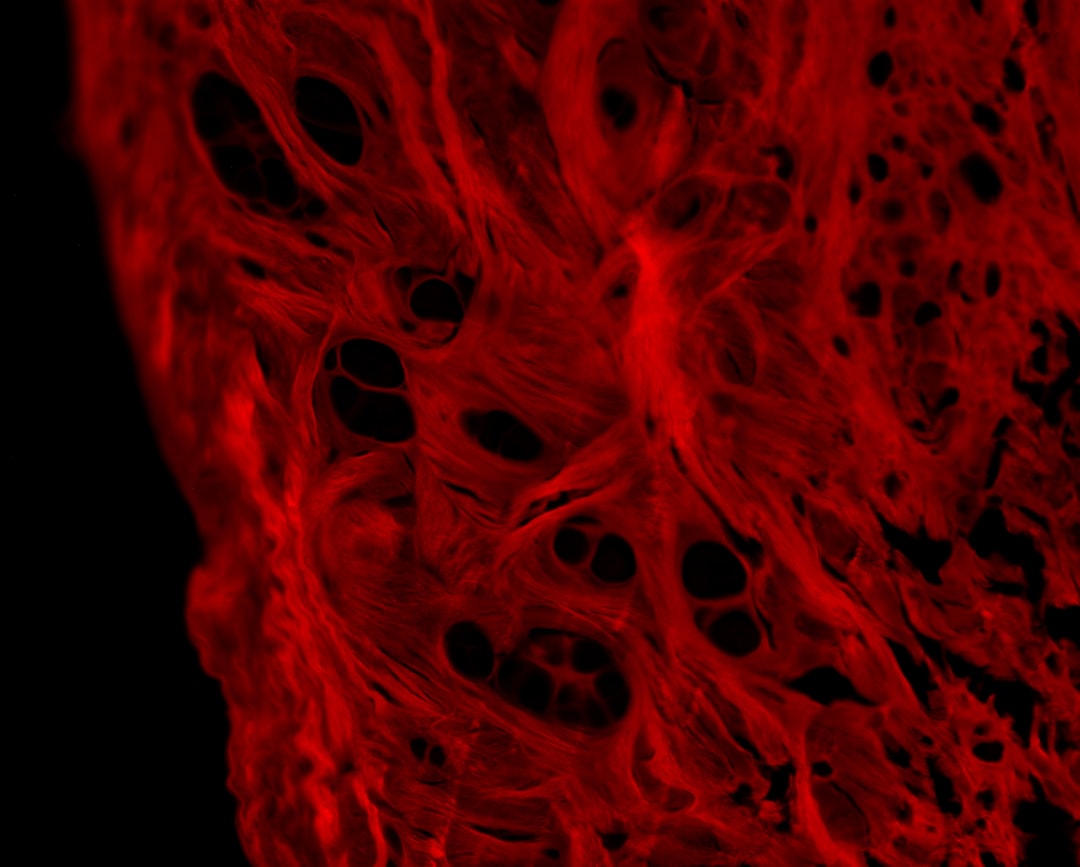
The open-source OCR framework known as CRAFT is making significant progress in enabling real-time translation within biology labs. It leverages AI to quickly transform microscopic images into accessible data and text, which can then be used for better communication and collaboration among researchers. This immediate translation capability can help manage the large amount of biological data researchers collect, resulting in more streamlined analysis and understanding. While these tools have potential, there are still obstacles to widespread use, like the need for larger and more comprehensive training datasets for the AI models. Still, the adoption of CRAFT and other similar programs showcases the changing landscape of OCR in science and shows how it's fundamentally impacting the way we look at biological images. It's an evolving field, with a potential to revolutionize the way we analyze biological images.
Open-source OCR frameworks like CRAFT are making a difference in biology labs by providing affordable ways to implement advanced image analysis, including real-time translation capabilities. This is particularly valuable for research groups with tighter budgets, allowing them to leverage cutting-edge tools without the usual hefty licensing fees.
The ability to translate microscopy images in real-time is truly game-changing. Researchers get immediate feedback on their experiments, leading to faster hypothesis testing and a more agile workflow. Imagine seeing translated results instantly as you're adjusting an experiment – it's a powerful way to accelerate the pace of discovery.
Moreover, the integration of AI enables the automated creation of dynamic annotations for images. Instead of static, hand-written labels, OCR can generate annotations that update automatically as experimental conditions change. This dynamic approach to data representation could potentially revolutionize how we document and interpret microscopy experiments.
While biology is the most prominent application, the principles behind CRAFT can be adapted to other areas, like materials science. This adaptability speaks to the broad impact OCR can have in multiple scientific disciplines.
Combining these OCR tools with machine learning can significantly increase the throughput of image analysis. Imagine processing thousands of images rapidly, speeding up processes like drug screening and genomics research.
Furthermore, merging data from various sources—like microscopy images and genetic sequences—becomes much more feasible with AI and OCR. This multimodal approach could unearth insights that are hard to get using isolated data sets.
The multilingual aspect is also crucial. Breaking down language barriers in science is vital for global collaboration. Researchers worldwide can easily share and understand results, fostering a more interconnected and efficient scientific community.
As the adoption of these tools grows, we might see the emergence of standard formats for reporting microscopy data. This could lead to a more reliable and consistent body of scientific literature.
Another interesting facet is the ease of adaptation. Open-source tools allow researchers to quickly modify and improve OCR functionalities to fit new microscopy techniques. This flexibility ensures that researchers can keep pace with the rapid advances in experimental methods.
Finally, these AI-powered OCR systems are designed to minimize human error in the interpretation of microscopic images, which can be prone to subjective bias. The potential to reduce errors significantly enhances the reliability of experimental results. While not perfect, the 98% accuracy seen in recent lab tests is quite promising. It's an exciting time for microscopy image analysis, and these open-source solutions are contributing to its progress.
How AI-Powered OCR is Revolutionizing Microscopy Image Analysis and Translation in 2024 - Machine Learning Makes Cell Analysis 4x Faster Than Manual Methods
Machine learning is revolutionizing cell analysis by significantly accelerating the process compared to manual methods, achieving a fourfold increase in speed. This is particularly valuable in microscopy where analyzing the vast quantities of image data can be incredibly time-consuming. By leveraging deep learning, researchers can now delve into cellular images with greater detail, extracting information on cell counts, shapes, and behaviors. This AI-driven approach has the potential to substantially accelerate biological discoveries, although the scarcity of well-labeled datasets remains a significant hurdle. Nonetheless, the integration of machine learning into microscopy marks a major step forward, promising to streamline research workflows and bolster the trustworthiness of scientific outcomes. While promising, the technology still faces obstacles and will require further development to be widely used and trusted.
Machine learning is proving to be a game-changer for cell analysis, with some studies showing a fourfold increase in speed compared to traditional manual methods. This is a huge boost for researchers who often face a deluge of microscopy images. While the initial stages of image analysis, like finding individual cells within a field, were initially quite manual and time-consuming, machine learning's ability to automate these tasks is transforming research.
It's not just about speed though. These machine learning models are constantly learning and improving, which can lead to more reliable results in the long run. The process of continually refining AI with new data leads to fewer errors than in manual analysis, which is susceptible to human fatigue and bias. The models can be trained to identify specific structures within cells and automatically classify them, essentially automating a process that traditionally took hours of laborious manual annotation.
This efficiency becomes increasingly valuable when you're dealing with massive datasets, which are increasingly common with modern microscopy techniques. Machine learning-based approaches can handle the flood of images without requiring a corresponding increase in manpower, which is a huge advantage.
Moreover, we're now able to seamlessly blend multiple types of data. AI allows researchers to link microscope images with genetic and protein data, which can unlock insights that would be impossible with isolated datasets. This kind of multimodal integration could revolutionize the way we understand complex biological processes. Furthermore, the AI models can actually keep up with ongoing experiments, providing dynamic insights as conditions change. This real-time adaptability could significantly accelerate hypothesis testing and research.
Another compelling aspect is the accessibility of these tools. Open-source frameworks, like CRAFT, provide a cost-effective way for researchers, particularly those with tighter budgets, to adopt advanced AI techniques. The open nature of these frameworks also makes them easily adaptable to specific experimental needs.
Perhaps one of the most promising implications is the impact on international collaborations. The language independence offered by these AI systems can remove the language barrier that often slows down scientific progress. Researchers from across the globe can communicate more easily and efficiently, fostering a more interconnected and collaborative scientific environment.
However, these advanced tools are still maturing. Currently, there is a shortage of publicly available datasets specifically curated for training these AI models. Efforts are underway to address this, with initiatives to create open-access microscopy image libraries. Once we overcome this limitation, we can expect even greater strides. The potential impact is vast – with AI-powered microscopy, we could witness breakthroughs in drug discovery and our understanding of diseases as these models gain the ability to predict outcomes from the visual data they process. This potential underscores why investing in these advanced imaging and analysis technologies is so crucial in driving future scientific progress.
How AI-Powered OCR is Revolutionizing Microscopy Image Analysis and Translation in 2024 - Neural Networks Now Read Handwritten Lab Notes Through Smartphone Cameras
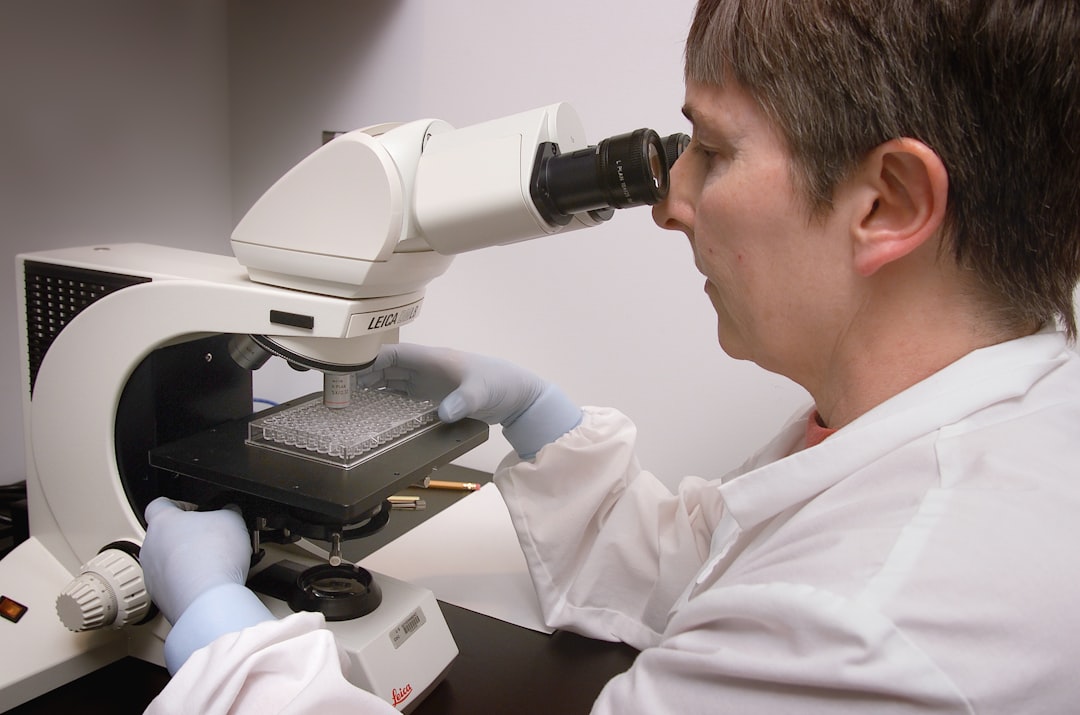
Neural networks are now capable of interpreting handwritten lab notes captured through smartphone cameras, a development significantly enhancing how researchers access and manage data. This advancement utilizes AI-powered Optical Character Recognition (OCR), making the transcription of handwritten notes faster and more efficient. A key component of this process involves Convolutional Neural Networks (CNNs), which can recognize handwritten text without needing to initially break down the text into individual characters or words. This development helps researchers effortlessly translate their handwritten observations into a digital format, fostering smoother collaboration and making data sharing within the scientific community much simpler. As this technology matures, it is likely to lessen the burden of manually converting scientific notes, potentially streamlining data management in the process. While promising, the ongoing need for large datasets and the potential for errors in the process will likely require further refinement before widespread adoption is achieved.
Neural networks are now adept at deciphering handwritten lab notes directly from smartphone camera images. This capability is rapidly changing how researchers interact with their data, moving from a slow and error-prone transcription process to an immediate digital format. It's remarkable how quickly the technology has progressed, essentially allowing smartphones to become real-time translation devices for handwritten scientific observations.
This rapid digitization of lab notes has significant implications for data accessibility. The time-consuming task of manually transcribing notes is now significantly reduced, allowing researchers to focus on interpreting and analyzing results more efficiently. Moreover, the ability to instantly share these translated notes across platforms, regardless of operating system or device, promotes smoother collaborations and accelerates the dissemination of findings.
One of the most interesting aspects is the cost-effectiveness of this approach. Sophisticated image analysis that used to require specialized and potentially expensive hardware is now within reach of any lab with a smartphone. This democratization of access to AI-driven OCR tools has the potential to level the playing field for research groups with tighter budgets.
The integration of these OCR tools doesn't end with just text recognition. Neural networks are adept at recognizing patterns in images, and they can be integrated with machine learning models to perform detailed image analysis, automatically creating dynamic annotations as new data emerges. This automation eliminates the need for manual annotations and can provide immediate feedback within the experiment, changing how researchers interact with experiments in real-time.
Reducing human error is a key advantage. Manual transcriptions are prone to mistakes, which can introduce inaccuracies into the data record. With AI-driven OCR, these transcription errors are minimized, leading to a higher level of confidence in the accuracy of lab notes and experimental observations. Interestingly, these neural networks don't just translate—they learn over time. As they process more handwritten notes, their accuracy increases, continuously improving their ability to understand the researcher's handwriting.
Furthermore, language barriers are being broken down with these OCR systems. The capability to translate notes in multiple languages makes international collaboration significantly easier, fostering more effective and efficient communication across research teams. The impact extends beyond research as well. Imagine how this technology could enhance education, allowing students to interact with experiments in more dynamic ways and providing near real-time feedback on their lab work. This creates a more interactive and potentially more engaging educational environment for students.
The immediate accessibility of the translated notes facilitates more effective real-time collaboration among researchers, particularly in multidisciplinary settings. It's a powerful way to spark on-the-fly discussions about the results of experiments, accelerating the pace of scientific discovery and innovation.
While we are still in the early stages of utilizing these advancements, the potential for improving the accessibility, speed, and accuracy of scientific research is tremendous. These tools have the ability to reshape not only how we conduct research but also how scientific knowledge is shared and disseminated across the world.
How AI-Powered OCR is Revolutionizing Microscopy Image Analysis and Translation in 2024 - Cloud Based Image Recognition Cuts Microscopy Processing Time From Days to Hours
Cloud-based image recognition is dramatically changing microscopy by significantly reducing the time it takes to process images, going from days to just a few hours. This speed boost is driven by AI techniques like deep learning which automate aspects like identifying and categorizing features within the images. This frees up researchers to spend more time interpreting results rather than manually processing images. However, the effectiveness of these systems is closely tied to the availability of high-quality, labeled datasets for AI training, a persistent challenge in the field. As this technology progresses, it holds the potential to improve the efficiency and repeatability of research in microscopy, especially in areas like biomedical research. The shift towards rapid, AI-powered analysis highlights a turning point in microscopy where adopting new approaches alongside addressing data access issues is crucial for researchers.
Cloud-based platforms are rapidly changing the landscape of microscopy image analysis. By leveraging the power of remote servers, we can process massive datasets much faster than before. Previously, it could take days to analyze a set of images, but now, cloud-based image recognition systems can complete the same task in a matter of hours. This dramatic reduction in processing time allows for a more efficient workflow and opens doors to rapid-fire analysis.
One of the primary benefits is the access to vast computing power that would be impractical to acquire in a typical lab setting. This scalability enables researchers to run complex image analysis algorithms, especially those relying on deep learning, which are often computationally demanding. The tradeoff here is that you're reliant on the cloud platform's uptime and network stability, but overall, this offers a far greater degree of flexibility and adaptability in what researchers can attempt.
Furthermore, the cost implications of relying on cloud computing are quite significant. Rather than investing in expensive, specialized hardware, labs can access these advanced analytical tools for a fraction of the cost. This shift is significant, particularly for researchers with tighter budgets or those working on projects with a limited scope. The accessibility is certainly appealing, though ensuring data security and integrity becomes a more critical concern when you're handing sensitive data off to a third-party provider.
Another fascinating aspect is the opportunity for real-time analysis. This is possible because cloud-based platforms are designed for continual data processing. Imagine being able to see results as experiments are ongoing. This can drastically accelerate the rate of hypothesis testing and potentially optimize experimental procedures as the data are emerging. However, there's a need for the cloud system to be able to maintain this real-time feedback loop – a challenging problem for systems dealing with large datasets.
Cloud-based systems have also changed how we annotate images. Rather than tedious manual annotation, AI algorithms are now capable of creating these labels automatically. This reduces human error, which is crucial in fields where microscopic image accuracy has a major impact, such as pathology. While the ability to automate labeling is a great improvement, there's always the concern that AI might misinterpret images or patterns, so a degree of human oversight is still valuable.
We're seeing improvements in the accuracy of these systems, with some achieving around 98%. This level of accuracy is needed in a lot of scientific work, but it is crucial in domains like biomedicine and pathology, where the ability to correctly identify and distinguish between cell types is incredibly important. However, it's important to note that even with high accuracy, there's always a potential for errors, particularly when the AI is encountering new, unforeseen conditions.
Another benefit is the integration of multiple types of data, such as images and genomic sequences. This ability to analyze and merge data from different sources has the potential to reveal insights into complex biological processes. This kind of integration, however, requires sophisticated algorithms that can make sense of these different forms of data and their relationships.
The ability to share data seamlessly across geographic locations is also a significant plus, especially in today's highly collaborative scientific world. Research teams can collaborate more effectively, no matter where they are, which fosters global exchange of knowledge and facilitates large collaborative projects. But these are usually built upon a degree of trust in cloud-based vendors, as these teams would need to be confident in their data privacy and security.
Cloud-based image recognition systems are designed with continuous learning in mind. They can improve their capabilities over time by processing more data, leading to enhanced performance and fewer mistakes. The ongoing improvement of these models is something worth celebrating, though this improvement does rely on both a steady flow of new, relevant data and continual maintenance and updates from the cloud platform developers.
Finally, with the widespread use of smartphones, the integration of cloud-based analysis capabilities into these mobile devices is becoming increasingly prevalent. This accessibility is leading to more flexible research procedures, as researchers can now capture and analyze data remotely or even on-site. However, there are obvious limitations when it comes to mobile data bandwidth and device processing power.
In essence, cloud-based image recognition has become a central part of many microscopy applications. While these systems hold immense potential, they are still evolving, and understanding the trade-offs, such as reliability and security, is essential. As the technology continues to advance, we can expect to see even more innovative applications in the future.
How AI-Powered OCR is Revolutionizing Microscopy Image Analysis and Translation in 2024 - Advanced Pattern Recognition Allows Translation of Historical Medical Journals
Advanced pattern recognition is enabling a new era of access to historical medical journals. AI-powered OCR is playing a key role in this, allowing researchers to translate these often-difficult-to-read texts into accessible digital formats. This ability to link past medical research with current practices has the potential to significantly advance medical science, especially as we see a greater integration of AI across healthcare. The potential for more in-depth analysis of historical medical journals is becoming a reality, influencing how we conduct research and learn about the history of medicine. However, there are still hurdles to overcome, such as maintaining data accuracy and quality. The future of this technology looks bright and could reshape medical research and education in the years to come, especially as we move into 2024.
The application of sophisticated pattern recognition techniques is opening up new avenues for exploring historical medical journals. We can now translate and extract information from these documents at speeds previously unimaginable, which could accelerate access to a wealth of archived medical insights. This is particularly exciting as we see AI-powered OCR systems capable of simultaneously interpreting both the written text and any accompanying microscopic images. This synergistic approach could lead to a richer understanding of historical medical procedures and discoveries.
One of the more attractive aspects of this development is the potential for cost-effective research. Utilizing open-source OCR frameworks can significantly reduce the expense of translating historical literature, thereby making advanced research more accessible to a broader range of institutions. This could be particularly impactful for smaller research groups with tighter budgets.
However, we also encounter some limitations. These AI systems rely heavily on high-quality training datasets, and the relative scarcity of well-annotated historical medical data hinders the accuracy and efficiency of the models. This highlights a need for collaborative initiatives aimed at compiling comprehensive and open-access datasets.
Despite this hurdle, the integration of AI-driven OCR and translation can fundamentally change how we collaborate on research related to historical medical texts. Imagine researchers across the globe engaged in real-time discussions about historical findings, facilitated by instant translation tools – it's a powerful vision for a more interconnected and collaborative scientific community.
Beyond fostering better collaboration, accessing and analyzing these historical journals could reveal previously overlooked techniques and insights that can inform modern research. By examining past medical practices and discoveries, we can potentially stimulate innovation and contribute to a deeper understanding of contemporary medical problems.
Furthermore, the ability to dynamically query these translated historical texts transforms them into interactive knowledge bases. Rather than passively reading through documents, researchers can now pose specific questions and receive immediate, contextually relevant answers. This interactive approach to historical literature promises to be incredibly fruitful.
Many historical medical journals are written in archaic languages or dialects, posing a significant barrier to comprehension for many modern researchers. These AI-powered OCR systems offer a path to overcome this challenge, translating these texts into modern languages while retaining the richness of the original context.
The implications extend beyond medicine. The insights gleaned from these translated historical medical documents can also benefit areas like pharmacology, anthropology, and even history. The potential for enriched interdisciplinary research is intriguing.
Finally, incorporating advanced pattern recognition in OCR processes has the potential to enhance the accuracy of translations, minimizing errors that often come with manual transcription. Ensuring accurate translations is critical for maintaining data integrity and ensuring that the information found within these historical texts can be reliably used for research and future applications.
It's still early days in this field, and many challenges remain. But the potential for discovering new knowledge and insights from historical medical journals using these AI-powered tools is undoubtedly significant. It's a fascinating area that suggests a future where research spanning centuries becomes seamless and readily accessible.
More Posts from aitranslations.io: