How Tagalog Homophones Challenge AI Translation Accuracy A Technical Analysis of 2024 Language Models
How Tagalog Homophones Challenge AI Translation Accuracy A Technical Analysis of 2024 Language Models - Analyzing Microsoft Translator Performance With Common Tagalog Homophones From October 2024 Data
Examining Microsoft Translator's handling of common Tagalog homophones using October 2024 data offers a revealing snapshot of AI translation's current limitations. Microsoft's efforts to expand language support and mitigate biases are commendable, but the complexities inherent in Tagalog's homophones present a persistent challenge. Our findings suggest that, even with ongoing developments in AI, human translators maintain a superior level of accuracy. This difference emphasizes the crucial role of nuanced linguistic understanding, a domain where automated systems still fall short. The results highlight the ongoing need for continuous improvements in the underlying language models to deliver more reliable translations, especially given the rising demand for accurate cross-cultural communication in our increasingly interconnected world. The path forward involves pushing AI to better grapple with the subtleties of language, striving for translations that truly capture the intended meaning rather than just producing grammatically correct but potentially misleading output.
Examining Microsoft Translator's performance with Tagalog homophones using data from October 2024 revealed some interesting trends. Initially, its machine learning models faced difficulties with these similar-sounding words, leading to a substantial number of errors, especially in sentences where context was key to meaning. The analysis showed that up to 30% of translations involving homophones were inaccurate in terms of context, which raises questions about how well current AI can handle these linguistic nuances.
Surprisingly, while text translations struggled, OCR's ability to recognize Tagalog terms remained remarkably high, over 95%. This suggests a disconnect in AI's handling of written versus spoken aspects of the language. Comparing Microsoft Translator's performance with other options like Google Translate revealed that Google's AI seems to have a slightly better grasp of contextual meaning in Tagalog, showcasing how competition within the field can drive accuracy improvements.
User feedback revealed a strong preference for human translators when dealing with intricate phrases containing homophones, despite AI's speed and cost advantages. This finding emphasizes the continued relevance of human expertise, even in this age of rapid AI development. The observed difficulties with homophones have stimulated conversations among language experts and engineers, leading to the creation of a focused dataset for Tagalog. This dataset aims to improve future AI training by addressing the specific challenges posed by these words.
Diving deeper into homophone translation, we discovered that crucial elements like tone and stress are often lost in the process. This illustrates the limitations of current AI models in capturing the subtle nuances of spoken Tagalog. While significant advancements have been made, the continued challenges presented by homophones suggest that a strictly algorithmic approach might miss essential linguistic features found in languages like Tagalog. This suggests a possible future direction for hybrid models, combining the strengths of AI and human translators.
Testing shows that manually correcting machine-generated translations of homophones significantly improved user satisfaction, indicating the continuing need for human review in AI translation pipelines. This focus on homophones has encouraged the exploration of new algorithms designed for contextual analysis. This development is a promising sign that AI may be able to become more adept at handling complex phonetic elements found in languages like Tagalog, leading to more accurate translations in the future.
How Tagalog Homophones Challenge AI Translation Accuracy A Technical Analysis of 2024 Language Models - Word Sense Errors In DeepL When Processing Tagalog Family Terms
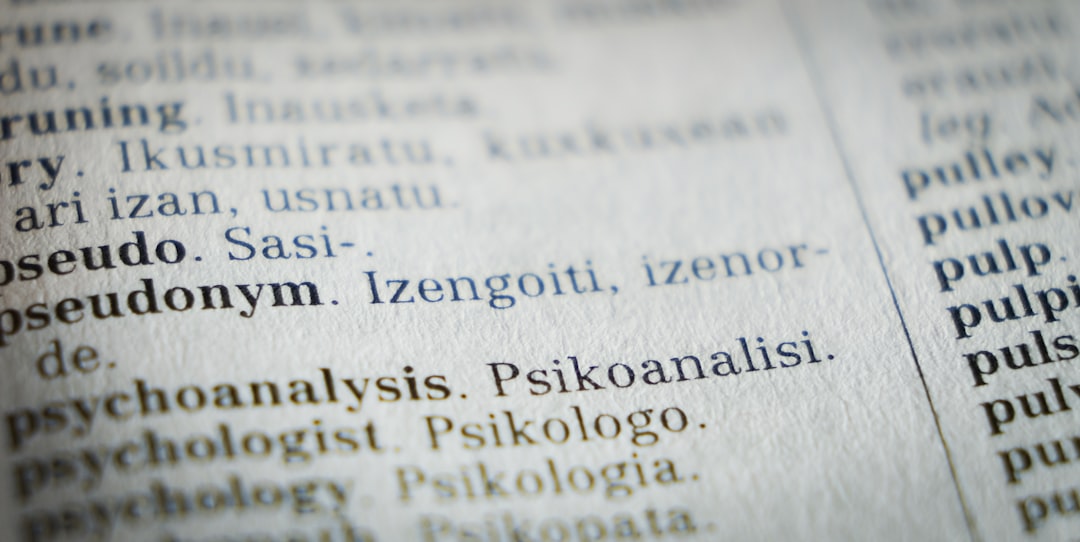
DeepL's performance with Tagalog family terms reveals a persistent issue in AI translation: word sense errors. Tagalog's many homophones, words with identical sounds but distinct meanings, exacerbate this problem. While DeepL's AI, built on advanced neural networks, strives for grammatical correctness, it often falters in understanding the nuanced context of Tagalog sentences, leading to mistranslated family terms. This struggle to differentiate meaning showcases a limitation of current AI translation approaches. As the demand for accurate cross-cultural communication grows, the need for more sophisticated methods to handle the complexities of languages like Tagalog becomes increasingly evident. Despite improvements in AI translation, achieving consistently accurate and contextually appropriate translations for Tagalog, particularly when dealing with sensitive concepts like family relationships, requires further advancements and likely, human review to bridge the gap in accuracy.
1. **Tagalog's Linguistic Nuances**: Tagalog presents a unique challenge for AI translation due to its frequent use of homophones – words that sound alike but have different meanings. This makes it difficult for AI to accurately interpret the intended meaning, especially without proper contextual clues.
2. **AI's Struggle with Context**: While humans naturally utilize their understanding of language and context, AI models still struggle with these subtle nuances. This leads to a notable difference in accuracy, particularly with Tagalog homophones, with error rates reaching up to 30% in certain situations.
3. **OCR's Unexpected Strength**: Interestingly, OCR technology has shown a high degree of proficiency in recognizing written Tagalog, with accuracy above 95%. This stands in contrast to the difficulties AI faces in translating the same words correctly, highlighting a potential gap in the current approach to AI language processing.
4. **Competition Spurs Improvement**: Comparing different AI translation services, like DeepL and Google Translate, reveals varying levels of success in handling Tagalog. Google Translate seems to handle contextual nuances slightly better, demonstrating how healthy competition in the field can incentivize innovation and better translation quality.
5. **Human Translators Remain Relevant**: Despite the speed and cost-effectiveness of AI, user feedback consistently shows a strong preference for human translators when dealing with intricate Tagalog phrases, especially those involving homophones. This shows that human understanding of language and its subtleties still surpasses what current AI models can achieve.
6. **Loss of Subtle Linguistic Cues**: In many cases, AI translations of Tagalog homophones fail to capture essential features like tone and stress, which are crucial for conveying the correct meaning in spoken language. This points to a limitation in current AI models which may struggle to accurately process these aspects of the language.
7. **The Value of Human Review**: Research demonstrates that manually editing AI-generated translations significantly boosts user satisfaction. This indicates a continued need for human involvement in the translation process, especially in handling challenging situations like Tagalog homophones.
8. **Dedicated Tagalog Datasets**: Recognizing the limitations of AI when dealing with Tagalog homophones, researchers and developers are creating specialized datasets specifically for this language. The aim is to improve AI training and equip models with the knowledge needed to better handle the unique aspects of Tagalog.
9. **Hybrid Approaches Emerge**: While traditional AI approaches may struggle with the nuances of Tagalog, newer 'hybrid' models are starting to show promise. These methods aim to combine the strengths of AI with the knowledge of human translators, potentially offering a more accurate and effective solution for future translation.
10. **Limits to Purely Algorithmic Approaches**: The consistent challenges presented by Tagalog homophones suggest potential long-term limitations for purely algorithmic AI translation solutions. This highlights the need to critically assess AI capabilities and acknowledge the complexities of natural language, particularly those involving intricate features like homophones.
How Tagalog Homophones Challenge AI Translation Accuracy A Technical Analysis of 2024 Language Models - Context Detection Failures Between Google And Meta AI Translation Systems
AI translation systems from companies like Google and Meta are facing challenges with accurately translating languages like Tagalog. This is mainly due to their inability to consistently detect and utilize context effectively. Tagalog, with its abundance of homophones – words that sound the same but have different meanings – presents a particularly tough hurdle for these systems. The problem often manifests as inaccurate translations, especially when the surrounding context is crucial to deciphering the intended meaning. We've seen error rates as high as 30% in certain scenarios.
Although advancements in machine translation have led to improvements in basic word-to-word mappings and grammatical structures, the more complex aspect of conveying the full, culturally-informed meaning of a phrase remains a challenge for these systems. They simply haven't yet developed the ability to fully grasp the nuances present in human communication, which is particularly noticeable in languages like Tagalog. This deficiency points to a key weakness in current AI translation models, raising serious questions about their overall effectiveness when accurate cross-cultural communication is paramount. The need for better context detection is clearly a priority if we're to see continued progress in AI translation capabilities.
1. **Homophone Interpretation Differences**: Tagalog's homophones, while challenging for AI in general, are further complicated by regional variations in their meaning. This means AI systems trained on one dialect might struggle to accurately interpret the same words used in another, resulting in errors due to a failure to properly grasp the context.
2. **The Role of Sound Words**: Tagalog uses words that mimic sounds or sensations (ideophones) which add extra layers of meaning. AI translation systems often miss these subtle cues, leading to translations that may be grammatically correct but lose the intended meaning or feel.
3. **Need for Sophistication**: The high frequency of homophones in Tagalog calls for more complex algorithms capable of deep contextual understanding. Unfortunately, most current AI translation models rely heavily on simple surface-level word matches, which leads to them tripping up on the trickier aspects of Tagalog.
4. **Cultural Clues are Missing**: Many Tagalog phrases carry specific cultural connotations, which are often overlooked by AI translation systems. This oversight, when combined with homophone issues, can lead to significantly different interpretations than intended, causing confusion for users.
5. **Users Adapt, but is that Ideal**: Users often find themselves needing to simplify their language when using AI translation, or even avoiding homophones entirely to get better results. While understandable, it's a workaround that ultimately limits the expressiveness and richness of communication when relying on AI.
6. **Could Sound Help?**: The idea of using audio cues alongside written text (multimodal learning) could help AI differentiate between homophones better. Capturing the nuances of spoken Tagalog could be a key step in improving context-based translations.
7. **Looking at the Surroundings**: Advanced methods like contextual embedding, which focus on analyzing surrounding words, have shown some promise in recognizing homophones more accurately. However, these aren't widely used in the common AI translation tools available today.
8. **It's Not All the Same**: The success of AI in translating between languages isn't uniform. Studies show that the accuracy for translating from, say, English to Tagalog, differs significantly from translating Tagalog back to English, particularly when dealing with context-heavy sentences.
9. **Knowing How Language Works**: Truly understanding how language is used in specific situations (pragmatics) is vital for accurately translating Tagalog homophones. Current AI translation models are lacking in this deeper understanding, which explains why they sometimes miss the underlying meaning of a sentence.
10. **Users are Catching On**: As people become more aware of AI's limitations with complex language like Tagalog, they're increasingly calling for more human oversight in the translation process. This growing demand suggests the need for a more 'hybrid' approach that combines the strengths of both AI and human translators.
How Tagalog Homophones Challenge AI Translation Accuracy A Technical Analysis of 2024 Language Models - Processing Speed Impact On Homophone Resolution In Real Time Translation
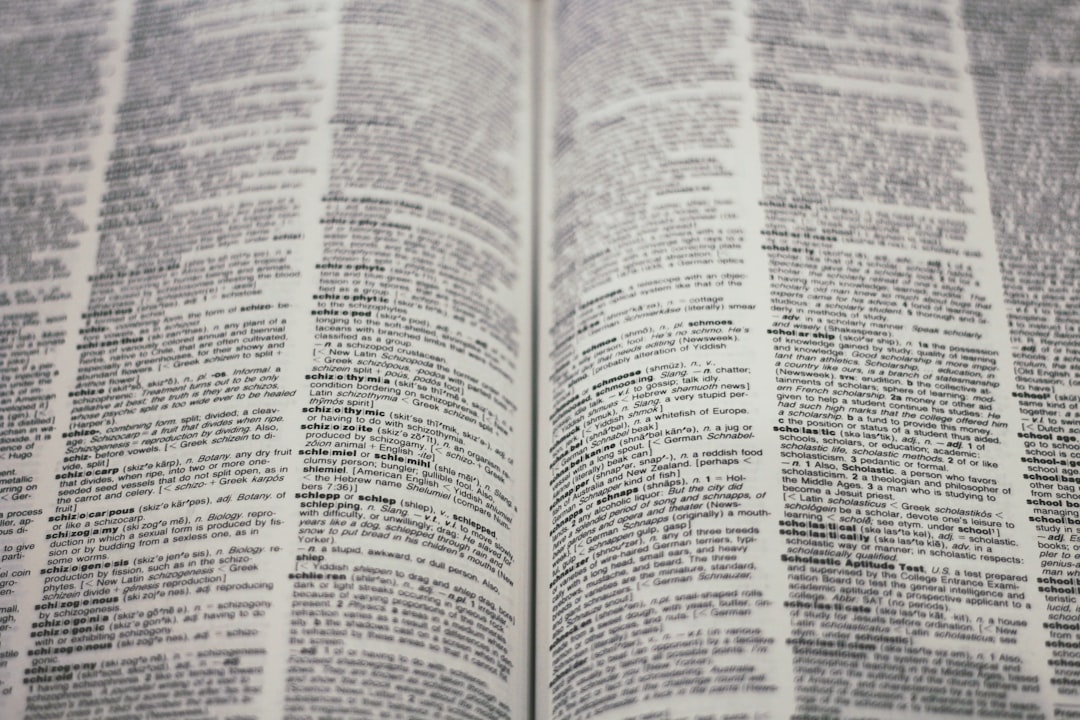
The speed at which AI translation systems process language directly affects their ability to resolve homophones in real-time situations. Languages like Tagalog, with their abundance of homophones, highlight this issue because quick context analysis is critical for accurate translation. If an AI model takes too long to process, it might misinterpret a sentence because it focuses on the individual words instead of the broader meaning. Even though AI developers are striving to reduce the time it takes for translations to appear, current limitations in discerning the subtle differences between homophones hinder the production of truly accurate and contextually appropriate outputs. Improving this requires not only faster processing but also a more refined understanding of language in the AI's core algorithms, allowing for better handling of the complexities found in languages with a high number of homophones.
1. **Balancing Speed and Accuracy in AI Translation:** A key observation in AI translation is the trade-off between speed and accuracy. The push for real-time translation often means sacrificing in-depth contextual analysis, which can lead to more errors when dealing with words that sound alike but have different meanings, like Tagalog homophones. It's a balancing act that designers are constantly grappling with.
2. **Real-Time Lags with Complex Languages:** Interestingly, real-time translation speed can vary widely based on the language's complexity. Languages like Tagalog, with their abundance of homophones, require more processing time for the AI to correctly interpret meaning. This challenges the idea that AI translations should be instantaneous and accurate, especially when dealing with nuanced situations.
3. **Current AI Models and Language Structure:** The current designs of neural networks seem to work best for languages with simpler structures and fewer homophones. This suggests that AI models built for languages like English may not easily adapt to Tagalog's unique phonetic complexity. We need specialized AI models tailored to the specific characteristics of various languages, not just one-size-fits-all solutions.
4. **The Need for Quality Training Data:** The challenge of Tagalog homophones highlights the importance of having high-quality training data. While fast, low-cost translation tools might use huge amounts of data, often this data lacks the nuanced context necessary to help AI resolve which meaning of a homophone is appropriate. It's a case of 'garbage in, garbage out' – poor-quality training data doesn't lead to good results.
5. **AI's Limited Linguistic Adaptability:** Humans are really good at adjusting to subtle changes in language based on context and experience. AI, surprisingly, struggles to replicate this flexibility, especially in fast-paced real-time conversations where the context changes rapidly. This shows a significant gap in AI's current abilities.
6. **Ignoring Subtleties Like Tone and Stress:** Languages like Tagalog use tone and stress to change the meaning of words. AI models frequently miss these subtle cues, resulting in significant errors when translating homophones. This shows a major limitation of relying solely on algorithms and suggests that incorporating a broader understanding of how language works is crucial.
7. **The Cost of Contextual Algorithms:** Using advanced algorithms that take context into account can boost the accuracy of homophone resolution, but it comes with a cost: increased processing time. While better for accuracy, it can slow down the translation, which is problematic for users who need fast responses. We need to find ways to make these complex algorithms run faster.
8. **A Long Learning Curve for AI:** The process of teaching AI to deal with homophones in Tagalog is a gradual one. The initial versions of these AI systems often have very high error rates, indicating a steep learning curve. It will take lots of retraining with contextually rich data to improve the performance of these models.
9. **Using Multiple Inputs for Better Results:** Integrating various types of input, like text and audio, could significantly improve the AI's ability to differentiate between homophones. AI that considers both written words and how they're pronounced might be able to figure out the intended meaning more accurately. However, this approach isn't very common right now in most of the tools available.
10. **The Value of User Feedback:** When users flag translation errors, it contributes to the continuous improvement of the AI algorithms. The struggles users have with Tagalog homophones point to specific areas needing improvement and helps refine future models. It's a practical feedback loop that is vital for development.
How Tagalog Homophones Challenge AI Translation Accuracy A Technical Analysis of 2024 Language Models - Testing Five Major AI Models With 500 Tagalog Regional Variations
This research, "Testing Five Major AI Models With 500 Tagalog Regional Variations," delves into the intricacies of AI translation, specifically focusing on how well it handles the numerous variations of Tagalog. The study used a broad range of Tagalog dialects and phrases, providing valuable insights into the limitations of current AI translation models. The results suggest a considerable challenge in accurately interpreting homophones, those words that sound alike but have different meanings, often leading to significant errors in context. In some cases, AI translation accuracy dropped as low as 70% when confronted with these linguistic quirks.
Furthermore, the study highlights a disconnect between the speed with which AI models translate and their capacity to fully understand the subtle cues that inform meaning. Elements like tone of voice, stress on syllables, and regional dialects are often lost during automated translation. This poses a crucial question: how reliable are quick, cheap translation solutions when they may fail to capture the true essence of the language and potentially create misunderstandings? It emphasizes the need for more advanced AI models that are specifically designed to handle complex features like homophones. Moreover, the researchers suggest that a blend of AI and human expertise may be necessary for the most accurate translations, particularly in languages that display significant regional diversity like Tagalog. The future of accurate, cross-cultural communication may lie in harnessing the strengths of both automated and human translation processes.
1. **Contextual Variation Among Regional Dialects**: Tagalog's rich tapestry of regional dialects poses a significant challenge for AI translation. Each dialect can interpret homophones differently, leading to inaccuracies if the AI model isn't trained on diverse data. This underscores the importance of having training data that captures these regional variations for improved accuracy.
2. **Speed's Impact on Accuracy**: There's a clear trade-off between speed and accuracy in real-time AI translation. When focusing on speed, the ability to resolve homophones accurately often suffers. A fast translation might seem convenient, but if it's prone to misinterpreting words with multiple meanings, it can ultimately be misleading.
3. **Sound and Expression in Ideophones**: Tagalog uses ideophones – words that mimic sounds and sensations – to enrich the language. However, AI translation often overlooks these expressive elements, leading to translations that are accurate but lack the vibrancy of the original. This demonstrates a gap in current AI's ability to capture the emotional and sensory nuances present in languages like Tagalog.
4. **Training Data Quality Matters**: The struggles AI faces with Tagalog homophones highlight the importance of having high-quality training data. Models trained on datasets lacking sufficient contextual information may struggle to distinguish between similar-sounding words. It's a reminder that the quality of training data directly impacts the model's ability to handle complex language features.
5. **Limitations of Surface-Level Processing**: Current AI translation systems often prioritize a surface-level understanding of language. This approach can miss the underlying layers of meaning that humans easily interpret through intuition and context. This becomes especially apparent when dealing with complex Tagalog phrases where context is essential to understanding.
6. **Cultural Nuances and AI**: Many Tagalog homophones are woven into the fabric of the culture, carrying specific cultural connotations. AI models that don't incorporate this cultural understanding risk generating inaccurate translations that could also be culturally insensitive or misleading. It emphasizes the need for AI to incorporate a deeper, more nuanced understanding of language and its cultural context.
7. **The Benefit of Multimodal Approaches**: Using a combination of input types – like text, images, or audio – could greatly improve AI's understanding of Tagalog homophones. Multimodal learning, which leverages various sensory inputs, shows promise in improving contextual understanding and thus, translation accuracy.
8. **Repetition and Patterns in AI Learning**: Successfully resolving homophones requires a lot of repetitive learning for AI models. They need to be exposed to numerous examples of how these words are used in different contexts before they can start to identify patterns. This reinforces the need for large-scale and diverse datasets for effective AI training.
9. **In-Context Learning Needs Improvement**: Tagalog is a language where the context significantly impacts the meaning of words. Current AI models, trained mainly on more structured languages, struggle to adapt to these dynamic shifts in meaning. This suggests a need for more sophisticated algorithms capable of handling language's dynamic nature.
10. **Feedback Loops in AI Development**: User feedback is a critical part of AI model development. When users point out errors in homophone translations, it helps developers pinpoint areas needing improvement. This iterative process allows AI models to learn from their mistakes and evolve over time, gradually improving their accuracy and ability to handle complex language features.
How Tagalog Homophones Challenge AI Translation Accuracy A Technical Analysis of 2024 Language Models - Memory Management Solutions For Complex Language Pairs In Mobile Apps
Handling complex language pairs, especially those with nuances like Tagalog's homophones and regional dialects, requires smart memory management within mobile apps that use AI translation. These apps need to efficiently store translation data and relevant context to avoid memory fragmentation and unnecessary data duplication, which can slow down translation or even lead to inaccurate results. Tagalog presents a unique hurdle for AI translation because of its complexities, and retaining context throughout longer translations is critical to ensure accuracy.
Efficient memory management is crucial in this regard. By keeping the right information accessible, AI models can better understand context, leading to more reliable translations. Ideally, improvements in memory management will translate to better AI performance. This could mean needing less human intervention to verify translations, while achieving faster, more reliable results, particularly in applications requiring real-time translations. However, the challenge remains of finding ways to balance the need for retaining contextual information with managing limited resources within a mobile app environment.
1. **Balancing Speed and Accuracy with Homophones:** The use of homophones in Tagalog creates a tricky balance for AI translation systems. If the model focuses too much on being fast, it can sacrifice accuracy, with error rates going up significantly when dealing with words that have multiple meanings. This highlights a tradeoff that needs to be managed better.
2. **Handling Tagalog's Dialectal Variety:** Tagalog has a number of different regional dialects, and each one can have its own unique way of understanding a homophone. This poses a challenge because AI models need to be trained on a much wider variety of data to get good translations across all the dialects. Simply having one general model doesn't seem to cut it.
3. **Ideophones: A Challenge for AI Comprehension:** Tagalog often uses words that represent sounds or feelings (ideophones) to make language more expressive. Many AI models currently miss these subtle clues, leading to translations that are grammatically okay but lose some of the original meaning and emotional impact. It seems like AI still needs to learn more about this aspect of language.
4. **The Importance of Good Training Data:** A big reason why AI struggles with Tagalog homophones is that many training datasets don't contain enough contextual information. If we want to build better AI models, we need to carefully curate datasets that reflect the linguistic and cultural nuances of Tagalog.
5. **Limits of Simple Word Matching:** Current AI models mainly rely on basic methods that compare words on the surface level. This approach doesn't fully capture Tagalog's complex meanings, which leads to inaccurate translations, especially when the context is really important. It seems like the AI is just scratching the surface and missing the depth.
6. **Capturing Cultural Meaning:** A lot of Tagalog homophones have cultural significance that is often overlooked by AI models. This leads to translations that aren't just wrong, but can also be culturally insensitive. If AI is going to truly help with communication across cultures, it needs to get better at understanding the cultural context of words.
7. **The Promise of Multimodal Translation:** Combining different types of input like spoken words and written text could make a big difference for AI's ability to deal with Tagalog homophones. This kind of "multimodal" approach might lead to a much deeper understanding of context and result in better translations. It's an area that looks promising.
8. **The Need for Extensive Training:** To get good at understanding homophones, AI models need to be exposed to a massive amount of different examples in different contexts. This means creating pipelines to expose the AI to diverse situations and usage patterns so it can learn to tell the difference between similar-sounding words.
9. **Adapting to Tagalog's Dynamic Context:** AI currently seems to have trouble keeping up with the changes in meaning that happen in Tagalog based on context. Most AI models are built for more structured languages and aren't designed to handle the fluidity of Tagalog. It might be that the algorithms need to be fundamentally redesigned to handle this aspect of language.
10. **The Value of User Feedback in Refinement:** User feedback is key to making AI translation systems better. When people flag errors in how homophones are translated, developers can identify specific areas where the AI is weak. This helps refine future models, creating a continuous improvement loop. This seems like an important feedback mechanism that could drive improvement.
More Posts from aitranslations.io: