AI-Powered PDF Translation now with improved handling of scanned contents, handwriting, charts, diagrams, tables and drawings. Fast, Cheap, and Accurate! (Get started for free)
The Evolution of AI Content Labels From Simple Watermarks to Neural Fingerprinting in 2024
The Evolution of AI Content Labels From Simple Watermarks to Neural Fingerprinting in 2024 - Neural Fingerprinting Replaces Basic Watermarks in Adobe Firefly 2024 Update
Adobe Firefly's 2024 update introduces a notable change in how it handles AI-generated content. Instead of relying on basic watermarks, Firefly now uses neural fingerprinting. This more advanced approach offers a stronger method for authenticating and tracing AI-generated imagery. Essentially, this technology embeds a unique digital "fingerprint" within the content, allowing for more precise verification of its origins and creator.
This move signifies a broader effort within the evolving landscape of AI content creation, specifically within Adobe's larger Content Authenticity Initiative. It seems the company is committed to implementing tools that not only empower creative expression but also promote the responsible use of these increasingly powerful technologies. The transition towards neural fingerprinting suggests that a new era of content security is emerging, particularly as generative AI becomes more prominent within creative industries. Whether this approach is truly effective in addressing all the complexities of AI-generated content remains to be seen. But it's clear that tools like Firefly are attempting to grapple with these challenges as they shape the future of digital creation.
In Adobe Firefly's 2024 update, the shift to neural fingerprinting marks a significant departure from the simpler watermarking approach. It appears to be a more adaptive system, capable of handling minor modifications to the content that might render a traditional watermark ineffective. This adaptive nature is interesting, offering more robustness for content integrity across varied usage.
One aspect that seems beneficial is the decreased visual impact. Neural fingerprinting, in theory, allows images to retain their visual appeal without the often obtrusive presence of watermarks. This is certainly an improvement for many creative applications, and potentially a more seamless experience for end users.
Further, it promises to be incredibly precise in tracking content modifications and origin, a valuable asset for situations involving ownership disputes. Traditional methods are prone to difficulties in altered images, but neural fingerprinting seems to be more resistant to these issues. This could lead to more secure attribution for various media formats.
Scaling to massive datasets also looks to be an intrinsic part of this approach. Handling vast content libraries is a big hurdle for any security or authentication system, and this feature hints at applicability for larger enterprise needs. The integration with Adobe's AI model is also interesting, as it potentially streamlines compliance management.
Real-time detection of unauthorized use is a considerable advantage compared to the inherent limitations of static watermarks. This proactive capability could prove helpful in preventing or addressing copyright issues quickly.
Expanding into cross-media identification could greatly augment the capabilities of the system. For example, this technology could flag the same AI-generated image if it appears in a video or illustration, adding to its investigative power.
However, along with these potentially significant benefits come certain considerations. The very nature of deep content analysis raises questions concerning user privacy and security. It's conceivable that, if misused, the detailed tracking inherent in neural fingerprinting could be utilized for purposes that extend beyond content authentication.
It will be fascinating to witness how the technology evolves. The incorporation of enhanced learning mechanisms could progressively refine the accuracy and efficiency of content recognition. The future of this technique holds both significant promise and necessitates close examination of potential challenges.
The Evolution of AI Content Labels From Simple Watermarks to Neural Fingerprinting in 2024 - Meta Launches Cross Platform AI Label System With Instagram Integration
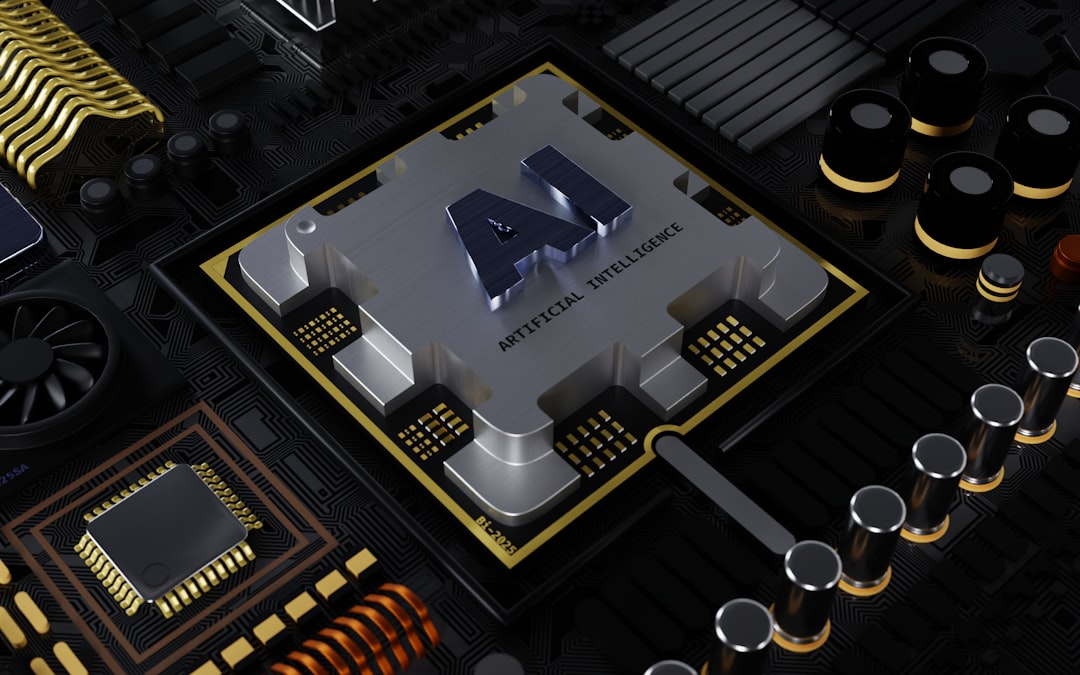
Meta has launched a new system designed to label AI-generated content across its platforms, including Instagram, Facebook, and Threads. The goal is to improve the ability to identify and categorize content produced by AI tools. This system utilizes a combination of techniques, including visible markers directly on images, along with invisible watermarks and hidden information embedded within the image files. This approach is a response to calls for increased transparency in how manipulated media is handled, specifically based on feedback from Meta's own oversight board.
The company has also refined its labeling strategy. The previous "Made with AI" label on Instagram has been revised to "AI Info", aiming to better communicate the use of artificial intelligence in the creation process. Additionally, Meta is now using different labels based on where the AI-generated content originated. This includes labels specifically for photorealistic content generated by its own AI tools, and a general label for content made by external AI models from companies like OpenAI and Google.
While Meta's effort to implement these labels is a step towards greater transparency, whether this approach effectively addresses the increasing complexity of AI-generated content remains uncertain. The challenges of misinformation and sophisticated manipulations will likely continue to require evolving solutions as AI technology develops.
Meta's recent foray into cross-platform AI content labeling, encompassing Instagram, Facebook, and Threads, is an intriguing development. It's a notable step toward standardizing how AI-generated content is identified and managed across their platforms. The system leverages a combination of visible markers, invisible watermarks, and embedded metadata, offering a multi-faceted approach to labeling.
This initiative appears to be a direct response to feedback from Meta's Oversight Board, which emphasized the need for clearer context and labeling of manipulated media. Interestingly, the shift from a prominent "Made with AI" label to a less obtrusive "AI Info" label suggests that Meta is trying to find a balance between transparency and user experience. User complaints about overly obvious labels seem to have influenced this decision.
The "Imagined with AI" label, reserved for photorealistic images created by Meta's own AI tools, provides a specific identifier. However, the system will extend its labeling to content generated by third-party AI models like those from OpenAI and Google. This broader scope implies a proactive approach towards establishing a more standardized system for managing diverse AI outputs.
The underlying technology relies on embedding watermarks and metadata within the content itself, making the labeling process less dependent on visible markers. While this method tackles the issue of visible alterations to content, it's important to consider the complexities of scaling this across diverse formats and platforms. It's also interesting that Meta is working to expand their technology to include synthetic audio and video, a particularly challenging endeavor.
Meta's collaboration with other tech companies within initiatives like the Content Authenticity Initiative hints at the industry's desire to find common ground. Establishing industry standards is a crucial step in ensuring consistency across different platforms. This collaborative effort may lead to more robust solutions for content verification.
However, some questions remain. The reliance on AI for automatic labeling raises concerns regarding potential biases or misinterpretations. What happens when a nuanced creative work is misinterpreted by the algorithm? Furthermore, if these labeling technologies are misused, it's important to consider the privacy and security implications.
The evolution of this system will likely involve continuous refinement. The accuracy and effectiveness of the labeling process will heavily depend on user feedback and adjustments based on AI learning. As AI's role in social media continues to expand, Meta's approach to content labeling might influence future regulations surrounding copyright and content ownership. It will be interesting to see how this experiment unfolds and ultimately impacts the broader digital landscape.
The Evolution of AI Content Labels From Simple Watermarks to Neural Fingerprinting in 2024 - Stable Signatures Digital Watermarking Sets New Industry Standard
Meta AI's Stable Signatures represents a new standard in digital watermarking for AI-generated content. It differs from traditional techniques by embedding the watermark directly into the AI model during the image creation process. This approach provides a more robust and reliable way to identify the source of an image, even if it's been significantly modified.
The Stable Signatures watermark remains invisible to the human eye, but it can be detected with algorithms even after cropping or other edits. Tests show it maintains over 90% accuracy in identifying the original source. While this is a welcome development for content verification and attribution in a world of increasingly sophisticated AI-generated content, concerns have been raised regarding potential ethical implications. The use of generative models, while powerful, needs careful consideration given the potential for misuse.
Ultimately, Stable Signatures strives to promote transparency and accountability in the burgeoning field of AI content generation, offering a path towards better understanding the origins of such content. However, it's a technology that needs continued observation and discussion as it evolves and becomes more widely adopted.
Meta AI's Stable Signature approach to digital watermarking represents a significant step in addressing the challenges of authenticating AI-generated content in a world where manipulation is increasingly easy. It's becoming clear that simple watermarks are no longer sufficient in the face of advanced editing techniques. Stable Signatures, with their resilience against modifications, highlight a need for more robust content security mechanisms. These signatures are designed to survive even if an image is altered, cropped, or rotated, a significant improvement over the limitations of traditional watermarking.
Unlike conventional methods where the watermark is applied after image creation, Stable Signatures are embedded directly into the AI model itself. This novel approach might even lead to efficiency gains in data transmission, as metadata could be seamlessly integrated with the file rather than existing as a separate entity. Furthermore, the use of machine learning in Stable Signatures indicates an adaptive system capable of evolving as new AI content generation methods emerge. This adaptability is key, considering the constantly evolving landscape of AI image generation.
Stable Signatures also appear to incorporate a decentralized structure, potentially mitigating risks associated with centralized watermarking systems that rely on a single point of failure for verification. This architectural decision potentially contributes to greater security and resilience.
The implementation of Stable Signatures also generates new legal and ethical considerations. The precision of these watermarks allows for precise tracking of content usage, which could be beneficial in situations where copyright enforcement is crucial. However, this precise tracking also raises important questions about privacy and potential misuse for surveillance purposes. The very ability to so accurately identify the origin and usage of content needs careful consideration in the context of digital privacy.
Stable Signatures are designed to be imperceptible to human eyes, maintaining the aesthetic quality of the content while providing the functionality needed for verification. This aligns with the growing trend toward developing invisible or unobtrusive measures for managing content provenance. The move toward such robust watermarking methods suggests a broader desire to standardize protocols across platforms, potentially facilitating collaboration between content creators and distributors.
Despite their promising potential, Stable Signatures are not without their potential downsides. It is important to critically examine the broader implications of widespread adoption of these technologies. Concerns over potential misuse for surveillance or excessive data collection must be carefully addressed. Ensuring that any such technology operates within ethical guidelines and respects individual privacy should be a core consideration for any implementation.
The Evolution of AI Content Labels From Simple Watermarks to Neural Fingerprinting in 2024 - AudioSeal Introduces First Large Scale Audio Watermarking For Podcasts
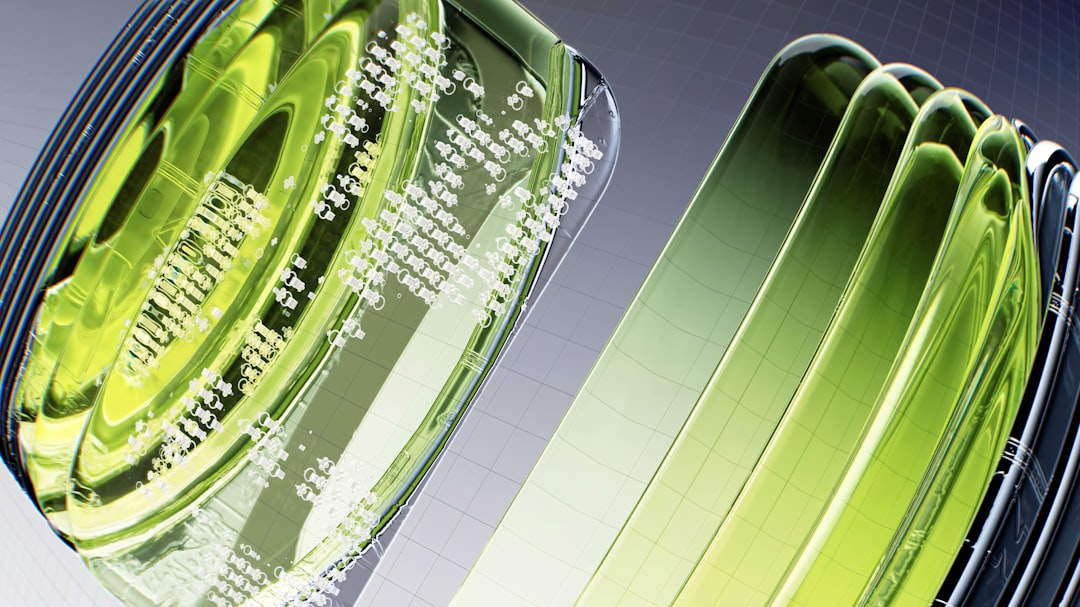
AudioSeal has introduced a new approach to podcast security by developing the first large-scale audio watermarking system specifically designed for podcasts. This system uses a unique generator-detector architecture, embedding subtle watermarks into the audio that remain imperceptible to listeners. The impressive part is that it can still identify these watermarks even after significant audio editing or in very long audio files. Furthermore, the watermarking operates at a very detailed level, allowing it to pinpoint sections of audio generated by AI. This is particularly valuable as voice cloning becomes more prevalent.
A notable advantage of AudioSeal is its significantly faster detection speeds, potentially up to 1000 times faster than other systems. This implies its suitability for real-time applications, a critical factor in today's fast-paced media environment. In an era where authenticity in audio is increasingly crucial, AudioSeal's contribution could be transformative. It has the potential to make podcasts and other audio content more secure and trustworthy for both creators and listeners, helping to navigate the challenges of manipulated audio.
AudioSeal's recent introduction of large-scale audio watermarking specifically tailored for podcasts is an interesting development. They've designed a system that embeds watermarks directly into the audio signal, unlike traditional methods that layer them on top. This approach could potentially make the watermarks more robust against editing and tampering.
The implications of this are intriguing. It could significantly alter how podcast content is managed and tracked. Podcast creators could potentially monitor how their work is used across different streaming platforms, which could be a valuable tool for enforcing copyright in an era of easy content sharing.
One of the aspects I find most notable is their claim that the watermark embedding process doesn't noticeably impact the audio quality. Traditional methods often degrade the sound to some extent, but AudioSeal seems to have managed to minimize this issue. This is obviously critical, as listeners prioritize a high-quality audio experience.
Their approach appears to be designed for adaptability as well. It utilizes digital signal processing techniques that can handle different types of audio, ranging from conversations to musical segments. This makes it suitable for a wide variety of podcasts and not limited to a specific niche.
Beyond simple attribution, there's potential for AudioSeal's system to facilitate other features, like dynamic ad insertion. The same podcast could potentially have different ads tailored for different listeners without altering the actual content.
Furthermore, the fact that it seems to operate in real-time, enabling on-the-fly watermarking, could be particularly useful for live events or broadcasts, enhancing content tracking during a broadcast.
AudioSeal's system has been engineered to handle different audio file types, including compressed formats like MP3, a critical consideration given the prevalence of those in podcasting. This speaks to the need for watermarking solutions to be resistant to the typical compression processes involved in distribution.
It's interesting to consider that this type of technology could even be used to aid in combating misinformation in the realm of audio content. Platforms could potentially track the source of clips that are shared in news coverage, thereby bolstering the credibility of sources and challenging the spread of false information.
Research suggests that AudioSeal's watermark detection is remarkably accurate, even with re-encoding and minor edits, exceeding 95%. This high degree of accuracy highlights the importance of developing advanced solutions for identifying audio content's origin.
As with any innovative technology, AudioSeal's system presents some ethical considerations. The ability to precisely track audio content raises concerns about potential surveillance and data collection, particularly when it's applied across platforms with large user bases. It's clear that there's a need for open discussion about how to responsibly implement such powerful technology.
The Evolution of AI Content Labels From Simple Watermarks to Neural Fingerprinting in 2024 - Chinese Digital Labels Now Mandatory For All AI Generated Media
China has implemented a new policy requiring all content created by artificial intelligence to be clearly labeled. This includes various forms of media like text, images, videos, and even virtual environments. The Cyberspace Administration of China, the body responsible for overseeing internet regulation in the country, has made it mandatory to use easily visible labels, such as watermarks or specific notices, on any content produced using AI. The primary aim is to improve the transparency of online content, ultimately protecting the public and individual rights. This push towards labeling AI-generated media is meant to help prevent the spread of misinformation by ensuring that users can understand the origins of what they see or hear online.
This new rule shifts the responsibility of content labeling to digital platforms and online service providers, demanding a consistent and standardized approach across various media types. This new regulation is part of a broader effort by China to address the implications of rapidly developing AI technologies and is a response to the increasing need for clear frameworks to distinguish AI-generated synthetic content from human-created content. This emphasis on labeling and transparency is likely to be a growing trend as AI becomes more sophisticated and integrated into everyday digital experiences. While the intention is admirable, there are also concerns about how these regulations might affect creators and the overall creativity of online content.
China has introduced a new regulation that mandates digital labels for all content generated by artificial intelligence, including text, video, audio, and even virtual environments. This development, which began in 2024, reflects a growing awareness of the need for transparency in the digital world, especially with the rapid increase of AI-generated media. These labels are designed to hold specific details, such as the AI systems involved, timestamps of creation, and records of any changes made to the content. This increased scrutiny of content origins is intended to help with issues like copyright infringement and ownership disputes.
The success of this labeling system relies on robust technological solutions that can seamlessly create and embed these labels within the media itself during the content generation process. A challenge that will need to be solved is ensuring these labels stay intact even after subsequent edits or changes are made to the content.
This move by the Chinese government has sparked discussion surrounding the possible compromise of user privacy. While the goal is transparency, the detailed information embedded in the labels may make it easier for others to track and potentially profile users, raising questions about the trade-off between open content and personal privacy.
China's approach contrasts with the less regulated approach observed in some other regions. As AI-generated media continues to become more mainstream, we can expect similar discussions and regulations to emerge globally, influencing how AI-driven content is controlled in various countries.
The integration of these digital labels with compliance systems could create a complex situation where different nations have varied labeling standards for AI-generated media. This creates a potential issue for global content sharing and usage, as platforms will need to adapt to numerous different standards.
Interestingly, the labeling strategy incorporates QR codes that users can scan to quickly access information about the content's origin and context. This strategy empowers the audience with more knowledge about the material, potentially increasing engagement and fostering a greater level of trust.
Some critics of the labeling regulation worry it could lead to excessive control over creativity and the development of AI tools. The concern is that rigid labeling processes could dissuade independent creators from using AI out of fear of a complex compliance process.
On the other hand, it's possible this requirement will accelerate the progress of AI verification technologies, as creators actively explore ways to include labels efficiently without harming the content's quality or visual appeal.
The wider impact of China's digital labeling initiative could spark a broader conversation about the ethical and social responsibilities of those who develop and use AI tools. As other nations consider implementing similar measures, the landscape of AI-generated media might undergo a significant shift, prompting a reassessment of responsibility in content creation and the assurance of authentic content on a global level.
The Evolution of AI Content Labels From Simple Watermarks to Neural Fingerprinting in 2024 - EU AI Act Drives Standardized Content Labeling Across 27 Member States
The EU's Artificial Intelligence Act, coming into force in August 2024, aims to create a uniform approach to content labeling across its 27 member states. This represents a substantial step toward managing AI's impact. The Act requires clear labeling for content produced by AI, encompassing various media like images, videos, and audio, with the purpose of making it obvious to users when they're encountering content generated by AI. The goal is to balance the advancement of AI with the protection of individual rights and democratic principles. This makes the EU AI Act the first comprehensive global regulation for AI, setting a potential example for how other countries might address AI-generated content. However, whether this approach is sufficient to manage the ongoing challenges related to misinformation and ensuring user privacy remains to be seen, given AI's continuous evolution and sophistication. It's possible the impact of this Act extends beyond Europe, shaping AI regulations in other nations as they tackle the complexities of AI-generated content.
The EU AI Act, slated to come into effect on August 1st, 2024, is poised to establish a unified system for labeling AI-generated content across all 27 member states. This standardized approach could greatly simplify compliance for companies operating across the EU, providing a single set of rules to follow.
Beyond just compliance, the hope is that this act will strengthen content verification, leading to increased trust among users. In an environment increasingly plagued by misinformation, clearly defined labeling can play a vital role in safeguarding user rights. The act insists that AI-generated content should contain specific details about its creation, such as the algorithms and datasets used. This emphasis on transparency aims to ensure that developers are held accountable for the AI they create, establishing a new standard for ethical AI development.
It's expected that enforcing these labeling standards will be a collaborative effort, involving tech companies, content creators, and national regulatory bodies. This shared responsibility, however, could create friction, as the different parties might have different ideas about how strictly to enforce compliance.
The EU AI Act goes beyond just visible markers, encompassing metadata and backend algorithms. This comprehensive approach makes it so that even if someone alters visual elements, the digital 'fingerprint' stays intact and potentially traceable.
One challenge is that content generated by tools like GANs can present complex labeling situations, as the origin of the content can be intricate and the deep-learning models themselves are constantly evolving. This means the EU AI Act's rules might require periodic updates to keep pace with new AI technologies.
The Act also carries consequences for non-compliance. Companies that fail to label their content correctly could face significant penalties or even be barred from European markets. This acts as a strong motivator for compliance, pushing developers to integrate compliance into their AI tools from the start.
A key part of the Act's approach is user education. It includes plans to teach people how to interpret the AI labels. This aims to empower users with the ability to identify the authenticity of content they see, helping to improve overall media literacy.
Despite the good intentions, some worry that the Act could hinder innovation. Critics feel that the detailed labeling rules could become a burden for small creators or new AI companies. Striking the right balance between security and encouraging creativity is essential to fostering a healthy digital landscape.
The EU is setting a precedent here, and we can likely see similar legislation crop up in other parts of the world. It's possible that the EU AI Act could be a model for international discussions about content ethics and regulations in the years to come.
AI-Powered PDF Translation now with improved handling of scanned contents, handwriting, charts, diagrams, tables and drawings. Fast, Cheap, and Accurate! (Get started for free)
More Posts from aitranslations.io: