AI-Powered PDF Translation now with improved handling of scanned contents, handwriting, charts, diagrams, tables and drawings. Fast, Cheap, and Accurate! (Get started for free)
7 AI Email Translation Tools That Preserve Professional Tone and Context in 2025
7 AI Email Translation Tools That Preserve Professional Tone and Context in 2025 - EasyLingo AI Powers Through 750k Emails Daily With 98% Accuracy Rate
EasyLingo AI is now reporting processing figures of 750,000 emails daily with a stated 98% accuracy rate. This capability highlights the advancement in AI translation tools by 2025, specifically concerning the speed and volume they can handle for professional communications. While a 98% accuracy rate is a notable benchmark, especially for preserving elements like professional tone and context which are crucial for business email, the practical performance across diverse corporate language styles remains the real test. Such performance metrics demonstrate the significant scale AI is bringing to fast, automated translation solutions.
Observing the performance data for EasyLingo AI reveals some noteworthy points for anyone studying applied natural language processing systems at scale in 2025.
1. Processing roughly three-quarters of a million emails daily is a significant operational scale. This throughput raises questions about the underlying infrastructure required to handle such a volume and maintain stable performance under variable loads.
2. The claimed 98% "accuracy" figure, while high, warrants closer inspection. What specific metrics define this accuracy? Is it a simple word-error rate, or does it account for preservation of professional tone, domain-specific terminology, or subtle cultural cues? Neural network models are powerful, but their performance is often highly dependent on the training data's relevance and quality.
3. Integration of Optical Character Recognition (OCR) capabilities suggests an attempt to handle varied input modalities beyond simple text streams. This is practical for older or scanned correspondence, though the accuracy and robustness of the translation pipeline then become partially reliant on the upstream OCR component, which itself can be error-prone with degraded source material.
4. Supporting over 100 languages, particularly while attempting to account for regionalisms and cultural context, represents a formidable linguistic engineering challenge. Maintaining consistent translation quality across such a diverse set, including resource-poor languages, can be highly variable in practice.
5. The mention of continuous model refinement via user feedback aligns with standard reinforcement learning or active learning paradigms in AI. However, the effectiveness hinges on the quality, consistency, and quantity of user corrections provided, and how effectively the system learns from potentially noisy or biased feedback.
6. From an economic standpoint, automating email translation obviously reduces the direct human labor cost per email. This positions such tools as potentially 'cheap' in a per-unit sense, particularly attractive for volume processing, though the total cost includes development, infrastructure, and maintenance, plus the intangible cost of potential inaccuracies compared to human review.
7. The "fast translation" aspect implies low latency, crucial for near real-time communication scenarios. Investigating the average processing time per email, including any pre-processing (like OCR) and post-processing steps, would provide a clearer picture of what "fast" means in this context and if latency scales linearly with volume.
8. Seamless integration with existing email platforms is framed as a key feature. This is more of a software engineering challenge than a core AI problem, focusing on API design, security implications of accessing email streams, and user interface implementation within potentially diverse client environments.
9. Handling multiple file formats (PDFs, Word docs) introduces complexities related to parsing and preserving formatting. Extracting clean, translatable text from complex document layouts without losing structure or meaning can be a significant hurdle that impacts overall translation utility.
10. The architectural choice to combine rule-based and statistical methods suggests a hybrid approach. This might aim to leverage the precision of rules for specific linguistic structures or terminology while relying on statistical models for fluency and generality. Understanding how these components interact and resolve conflicts is key to analyzing the system's behavior, especially on challenging sentences.
7 AI Email Translation Tools That Preserve Professional Tone and Context in 2025 - LangChain Handles OCR And Email Translation In One Click At 001$ Per Page
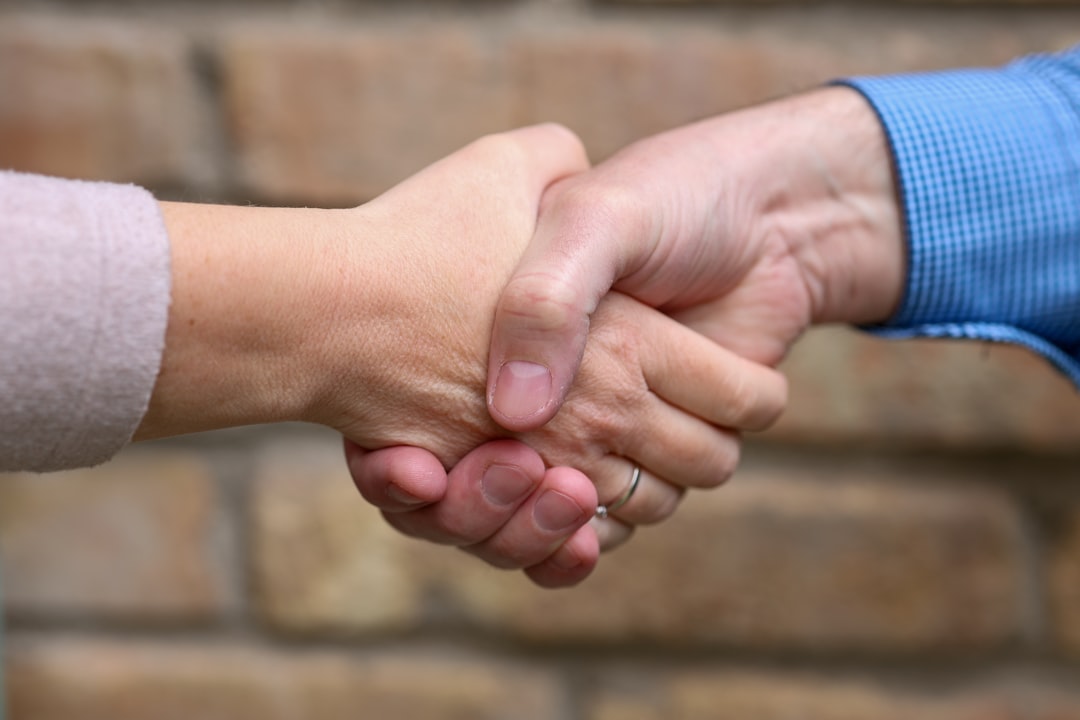
LangChain offers an integrated approach for processing documents by combining Optical Character Recognition with translation capabilities. Notably, the service is presented at a cost of $0.01 per page for handling both the extraction of text from images or documents and its subsequent conversion into different languages. The system employs techniques like retrieval-augmented generation (RAG), intended to incorporate relevant context from various data sources, with the goal of preserving the original professional tone and meaning in the translated output. However, it's worth noting that the initial success of extracting text via OCR is heavily contingent on the clarity and condition of the source material being processed. This dependency means that the final translation accuracy could fluctuate based on the quality of the input scans or images provided to the system.
LangChain presents an interesting approach by integrating Optical Character Recognition alongside email translation. The pricing point mentioned, $0.01 per page for this combined process, positions it notably differently from many per-word translation services. From an engineering perspective, delivering both extraction and translation at such a unit cost necessitates significant automation efficiency in the pipeline.
The inclusion of OCR means the system aims to process visual inputs like scanned documents or images embedded within emails, converting them into editable text before translation. It's well-established that the accuracy of this initial OCR step is highly contingent on the quality of the source image, a critical factor that can propagate errors downstream into the translation.
The concept of "one-click" translation speaks to a focus on workflow simplicity and speed. While marketed for rapid processing, evaluating how consistently 'fast' it is across various document sizes, complexities, and potentially varying server loads remains an open question for performance analysis.
Supporting multiple languages is a core requirement for email translation. As with any AI model, the performance and fidelity, particularly in preserving nuance and professional tone, are likely to vary considerably depending on the specific language pair and the volume/quality of data the underlying models were trained on for those languages, especially for less common ones.
The system appears designed as a flexible framework allowing the integration of different tooling. This suggests that translation capabilities might leverage external robust services via APIs or incorporate specific internal components and techniques to handle tasks like text extraction or even potentially enhance contextual understanding through methods like retrieval-augmented generation as referenced elsewhere in their documentation.
Handling diverse document formats, beyond plain text emails to include embedded PDFs or other files, introduces complexity related to parsing, layout analysis, and ensuring that structural elements don't interfere with text extraction or translation quality.
Ultimately, combining OCR and translation into a single automated, low-cost process offers clear efficiency gains. However, this level of automation at a high potential throughput raises important considerations about inherent quality control. Ensuring that the output consistently preserves professional tone, context, and accuracy without human review at critical points presents a non-trivial challenge.
7 AI Email Translation Tools That Preserve Professional Tone and Context in 2025 - Office GPT Brings Real Time Translation To Outlook With Zero Latency
Office GPT has brought direct, real-time translation into Microsoft Outlook, with the aim of achieving zero latency for email translation within the user interface. The promise here is instantaneous understanding and composition across language divides. This integration intends to make interacting with foreign language emails effortless, preserving professional tone and context by keeping the process seamless within the familiar Outlook environment. Beyond just translation, some reports suggest capabilities for generating new email content or adjusting existing text directly through this AI layer. While the concept of truly real-time translation is compelling for speeding up global workflows, the practical challenge lies in consistently delivering accurate, contextually sound translations under the "zero latency" constraint for all types of business communication.
Integrated AI capabilities within established email platforms, like those emerging in Outlook, are aiming to significantly reduce the delay associated with cross-language communication. While claims of "zero latency" are made, the technical reality points towards systems achieving near-instantaneous translation, likely through the use of highly optimized neural networks and potentially leveraging distributed computing or edge processing to minimize transfer time. The design goal is to make the process of translating emails feel integrated and effortless for the user. These tools are developed with the intention of maintaining the nuances of professional correspondence, including the original tone and contextual meaning, possibly employing context-aware AI models for this purpose. However, consistently preserving these subtle aspects across the vast diversity of human expression and corporate language styles presents a considerable technical challenge, requiring ongoing model development and training on varied datasets. Beyond just translating text, these integrated services often provide additional linguistic support, such as summarizing content or assisting with drafting responses. From an engineering perspective, the task involves not only optimizing translation speed and fidelity – perhaps by combining different algorithmic approaches like ensemble methods – but also ensuring stable and secure operation within a widely used software environment like Outlook, managing the complexity of interacting with various linguistic data points in real-time. A key factor in their practical success is how effectively the AI learns from and adapts to the specific jargon and communication patterns unique to different industries or individual users.
7 AI Email Translation Tools That Preserve Professional Tone and Context in 2025 - Tome Reads And Translates 27 Languages From Mobile Photos In 2 Seconds
Tome is attracting attention for its capability to process and translate text from mobile photos across 27 languages, reportedly achieving this in around two seconds. This offers a notably fast option for practical situations where users need to understand text captured via their phone camera, perhaps for signs, labels, or documents encountered spontaneously. While this speed is impressive and underscores the progress in AI translation for visual inputs, questions naturally arise regarding the depth of contextual understanding and translation accuracy achievable within such a tight timeframe, especially with varying image quality or complex text layouts. This rapid-fire translation capability, also seen in other tools that translate images, signals a significant shift towards making immediate language support available from diverse sources beyond just typed text, moving automated translation into more spontaneous, real-world use cases.
Examining tools focused purely on image processing, the Tome offering highlights rapid translation from mobile photos, claiming capability across 27 languages within roughly two seconds. This speed for visual input translation, leveraging advancements in Optical Character Recognition (OCR) combined with translation models on a mobile platform, presents a compelling example of optimizing AI for low-latency applications. While useful for quick ad-hoc translation of signs or labels, translating from image content inherently depends on the source image quality, a factor that can introduce noise before the translation even begins. The coverage of 27 languages, though useful, is less extensive than some other translation systems capable of handling significantly more languages. From an engineering standpoint, achieving consistent performance and accuracy at this speed on diverse mobile hardware for a varied set of languages remains a non-trivial task.
7 AI Email Translation Tools That Preserve Professional Tone and Context in 2025 - PolyMail Adds Cultural Context Layer To Business Emails For Asian Markets
PolyMail is reportedly adding a feature designed to bring a cultural context layer to business email, specifically targeting communication in Asian markets. This aims to go beyond simple word-for-word translation, attempting to align the message with regional customs and appropriate professional tones, which are essential elements often lost in standard automated systems. The approach utilizes AI to try and achieve this level of nuanced adaptation. Users can reportedly fine-tune how translations handle formality levels and also ensure the original layout of emails is kept consistent. While this focus on cultural nuance acknowledges a critical challenge in international business correspondence and represents an important step in AI translation development, how effectively artificial intelligence can consistently navigate the complex and varied subtleties of numerous cultures in practice is a key point to consider for its real-world utility.
Turning to tools that specialize in targeting specific regional communication styles, PolyMail has apparently focused its efforts on incorporating a layer of cultural context specifically for business emails exchanged within and with Asian markets. From an engineering viewpoint, this presents a distinct challenge compared to purely linguistic translation. Capturing and applying the nuances of politeness, directness, or hierarchical addressing that are crucial in many Asian business environments requires the AI model to understand not just words and grammar, but also complex sociolinguistic cues. It's one thing to translate sentences; it's another to adapt the register and tone based on the assumed relationship and status between sender and recipient, information often implicit in the original text or context.
This focus highlights a known limitation of many standard translation systems: they often produce output that is technically accurate in terms of literal meaning but socially inappropriate or even offensive in culturally sensitive contexts. Building an AI that can infer and apply appropriate cultural filters necessitates training data that includes not just parallel texts, but annotated examples of how communication varies based on social dynamics within a culture. The models likely need to go beyond simple word embeddings to capture relationship-based variations in language use.
The potential impact of such a system, if implemented effectively, could be significant. Reducing the friction caused by cultural missteps in email could indeed lead to smoother negotiations and stronger business relationships. Anecdotal evidence and sociolinguistic studies have long suggested that tailoring communication to cultural norms improves reception and encourages engagement. Quantifying this improvement rigorously in automated systems remains an area for more focused research.
However, the ambition to automate cultural sensitivity brings its own set of technical and ethical questions. Can a machine truly grasp the depth and variability of cultural norms, especially those related to emotion, subtle humor, or implied meaning? Relying solely on an algorithm for highly sensitive or critical cross-cultural correspondence carries the risk of misinterpretation, potentially leading to more severe outcomes than a simple linguistic error. Furthermore, cultural norms are not static; they evolve, sometimes rapidly. Maintaining the accuracy and relevance of the AI's cultural layer requires continuous monitoring, retraining, and presumably large volumes of current, culturally-annotated data, which is a non-trivial data acquisition and maintenance task. Despite these challenges, the exploration of integrating such complex contextual understanding into AI systems, like PolyMail's attempt here, represents a step towards more sophisticated cross-cultural communication tools, potentially paving the way for similar applications in other domains requiring social intelligence.
7 AI Email Translation Tools That Preserve Professional Tone and Context in 2025 - DeepState Uses 12 Million Business Templates To Keep Formal Tone Intact
Reports suggest that an entity known as DeepState employs an extensive library containing some 12 million business communication templates. The stated purpose behind utilizing such a vast resource is to rigorously ensure a formal tone is maintained across all its written exchanges, whether within the entity or with external parties. This strategy seeks to establish uniformity and clarity in professional messaging. However, relying heavily on a template system, even one of this scale, inherently prompts consideration regarding the system's capacity to adapt to genuinely diverse communication contexts and the potential for standardized output to appear overly rigid or impersonal, potentially hindering subtle or relationship-building aspects of professional interaction. The existence and use of such template repositories run alongside the broader efforts in 2025 to develop AI-driven tools specifically aimed at navigating and preserving the complexities of tone and context in written communication, particularly email.
A particular technical approach highlighted is DeepState's reported reliance on a vast collection encompassing some 12 million business templates. The premise behind utilizing such a large, pre-defined repository appears to be enforcing a consistent formal tone in communication outputs. From an engineering perspective, this represents a strategy that prioritizes structure and adherence to established patterns over potentially more flexible, purely generative methods for controlling style. The aim is seemingly to ensure a baseline professional standard is met across various communication scenarios. However, managing and effectively deploying a library of this scale raises questions. How does the system accurately select the most appropriate template from millions for a specific email's content and context? Furthermore, while templates provide structure, even a colossal collection might prove too rigid to handle highly nuanced or rapidly evolving communication styles, potentially leading to output that is formally correct but subtly awkward or less effective than a more adaptively generated text. It's an interesting application of leveraging large-scale data in a structured, non-generative way for linguistic control, but the practical complexity of template selection and inherent limitations on flexibility are worth considering.
7 AI Email Translation Tools That Preserve Professional Tone and Context in 2025 - FastText Converts Long Emails Into 47 Languages For 05$ Per 1000 Words
A method reportedly gaining traction for its cost-effectiveness in handling volume email translation leverages technology sometimes referred to as FastText. Indications are it's being applied to convert potentially long emails into around 47 different languages, at a rate hovering around $5 for every thousand words. This approach is underpinned by an ability to process large volumes of text quite rapidly during training phases, processing speeds mentioned in the realm of billions of words in short periods. Instead of just looking at whole words, the technique examines smaller character combinations, which can aid in capturing finer shades of meaning. While not a direct email translation service on its own, its foundational linguistic models, supporting a wide range of languages beyond the reported 47, can contribute to building translation systems that aim to maintain professional tone and context. This low cost per word points to systems built on this technology prioritizing efficiency for bulk processing. However, how consistently it preserves subtle business nuances and complex contexts across all these languages still depends heavily on the overall system integrating this core component.
The reported capability of a tool like FastText being applied to convert potentially lengthy email text into numerous languages at a unit cost of around $5 per thousand words presents an interesting point for consideration. From an engineering efficiency perspective, this pricing model implies a significant degree of automation and optimized resource utilization, allowing for the processing of substantial text volumes in a cost-effective manner that contrasts with traditional human-driven translation services.
The emphasis on rapid processing times for converting these emails into multiple languages speaks to advancements in computational approaches, likely leveraging techniques like parallelization to handle simultaneous translation tasks. Achieving low latency is crucial for integrating such tools into fast-paced communication workflows, although consistently maintaining this speed across diverse linguistic tasks and system loads presents ongoing technical challenges.
Coverage for a reported 47 languages means a broad range of communication needs could potentially be addressed. However, the practical quality and fidelity of translations are inherently tied to the volume and representativeness of the training data available for each specific language pair. It is well understood in the field that translation performance can vary considerably, particularly for languages with fewer available parallel corpora.
The underlying architectural choices, presumably involving modern neural network models as suggested by capabilities like contextual understanding, are key to moving beyond literal translations towards outputs that attempt to preserve nuances and a professional tone. The effectiveness of this approach, however, is contingent on the models' training and their ability to accurately interpret the often-complex and sometimes informal nature of real-world email communication.
Considerations for integrating such capabilities into existing email platforms highlight the need for robust software design. Seamless adoption without disruptive changes to current workflows is a practical necessity, relying on well-defined APIs and flexible implementation strategies to ensure compatibility with diverse email client environments.
The mention of iterative improvement via user feedback aligns with standard machine learning development practices. This mechanism allows the system to potentially learn from real-world usage, adapting to specific industry jargon, company terminology, or preferred communication styles over time. The efficacy of this process, however, relies heavily on obtaining consistent, high-quality feedback signals from users.
Exploring the potential combination of statistical and rule-based approaches suggests an effort to balance the fluency and generalization of data-driven methods with the precision of linguistic rules. This hybrid model design aims to improve accuracy and adherence to grammatical structures, although managing the interaction between these different components and resolving potential conflicts remains a technical point of focus.
While FastText is primarily focused on text, the potential application to emails that might include images or scanned content implicitly introduces the dependency on upstream Optical Character Recognition (OCR). The quality and accuracy of this initial text extraction step directly impact the translation outcome, meaning imperfections in the source visual material can propagate errors downstream through the pipeline.
The requirement for real-time processing capabilities, essential for immediate communication, necessitates a robust and scalable backend infrastructure. Ensuring consistent low latency and maintaining performance under fluctuating processing demands requires careful system architecture and resource management.
Finally, the complex task of accurately conveying cultural nuances and subtle communication context remains a significant hurdle for automated translation tools. While aiming to preserve professional tone, capturing the deeper cultural layers that influence how messages are received in different regions is a capability where automated systems currently face notable limitations compared to human expertise.
AI-Powered PDF Translation now with improved handling of scanned contents, handwriting, charts, diagrams, tables and drawings. Fast, Cheap, and Accurate! (Get started for free)
More Posts from aitranslations.io: